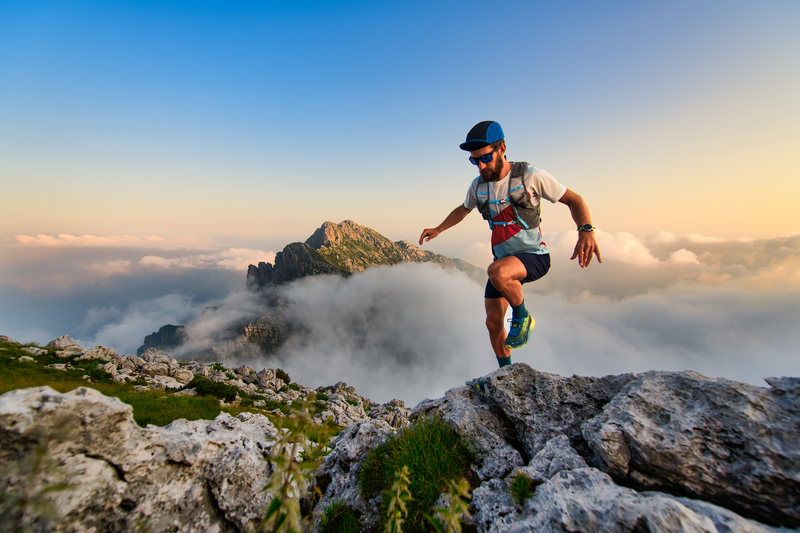
95% of researchers rate our articles as excellent or good
Learn more about the work of our research integrity team to safeguard the quality of each article we publish.
Find out more
ORIGINAL RESEARCH article
Front. Endocrinol. , 06 August 2024
Sec. Cancer Endocrinology
Volume 15 - 2024 | https://doi.org/10.3389/fendo.2024.1442740
This article is part of the Research Topic Obesity and Metabolism in Endocrine-Related Cancers View all 3 articles
Background: Obesity-induced metabolic dysfunction increases the risk of developing tumors, however, the relationship between metabolic obesity phenotypes and prostate cancer (PCa) remains unclear.
Methods: The term metabolic obesity phenotypes was introduced based on metabolic status and BMI categories. Participants were categorized into four groups: metabolically healthy nonobesity (MHNO), metabolically healthy obesity (MHO), metabolically unhealthy nonobesity (MUNO), and metabolically unhealthy obesity (MUO). Propensity score matching was conducted based on age, ethnicity, marriage, etc. Univariate and multivariate conditional logistic regression analyses were used to assess the relationship between metabolic obesity phenotypes, metabolic risk factors, and PCa. Sensitivity analysis was performed to verify the robustness of the results.
Results: After propensity score matching among 564 PCa patients and 1418 healthy individuals, 209 were selected for each of the case and control groups. There were no statistically significant differences in the basic characteristics between the two groups. Univariate and multivariate conditional logistic regression suggested that the risk of developing PCa in both MHO and MUO individuals was higher than in MHNO individuals. Specifically, the risk of developing PCa in MHO individuals was 2.166 times higher than in MHNO individuals (OR=2.166, 95%CI: 1.133-4.139), and the risk in MUO individuals was is 2.398 times higher than in MHNO individuals(OR=2.398, 95%CI:1.271-4.523). Individuals with hyperglycemia and elevated triglycerides also had a higher risk of developing PCa (hyperglycemia:OR=1.488, 95%CI: 1.001-2.210; elevated triglycerides: OR=2.292, 95%CI: 1.419-3.702). Those with more than or equal to three metabolic risk factors had an increased risk of PCa (OR=1.990, 95%CI: 1.166-3.396). Sensitivity analysis indicated an increased risk of PCa in MUO individuals compared to MHNO individuals.
Conclusion: In this retrospective study, individuals with MHO and MUO had a higher risk of developing PCa.
PCa is a complex, hormone-dependent disease and the most common malignant cancer in males (1). The development of PCa involves multiple factors, with recognized risk factors including age, smoking, family history, and genetic susceptibility/genetic variants (2, 3), a minority of patients may have both granulomatous prostatitis and PCa (4). Additionally, exogenous factors such as obesity and metabolic syndrome have been associated with the development and progression of PCa, as well as with an increased risk of adverse pathology (5). Obesity and overweight are growing public health problems driven by unhealthy eating patterns and lifestyles. Since 1980, approximately one-third of the global population has been classified as overweight or obesity (6).
Obesity is strongly linked to numerous metabolic abnormalities, including hyperinsulinemia, elevated free fatty acid levels, high triglycerides, and reduced high density lipoprotein cholesterol. The metabolism related thyroid hormones have an effect on both the tumour and its microenvironment, and involve in tumour growth, invasion and metastasis (7). The World Obesity Federation predicts that the prevalence of obesity will increase 24% by 2035, with nearly 2 billion people expected to suffer from metabolic disorders (8). As a metabolic pathogenic status, obesity is often accompanied by systemic chronic low-grade inflammation and elevated levels of immune markers such as leptin, IL-6, and TNF, which may influence tumor growth (9, 10). Metabolic dysfunction due to obesity increases cancer risk (11). PCa is considered one of the cancers potentially linked causally to obesity. While many studies have reported a correlation between obesity and PCa risk, the results have been conflicting (12–14). To resolve these conflicting findings, further investigation into the underlying mechanisms is necessary.
Obesity is increasingly considered a heterogeneous disease (15). There is evidence that some individuals with a BMI in the overweight or obese range do not exhibit metabolic disorders, maintaining normal blood glucose levels, insulin sensitivity, and low blood pressure (16, 17). Conversely, a significant proportion of individuals with a normal weight also present metabolic abnormalities (18). Metabolic dysfunction is recognized as a key risk factor for obesity-related cancers, regardless of the presence of obesity (11). Body mass index (BMI), as a solitary measure, does not distinguish between fat and muscle mass, and does not account for the increased risk associated with variations in body fat distribution. In contrast, metabolic health provides a comprehensive perspective on the body’s metabolic processes and overall physical health. Sims first introduced the concept of a metabolically healthy obesity (MHO) phenotype in 2001 (19). This concept integrates metabolic health and obesity, defining individuals with both metabolic unhealth and obesity as having metabolically unhealthy obesity (MUO) (20). Some studies have indicated that MHO individuals have a reduced cancer risk compared to MUO individuals, suggesting that an unhealthy metabolism may play a critical role in cancer development (21).
Previous literature has aimed to clarify the relationship between metabolic syndrome and PCa risk, identifying metabolic syndrome as a complex clinical feature characterized by obesity (22–24). While obesity is generally associated with a higher risk of most cancer types, a recent mendelian randomization study suggested that obesity might reduce PCa risk (25). Currently, the relationship between obesity, metabolic status, and PCa risk remains controversial. Thus, in this study, we evaluated the correlation between metabolic obesity phenotypes and PCa, to investigate whether these phenotypes influence the risk of developing PCa. The aim of this study was to evaluate the relationship between metabolic obesity phenotypes and PCa based on data from a large-scale general hospital in Xinjiang, and to identify the risk factors of developing PCa.
We studied 2917 subjects from the Department of Urology of the First Affiliated Hospital of Xinjiang Medical University from 2020 to 2023. A total of 1982 participants remained for analysis after the exclusion. This study included 564 patients with PCa confirmed by prostate biopsy and puncture, and 1,418 people who underwent physical examination at the physical examination center. After propensity score matching, 209 were selected for each of the case and control groups; Figure 1.
Inclusion criteria for control group: (1) males attending for the physical examination at the Physical Examination Centre of the First Affiliated Hospital of Xinjiang Medical University during the same period; (2) complete physical examination data; (3) can read, understand and sign the informed consent form.
Exclusion criteria for control group: (1) participants with any type of cancer or history of cancer; (2) participants with BMI <18.5Kg/m2.
Inclusion criteria for case group: (1) patients first diagnosed with PCa by prostate biopsy from 2020 to 2023; (2) complete test data; (3) can read, understand and sign the informed consent form.
Exclusion criteria for case group: (1) PCa patients with a history of other types of cancer; (2) patients with BMI <18.5Kg/m2.
The protocol was approved by the Ethics Committee of the First Affiliated Hospital of the Xinjiang Medical University (Approval No. 20220 308-166), and all study participants signed an informed consent form with a clear understanding of the purpose of the study protocol.
A physical examination was conducted to obtain anthropometric characteristics (height and weight), blood pressure, and blood sample data. Participants were asked to wear light clothing and have their height and weight measured while barefoot. Blood samples were collected early in the morning after fasting for 10-12 hours, using a vein in front of the elbow. These samples were analyzed for fasting fasting blood glucose, total cholesterol, triglycerides, high density lipoprotein cholesterol, low density lipoprotein cholesterol, creatinine, and uric acid. Laboratory technicians enzymatically analyzed all blood samples using an automated analyzer, with fasting blood glucose, triglycerides, and high density lipoprotein cholesterol measured by hexokinase, enzyme, and clearance methods, respectively.
According to the Adult Treatment Panel III (ATP-III) criteria, the International Diabetes Federation (IDF) consensus, and the JACC Health Promotion Series (26–28), we defined metabolic risk factors: (1) Elevated triglycerides (blood triglyceride≥1.7 mmol/L or 150 mg/dL). (2) Hypertension (systolic pressure≥130 mmHg or diastolic pressure≥85 mmHg). (3) Hyperglycemia (fasting blood glucose ≥ 5.6 mmol/L or 100mg/dL). (4) Reduced HDL-c (HDL-c <1.04 mmol/L or 40mg/dL). BMI (Kg/m2) is calculated based on weight (Kg) divided by the square of height (m). The study population was categorized according to the WHO criteria for Asian populations, BMI threshold is 25 Kg/m2, nonobesity defined as BMI<25Kg/m2 (normal weight: 18.5Kg/m2 ≤ BMI<23Kg/m2, overweight: 23Kg/m2 ≤ BMI<25Kg/m2), individuals with a BMI ≥ 25 Kg/m2 were classified as obesity (29, 30). Evidence suggests that there is a collinearity between waist circumference and BMI (31), therefore diagnostic criteria for metabolic status did not include waist circumference. Metabolically unhealthy status is defined as having two or more of the above metabolic risk factors. Subjects were categorized into four metabolic obesity phenotypes based on the combination of metabolic status and BMI: (1) Metabolically healthy nonobesity (MHNO): No or only one metabolic risk factor and BMI <25kg/m2. (2) Metabolically healthy obesity (MHO): No or only one metabolic risk factor and BMI ≥ 25 kg/m2. (3) Metabolically unhealthy nonobesity (MUNO): two or more metabolic risk factors and BMI<25kg/m2. (4) Metabolically unhealthy obesity (MUO): two or more metabolic risk factors and BMI ≥ 25 kg/m2.
PASS 15 software was used to calculate the sample size required for this study. For missing secondary study variables, we implemented multiple interpolation. Baseline information before propensity score matching were compared using t tests, Mann-Whitney U test, and χ2 test. Nearest neighbor matching propensity score matching in a 1:1 ratio for case and control groups, caliper value of 0.05, to reduce the interference of confounding factors. Baseline information after propensity score matching were compared using paired t-test, paired rank sum test, and paired χ2 test. Association between metabolic obesity phenotypes, number of metabolic risk factors and the risk of developing PCa were assessed by univariate and multivariate conditional logistic regression. Sensitivity analysis to ensure the robustness of the results. All statistical analyses were conducted using SPSS and R (version 4.2.0). All statistical tests were 2-sided, with P < 0.05 considered statistically significant.
Before propensity score matching, there were 564 PCa patients in the case group and 1,418 participants in the control group who participated in the physical examination. The median age of the case and control groups were 72 and 44 years, respectively. The metabolic obesity phenotypes were statistically different between two groups (P < 0.05); Table 1.
We calculated that the required sample size after propensity score matching on both the case and control groups was 175 (32, 33). After propensity score matching, 209 PCa patients and 209 non-PCa participants were included in the case and control groups. The mean age of the case and control groups was 67.19 and 67.05. There were no statistically significant differences between the two groups in the basic characteristics of age, race and marital status (P > 0.05). The metabolic obesity phenotypes were statistically different between two groups (P < 0.05); Table 2.
After propensity score matching, univariate conditional logistic regression analysis showed that the risk of developing PCa in both MHO and MUO individuals was higher than in MHNO individuals (MHO: OR=1.855,95%CI: 1.007-3.417; MUO: OR=2.310,95%CI: 1.289-4.140). Albumin, globulin and alkaline phosphatase were positively associated with the risk of developing PCa; Table 3.
Table 3 Impact of metabolic obesity phenotypes on the risk of developing PCa after propensity score matching.
The factors included in the univariate conditional logistic regression that were statistically significant (P < 0.05) were followed by multivariate conditional logistic regression. The results demonstrated that the risk of developing PCa was 2.166 times higher in MHO individuals than in MHNO individuals (OR=2.166, 95%CI: 1.133-4.139), and the risk in MUO individuals was 2.398 times higher than in MHNO individuals (OR=2.398, 95%CI: 1.271-4.523). Globulin and alkaline phosphatase were risk factors of developing PCa. Specifically, there was a 7.2% increase in PCa risk for every 1-unit rise in globulin (OR=1.072, 95%CI: 1.006-1.143). Additionally, for every 1-unit increase in alkaline phosphatase, there was a 0.9% increase in the risk of developing PCa (OR=1.009, 95%CI: 1.004-1.015); Table 3.
As mentioned above, we defined metabolic risk factors as hypertension, hyperglycemia, elevated triglycerides, and reduced HDL-c. The results indicated that hyperglycemia and elevated triglycerides were risk factors of PCa. The risk of developing PCa in individuals with hyperglycemia was 1.488 times higher than in individuals with non-hyperglycemia (OR=1.488, 95%CI: 1.001-2.210). Additionally, the risk of developing PCa in individuals with elevated triglycerides was 2.292 times higher than in individuals with non-elevated triglycerides (OR=2.292, 95%CI: 1.419-3.702). There were no statistically significant differences between other metabolic risk factors and PCa (P>0.05); Table 4.
The risk of developing PCa individuals with more than or equal to three metabolic risk factors was 1.990 times higher than those with less than or equal to one metabolic risk factor (OR=1.990, 95%CI: 1.166-3.396). With the number of metabolic risk factors increasing, the risk of developing PCa also increases; Table 5.
To demonstrate the robustness of the results, we performed sensitivity analyses. The results of the sensitivity analyses showed that our results were stable in the various populations.
We use different criteria to define obesity, BMI classification according to the Chinese Obesity Working Group (34). BMI≥28Kg/m2 was considered obesity. Re-analysis of the relationship between metabolic obesity phenotypes and the risk of developing PCa, to ensure robustness of results. The results showed that the risk of developing PCa was 4.598 times higher in MHO individuals than in MHNO individuals (OR= 4.598, 95%CI: 1.476 -14.324), and the risk in MUO individuals was 3.296 times higher than in MHNO individuals (OR=3.296, 95%CI: 1.357 -8.007); Table 6.
In defining metabolic unhealthy status, hyperglycemia was considered one of the metabolic risk factors. We excluded participants with hypoglycemia (FPG <2.8 mmol/L) to account for the possibility that these individuals might have issues with their own blood glucose regulation. The results demonstrated that the risk of developing PCa in MUO individuals was 2.628 times higher than in MHNO individuals (OR= 2.628, 95%CI: 1.493- 4.626); Table 7.
The combination of a metabolically unhealthy status and obesity (MUO), exhibited a higher risk of PCa compared to other combinations of metabolic status and BMI. Epidemiological evidence strongly supports the association between obesity and metabolic abnormalities (35, 36). This link is due to excessive nutrient intake, which alters several metabolic pathways involved in the pathogenesis of obesity-related diseases, including adipotoxicity, inflammatory pathways, aberrant adipokine expression, and adipose tissue senescence (37). Both metabolic status and obesity are strongly linked to inflammation (38), with chronic, low-grade inflammation playing a role in the pathogenesis of metabolic disorders (39). Obesity is characterized by the excessive accumulation of adipose tissue, which functions as a significant metabolic organ that secretes pro-and anti-inflammatory cytokines to modulate the inflammatory response (40). Increased proinflammatory cytokines in the adipose tissue of obese individuals reduce insulin sensitivity, leading to insulin resistance (41). Free fatty acids inhibit glucose transporter activity, reducing glucose uptake by muscle, and obesity can induce insulin resistance by increasing circulating free fatty acids (42). Gut leakage and microbiota dysbiosis in obese individuals result in elevated circulating levels of lipopolysaccharides, which are major contributors to adipose tissue inflammation and insulin resistance (43). Reduced extracellular matrix flexibility in adipose tissue is commonly associated with MUO (44). The expansion of adipose tissue is driven by the proliferation and hypertrophy of adipocytes and requires continuous remodeling of the extracellular matrix to accommodate this expansion (45). Adipose tissue fibrosis can lead to adipocyte apoptosis and chronic inflammation, characterized by infiltrating macrophages (46). Consequently, adipose tissue fibrosis with chronic inflammation is a hallmark of obesity-related metabolic disorders and a major cause of obesity-related metabolic dysfunction (47).
The risk of PCa was higher in both MHO and MUO individuals compared to MHNO individuals, suggesting that obesity plays a significant role in PCa. According to the World Health Organization (WHO), obesity results from a positive energy balance due to increased energy intake or decreased energy expenditure, leading to excessive fat accumulation in adipose tissue (48). Adipogenesis activation is a key marker of metabolic changes in tumor cells, and androgens, which are major regulators of PCa tumor cells, stimulate adipogenesis by interfering with the molecular mechanisms controlling cellular lipid homeostasis (49). Androgens cause a synergistic increase in the expression of several genes involved in triglyceride and cholesterol synthesis in various PCa cell lines. This effect is mediated by the androgen-induced activation of the secondary transcriptional regulator, sterol regulatory element-binding protein (SREBP). SREBP is crucial for intracellular lipid homeostasis and is involved in the synthesis of fatty acids and cholesterol (50). Elevated triglycerides, reduced HDL-c, and obesity are strongly associated with lower testosterone levels (51–54). The exact relationship between low testosterone and obesity or dyslipidemia is not fully understood. Some studies suggest that suppression of the hypothalamic-pituitary-gonadal (HPG) axis may be involved. Testosterone synthesis, regulated by the HPG axis, is reduced in the presence of obesity or dyslipidemia, and this reduction is linked to decreased insulin sensitivity and potential insulin resistance in HPG axis neurons (55). Visceral fat acts as an active endocrine tissue, and in patients with obesity or abnormal fat distribution, the secretion of adipose-specific cytokines (leptin, IL-6, TNF-α) is increased. These cytokines inhibit the secretion of gonadotropins and subsequently affect the HPG axis (56, 57). Low testosterone likely plays a role in regulating inflammation in various tissues, leading to increased levels of proinflammatory cytokines, which further inhibit testosterone release from the HPG axis (58). Previous studies have shown that obesity can lead to inflammation, and inflammation is attributed to overexpression of Monocyte Chemoattractant Protein-1 (MCP-1), which leads to defective insulin secretion, insulin resistance, and interference with other process of energy homeostasis. MCP-1 plays an important role in activating endothelial inflammatory markers, by stimulating IL-6 secretion and synthesis of Intercellular adhesion molecule-1 (ICAM-1) (59).
MUO individuals are at higher risk than MHO individuals, indicating that a metabolically unhealthy status increases the risk of developing PCa. Metabolically unhealthy patients exhibit higher levels of inflammation markers (IL-6, hs-CRP) and lymphocyte counts, and growing evidence suggests that inflammation influences PCa formation. Tumor-associated neutrophils, B cells, and complement components may promote PCa (60). The proportion of immune cell populations changes at different stages of PCa (61). Metabolic status affects HDL-c subclasses and is associated with insulin resistance and elevated triglycerides (62). Metabolic status changes and obesity may contribute to endothelial dysfunction. Metabolically unhealthy obesity (MUO) is associated with elevated biomarkers of endothelial dysfunction (63). One of the significant mechanisms for developing endothelial dysfunction in obese individuals is metabolic dysfunction, endothelial dysfunction is similarly one of the earliest vascular alterations observed in obesity as a result of obesity-related metabolic changes (64, 65). Hypertension, hyperglycemia, elevated triglycerides, and reduced high-density lipoprotein cholesterol (HDL-c) directly contribute to endothelial dysfunction (66). One study found that MHO might be a transient phenotype (36). A 20-year follow-up study revealed that about half of adults with MHO transitioned to MUO, only 10% of adults with MHO transitioned to MHNO, and adults with MHO were nearly eight times more likely to progress to MUO than adults with MHNO (67). Although MHO individuals are overweight, they still show a relatively beneficial hormonal and metabolic profile, with greater insulin sensitivity, a favorable immune profile, and reduced inflammation compared to the MUO population (18, 68).
This study has some limitations. Firstly, as a retrospective study, causal relationship between metabolic obesity phenotypes and PCa cannot be inferred, which limits the generalization of the results. The metabolic obesity phenotypes might change over time, indicating that physiological states at different time stages probably have an impact on the concentration of metabolic markers. Future prospective studies are needed to validate these findings. Secondly, we employ prostate puncture biopsy, and pathological tests to diagnose PCa, diagnostic methods are not sufficiently comprehensive. Finally, the criteria for measuring metabolically unhealthy status and obesity are not uniform and should be determined through further clinical trials. The advantage of this study is the use of propensity score matching, which reduces the effect of confounding factors, and the sample size calculation ensures sufficient certainty to detect statistical significance in the results.
In conclusion, both MHO and MUO individuals are associated with a higher risk of developing PCa compared to MHNO individuals. Although the risk of PCa in MHO individuals is lower than in MUO individuals, this indicates that obesity is still a risk factor for PCa in metabolically healthy status. This emphasizes the importance of different types of metabolic obesity phenotypes in assessing PCa risk. Metabolically unhealthy status and obesity may be valid targets for PCa prevention.
The original contributions presented in the study are included in the article/Supplementary Material. Further inquiries can be directed to the corresponding authors.
The studies involving humans were approved by Ethics Committee of the First Affiliated Hospital of the Xinjiang Medical University (Approval No. 20220 308-166). The studies were conducted in accordance with the local legislation and institutional requirements. The participants provided their written informed consent to participate in this study. Written informed consent was obtained from the individual(s) for the publication of any potentially identifiable images or data included in this article.
JW: Conceptualization, Formal analysis, Methodology, Software, Validation, Visualization, Writing – original draft. AA: Data curation, Formal analysis, Investigation, Writing – original draft. HQ: Data curation, Formal analysis, Software, Validation, Writing – original draft. NT: Conceptualization, Funding acquisition, Methodology, Supervision, Validation, Writing – review & editing. HA: Data curation, Funding acquisition, Methodology, Software, Supervision, Validation, Writing – review & editing.
The author(s) declare financial support was received for the research, authorship, and/or publication of this article. This work is supported by the Excellence Youth Science Foundation of Xinjiang Uygur Autonomous Region (Grant No.2023D01E05), National Natural Science Foundation of China (Grant No. 82360476), Key Projects of Xinjiang Uyghur Autonomous Region (Grant No. 2022D01D39), Xinjiang Uygur Autonomous Region “Tianshan Talents” youth science and technology top talent project (Grant No. 2022TSYCCX0026).
The authors thank all patients and research staffs who participated in this study, and also gratefully thank the nurses in the medical examination center for their help in the process of data collection.
The authors declare that the research was conducted in the absence of any commercial or financial relationships that could be construed as a potential conflict of interest.
All claims expressed in this article are solely those of the authors and do not necessarily represent those of their affiliated organizations, or those of the publisher, the editors and the reviewers. Any product that may be evaluated in this article, or claim that may be made by its manufacturer, is not guaranteed or endorsed by the publisher.
The Supplementary Material for this article can be found online at: https://www.frontiersin.org/articles/10.3389/fendo.2024.1442740/full#supplementary-material
1. Sung H, Ferlay J, Siegel RL, Laversanne M, Soerjomataram I, Jemal A, et al. Global cancer statistics 2020: GLOBOCAN estimates of incidence and mortality worldwide for 36 cancers in 185 countries. CA Cancer J Clin. (2021) 71:209–49. doi: 10.3322/caac.21660
2. Zi H, He SH, Leng XY, Xu XF, Huang Q, Weng H, et al. Global, regional, and national burden of kidney, bladder, and prostate cancers and their attributable risk factors, 1990-2019. Mil Med Res. (2021) 8:60. doi: 10.1186/s40779-021-00354-z
3. Bergengren O, Pekala KR, Matsoukas K, Fainberg J, Mungovan SF, Bratt O, et al. 2022 update on prostate cancer epidemiology and risk factors-A systematic review. Eur Urol Oncol. (2023) 84:191–206. doi: 10.1016/j.eururo.2023.04.021
4. Crocetto F, Barone B, De Luca L, Creta M. Granulomatous prostatitis: a challenging differential diagnosis to take into consideration. Future Oncol. (2020) 16:805–6. doi: 10.2217/fon-2020-0185
5. Gandaglia G, Leni R, Bray F, Fleshner N, Freedland SJ, Kibel A, et al. Epidemiology and prevention of prostate cancer. Eur Urol Oncol. (2021) 4:877–92. doi: 10.1016/j.euo.2021.09.006
6. Lin X, Li H. Obesity: epidemiology, pathophysiology, and therapeutics. Front Endocrinol (Lausanne). (2021) 12:706978. doi: 10.3389/fendo.2021.706978
7. Torabinejad S, Miro C, Barone B, Imbimbo C, Crocetto F, Dentice M. The androgen-thyroid hormone crosstalk in prostate cancer and the clinical implications. Eur Thyroid J. (2023) 12:e220228. doi: 10.1530/ETJ-22-0228
9. Gilbert CA, Slingerland JM. Cytokines, obesity, and cancer: new insights on mechanisms linking obesity to cancer risk and progression. Ann Rev Med. (2013) 64:45–7. doi: 10.1146/annurev-med-121211-091527
10. Fuster JJ, Ouchi N, Gokce N, Walsh K. Obesity-induced changes in adipose tissue microenvironment and their impact on cardiovascular disease. Circ Res. (2016) 118:1786–807. doi: 10.1161/CIRCRESAHA.115.306885
11. Karra P, Winn M, Pauleck S, Bulsiewicz-Jacobsen A, Peterson L, Coletta A, et al. Metabolic dysfunction and obesity-related cancer: Beyond obesity and metabolic syndrome. Obes (Silver Spring). (2022) 30:1323–34. doi: 10.1002/oby.23444
12. Pernar CH, Ebot EM, Wilson KM, Mucci LA. The epidemiology of prostate cancer. Cold Spring Harb Perspect Med. (2018) 8:a030361. doi: 10.1101/cshperspect.a030361
13. Hurwitz LM, Dogbe N, Barry KH, Koutros S, Berndt SI. Obesity and prostate cancer screening, incidence, and mortality in the Prostate, Lung, Colorectal, and Ovarian Cancer Screening Trial. J Natl Cancer Inst. (2023) 115:1506–14. doi: 10.1093/jnci/djad113
14. Shi X, Deng G, Wen H, Lin A, Wang H, Zhu L, et al. Role of body mass index and weight change in the risk of cancer: A systematic review and meta-analysis of 66 cohort studies. J Glob Health. (2024) 14:4067. doi: 10.7189/jogh.14.04067
15. Bonora E, Kiechl S, Willeit J, Oberhollenzer F, Egger G, Targher G, et al. Prevalence of insulin resistance in metabolic disorders: the Bruneck Study. Diabetes. (1998) 47:1643–9. doi: 10.2337/diabetes.47.10.1643
16. Martin S, Cule M, Basty N, Tyrrell J, Beaumont RN, Wood AR, et al. Genetic evidence for different adiposity phenotypes and their opposing influences on ectopic fat and risk of cardiometabolic disease. Diabetes. (2021) 70:1843–56. doi: 10.2337/db21-0129
17. Paczkowska-Abdulsalam M, Kretowski A. Obesity, metabolic health and omics: Current status and future directions. World J Diabetes. (2021) 12:420–36. doi: 10.4239/wjd.v12.i4.420
18. Kwon BJ, Kim DW, Her SH, Kim DB, Jang SW, Cho EJ, et al. Metabolically obese status with normal weight is associated with both the prevalence and severity of angiographic coronary artery disease. Metabolism. (2013) 62:952–60. doi: 10.1016/j.metabol.2013.01.006
19. Sims EA. Are there persons who are obese, but metabolically healthy? Metab Clin Exp. (2001) 50:1499–504. doi: 10.1053/meta.2001.27213
20. Brandão I, Martins MJ, Monteiro R. Metabolically healthy obesity-heterogeneity in definitions and unconventional factors. Metabolites. (2020) 10:48. doi: 10.3390/metabo10020048
21. Zheng X, Peng R, Xu H, Lin T, Qiu S, Wei Q, et al. The association between metabolic status and risk of cancer among patients with obesity: metabolically healthy obesity vs. metabolically unhealthy obesity. Front Nutr. (2022) 9:783660. doi: 10.3389/fnut.2022.783660
22. Gacci M, Russo GI, De Nunzio C, Sebastianelli A, Salvi M, Vignozzi L, et al. Meta-analysis of metabolic syndrome and prostate cancer. Prostate Cancer Prostatic Dis. (2017) 20:146–55. doi: 10.1038/pcan.2017.1
23. Motterle G, DE Zorzi L, Zecchini G, Mandato FG, Ferraioli G, Bianco M, et al. Metabolic syndrome and risk of prostate cancer: a systematic review and meta-analysis. Panminerva Med. (2022) 64:337–43. doi: 10.23736/S0031-0808.21.04507-9
24. Hammarsten J, Damber JE, Haghsheno MA, Mellström D, Peeker R. A stage-dependent link between metabolic syndrome components and incident prostate cancer. Nat Rev Urol. (2018) 15:321–33. doi: 10.1038/nrurol.2018.8
25. Loh NY, Wang W, Noordam R, Christodoulides C. Obesity, fat distribution and risk of cancer in women and men: A Mendelian randomisation study. Nutrients. (2022) 14:5259. doi: 10.3390/nu14245259
26. Guerrero-Romero F, Rodríguez-Morán M. Concordance between the 2005 International Diabetes Federation definition for diagnosing metabolic syndrome with the National Cholesterol Education Program Adult Treatment Panel III and the World Health Organization definitions. Diabetes Care. (2005) 28:2588–9. doi: 10.2337/diacare.28.10.2588a
27. Expert Panel on Detection, Evaluation, and Treatment of High Blood Cholesterol in Adults. Executive summary of the third report of the national cholesterol education program (NCEP) expert panel on detection, evaluation, and treatment of high blood cholesterol in adults (Adult treatment panel III). JAMA. (2001) 285:2486–97. doi: 10.1001/jama.285.19.2486
28. Lavie CJ, Laddu D, Arena R, Ortega FB, Alpert MA, Kushner RF. Healthy weight and obesity prevention: JACC health promotion series. J Am Coll Cardiol. (2018) 72:1506–31. doi: 10.1016/j.jacc.2018.08.1037
29. WHO Western Pacific Region, International Association for the Study of Obesity, and International Obesity Taskforce. The Asian-Pacific Perspective: Redefining Obesity and Its Treatment. Geneva, Switzerland: WHO Western Pacific Region (2000).
30. WHO Expert Consultation. Appropriate body-mass index for Asian populations and its implications for policy and intervention strategies. Lancet. (2004) 363:157–63. doi: 10.1016/S0140-6736(03)15268-3
31. Hinnouho GM, Czernichow S, Dugravot A, Nabi H, Brunner EJ, Kivimaki M, et al. Metabolically healthy obesity and the risk of cardiovascular disease and type 2 diabetes: the Whitehall II cohort study. Eur Heart J. (2015) 36:551–9. doi: 10.1093/eurheartj/ehu123
32. Sun M, Fritz J, Häggström C, Bjørge T, Nagel G, Manjer J, et al. Metabolically (un)healthy obesity and risk of obesity-related cancers: a pooled study. J Natl Cancer Inst. (2023) 115:456–67. doi: 10.1093/jnci/djad008
33. Liu C, Wang C, Guan S, Liu H, Wu X, Zhang Z, et al. The prevalence of metabolically healthy and unhealthy obesity according to different criteria. Obes Facts. (2019) 12:78–90. doi: 10.1159/000495852
34. Zhou BF, Cooperative Meta-Analysis Group of the Working Group on Obesity in China. Predictive values of body mass index and waist circumference for risk factors of certain related diseases in Chinese adults–study on optimal cut-off points of body mass index and waist circumference in Chinese adults. BioMed Environ Sci. (2002) 15:83–96.
35. Bauer UE, Briss PA, Goodman RA, Bowman BA. Prevention of chronic disease in the 21st century: elimination of the leading preventable causes of premature death and disability in the USA. Lancet. (2014) 384:45–52. doi: 10.1016/S0140-6736(14)60648-6
36. Blüher M. Metabolically healthy obesity. Endocr Rev. (2020) 41:bnaa004. doi: 10.1210/endrev/bnaa004
37. Liu Z, Wu KKL, Jiang X, Xu A, Cheng KKY. The role of adipose tissue senescence in obesity- and ageing-related metabolic disorders. Clin Sci (Lond). (2020) 134:315–30. doi: 10.1042/CS20190966
38. González-Gil EM, Cadenas-Sanchez C, Santabárbara J, Bueno-Lozano G, Iglesia I, González-Gross M, et al. HELENA study group: Inflammation in metabolically healthy and metabolically abnormal adolescents: The HELENA study. Nutr Metab Cardiovasc Dis. (2018) 28:77–83. doi: 10.1016/j.numecd.2017.10.004
39. Esser N, Legrand-Poels S, Piette J, Scheen AJ, Paquot N. Inflammation as a link between obesity, metabolic syndrome and type 2 diabetes. Diabetes Res Clin Pract. (2014) 105:141–50. doi: 10.1016/j.diabres.2014.04.006
40. Tilg H, Moschen AR. Adipocytokines: mediators linking adipose tissue, inflammation and immunity. Nat Rev Immunol. (2006) 6:772–83. doi: 10.1038/nri1937
41. Wondmkun YT. Obesity, insulin resistance, and type 2 diabetes: associations and therapeutic implications. Diabetes Metab Syndr Obes. (2020) 13:3611–6. doi: 10.2147/DMSO.S275898
42. Oh YS, Bae GD, Baek DJ, Park EY, Jun HS. Fatty acid-induced lipotoxicity in pancreatic beta-cells during development of type 2 diabetes. Front Endocrinol (Lausanne). (2018) 9:384. doi: 10.3389/fendo.2018.00384
43. Cani PD, Bibiloni R, Knauf C, Waget A, Neyrinck AM, Delzenne NM, et al. Changes in gut microbiota control metabolic endotoxemia-induced inflammation in high-fat diet-induced obesity and diabetes in mice. Diabetes. (2008) 57:1470–81. doi: 10.2337/db07-1403
44. Smith GI, Mittendorfer B, Klein S. Metabolically healthy obesity: facts and fantasies. J Clin Invest. (2019) 129:3978–89. doi: 10.1172/JCI12918645
45. Sun K, Kusminski CM, Scherer PE. Adipose tissue remodeling and obesity. J Clin Invest. (2011) 121:2094–101. doi: 10.1172/JCI45887
46. Thomas D, Apovian C. Macrophage functions in lean and obese adipose tissue. Metabolism. (2017) 72:120–43. doi: 10.1016/j.metabol.2017.04.005
47. Crewe C, An YA, Scherer PE. The ominous triad of adipose tissue dysfunction: inflammation, fibrosis, and impaired angiogenesis. J Clin Invest. (2017) 127:74–82. doi: 10.1172/JCI88883
48. Obesity: preventing and managing the global epidemic: Report of a WHO consultation. World Health Organ Tech Rep Ser. (2000) 894:i–253.
49. Swinnen JV, Heemers H, van de Sande T, de Schrijver E, Brusselmans K, Heyns W, et al. Androgens, lipogenesis and prostate cancer. J Steroid Biochem Mol Biol. (2004) 92:273–9. doi: 10.1016/j.jsbmb.2004.10.013
50. Verhoeven G. Androgenen en verhoogde lipogenese in prostaatkanker. Cel-biologische en klinische perspectieven [Androgens and increased lipogenesis in prostate cancer. Cell biologic and clinical perspectives]. Verh K Acad Geneeskd Belg. (2002) 64:189–96.
51. Zmuda JM, Cauley JA, Kriska A, Glynn NW, Gutai JP, Kuller LH. Longitudinal relation between endogenous testosterone and cardiovascular disease risk factors in middle-aged men. A 13-year follow-up of former Multiple Risk Factor Intervention Trial participants. Am J Epidemiol. (1997) 146:609–17. doi: 10.1093/oxfordjournals.aje.a009326
52. Mohr BA, Bhasin S, Link CL, O’Donnell AB, McKinlay JB. The effect of changes in adiposity on testosterone levels in older men: longitudinal results from the Massachusetts Male Aging Study. Eur J Endocrinol. (2006) 155:443–52. doi: 10.1530/eje.1.02241
53. Derby CA, Zilber S, Brambilla D, Morales KH, McKinlay JB. Body mass index, waist circumference and waist to hip ratio and change in sex steroid hormones: the Massachusetts Male Ageing Study. Clin Endocrinol (Oxf). (2006) 65:125–31. doi: 10.1111/j.1365-2265.2006.02560.x
54. Mulligan T, Frick MF, Zuraw QC, Stemhagen A, McWhirter C. Prevalence of hypogonadism in males aged at least 45 years: the HIM study. Int J Clin Pract. (2006) 60:762–9. doi: 10.1111/j.1742-1241.2006.00992.x
55. Yu Y, Wang Y, Xu L, Li W, Wang Y. Combined obesity- and lipid-related indices are associated with hypogonadism in Chinese male patients with type 2 diabetes: a cross-sectional study. Front Endocrinol (Lausanne). (2024) 14:1319582. doi: 10.3389/fendo.2023.1319582
56. Grossmann M. Hypogonadism and male obesity: Focus on unresolved questions. Clin Endocrinol (Oxf). (2018) 89:11–21. doi: 10.1111/cen.13723
57. Makki K, Froguel P, Wolowczuk I. Adipose tissue in obesity-related inflammation and insulin resistance: cells, cytokines, and chemokines. ISRN Inflamm. (2013) 2013:139239. doi: 10.1155/2013/139239
58. Fallara G, Pozzi E, Corsini C, Belladelli F, Boeri L, Capogrosso P, et al. Morbidity and mortality in men: Role of androgens. Best Pract Res Clin Endocrinol Metab. (2022) 36:101662. doi: 10.1016/j.beem.2022.101662
59. Takada Y, Hisamatsu T, Kamada N, Kitazume MT, Honda H, Oshima Y, et al. Monocyte chemoattractant protein-1 contributes to gut homeostasis and intestinal inflammation by composition of IL-10-producing regulatory macrophage subset. J Immunol. (2010) 184:2671–6. doi: 10.4049/jimmunol.0804012
60. Fujita K, Hayashi T, Matsushita M, Uemura M, Nonomura N. Obesity, inflammation, and prostate cancer. J Clin Med. (2019) 8:201. doi: 10.3390/jcm8020201
61. Solinas C, Chanzá NM, Awada A, Scartozzi M. The immune infiltrate in prostate, bladder and testicular tumors: An old friend for new challenges. Cancer Treat Rev. (2017) 53:138–45. doi: 10.1016/j.ctrv.2016.12.004
62. Tang H, Xiang Z, Li L, Shao X, Zhou Q, You X, et al. Potential role of anti-inflammatory HDL subclasses in metabolic unhealth/obesity. Artif Cells Nanomed Biotechnol. (2021) 49:565–75. doi: 10.1080/21691401.2021.196179863
63. Liu M, Wang P, Xie P, Xu X, He L, Chen X, et al. Expression of ICAM-1 and E-selectin in different metabolic obesity phenotypes: discrepancy for endothelial dysfunction. J Endocrinol Invest. (2023) 46:2379–89. doi: 10.1007/s40618-023-02094-4
64. Kwaifa IK, Bahari H, Yong YK, Noor SM. Endothelial dysfunction in obesity-induced inflammation: molecular mechanisms and clinical implications. Biomolecules. (2020) 10:291. doi: 10.3390/biom10020291
65. Pi X, Xie L, Patterson C. Emerging roles of vascular endothelium in metabolic homeostasis. Circ Res. (2018) 123:477–94. doi: 10.1161/CIRCRESAHA.118.313237
66. Sena CM, Pereira AM, Seiça R. Endothelial dysfunction - a major mediator of diabetic vascular disease. Biochim Biophys Acta. (2013) 1832:2216–31. doi: 10.1016/j.bbadis.2013.08.006
67. Bell JA, Hamer M, Sabia S, Singh-Manoux A, Batty GD, Kivimaki M. The natural course of healthy obesity over 20 years. J Am Coll Cardiol. (2015) 65:101–2. doi: 10.1016/j.jacc.2014.09.077
Keywords: prostate cancer, metabolic obesity phenotypes, propensity score matching, metabolic risk factors, conditional logistic regression
Citation: Wang J, Apizi A, Qiu H, Tao N and An H (2024) Association between metabolic obesity phenotypes and the risk of developing prostate cancer: a propensity score matching study based on Xinjiang. Front. Endocrinol. 15:1442740. doi: 10.3389/fendo.2024.1442740
Received: 02 June 2024; Accepted: 19 July 2024;
Published: 06 August 2024.
Edited by:
Natalia Simona Pellegata, University of Pavia, ItalyReviewed by:
Biagio Barone, Azienda Ospedaliera di Caserta, ItalyCopyright © 2024 Wang, Apizi, Qiu, Tao and An. This is an open-access article distributed under the terms of the Creative Commons Attribution License (CC BY). The use, distribution or reproduction in other forums is permitted, provided the original author(s) and the copyright owner(s) are credited and that the original publication in this journal is cited, in accordance with accepted academic practice. No use, distribution or reproduction is permitted which does not comply with these terms.
*Correspondence: Ning Tao, Mzg1MTg0MTJAcXEuY29t; Hengqing An, OTI2OTczNUBxcS5jb20=
†These authors have contributed equally to this work
Disclaimer: All claims expressed in this article are solely those of the authors and do not necessarily represent those of their affiliated organizations, or those of the publisher, the editors and the reviewers. Any product that may be evaluated in this article or claim that may be made by its manufacturer is not guaranteed or endorsed by the publisher.
Research integrity at Frontiers
Learn more about the work of our research integrity team to safeguard the quality of each article we publish.