- 1Department of Scientific Research, Minzu Hospital of Guangxi Zhuang Autonomous Region, Nanning, China
- 2Department of Smart Health Elderly Care Services and Management, School of Nursing, Guangxi Health Science College, Nanning, China
- 3Department of Epidemiology and Health Statistics, School of Public Health, Guangxi Medical University, Nanning, China
- 4Department of Experimental Research, Guangxi Medical University Cancer Hospital, Nanning, China
Background: Body mass index (BMI) consistently correlates with the triglyceride-glucose (TyG) index, a marker of insulin resistance, which in turn is linked to heightened cardiovascular disease (CVD) risk. Thus, insulin resistance could potentially mediate the association between BMI and CVD risk. However, few studies have explored this mechanism in the general population.
Methods: We used data from the China Health and Retirement Longitudinal Study, which is an ongoing prospective cohort study. It initially enrolled 7233 middle-aged and older Chinese adults who were free of heart disease and stroke at baseline. The exposure variable was BMI. Incident CVD, defined as self-reported physician-diagnosed heart disease and stroke combined, served as the main outcome.
Results: Of the 7 233 participants (mean [SD] age, 58.93 [9.33] years), 3 415 (47.2%) were men. During the 7 years of follow-up, 1 411 incident CVD cases were identified. Both BMI and TyG index were associated with CVD risk (HR per 1-SD increase: BMI, 1.23; 95% CI, 1.17–1.29; TyG, 1.13; 95% CI, 1.07–1.19). The 4-way decomposition analysis show that, overweight increased CVD risk by 28% (HR [total association], 1.28; 95% CI, 1.14–1.45), with 18.1% (95% CI, 2.2%–34.0%) mediated by TyG index (HR [pure indirect association], 1.05; 95% CI, 1.02–1.09); while obesity increased CVD risk by 91% (HR [total association], 1.91; 95% CI, 1.63–2.23), with 9.5% (95% CI, 2.2%–16.7%) mediated by TyG index (HR [pure indirect association], 1.09; 95% CI, 1.03–1.15). No evidence suggested TyG index modified BMI’s association with incident CVD.
Conclusions: The study revealed that the TyG index was associated to CVD risk and acted as a small partial mediator in the relationship between BMI and CVD among middle-aged and older Chinese adults. Consequently, solely addressing insulin resistance might not significantly mitigate the impact of body weight on CVD. Thus, exploring alternative pathways and potential mediators of CVD risk becomes imperative.
Introduction
The ongoing challenge of cardiovascular disease (CVD) persists in its impact on global health, affecting both morbidity and mortality rates, and imposing a significant burden on healthcare systems and individual well-being (1, 2). In the last thirty years, the global burden of CVD has surged, with a 92.3% increase in total prevalent cases from 271 million to 523 million and a 53.7% rise in deaths from 12.1 million to 18.6 million between 1990 and 2019 (3). This upward trend is driven by factors such as an aging population and lifestyle changes, including a higher prevalence of obesity, hypertension, and diabetes (1, 4). Although recent studies suggest a potential slowdown in the rise of overweight and obesity in high-income countries (5, 6), there is mounting evidence indicating an acceleration of this epidemic in low- and middle-income countries (7, 8). Notably, obesity globally is associated to an elevated risk of CVD across the general population (9, 10).
Although hemodynamic and metabolic factors have been suggested as factors that influence the relationship between BMI and CVD, the exact mechanisms are not yet fully understood (9, 11). Reduced insulin sensitivity constitutes a potential constituent, as evidence suggests that oxidative stress and inflammation instigated by obesity are intricately associated with the emergence of both localized and systemic insulin resistance (12). Conversely, insulin resistance is implicated in endothelial dysfunction, fostering the development of atherosclerotic plaques through alteration of gene expression patterns related to the estrogen receptor, Hence, it could potentially play a substantial role in the pathogenesis of CVD (13). It is therefore possible that insulin resistance could exert an indirect influence on CVD through BMI. TyG (triglyceride-glucose) index has been verified as a straightforward indicator of insulin resistance based on the logarithmization of glucose levels and fasting triglyceride (14). There has been evidence of a correlation between this test and the euglycemic-hyperinsulinemic clamp test, as well as a similar validity to that of the insulin resistance index calculated from the homeostatic model assessment (15). Given its accessibility and reliable performance, it is convenient for epidemiological studies to use the TyG index to measure insulin resistance as a simple proxy.
Prior studies have combined TyG index and BMI as a TyG-BMI index to examine the association with CVD and outcomes (16–19). Yet, to our knowledge, only one study has formally investigated the TyG index’s role as a mediator in the connection between BMI and incident CVD within a community-based setting, with the majority of participants being coal miners (20). Limitations include population heterogeneity, absence of generalizable, or absence of interaction between BMI and TyG index.
Hence, the objective of this study was to examine whether TyG acts as a mediator or modifier in the relationship between BMI and incident CVD within the general population. Using a causal mediation approach, we disassembled the overall association of BMI with incident CVD into four components: (1) the association unaffected by mediation or interaction, (2) the association influenced solely by interaction, (3) the association driven solely by mediation, and (4) the association influenced by both mediation and interaction.
Methods
Study population
This cohort study represents a secondary analysis of the CHARLS dataset, which is an ongoing, nationally representative cohort study. Detailed information regarding the study design is available elsewhere (21, 22). In summary, the study recruited 17708 participants from June 2011 to March 2012. For the purpose of gathering information, participants underwent assessments using standardized questionnaires using a multistage stratified probability proportional-to-size sampling method. The baseline survey achieved an 80.5% response rate. Following the baseline assessment, participants underwent follow-up evaluations every 2 years.
All participants provided written informed consent to participate in the CHARLS study, which was approved by the institutional review board of Peking University. All study protocols were conducted in accordance with the principles outlined in the Declaration of Helsinki (23), and adherence to the Strengthening the Reporting of Observational Studies in Epidemiology (STROBE) reporting guideline was ensured for this study (24).
Assessment of exposure and mediator
The exposure variable in this study was BMI, calculated from height and weight measurements as weight in kilograms divided by height in meters squared. BMI was categorized according to the Chinese BMI classification (25) as follows: underweight (BMI <18.5 kg/m2), normal weight (BMI 18.5–23.9 kg/m2), overweight (BMI 24.0–27.9 kg/m2), and obesity (BMI ≥28 kg/m2). Trained nurses conducted the measurements of height and weight.
The mediator was TyG index, which was calculated as ln [fasting blood glucose (milligrams per deciliter) × triglycerides (milligrams per deciliter)/2) (14) and splitting into quartiles. A colorimetric enzyme assay was used at Capital Medical University’s Youanmen Clinical Laboratory to determine triglycerides and fasting blood glucose levels.
Ascertainment of outcome
The primary outcome was incident CVD, the secondary outcome were incident stroke events and incident heart disease events. Consistent with prior studies (21, 26, 27), the following standardized questions were used to assess CVD events: “Have you received a diagnosis from a doctor indicating that you have experienced a heart attack, coronary heart disease, angina, congestive heart failure, or any other heart-related conditions?” or “Have you been diagnosed by a doctor with having had a stroke?” Participants who reported either a stroke or heart disease during follow-up were categorized as having experienced a CVD event. The date of CVD diagnosis was recorded between the last interview and the one in which the CVD event was reported (21, 26, 27).
Covariates
At baseline, trained interviewers used a structured questionnaire to collect information included age, sex, living residence, marital status (categorized as married or other), and educational level (grouped into no formal education, primary school, middle or high school, and college or above), self-reported smoking and drinking status (classified as never, former, or current), self-reported physician-diagnosed medical conditions (including diabetes, hypertension, dyslipidemia, and kidney disease), and the use of medications for these conditions. Metabolic factors comprised fasting plasma glucose, total cholesterol, triglycerides, high-density lipoprotein cholesterol (HDL-C), low-density lipoprotein cholesterol (LDL-C), high-sensitivity C-reactive protein (hsCRP), and serum creatinine. The estimated glomerular filtration rate (eGFR) was calculated using the Chronic Kidney Disease Epidemiology Collaboration’s 2009 creatinine equation (28).
Chronic kidney disease was defined as eGFR <60 mL/min/1.73 m2 or self-reported history of chronic kidney disease. Diabetes was defined as fasting plasma glucose ≥126 mg/dL, current use of antidiabetic medication, or self-reported history of diabetes. Dyslipidemia was defined as total cholesterol ≥240 mg/dL, triglycerides ≥150 mg/dL, LDL-C ≥160 mg/dL, HDL-C <40 mg/dL, current use of lipid-lowering medication, or self-reported history of dyslipidemia. Hypertension was defined as systolic blood pressure ≥140 mmHg, diastolic blood pressure ≥90 mmHg, current use of the antihypertensive medication, or self-reported history of hypertension.
Statistical analysis
Descriptive statistics included mean ± standard deviation (SD) and median with interquartile range (IQR). Categorical variables were depicted as n(%). Baseline characteristics were stratified by TyG index quartiles and compared using appropriate tests: χ² test, analysis of variance, or Kruskal-Wallis rank sum test. Missing data were imputed using the multiple imputation of chained equations method.
We first evaluated the association of BMI (both as a linear term and classification) and TyG index (both linearly and in quartiles) with CVD using Cox proportional hazard models. Additionally, we explored linear trends through entering the median value of each BMI group or TyG index quartile to test association across the various BMI groups or TyG index quartiles. We then assessed the association of BMI with mediators using linear models. All models were adjusted for age, gender, marital status, residence, education level, smoking status, and drinking status. Subsequently, we applied 4-way decomposition causal mediation techniques to estimate the controlled direct association (CDA), reference interaction (INTref), mediated interaction (INTmed), and pure indirect association (PIA) individually (29). Utilizing the framework depicted in Figure 1 (30).
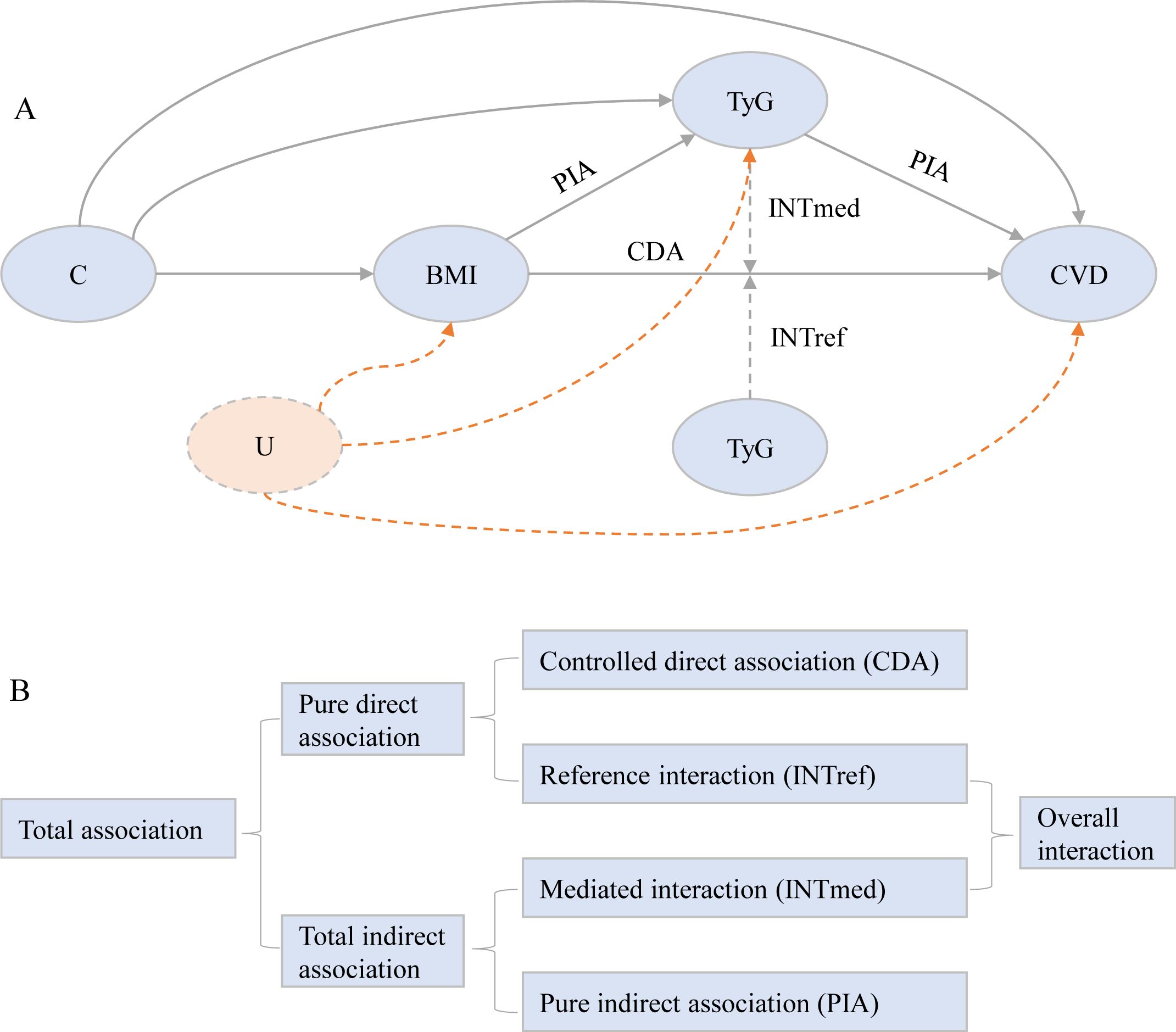
Figure 1. Conceptual model for the analysis of TyG index mediating the association of body mass index with incident cardiovascular disease. (A) The figure shows how TyG index could serve as a mediator of the association of body mass index with cardiovascular disease. The direct association between BMI and CVD is also caused by other potential mechanisms, such as hypertension, hypercholesterolemia, and/or diabetes. All statistical models were based on this structure and were adjusted for age, gender, marital status, residence, education level, smoking status, and drinking status. Following the theory of causal graphs, variables such as blood pressure (hypertension, systolic blood pressure, and diastolic blood pressure), cholesterol (total cholesterol, HDL-C, LDL-C, and dyslipidemia), renal function (kidney disease and eGFR), diabetes, glycated hemoglobin, and hsCRP represent alternative pathways that could potentially mediate aspects of the association between BMI and CVD. As such, these variables were not included as covariates in our models. C denotes the potential exposure-mediator, exposure-outcome, and mediator-outcome confounders. U denotes unmeasured confounding, which remains unavoidable in observational research settings. (B) Illustration of the 4-way decomposition of total association. The CDA is due to neither mediation nor interaction. The INTref is only due to interaction. The mediated INTmed is due to both mediation and interaction. PIA is only due to mediation. CDA, controlled direct association; INTref, reference interaction; INTmed, mediated interaction; PIA, pure indirect association; BMI, body mass index; CVD, cardiovascular disease; eGFR, estimated glomerular filtration ratio; HDL-C, high-density lipoprotein cholesterol; hsCRP, high-sensitivity C-reactive protein;IQR, interquartile range; LDL-C, low-density lipoprotein cholesterol; TyG, triglyceride-glucose.
To evaluate the indirect and direct association between BMI and CVD events, we utilized VanderWeele’s two-stage regression method for time-to-event data (29, 31). This approach involves fitting two regression models: one for the mediator (TyG index) and another for the outcome (CVD). The outcome (CVD) was modeled using a Cox model, while the mediator (TyG index) was modeled using a linear model. We also conducted similar mediation analyses using BMI categories, treating the TyG index as a linear indicator due to its confirmed linear association with CVD risk. All models were adjusted for age, gender, marital status, residence, education level, smoking status, and drinking status. Subsequently, we used the model parameters from these models to calculated the CDA, INTref, INTmed, and PIA, estimating the proportions of the total excess association attributable to each component according to VanderWeele’s derivations (29). The 95% CIs for estimates and proportion mediated were calculated by delta method (32).
We implemented several sensitivity analyses to assess the robustness. Initially, we conducted mediation analyses according to gender. Subsequently, we assessed our results using the complete dataset (6884 participants). Finally, we repeated the mediation analysis excluding participants with a BMI <18.5 kg/m² (6723 participants). We considered two-sided P < 0.05 as statistically significant. All analyses were carried out using R statistical software version 4.3.0 (R Foundation), and mediation analysis was performed using the CMAverse package developed by Baoyi Shi, Christine Choirat, and Linda Valeri (https://bs1125.github.io/CMAverse/index.html).
Results
Baseline characteristics
There were 17 708 participants at baseline, we excluded 777 participants below 45 years, 2 650 with baseline heart disease or stroke, 5 622 had no blood samples, 1 409 had incomplete TyG index or BMI, and 17 with extreme BMI values. Finally, 7 233 participants were included for analysis. Baseline characteristics between included and excluded participants is shown in Supplementary Table S1.
Of the 7 233 participants, the mean (SD) age at baseline was 58.93 (9.33) years; 3 415 (47.2%) were men. Participants’ characteristics are presented in Table 1. At baseline, 2 088 (28.9%) participants had overweight and 755 (10.4%) had obsity, the mean (SD) TyG index was 8.65 (0.65).
Risk of CVD by TyG index or BMI
Between 2011 and 2018, 1411 participants experienced CVD events, including 1 077 heart attacks and 464 strokes, a 19.5% incidence rate. In Table 2, we show how BMI and TyG index are related to CVD events after adjusting for potential confounders (model 2), by comparing to under and normal weight, obesity was associated with a 90.0% increased risk of incident CVD (CVD: adjusted HR, 1.90; 95% CI, 1.63–2.22; stroke: adjusted HR, 2.13; 95% CI, 1.63–2.78; heart disease: adjusted HR, 1.85; 95% CI, 1.55–2.20). When modeling the TyG index as quartiles, by comparing quartile 4 with quartile 1, the adjusted HRs were 1.35 (95% CI, 1.16–1.57) for incident CVD, 2.17 (95% CI, 1.63–2.88) for stroke, and 1.14 (95% CI, 0.96–1.35) for heart disease. BMI and CVD risk are linearly associated and positive (for trend, P <0.001 for CVD, stroke, and heart disease), as well as, the TyG indx (for trend, P <0.001 for CVD and stroke, P = 0.131 for heart disease).
Association of BMI and TyG index
Table 3 shows the associations of BMI with TyG index. After adjusting for potential confounders (in model 2), compared with participants with underweight and normal weight, participants with overweight and obesity had higher TyG index (overweight: adjusted β, 0.28; 95% CI, 0.25– 0.32; obesity: adjusted β, 0.47; 95% CI, 0.42–0.52).
Mediation and interaction analysis
Table 4 show the findings from 4-way decomposition model. Analysis by BMI categories yielded the adjusted HR for the total association of BMI with incident CVD was 1.28 for overweight vs the reference normal weight (CVD: adjusted HR, 1.28; 95% CI, 1.14–1.45; stroke: adjusted HR, 1.41; 95% CI, 1.15–1.74; heart disease: adjusted HR, 1.26; 95% CI, 1.10–1.45), which increased to 1.91 for the obesity group (CVD: adjusted HR, 1.91; 95% CI, 1.63–2.23; stroke: adjusted HR, 2.13; 95% CI, 1.63–2.78; heart disease: adjusted HR, 1.85; 95% CI, 1.55–2.21). The 4 components method show that when using TyG index as a mediator, There was no evidence that BMI interacted with TyG via INTref or INTmed, and the majority of the association was direct, with the remainder being purely indirect, the proportions mediated were 18.1% for overweight (CVD: 18.1%; 95% CI, 2.2%–34.0%; stroke: 30.3%; 95% CI, 4.1%–56.5%), and 9.5% for obesity (CVD: 9.5%; 95% CI, 2.2%–16.7%; stroke: 19.1%; 95% CI, 5.6%–32.7%). Notably, for heart disease, virtually all of the association was direct, no evidence of mediation and interaction.
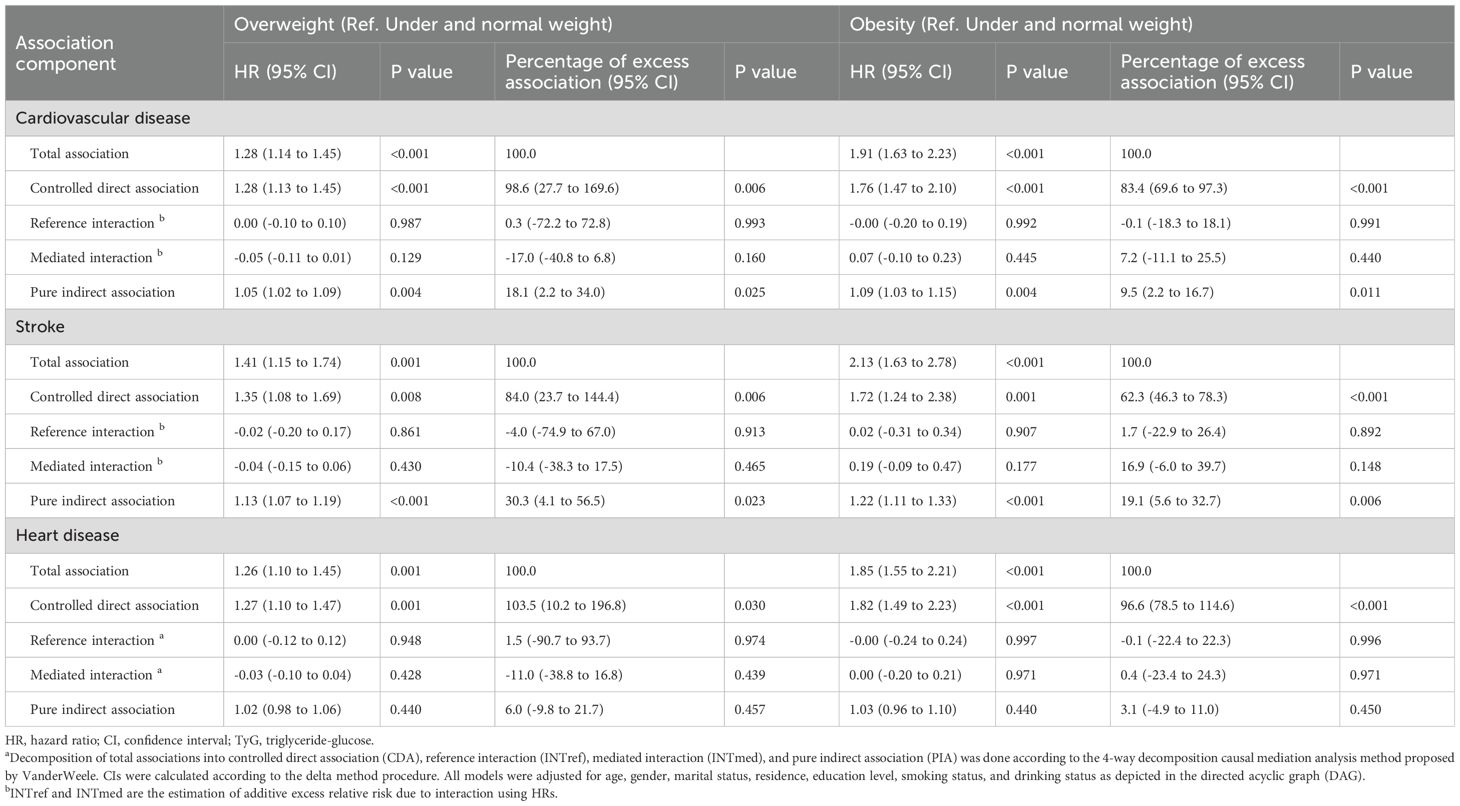
Table 4. Decomposition of the association of body mass index with incident cardiovascular disease including mediation and interaction associations by TyG index using causal mediation analysisa.
Subgroup and sensitivity analysis
Subgroup analysis among women (Supplementary Table S2), the proportions mediated of TyG index between BMI and CVD were increased (overweight, 37.5%; obesity, 12.3%), while the proportions mediated decreased (overweight, 6.2%; obesity, 4.6%) among men (Supplementary Table S3). Similar trends were observed in the complete data analysis (Supplementary Table S4). Moreover, the results remained consistent even after excluding participants with a BMI <18.5 kg/m2 (Supplementary Table S5).
Discussion
In this large cohort study, we found that the TyG index independently raised the risk of CVD. Additionally, a minor portion of the BMI-CVD association was mediated by the TyG index. Epidemiological studies consistently show a positive correlation between higher BMI and subsequent CVD risk (33–37). Our study findings align with these conclusions, revealing that an increase in BMI by per 1-SD increased the risk of CVD by 23%. Moreover, stratifying participants by BMI categories revealed a 28% increased CVD risk among overweight individuals and a nearly twofold elevation (HR, 1.90) among those with obesity compared to the baseline population of normal weight. Notably, individuals classified as overweight or obese exhibit a higher propensity for developing insulin resistance, signaling early signs of disrupted glucose metabolism (38, 39). Epidemiological study have showed a direct correlation between insulin resistance and CVD, which persists independently of diabetes and is aggravated when obesity (40). Thus, BMI and CVD risk may be mediated by insulin resistance.
Our study furnishes empirical evidence substantiating the biologically conceivable conjecture that insulin resistance serves as a pivotal intermediary in the linkage between obesity and CVD. We determined that the TyG index accounted for 18.1% of the mediating proportion in cases of overweight and 9.5% in instances of general obesity. Notably, insulin resistance frequently coexists with an array of traditional risk factors including dyslipidemia, glucose dysregulation, and hypertension, all of which have been corroborated in prior research as mediators in the causal pathway between obesity and CVD (41, 42). A retrospective cohort analysis including 6 078 participants aged 60 years and older elucidated that the TyG index served as a mediator in the relationship between BMI and CVD events. Previous studies have not firmly established insulin resistance’s role in BMI and CVD. However, a study involving 6078 participants aged ≥60 years showed that BMI and CVD events were the mediated by TyG index. But, the study did not furnish information regarding the proportion mediated (43). Another prospective cohort study of 94 136 participants in which most were coal miners revealed that TyG index was a mediator in the relationship between BMI and CVD events (proportion mediated: 47.81% for overweight, 37.94% for obesity) (20). Limitations include population heterogeneity, absence of generalizable, which results in a higher proportion of TyG mediation compared to our results. In contrast, our prospective analysis centered on the general population and employed a novel method to calculate the mediated proportion of TyG index. Collectively, our results, along with previous studies, suggest that controlling the TyG index may help mitigate the effects of BMI on CVD. However, this effect may not be pronounced in the Chinese general population.
The deleterious impact of BMI on CVD susceptibility is well-documented. The underlying pathophysiological mechanisms potentially involve several pathways. Adipose tissue expansion instigates heightened basal lipolysis, liberating free fatty acids (FFA), interleukins, and cytokines. These biochemical mediators contribute to cardiac dysfunction by expediting atherosclerotic progression and modulating factors implicated in inflammation, endothelial dysfunction, and coagulation abnormalities (44). Elevated FFA levels attributable to obesity precipitate insulin resistance, exacerbating impaired insulin signaling and attenuating insulin-mediated glucose uptake in skeletal muscle while augmenting hepatic glucose output (45). Moreover, a state of positive energy balance engenders adipocyte hypertrophy and ectopic fat deposition, fostering metabolic perturbations such as insulin resistance and beta-cell dysfunction (46). Additionally, the pro-inflammatory milieu associated with obesity potentiates lipolytic processes and hepatic triglyceride synthesis, exacerbating hyperlipidemia through heightened fatty acid esterification (13). Notably, insulin resistance constitutes a pivotal nexus in the interplay between obesity and CVD risk. Consequently, the TyG index emerges as a plausible intermediary linking obesity with heightened CVD susceptibility.
Our findings are notable as they stem from a comprehensive, representative cohort of the Chinese general population, with a prolonged follow-up period. This extended duration is crucial for meaningfully exploring longitudinal associations, especially those concerning obesity and CVD. Additionally, we applied a counterfactual framework to analyze mediation in an innovative way, our implementation of the 4-way decomposition approach enabled the simultaneous examination of the TyG index’s role as both modifiers and mediators.
However, our study also has limitations. First, we used BMI to ascertain overweight and obesity, while widely employed and easily calculable, offers a suboptimal estimate of fat mass proportion and distribution. There was a lack of alternative metrics, such as waist circumference (47), waist-to-hip ratio (47, 48), or body fat composition analysis (49), that could be used to quantify visceral fat more accurately. Second, the reliance on self-reporting for CVD diagnosis introduces a methodological challenge. While the CHARLS dataset lacks medical records, preventing the validation of self-reported CVD incidents, it’s important to acknowledge that other large-scale studies, like the English Longitudinal Study of Ageing, have demonstrated notable agreement between self-reported CVDs and medical records (50). Third, although we cannot definitively rule out the possibility of unmeasured confounding, the observed effect sizes’ magnitude makes it improbable for unmeasured confounding to entirely elucidate our observed associations. Four, the concurrent measurement of BMI and the TyG index at baseline does not guarantee temporality between exposure and mediator, introducing the potential for reverse causality. However, there exists sufficient biological rationale and explanation for BMI influencing insulin resistance (51–53). Last, due to the considerable sample size and associated costs, data on insulin resistance were not collected, preventing the use of homeostasis model assessment of insulin resistance (HOMA-IR) for reflecting insulin resistance, necessitating further investigations.
Conclusions
Our results indicate that the TyG index is valuable for identifying individuals prone to CVD development. Additionally, it acts as a minor mediator in the association between BMI and CVD within our general population cohort. Consequently, further exploration of the pathways connecting BMI to CVD is essential for comprehending disease origins and pinpointing populations that could gain the most from strategies aimed at reducing BMI.
Data availability statement
Publicly available datasets were analyzed in this study. This data can be found here: https://charls.pku.edu.cn/en/.
Ethics statement
The studies involving humans were approved by Ethics Review Committee of Peking University. The studies were conducted in accordance with the local legislation and institutional requirements. Written informed consent for participation was not required from the participants or the participants’ legal guardians/next of kin in accordance with the national legislation and institutional requirements.
Author contributions
Y-YG: Data curation, Formal analysis, Software, Writing – original draft. LZ: Validation, Visualization, Writing – review & editing. QL: Formal analysis, Software, Visualization, Writing – review & editing. R-RH: Project administration, Supervision, Writing – review & editing.
Funding
The author(s) declare that no financial support was received for the research, authorship, and/or publication of this article.
Acknowledgments
The authors express their gratitude to all members of the CHARLS for their contributions, as well as to the participants who generously provided their data.
Conflict of interest
The authors declare that the research was conducted in the absence of any commercial or financial relationships that could be construed as a potential conflict of interest.
Publisher’s note
All claims expressed in this article are solely those of the authors and do not necessarily represent those of their affiliated organizations, or those of the publisher, the editors and the reviewers. Any product that may be evaluated in this article, or claim that may be made by its manufacturer, is not guaranteed or endorsed by the publisher.
Supplementary material
The Supplementary Material for this article can be found online at: https://www.frontiersin.org/articles/10.3389/fendo.2024.1431087/full#supplementary-material
References
1. Crea F. The burden of cardiovascular risk factors: a global perspective. Eur Heart J. (2022) 43:2817–20. doi: 10.1093/eurheartj/ehac430
2. Collaborators GBDRF. Global burden of 87 risk factors in 204 countries and territories, 1990-2019: a systematic analysis for the Global Burden of Disease Study 2019. Lancet. (2020) 396:1223–49. doi: 10.1016/S0140-6736(20)30752-2
3. Roth GA, Mensah GA, Johnson CO, Addolorato G, Ammirati E, Baddour LM, et al. Global burden of cardiovascular diseases and risk factors, 1990-2019: update from the GBD 2019 study. J Am Coll Cardiol. (2020) 76:2982–3021. doi: 10.1016/j.jacc.2020.11.010
4. Vaduganathan M, Mensah GA, Turco JV, Fuster V, Roth GA. The global burden of cardiovascular diseases and risk: A compass for future health. J Am Coll Cardiol. (2022) 80:2361–71. doi: 10.1016/j.jacc.2022.11.005
5. Chooi YC, Ding C, Magkos F. The epidemiology of obesity. Metabolism. (2019) 92:6–10. doi: 10.1016/j.metabol.2018.09.005
6. Collaboration NCDRF. Trends in adult body-mass index in 200 countries from 1975 to 2014: a pooled analysis of 1698 population-based measurement studies with 19.2 million participants. Lancet. (2016) 387:1377–96. doi: 10.1016/S0140-6736(16)30054-X
7. Popkin BM, Slining MM. New dynamics in global obesity facing low- and middle-income countries. Obes Rev. (2013) 14 Suppl 2:11–20. doi: 10.1111/obr.12102
8. Ng M, Fleming T, Robinson M, Thomson B, Graetz N, Margono C, et al. Global, regional, and national prevalence of overweight and obesity in children and adults during 1980-2013: a systematic analysis for the Global Burden of Disease Study 2013. Lancet. (2014) 384:766–81. doi: 10.1016/S0140-6736(14)60460-8
9. Tsao CW, Aday AW, Almarzooq ZI, Anderson CAM, Arora P, Avery CL, et al. Heart disease and stroke statistics-2023 update: A report from the american heart association. Circulation. (2023) 147:e93–e621. doi: 10.1161/CIR.0000000000001123
10. Force USPST, Mangione CM, Barry MJ, Nicholson WK, Cabana M, Coker TR, et al. Behavioral counseling interventions to promote a healthy diet and physical activity for cardiovascular disease prevention in adults without cardiovascular disease risk factors: US preventive services task force recommendation statement. JAMA. (2022) 328:367–74. doi: 10.1001/jama.2022.10951
11. Makarem N, German CA, Zhang Z, Diaz KM, Palta P, Duncan DT, et al. Rest-activity rhythms are associated with prevalent cardiovascular disease, hypertension, obesity, and central adiposity in a nationally representative sample of US adults. J Am Heart Assoc. (2024) 13:e032073. doi: 10.1161/JAHA.122.032073
12. Steppan CM, Bailey ST, Bhat S, Brown EJ, Banerjee RR, Wright CM, et al. The hormone resistin links obesity to diabetes. Nature. (2001) 409:307–12. doi: 10.1038/35053000
13. Ormazabal V, Nair S, Elfeky O, Aguayo C, Salomon C, Zuniga FA. Association between insulin resistance and the development of cardiovascular disease. Cardiovasc Diabetol. (2018) 17:122. doi: 10.1186/s12933-018-0762-4
14. Simental-Mendia LE, Rodriguez-Moran M, Guerrero-Romero F. The product of fasting glucose and triglycerides as surrogate for identifying insulin resistance in apparently healthy subjects. Metab Syndr Relat Disord. (2008) 6:299–304. doi: 10.1089/met.2008.0034
15. Guerrero-Romero F, Simental-Mendia LE, Gonzalez-Ortiz M, Martinez-Abundis E, Ramos-Zavala MG, Hernandez-Gonzalez SO, et al. The product of triglycerides and glucose, a simple measure of insulin sensitivity. Comparison with the euglycemic-hyperinsulinemic clamp. J Clin Endocrinol Metab. (2010) 95:3347–51. doi: 10.1210/jc.2010-0288
16. Dang K, Wang X, Hu J, Zhang Y, Cheng L, Qi X, et al. The association between triglyceride-glucose index and its combination with obesity indicators and cardiovascular disease: NHANES 2003-2018. Cardiovasc Diabetol. (2024) 23:8. doi: 10.1186/s12933-023-02115-9
17. Yang X, Li K, Wen J, Yang C, Li Y, Xu G, et al. Association of the triglyceride glucose-body mass index with the extent of coronary artery disease in patients with acute coronary syndromes. Cardiovasc Diabetol. (2024) 23:24. doi: 10.1186/s12933-024-02124-2
18. Li F, Wang Y, Shi B, Sun S, Wang S, Pang S, et al. Association between the cumulative average triglyceride glucose-body mass index and cardiovascular disease incidence among the middle-aged and older population: a prospective nationwide cohort study in China. Cardiovasc Diabetol. (2024) 23:16. doi: 10.1186/s12933-023-02114-w
19. Huo RR, Zhai L, Liao Q, You XM. Changes in the triglyceride glucose-body mass index estimate the risk of stroke in middle-aged and older Chinese adults: a nationwide prospective cohort study. Cardiovasc Diabetol. (2023) 22:254. doi: 10.1186/s12933-023-01983-5
20. Tian X, Chen S, Wang P, Xu Q, Zhang Y, Luo Y, et al. Insulin resistance mediates obesity-related risk of cardiovascular disease: a prospective cohort study. Cardiovasc Diabetol. (2022) 21:289. doi: 10.1186/s12933-022-01729-9
21. Zhao Y, Hu Y, Smith JP, Strauss J, Yang G. Cohort profile: the China health and retirement longitudinal study (CHARLS). Int J Epidemiol. (2014) 43:61–8. doi: 10.1093/ije/dys203
22. Gu D, Dupre ME. Encyclopedia of gerontology and population aging. Cham, Switzerland: Springer (2021).
23. World Medical A. World Medical Association Declaration of Helsinki: ethical principles for medical research involving human subjects. JAMA. (2013) 310:2191–4. doi: 10.1001/jama.2013.281053
24. von Elm E, Altman DG, Egger M, Pocock SJ, Gotzsche PC, Vandenbroucke JP, et al. Strengthening the Reporting of Observational Studies in Epidemiology (STROBE) statement: guidelines for reporting observational studies. BMJ. (2007) 335:806–8. doi: 10.1136/bmj.39335.541782.AD
25. Pan XF, Wang L, Pan A. Epidemiology and determinants of obesity in China. Lancet Diabetes Endocrinol. (2021) 9:373–92. doi: 10.1016/S2213-8587(21)00045-0
26. Gao K, Cao LF, Ma WZ, Gao YJ, Luo MS, Zhu J, et al. Association between sarcopenia and cardiovascular disease among middle-aged and older adults: Findings from the China health and retirement longitudinal study. EClinicalMedicine. (2022) 44:101264. doi: 10.1016/j.eclinm.2021.101264
27. Li H, Zheng D, Li Z, Wu Z, Feng W, Cao X, et al. Association of depressive symptoms with incident cardiovascular diseases in middle-aged and older chinese adults. JAMA Netw Open. (2019) 2:e1916591. doi: 10.1001/jamanetworkopen.2019.16591
28. Levey AS, Stevens LA, Schmid CH, Zhang YL, Castro AF 3rd, Feldman HI, et al. A new equation to estimate glomerular filtration rate. Ann Intern Med. (2009) 150:604–12. doi: 10.7326/0003-4819-150-9-200905050-00006
29. VanderWeele TJ. A unification of mediation and interaction: a 4-way decomposition. Epidemiology. (2014) 25:749–61. doi: 10.1097/EDE.0000000000000121
30. Greenland S, Pearl J, Robins JM. Causal diagrams for epidemiologic research. Epidemiology. (1999) 10:37–48.
31. VanderWeele TJ. Causal mediation analysis with survival data. Epidemiology. (2011) 22:582–5. doi: 10.1097/EDE.0b013e31821db37e
32. Valeri L, Vanderweele TJ. Mediation analysis allowing for exposure-mediator interactions and causal interpretation: theoretical assumptions and implementation with SAS and SPSS macros. Psychol Methods. (2013) 18:137–50. doi: 10.1037/a0031034
33. Almuwaqqat Z, Hui Q, Liu C, Zhou JJ, Voight BF, Ho YL, et al. Long-term body mass index variability and adverse cardiovascular outcomes. JAMA Netw Open. (2024) 7:e243062. doi: 10.1001/jamanetworkopen.2024.3062
34. Powell-Wiley TM, Poirier P, Burke LE, Despres JP, Gordon-Larsen P, Lavie CJ, et al. Obesity and cardiovascular disease: A scientific statement from the american heart association. Circulation. (2021) 143:e984–e1010. doi: 10.1161/CIR.0000000000000973
35. Collaborators GBDO, Afshin A, Forouzanfar MH, Reitsma MB, Sur P, Estep K, et al. Health effects of overweight and obesity in 195 countries over 25 years. N Engl J Med. (2017) 377:13–27. doi: 10.1056/NEJMoa1614362
36. Kong KA, Park J, Hong SH, Hong YS, Sung YA, Lee H. Associations between body mass index and mortality or cardiovascular events in a general Korean population. PloS One. (2017) 12:e0185024. doi: 10.1371/journal.pone.0185024
37. Nordestgaard BG, Palmer TM, Benn M, Zacho J, Tybjaerg-Hansen A, Davey Smith G, et al. The effect of elevated body mass index on ischemic heart disease risk: causal estimates from a Mendelian randomisation approach. PloS Med. (2012) 9:e1001212. doi: 10.1371/journal.pmed.1001212
38. Liao C, Gao W, Cao W, Lv J, Yu C, Wang S, et al. Associations of metabolic/obesity phenotypes with insulin resistance and C-reactive protein: results from the CNTR study. Diabetes Metab Syndr Obes. (2021) 14:1141–51. doi: 10.2147/DMSO.S298499
39. Reaven GM. Banting lecture 1988. Role of insulin resistance in human disease. Diabetes. (1988) 37:1595–607. doi: 10.2337/diab.37.12.1595
40. Zhao Q, Zhang TY, Cheng YJ, Ma Y, Xu YK, Yang JQ, et al. Impacts of triglyceride-glucose index on prognosis of patients with type 2 diabetes mellitus and non-ST-segment elevation acute coronary syndrome: results from an observational cohort study in China. Cardiovasc Diabetol. (2020) 19:108. doi: 10.1186/s12933-020-01086-5
41. Bakhtiyari M, Schmidt N, Hadaegh F, Khalili D, Mansournia N, Asgari S, et al. Direct and indirect effects of central and general adiposity on cardiovascular diseases: The Tehran Lipid and Glucose Study. Eur J Prev Cardiol. (2018) 25:1170–81. doi: 10.1177/2047487318780030
42. Lu Y, Hajifathalian K, Rimm EB, Ezzati M, Danaei G. Mediators of the effect of body mass index on coronary heart disease: decomposing direct and indirect effects. Epidemiology. (2015) 26:153–62. doi: 10.1097/EDE.0000000000000234
43. Li S, Guo B, Chen H, Shi Z, Li Y, Tian Q, et al. The role of the triglyceride (triacylglycerol) glucose index in the development of cardiovascular events: a retrospective cohort analysis. Sci Rep. (2019) 9:7320. doi: 10.1038/s41598-019-43776-5
44. Grundy SM. Obesity, metabolic syndrome, and cardiovascular disease. J Clin Endocrinol Metab. (2004) 89:2595–600. doi: 10.1210/jc.2004-0372
45. Sinha R, Dufour S, Petersen KF, LeBon V, Enoksson S, Ma YZ, et al. Assessment of skeletal muscle triglyceride content by (1)H nuclear magnetic resonance spectroscopy in lean and obese adolescents: relationships to insulin sensitivity, total body fat, and central adiposity. Diabetes. (2002) 51:1022–7. doi: 10.2337/diabetes.51.4.1022
46. Lopez-Jimenez F, Almahmeed W, Bays H, Cuevas A, Di Angelantonio E, le Roux CW, et al. Obesity and cardiovascular disease: mechanistic insights and management strategies. A joint position paper by the World Heart Federation and World Obesity Federation. Eur J Prev Cardiol. (2022) 29:2218–37. doi: 10.1093/eurjpc/zwac187
47. Hu G, Tuomilehto J, Silventoinen K, Sarti C, Mannisto S, Jousilahti P. Body mass index, waist circumference, and waist-hip ratio on the risk of total and type-specific stroke. Arch Intern Med. (2007) 167:1420–7. doi: 10.1001/archinte.167.13.1420
48. Basri R, Shaik MM, Alam MK, Mondol MBA, Mohammad QD, Gan SH. Waist to hip ratio, waist to height ratio and body mass index predict stroke risk in a Bangladeshi population. Int Med J. (2013) 20:740–3.
49. Irisawa H, Mizushima T. Correlation of body composition and nutritional status with functional recovery in stroke rehabilitation patients. Nutrients. (2020) 12:1923. doi: 10.3390/nu12071923
50. Steptoe A, Breeze E, Banks J, Nazroo J. Cohort profile: the English longitudinal study of ageing. Int J Epidemiol. (2013) 42:1640–8. doi: 10.1093/ije/dys168
51. Kahn BB, Flier JS. Obesity and insulin resistance. J Clin Invest. (2000) 106:473–81. doi: 10.1172/JCI10842
52. Shoelson SE, Herrero L, Naaz A. Obesity, inflammation, and insulin resistance. Gastroenterology. (2007) 132:2169–80. doi: 10.1053/j.gastro.2007.03.059
Keywords: cardiovascular disease, triglyceride-glucose index, body mass index, mediator, 4-way decomposition approach, CHARLS
Citation: Gan Y-Y, Zhai L, Liao Q and Huo R-R (2024) Triglyceride-glucose index as a mediator of body mass index and cardiovascular disease in middle-aged and older Chinese adults: a nationally representative longitudinal cohort study. Front. Endocrinol. 15:1431087. doi: 10.3389/fendo.2024.1431087
Received: 11 May 2024; Accepted: 05 December 2024;
Published: 23 December 2024.
Edited by:
Ara Jo, University of Florida, United StatesReviewed by:
Velyn Wu, University of Florida, United StatesManoj Kumar Mahata, Belle Vue Clinic, India
Guangyao Zhai, Capital Medical University, China
Copyright © 2024 Gan, Zhai, Liao and Huo. This is an open-access article distributed under the terms of the Creative Commons Attribution License (CC BY). The use, distribution or reproduction in other forums is permitted, provided the original author(s) and the copyright owner(s) are credited and that the original publication in this journal is cited, in accordance with accepted academic practice. No use, distribution or reproduction is permitted which does not comply with these terms.
*Correspondence: Rong-Rui Huo, aHVvcm9uZ3J1aUBzci5neG11LmVkdS5jbg==
†ORCID: Rong-Rui Huo, orcid.org/0000-0002-3723-9619