- 1Department of Critical Care Medicine, Ningbo Medical Center Lihuili Hospital, Ningbo University, Ningbo, China
- 2Wenzhou Medical University Renji College, Wenzhou, China
- 3Ningbo Institute of Innovation for Combined Medicine and Engineering, Ningbo Medical Center Lihuili Hospital, Ningbo University, Ningbo, China
- 4Department of Endocrinology, Ningbo Medical Center Lihuili Hospital, Ningbo University, Ningbo, China
- 5Department of Cardiovascular, Ningbo Medical Center Lihuili Hospital, Ningbo University, Ningbo, China
Background: Observational studies and clinical trials have implicated polyunsaturated fatty acids (PUFAs) in potentially safeguarding against diabetic microvascular complication. Nonetheless, the causal nature of these relationships remains ambiguous due to conflicting findings across studies. This research employs Mendelian randomization (MR) to assess the causal impact of PUFAs on diabetic microvascular complications.
Methods: We identified instrumental variables for PUFAs, specifically omega-3 and omega-6 fatty acids, using the UK Biobank data. Outcome data regarding diabetic microvascular complications were sourced from the FinnGen Study. Our analysis covered microvascular outcomes in both type 1 and type 2 diabetes, namely diabetic neuropathy (DN), diabetic retinopathy (DR), and diabetic kidney disease (DKD). An inverse MR analysis was conducted to examine the effect of diabetic microvascular complications on PUFAs. Sensitivity analyses were performed to validate the robustness of the results. Finally, a multivariable MR (MVMR) analysis was conducted to determine whether PUFAs have a direct influence on diabetic microvascular complications.
Results: The study indicates that elevated levels of genetically predicted omega-6 fatty acids substantially reduce the risk of DN in type 2 diabetes (odds ratio (OR): 0.62, 95% confidence interval (CI): 0.47–0.82, p = 0.001). A protective effect against DR in type 2 diabetes is also suggested (OR: 0.75, 95% CI: 0.62–0.92, p = 0.005). MVMR analysis confirmed the stability of these results after adjusting for potential confounding factors. No significant effects of omega-6 fatty acids were observed on DKD in type 2 diabetes or on any complications in type 1 diabetes. By contrast, omega-3 fatty acids showed no significant causal links with any of the diabetic microvascular complications assessed.
Conclusions: Our MR analysis reveals a causal link between omega-6 fatty acids and certain diabetic microvascular complications in type 2 diabetes, potentially providing novel insights for further mechanistic and clinical investigations into diabetic microvascular complications.
1 Introduction
Diabetes poses a significant global health challenge, affecting approximately 10% of the adult population worldwide (1). This condition markedly elevates the risk of microvascular complications, such as diabetic neuropathy (DN), diabetic retinopathy (DR), and diabetic kidney disease (DKD) (2). Significantly, >50% of those diagnosed with diabetes have DN, which can result in chronic pain, progressive sensory loss, and non-traumatic amputations (3). Additionally, 30%–40% of the patients are at risk for DR, a leading cause of blindness (4), and 30%–40% of the patients may develop DKD—the leading cause of end-stage renal disease (5). These statistics highlight the urgent need for improved prevention and treatment strategies for diabetic microvascular complications (6).
Traditionally, research on diabetic complications has focused on glucose metabolism (7). Despite intense glucose control efforts, complications continue to be prevalent, suggesting that other factors also contribute (7). Recent evidence indicates that lipids, including polyunsaturated fatty acids (PUFAs), play a crucial role in these complications (8). PUFAs mainly include omega-3 and omega-6 fatty acids. Observational studies have found that omega-6 fatty acids might protect against DN (9–11) and DR (12, 13), but their impact on DKD is uncertain (14, 15). To date, no randomized controlled trial (RCT) has evaluated the effects of omega-6 fatty acids on these conditions. By contrast, the effects of omega-3 fatty acids have been comprehensively investigated in both observational studies and RCTs, suggesting that omega-3 fatty acids may reduce the risk and severity of DN (16, 17), but their effects on DR (18–20) and DKD (14, 15, 21, 22) have been inconsistent. This emphasizes the need for research on the roles of PUFA in diabetic microvascular complications.
Mendelian randomization (MR), which employs genetic variations to simulate the random allocation inherent in RCTs, serves as an effective method for examining causal relationships in scenarios where RCTs are impractical (23). This approach precludes reverse causation by utilizing genetic predispositions that are established before disease onset (24). Although one MR study associated PUFA intake with reduced risk of DR, it did not differentiate between types of diabetes (25). This research used MR to investigate the causal links between PUFAs, specifically omega-6 and omega-3 fatty acids, and diabetic microvascular complications, including type 1 DN (T1DN), type 1 DR (T1DR), type 1 DKD (T1DKD), type 2 DN (T2DN), type 2 DR (T2DR), and type 2 DKD (T2DKD).
2 Methods
2.1 Study design
We conducted a two-sample MR analysis using summary statistics from genome-wide association studies (GWAS) to investigate the causal relationship between PUFAs and diabetic microvascular complications. Genetic variants were used as instrumental variables (IVs) to assess the causal effects of exposure (omega-3 and omega-6) on outcomes (T1DN, T1DR, T1DKD, T2DN, T2DR, and T2DKD). Subsequently, an inverse MR analysis was performed to determine the effect of diabetic microvascular complications on PUFA levels. Given the role of lifestyle and physical conditions in the development of diabetic microvascular complications, we conducted a multivariable MR (MVMR) analysis to ascertain whether the effects of PUFAs on diabetic microvascular complications were direct or mediated by other factors. The study design is illustrated in Figure 1. Additionally, the causal effects of omega-3 and omega-6 fatty acids on type 1 diabetes (T1D) and type 2 diabetes (T2D) were evaluated to understand mechanisms of action. This study follows the STROBE-MR guidelines for reporting findings (26).
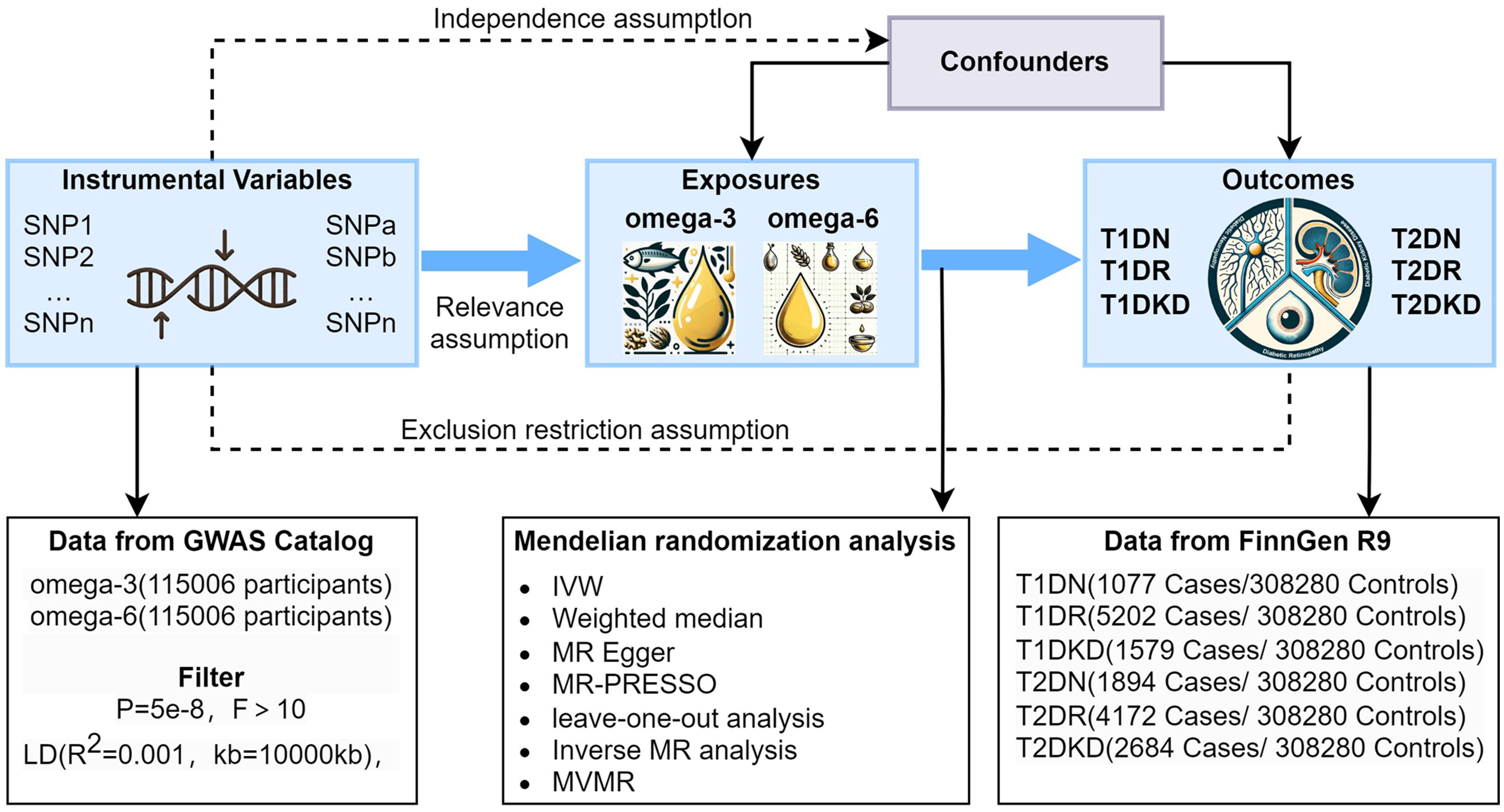
Figure 1 Graphical representation of the MR assumptions in a two-sample MR design, including (i) relevance; (ii) independence; and (iii) exclusion restriction. LD, linkage disequilibrium; SNPs, single nucleotide polymorphisms; IVW, inverse variance weighted; T1DN, type 1 diabetes neuropathy; T1DR, type 1 diabetes retinopathy; T1DKD, type 1 diabetic kidney disease; T2DN, type 2 diabetes neuropathy; T2DR, type 2 diabetes retinopathy; T2DKD, type 2 diabetic kidney disease.
2.2 Data source
Genetic data pertaining to PUFAs were sourced from the UK Biobank, a comprehensive cohort study in the United Kingdom that recruited participants between 2006 and 2010 (27). Eligibility criteria required participants to be 40–69 years of age at recruitment (27). The UK Biobank provides a rich resource of genetic and phenotypic information (27). From this repository, we selected a random subset of >110,000 baseline EDTA plasma samples from the general population. These samples underwent analysis at Nightingale Health, where nuclear magnetic resonance and specialized software facilitated the evaluation of >200 metabolic biomarkers, including omega-6 and omega-3 fatty acids, from each blood sample (28). Among UK Biobank participants, mean concentration of omega-3 fatty acids was 0.53 mmol/L (SD: 0.22) and omega-6 fatty acids was 4.51 mmol/L (SD: 0.69).
Summary-level data on diabetic microvascular complications were obtained from the FinnGen Study, a collaborative research project in Finland (29). This project utilizes nationwide longitudinal health registry data collected from every resident in Finland since 1969, with continuous follow-up and data collection (29). This project integrates genotype data from Finnish biobanks with digital health records from national registries, to understand the genetics of various diseases in a cohort comprising 500,000 participants (29). T1DN, T1DR, T1DKD, T2DN, T2DR, and T2DKD were identified using ICD-10 codes.
The UK Biobank and FinnGen studies are based on different cohorts and geographic regions, thus minimizing the risk of sample overlap and bias. Both studies primarily consist of individuals of European ancestry, ensuring that the genetic background is similar and the associations are comparable. Standard imputation algorithms were employed in both datasets to ensure data completeness, allowing for the inclusion of all available participants. The mRnd online calculator (https://shiny.cnsgenomics.com/mRnd/) was utilized to calculate statistical power (30). With sample sizes of 310,174, 312,452, 310,964, 309,357, 313,482, and 309,859 for T2DN, T2DR, T2DKD, T1DN, T1DR, and T1DKD, respectively, the powers to detect an odds ratio (OR) of 0.7 for developing these conditions per standard deviation increase in PUFA levels (assuming genetic variants explain approximately 5% variance in PUFA levels) were 0.83, 0.99, 0.93, 0.59, 1.0, and 0.76, respectively.
In the MVMR analysis, variables such as C-reactive protein (CRP), glucose, and smoking were sourced from the UK Biobank, while interleukin 6 (IL-6) data were obtained from the study by Jing Hua Zhao et al. (31). Additionally, data for T1D and T2D used in the supplementary analyses were sourced from the Finnish database. Further details on the datasets can be found in Supplementary Table 1.
The UK Biobank study was approved by the North West Multi-centre Research Ethics Committee, and all participants provided written informed consent (27). The FinnGen study protocol received approval from the Ethics Committee of the Hospital District of Helsinki and Uusimaa (29). For this project, ethical approval was not required because the data were derived from the summary statistics of published GWAS and did not involve individual-level data.
2.3 Selection of IVs
IVs, identified as single nucleotide polymorphisms (SNPs), were selected through a rigorous screening process consistent with the core MR principles of relevance (IV is closely related to exposure), independence (IV is not related to confounders), and exclusion restriction (IV affects the outcome only through exposure) (24). Genetic variants were chosen as IVs based on their strong association with exposure, applying stringent criteria (p < 5 × 10−8 and F-statistic > 10) to ensure robustness. Their independence was confirmed via linkage disequilibrium analysis, adopting an R2 threshold of <0.001 to affirm IV independence. Alignment of SNPs related to both outcome and exposure was verified to maintain methodological consistency. To identify and address potential confounding factors, we utilized the PhenoScanner database. SNPs introducing potential bias were iteratively excluded, guided by increasing p-values from the MR-PRESSO outlier test, until no significant outliers were detected (p > 0.05) in the MR-PRESSO global test. The detailed methodology, including the IV selection process, is further explicated in Supplementary Figure 1.
2.4 MR analysis and sensitivity analyses
Primary outcomes were analyzed using the inverse-variance-weighted (IVW) method. This approach is most accurate when all selected SNPs act as valid IVs (26). In the IVW method, the SNPs were weighted by the inverse of their variance. This weighting scheme provides more precise estimates by giving more weight to SNPs with smaller standard errors. The weights were derived from the GWAS summary statistics, ensuring that the contribution of each SNP to MR analysis was proportional to its precision (32). In cases of heterogeneity, we opted for a random-effects model IVW (33). We augmented the robustness of our estimates by incorporating the weighted median (WM) and MR-Egger methods. The WM method provides consistent estimates when >50% of the data comes from valid SNPs (34), whereas MR-Egger addresses potential pleiotropic effects independent of the variant-exposure association (35). Ultimately, the causal estimates were expressed as ORs with their corresponding 95% confidence intervals (CIs).MR-Egger regression was employed to assess the influence of pleiotropy among IVs. In MR-Egger regression, the intercept term serves as a test for directional pleiotropy. A non-significant intercept (p > 0.05) indicates the absence of systematic bias in the causal estimate due to pleiotropy. Relative symmetry in funnel plots suggests an absence of directional pleiotropy. Cochran’s Q test was conducted to assess potential heterogeneity among IVs, indicated by a non-significant result (p > 0.05). The “leave-one-out” analysis, which sequentially excludes one SNP at a time, further validated our results by assessing the influence of individual SNPs on the overall causal estimate (30).
2.5 Statistical analyses
All analyses were conducted in R version 4.3.2, using “Two-Sample MR,” “Mendelian Randomization,” “MVMR,” and “MR-PRESSO” to facilitate MR analyses. To tackle the issue of multiple testing arising from examining the relationship between two traits and six diabetes complications, Bonferroni’s correction was applied. Significance thresholds were set at p-value <0.004 (0.05/(2*6)) for significance, p-value <0.05 for nominal significance, and p-values between 0.004 and 0.05 for suggestive evidence.
3 Results
3.1 IVs for PUFAs and diabetic microvascular complications
Following a stringent screening process grounded in the principles of independence and exclusivity, in addition to the harmonization and elimination of palindromic SNPs with intermediate allele frequencies, we identified 36–44 SNPs for MR analysis (Supplementary Tables 2-13). The F-statistics for these IVs exceeded the threshold of 10, signifying their adequate strength for MR analysis and reducing the risk of bias due to weak instruments.
3.2 Association between genetically predicted omega-6 and diabetic microvascular complications
3.2.1 Omega-6 fatty acid and DN
Figure 2 demonstrates that our primary IVW analysis detected a significant risk reduction for T2DN correlated with an increase of one standard deviation in genetically predicted omega-6 fatty acid levels, yielding an OR of 0.62 (95% CI: 0.47–0.82, p = 0.001). This translates to a 38% reduction in risk, suggesting a protective role of omega-6 fatty acids against neuropathy in patients with T2D. The WM method confirmed this association with an OR of 0.64 (95% CI: 0.44–0.93, p = 0.020), closely mirroring the outcomes of IVW analysis. Although MR-Egger analysis did not reach statistical significance (OR: 0.72, 95% CI: 0.41–1.28; p = 0.268), it exhibited a consistent direction of effect (Figure 3).
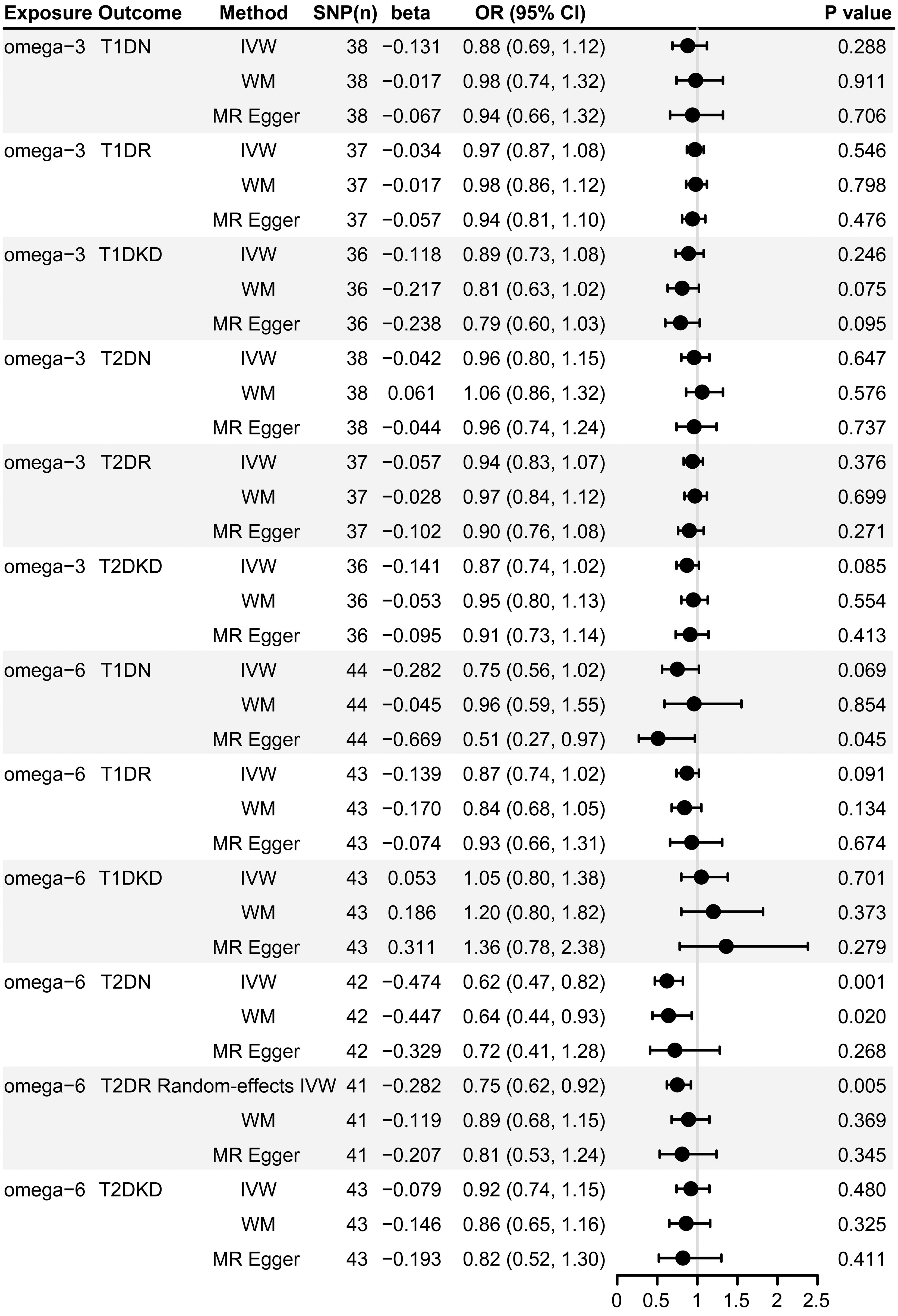
Figure 2 Forest plot of MR estimates of the causal associations of omega-3 and omega-6 with diabetic microvascular complications. SNP, single-nucleotide polymorphism; MR, Mendelian randomization; IVW, inverse variance weighted; WM, weighted median; T1DN, type 1 diabetes neuropathy; T1DR, type 1 diabetes retinopathy; T1DKD, type 1 diabetic kidney disease; T2DN, type 2 diabetes neuropathy; T2DR, type 2 diabetes retinopathy; T2DKD, type 2 diabetic kidney disease; CI, confidence interval; OR, odds ratio.
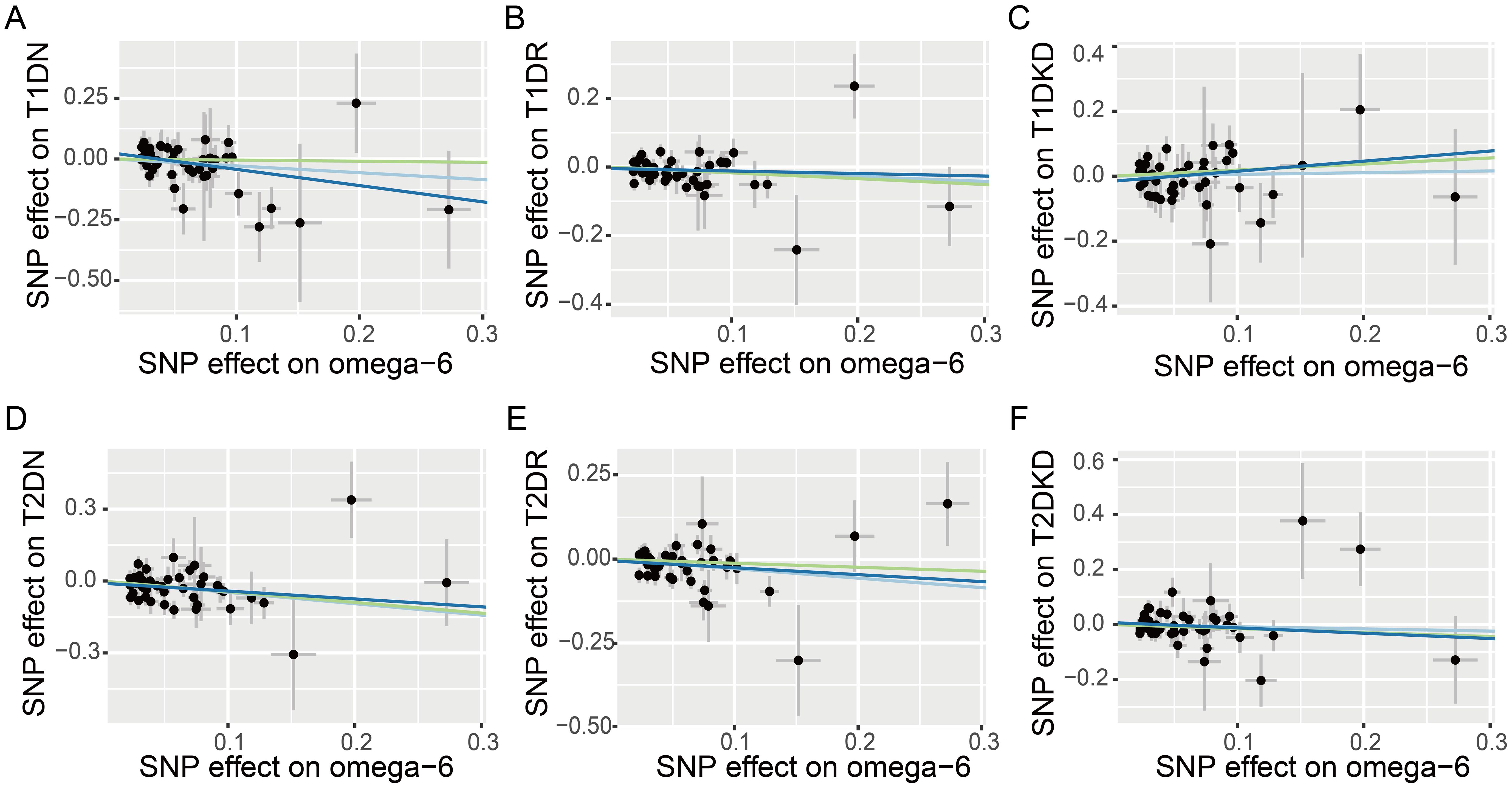
Figure 3 Scatter plots illustrating the effects of omega-6 polyunsaturated fatty acids on various diabetic microvascular complications, as determined by Mendelian Randomization analyses. Different colors represent the analytical methods used: light blue indicates the Inverse Variance Weighted (IVW) method, dark blue signifies MR-Egger regression, and green highlights the Weighted Median (WM) method. The conditions analyzed include: (A) type 1 diabetes neuropathy (T1DN); (B) type 1 diabetes retinopathy (T1DR); (C) type 1 diabetic kidney disease (T1DKD); (D) type 2 diabetes neuropathy (T2DN); (E) type 2 diabetes retinopathy (T2DR); (F) type 2 diabetic kidney disease (T2DKD).
However, our analyses did not identify a statistically significant causal link between omega-6 levels and T1DN risk, with both IVW and WM methods resulting in p-values > 0.05 (Figure 2). However, MR-Egger estimates suggested a potential protective effect of omega-6 (OR: 0.51, 95% CI: 0.27–0.97; p = 0.045), albeit with caution.
3.2.2 Omega-6 fatty acid and DR
The IVW method revealed a causal association between higher genetically predicted omega-6 fatty acid levels and decreased risk of T2DR, documented by an OR of 0.75 (95% CI: 0.62–0.92, p = 0.005). However, applying Bonferroni’s correction for multiple comparisons suggested that these findings are suggestive, and not conclusive, of a causal relationship. Additional MR methods, including WM (OR: 0.89, 95% CI: 0.68–1.15, p = 0.369) and MR-Egger (OR: 0.81, 95% CI: 0.53–1.24, p = 0.345) supported the direction of the effect but did not achieve statistical significance. This directional consistency is visually supported by Figure 3, indicating alignment across IVW, MR-Egger, and WM. Therefore, our research indicates a potential causal connection between omega-6 levels and reduced incidence of T2DR, awaiting further validation.
However, our analyses did not find a significant association between omega-6 fatty acids and T1DR using any MR approach (Figure 2, all p-values >0.05).
3.2.3 Omega-6 fatty acid and DKD
Our study did not reveal any significant correlations between omega-6 fatty acid levels and either T1DKD or T2DKD, as evidenced by all employed analytical methods (Figure 2, all p-values > 0.05).
3.3 Association between genetically predicted omega-3 and diabetic microvascular complications
Our analysis did not identify any significant association between omega-3 fatty acids and microvascular complications in both types of diabetes, as determined by all analytical approaches used (Figure 2, all p-values > 0.05). Scatter plots offering a visual representation of these results can be found in Supplementary Figure 2.
3.4 Sensitivity analysis
Heterogeneity was observed in the MR analysis of omega-6 fatty acids on T2DR, with MR-Egger and IVW p-values of 0.034 and 0.041, respectively (Supplementary Table 14). Given the heterogeneity, we employed a random-effects IVW estimate to assess the causal relationship (33). This approach confirmed that higher levels of omega-6 fatty acids are significantly associated with a reduced risk of T2DR (Figure 2). Importantly, the MR-Egger intercept test showed no evidence of horizontal pleiotropy (p-values > 0.05), indicating the associations are likely genuine and not confounded by pleiotropic effects (Supplementary Table 14). The symmetry in funnel plots (Supplementary Figure 3) further supports this, indicating no directional pleiotropy or biases affecting the results. Moreover, leave-one-out sensitivity analysis reinforced the robustness of our findings, showing that no single IV disproportionately influenced overall conclusion (Supplementary Figures 4, 5).
3.5 Inverse MR analysis
In the inverse analysis, the IVs for diabetic microvascular complications were 7–36. The IVW method revealed that T2DN and T2DKD had no effect on omega-3 or omega-6, whereas T2DR was negatively associated with omega-6 (OR: 0.98, 95% CI: 0.97–0.99, p = 3.71 × 10−4). The full details of inverse MR analysis, including results for T1DN, T1DR, and T1DKD, are provided in Supplementary Table 15. Analyses of heterogeneity and pleiotropy are presented in Supplementary Table 16.
3.6 MVMR analysis
After adjusting for confounding factors, including smoking, glucose, IL-6, and CRP, omega-6 fatty acids were negatively associated with both T2DN and T2DR (Figure 4 and Supplementary Table 17). Furthermore, the MVMR Egger regression showed no significant evidence of a nonzero intercept, thereby reinforcing the robustness of the MVMR analysis results (Supplementary Table 18).
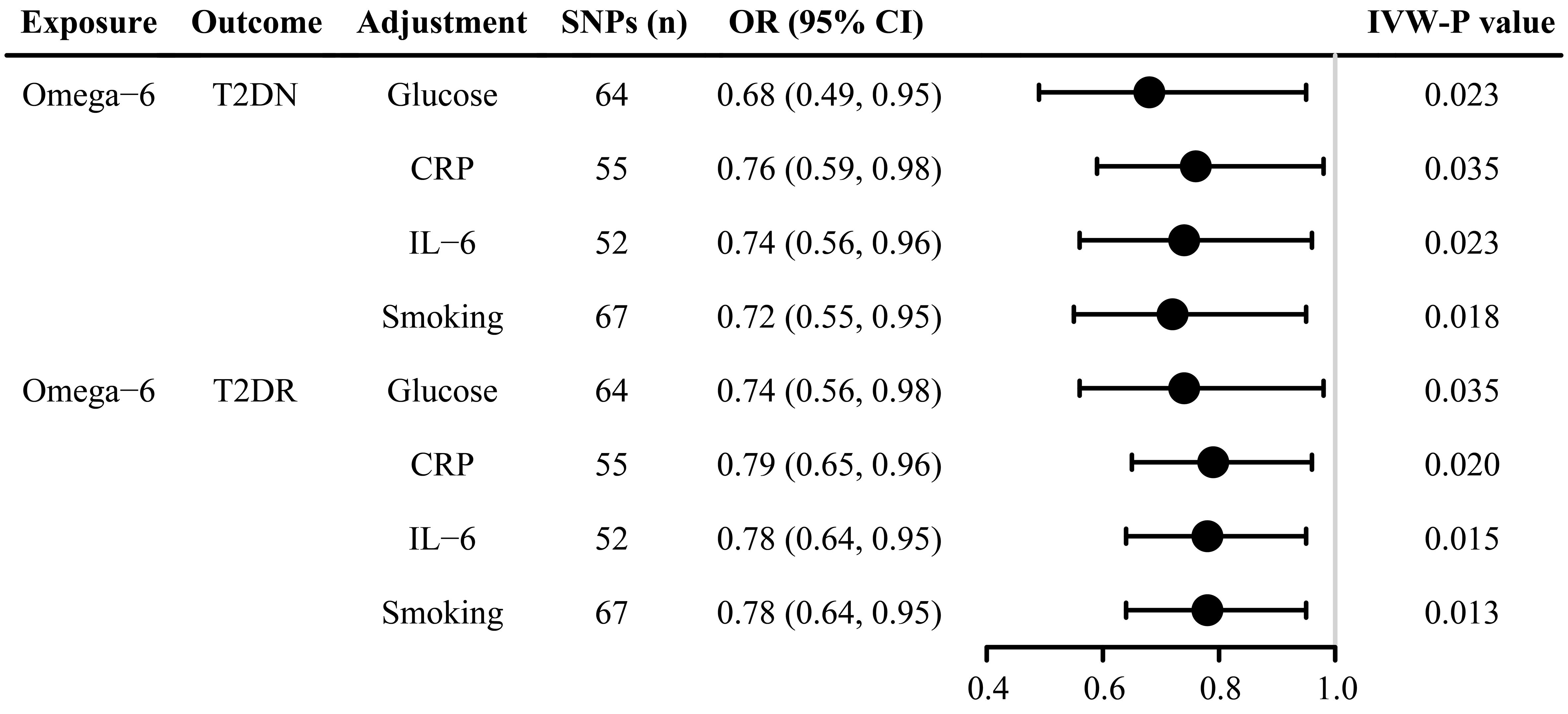
Figure 4 Forest plot of multivariate Mendelian randomization estimates of the causal associations after adjustment for glucose, CRP, IL-6, and smoking. SNP, single-nucleotide polymorphism; IVW, inverse variance weighted; T2DN, type 2 diabetes neuropathy; T2DR, type 2 diabetes retinopathy; CRP, C-reactive protein; IL-6, Interleukin 6; CI, confidence interval; OR, odds ratio. .
3.7 Association between genetically predicted PUFAs and diabetes
We evaluated the potential causal relationships between omega-3 and omega-6 fatty acids and the risk of both T1D and T2D. The IVs were 13–41, with all F-statistics >10 (Supplementary Tables 19-22). The IVW analysis identified a significant reduction in the risk of T2D associated with an increase of one standard deviation in genetically predicted omega-6 fatty acid levels (OR: 0.87, 95% CI: 0.82–0.93, p = 3.67 × 10−5). This finding was corroborated by the WM method, which yielded an OR of 0.83 (95% CI: 0.76–0.91, p = 3.39 × 10−5), closely aligning with the results of IVW. Although MR-Egger analysis did not achieve statistical significance (OR: 0.90, 95% CI: 0.79–1.03, p = 0.146), the direction of the effect remained consistent (Supplementary Table 23).
By contrast, our analyses did not reveal any significant association between omega-3 fatty acids and T1D or T2D, nor between omega-6 and T1D using any MR approach (Supplementary Table 23, all p-values > 0.05).
The Q-test did not detect heterogeneity in any of the analyses. Furthermore, the MR-Egger intercept test showed no evidence of horizontal pleiotropy (all p-values > 0.05), indicating that these associations are likely genuine and not confounded by pleiotropic effects (Supplementary Table 24).
4 Discussion
This study employed a two-sample MR approach to investigate the causal effects of genetically determined PUFAs, specifically omega-6 and omega-3, on microvascular complications in both T1D and T2D. Our findings indicate that in T2D, elevated omega-6 levels are linked to a decreased risk of DN and DR. This relationship remained significant after adjusting for confounding factors, including smoking, glucose, IL-6, and CRP. However, omega-6 levels do not significantly influence DKD. Conversely, omega-6 levels do not seem to have a substantial effect on microvascular complications in T1D, and no significant relationships were observed between omega-3 levels and microvascular complications in either diabetes type.
Studies have focused on the effects of omega-6 fatty acids on DN, especially within the context of T2D (9–11). Aligning with these findings (9–11), our MR analysis indicates a link between omega-6 fatty acids and decreased risk of T2DN. This correlation was not observed in T1DN, implying distinct pathophysiological mechanisms underpinning DN across diabetes types. In T1DN, effective glucose management halts disease progression, whereas its influence on T2DN is less significant (36). This suggests that T2DN might be subject to additional factors, including changes in inflammation (37, 38), plasma lipid levels, and metabolic alterations (8, 39).
Previous MR studies have investigated the effects of gut microbiota and PUFAs on DR (25, 40). Kangcheng Liu et al. demonstrated a causal relationship between specific gut microbiota and DR, supporting the “gut-retina” axis concept (40). Shaojie Ren et al. found that higher levels of PUFAs, including omega-3 and omega-6, were associated with a reduced risk of DR (25). However, these studies did not distinguish between T1DR and T2DR. In contrast, our study analyzed the impact of PUFAs on both T1D and T2D and their respective retinopathies (T1DR, T2DR), providing a more comprehensive understanding of the differential effects of PUFAs on these conditions. Our findings propose a protective role of omega-6 fatty acids against T2DR and T2D, corroborating other observational studies (12, 13, 41). However, this protective effect is not observed in T1DR, possibly due to different pathogenetic mechanisms. Managing glycemic levels is crucial in controlling T1DR (42, 43), whereas T2DR may be influenced by a broader array of factors, such as inflammation (44, 45), blood pressure (46), and lipid metabolism (47, 48).
RCTs have demonstrated that diets high in omega-6 fatty acids can reduce inflammation (49, 50) Linoleic acid (LA), a primary omega-6 fatty acids, can be metabolized to arachidonic acid (AA), which is proinflammatory (51). However, only ~0.2% of dietary LA is converted to AA (52). Moreover, the levels of AA in tissues do not change with the dietary intake of LA (53). Recent studies have shown that increasing dietary LA can mitigate inflammation (50, 51). For instance, LA is negatively associated with high-sensitivity CRP (54). Pinolenic acid, another omega-6 fatty acid, demonstrated significant anti-inflammatory and anti-atherosclerotic effects by reducing the expression of inflammatory markers, such as TNF-α and IL-6 (55). Elevated levels of high-sensitivity CRP, IL-6, and TNF-α were positively associated with the risk of microvascular complications in patients with diabetes (56, 57). Therefore, omega-6 fatty acids may protect against microvascular complications by reducing inflammation.
The presence of metabolic syndrome, which includes hyperglycemia, hypertension, low high-density lipoprotein cholesterol (HDL-C), high triglycerides, and increased waist circumference, significantly elevates the risk of developing microvascular complications in patients with T2D (58). Diets rich in PUFAs have been associated with a reduced risk of metabolic syndrome (59). Our study indicates a negative association between omega-6 fatty acids and the risk of T2D, while no significant relationship was found between omega-3 fatty acids and T2D risk. These findings are consistent with results from cohort studies conducted in Asian (n = 6,393) and European (n = 14,558) populations (41). In the study, 154 metabolic biomarkers were analyzed, and 13 metabolites, including omega-6 PUFAs, were identified as being associated with a lower risk of T2D (41). However, no statistically significant association was found between omega-3 fatty acids and T2D risk (41). Additionally, a meta-analysis indicated that omega-6 PUFAs were inversely related to the risk of hypertension (60). MR analysis further demonstrated that higher serum levels of omega-6 fatty acids, particularly adrenic acid, significantly increased HDL-C levels and significantly decreased triglyceride levels (61). Omega-6 fatty acids, especially LA, were negatively associated with increase in waist circumference (62). These results further support the potential protective role of omega-6 fatty acids against microvascular complications associated with T2D.
Observational studies originating from Brazil suggest a role for omega-6 fatty acids in reducing the risk of DKD (15), yet our MR analysis, alongside observational research from China (14), has failed to establish a significant link. This discrepancy may reflect methodological divergence and genetic difference across populations. Regarding omega-3 fatty acids, although certain observational studies have posited benefits for DKD (14, 15, 21), our MR findings, complemented by results from a subsequent RCT (22), do not corroborate a significant impact. This emphasizes the critical role of robust study designs, such as MR and RCTs, in identifying causal relationships. Similarly, although observational studies from the United States (16) and a single-arm, open-label clinical trial (17) have suggested that omega-3 fatty acids potentially mitigate the risk of DN, our MR study has not found a significant association between the two.
Our investigation into the effect of omega-3 on DR did not reveal benefits, aligning with a major RCT in the UK (20). Although evidence from an earlier MR study (25) and an RCT in Spain (19) suggest a potential role for omega-3 against proliferative DR, our study did not distinguish between retinopathy subtypes. Given the lower prevalence of sight-threatening DR, including proliferative DR, which accounts for ~6% of the patients with diabetes (63), compared with the general incidence of DR among patients with diabetes estimated at 30%–40% (4), this disparity in proportions might explain why we did not observe potential benefits of omega-3 on specific DR subtypes.
Although this study offers significant insights into the influence of PUFAs on diabetic microvascular complications, several limitations merit attention. First, the MR method is inherently dependent on the assumption that selected genetic variants are accurate proxies for the exposure of interest. Despite our diligence in choosing robust instruments, the risk of bias from pleiotropic effects—where a single gene impacts multiple traits—cannot be completely disregarded, which might skew our results. We conducted sensitivity analyses to address this concern (64). Second, the genetic makeup of our study population reflects European ancestry, potentially restricting the generalizability of our findings to other ethnic groups. The interactions between genetics and environmental factors influencing diabetic complications may vary considerably across populations (65), emphasizing the need for conducting similar studies in more ethnically diverse cohorts. Estimations of PUFA intake using genetic proxies might not capture the intricate relationship between diet and disease comprehensively. Direct biomarker analysis would provide a more accurate depiction of PUFA’s influence; however, such data were not available for this research. Last, concentrating on particular PUFA subtypes enabled the identification of their specific impacts on diabetic microvascular complications. Nonetheless, this method overlooks possible synergistic or cumulative effects of different fatty acids and does not encompass broader dietary and lifestyle factors. Future research should examine the overarching influence of diet on diabetic complications, incorporating a wider array of dietary patterns and lifestyle considerations.
5 Conclusion
In conclusion, our study has established that genetic variants linked to omega-6 fatty acids significantly modulate the risk of T2DN and T2DR. Conversely, our analysis revealed that omega-3 fatty acids exhibit no significant correlation with diabetic microvascular complications. These findings suggest that dietary strategies emphasizing omega-6 fatty acids offer a viable preventative approach for some diabetic microvascular complications. Our results question the protective efficacy of omega-3 fatty acids in this context. Crucially, our research sets the stage for further investigations into the differential effects of omega-6 and omega-3 fatty acids, providing a foundation for future studies dedicated to uncovering mechanisms by which PUFAs influence diabetic microvascular complications.
Data availability statement
The original contributions presented in the study are included in the article/Supplementary Material. Further inquiries can be directed to the corresponding author.
Ethics statement
The UK Biobank Study was approved by the National Information Governance Board for Health and Social Care and the NHS North West Multicentre Research Ethics Committee. Ethical oversight for the FinnGen study is managed by Fimea and the HUS Coordinating Ethics Committee (HUS/990/2017), with further endorsements from THL, the Digital and Population Data Service Agency, KELA, and Statistics Finland, ensuring thorough ethical and regulatory compliance across the board. The studies were conducted in accordance with the local legislation and institutional requirements. The human samples used in this study were acquired from gifted from another research group. Written informed consent for participation was not required from the participants or the participants’ legal guardians/next of kin in accordance with the national legislation and institutional requirements.
Author contributions
BL: Conceptualization, Funding acquisition, Investigation, Methodology, Software, Visualization, Writing – original draft. RL: Formal Analysis, Investigation, Writing – original draft. YG: Investigation, Methodology, Software, Writing – review & editing. XS: Investigation, Methodology, Writing – review & editing. JZ: Supervision, Writing – review & editing. CL: Conceptualization, Supervision, Writing – review & editing, Funding acquisition.
Funding
The author(s) declare financial support was received for the research, authorship, and/or publication of this article. Funding for this research was provided by the Medical and Health Science and Technology Project of Zhejiang Province (grant number 2023KY1032) and the Ningbo Natural Science Foundation (grant number 2023J229).
Acknowledgments
The data used in this study were sourced from the UK Biobank and the FinnGen Consortium. We extend our gratitude to the contributors of the data utilized in this research.
Conflict of interest
The authors declare that the research was conducted in the absence of any commercial or financial relationships that could be construed as a potential conflict of interest.
Publisher’s note
All claims expressed in this article are solely those of the authors and do not necessarily represent those of their affiliated organizations, or those of the publisher, the editors and the reviewers. Any product that may be evaluated in this article, or claim that may be made by its manufacturer, is not guaranteed or endorsed by the publisher.
Supplementary material
The Supplementary Material for this article can be found online at: https://www.frontiersin.org/articles/10.3389/fendo.2024.1406382/full#supplementary-material
References
1. Sun H, Saeedi P, Karuranga S, Pinkepank M, Ogurtsova K, Duncan BB, et al. IDF Diabetes Atlas: Global, regional and country-level diabetes prevalence estimates for 2021 and projections for 2045. Diabetes Res Clin practice. (2022) 183:109119. doi: 10.1016/j.diabres.2021.109119
2. Lyssenko V, Vaag A. Genetics of diabetes-associated microvascular complications. Diabetologia. (2023) 66:1601–13. doi: 10.1007/s00125-023-05964-x
3. Dillon BR, Ang L, Pop-Busui R. Spectrum of diabetic neuropathy: New insights in diagnosis and treatment. Annu Rev Med. (2024) 75:293–306. doi: 10.1146/annurev-med-043021-033114
4. Tan TE, Wong TY. Diabetic retinopathy: Looking forward to 2030. Front endocrinology. (2022) 13:1077669. doi: 10.3389/fendo.2022.1077669
5. Gupta S, Dominguez M, Golestaneh L. Diabetic kidney disease: An update. Med Clin North Am. (2023) 107:689–705. doi: 10.1016/j.mcna.2023.03.004
6. ElSayed NA, Aleppo G, Aroda VR, Bannuru RR, Brown FM, Bruemmer D, et al. 4. Comprehensive medical evaluation and assessment of comorbidities: Standards of care in diabetes—2023. Diabetes Care. (2022) 46:s49–67.2. doi: 10.2337/dc23-S004
7. Poonoosamy J, Lopes P, Huret P, Dardari R, Penfornis A, Thomas C, et al. Impact of intensive glycemic treatment on diabetes complications-A systematic review. Pharmaceutics. (2023) 15:1791. doi: 10.3390/pharmaceutics15071791
8. Eid S, Sas KM, Abcouwer SF, Feldman EL, Gardner TW, Pennathur S, et al. New insights into the mechanisms of diabetic complications: role of lipids and lipid metabolism. Diabetologia. (2019) 62:1539–49. doi: 10.1007/s00125-019-4959-1
9. Won JC, Kwon HS, Moon SS, Chun SW, Kim CH, Park IB, et al. γ-linolenic acid versus α-lipoic acid for treating painful diabetic neuropathy in adults: A 12-week, double-placebo, randomized, noninferiority trial. Diabetes Metab J. (2020) 44:542–54. doi: 10.4093/dmj.2019.0099
10. Jamal GA, Carmichael H. The effect of gamma-linolenic acid on human diabetic peripheral neuropathy: a double-blind placebo-controlled trial. Diabetic medicine: J Br Diabetic Assoc. (1990) 7:319–23. doi: 10.1111/j.1464-5491.1990.tb01397.x
11. Keen H, Payan J, Allawi J, Walker J, Jamal GA, Weir AI, et al. Treatment of diabetic neuropathy with gamma-linolenic acid. The gamma-Linolenic Acid Multicenter Trial Group. Diabetes Care. (1993) 16:8–15. doi: 10.2337/diacare.16.1.8
12. Koehrer P, Saab S, Berdeaux O, Isaïco R, Grégoire S, Cabaret S, et al. Erythrocyte phospholipid and polyunsaturated fatty acid composition in diabetic retinopathy. PLoS One. (2014) 9:e106912. doi: 10.1371/journal.pone.0106912
13. Li JS, Wang T, Zuo JJ, Guo CN, Peng F, Zhao SZ, et al. Association of n-6 PUFAs with the risk of diabetic retinopathy in diabetic patients. Endocr Connect. (2020) 9:1191–201. doi: 10.1530/EC-20-0370
14. Lin SP, Chen CM, Wang KL, Wu KL, Li SC. Association of dietary fish and n-3 unsaturated fatty acid consumption with diabetic nephropathy from a district hospital in Northern Taiwan. Nutrients. (2022) 14:2148. doi: 10.3390/nu14102148
15. Dos Santos ALT, Duarte CK, Santos M, Zoldan M, Almeida JC, Gross JL, et al. Low linolenic and linoleic acid consumption are associated with chronic kidney disease in patients with type 2 diabetes. PLoS One. (2018) 13:e0195249. doi: 10.1371/journal.pone.0195249
16. Tao M, McDowell MA, Saydah SH, Eberhardt MS. Relationship of polyunsaturated fatty acid intake to peripheral neuropathy among adults with diabetes in the National Health and Nutrition Examination Survey (NHANES) 1999 2004. Diabetes Care. (2008) 31:93–5. doi: 10.2337/dc07-0931
17. Durán AM, Beeson WL, Firek A, Cordero-MacIntyre Z, De León M. Dietary omega-3 polyunsaturated fatty-acid supplementation upregulates protective cellular pathways in patients with type 2 diabetes exhibiting improvement in painful diabetic neuropathy. Nutrients. (2022) 14:761. doi: 10.3390/nu14040761
18. Weir NL, Guan W, Karger AB, Klein BEK, Meuer SM, Cotch MF, et al. OMEGA-3 FATTY ACIDS ARE ASSOCIATED WITH DECREASED PRESENCE AND SEVERITY OF DIABETIC RETINOPATHY: A combined analysis of MESA and GOLDR cohorts. Retina (Philadelphia Pa). (2023) 43:984–91. doi: 10.1097/IAE.0000000000003745
19. Sala-Vila A, Díaz-López A, Valls-Pedret C, Cofán M, García-Layana A, Lamuela-Raventós RM, et al. Dietary marine ω-3 fatty acids and incident sight-threatening retinopathy in middle-aged and older individuals with type 2 diabetes: Prospective investigation from the PREDIMED trial. JAMA Ophthalmol. (2016) 134:1142–9. doi: 10.1001/jamaophthalmol.2016.2906
20. Sammons EL, Buck G, Bowman LJ, Stevens WM, Hammami I, Parish S, et al. ASCEND-eye: effects of omega-3 fatty acids on diabetic retinopathy. Ophthalmology. (2024) 131:526–33. doi: 10.1016/j.ophtha.2023.11.030
21. Han E, Yun Y, Kim G, Lee YH, Wang HJ, Lee BW, et al. Effects of omega-3 fatty acid supplementation on diabetic nephropathy progression in patients with diabetes and hypertriglyceridemia. PLoS One. (2016) 11:e0154683. doi: 10.1371/journal.pone.0154683
22. de Boer IH, Zelnick LR, Ruzinski J, Friedenberg G, Duszlak J, Bubes VY, et al. Effect of vitamin D and omega-3 fatty acid supplementation on kidney function in patients with type 2 diabetes: A randomized clinical trial. Jama. (2019) 322:1899–909. doi: 10.1001/jama.2019.17380
23. Emdin CA, Khera AV, Kathiresan S. Mendelian randomization. Jama. (2017) 318:1925–6. doi: 10.1001/jama.2017.17219
24. Skrivankova VW, Richmond RC, Woolf BAR, Davies NM, Swanson SA, VanderWeele TJ, et al. Strengthening the reporting of observational studies in epidemiology using mendelian randomisation (STROBE-MR): explanation and elaboration. Bmj. (2021) 375:n2233. doi: 10.1136/bmj.n2233
25. Ren S, Xue C, Xu M, Li X. Mendelian randomization analysis reveals causal effects of polyunsaturated fatty acids on subtypes of diabetic retinopathy risk. Nutrients. (2023) 15:4208. doi: 10.3390/nu15194208
26. Skrivankova VW, Richmond RC, Woolf BAR, Yarmolinsky J, Davies NM, Swanson SA, et al. Strengthening the reporting of observational studies in epidemiology using mendelian randomization: The STROBE-MR statement. Jama. (2021) 326:1614–21. doi: 10.1001/jama.2021.18236
27. Fry A, Littlejohns TJ, Sudlow C, Doherty N, Adamska L, Sprosen T, et al. Comparison of sociodemographic and health-related characteristics of UK biobank participants with those of the general population. Am J Epidemiol. (2017) 186:1026–34. doi: 10.1093/aje/kwx246
28. Julkunen H, Cichońska A, Slagboom PE, Würtz P. Metabolic biomarker profiling for identification of susceptibility to severe pneumonia and COVID-19 in the general population. eLife. (2021) 10:e63033. doi: 10.7554/eLife.63033.sa2
29. Kurki MI, Karjalainen J, Palta P, Sipilä TP, Kristiansson K, Donner KM, et al. FinnGen provides genetic insights from a well-phenotyped isolated population. Nature. (2023) 613:508–18. doi: 10.1038/s41586-022-05473-8
30. Brion MJ, Shakhbazov K, Visscher PM. Calculating statistical power in Mendelian randomization studies. Int J Epidemiol. (2013) 42:1497–501. doi: 10.1093/ije/dyt179
31. Zhao JH, Stacey D, Eriksson N, Macdonald-Dunlop E, Hedman ÅK, Kalnapenkis A, et al. Genetics of circulating inflammatory proteins identifies drivers of immune-mediated disease risk and therapeutic targets. Nat Immunol. (2023) 24:1540–51. doi: 10.1038/s41590-023-01588-w
32. Burgess S, Butterworth A, Thompson SG. Mendelian randomization analysis with multiple genetic variants using summarized data. Genet Epidemiol. (2013) 37:658–65. doi: 10.1002/gepi.21758
33. Burgess S, Davey Smith G, Davies NM, Dudbridge F, Gill D, Glymour MM, et al. Guidelines for performing Mendelian randomization investigations: update for summer 2023. Wellcome Open Res. (2019) 4:186. doi: 10.12688/wellcomeopenres
34. Bowden J, Davey Smith G, Haycock PC, Burgess S. Consistent estimation in mendelian randomization with some invalid instruments using a weighted median estimator. Genet Epidemiol. (2016) 40:304–14. doi: 10.1002/gepi.21965
35. Burgess S, Thompson SG. Interpreting findings from Mendelian randomization using the MR-Egger method. Eur J Epidemiol. (2017) 32:377–89. doi: 10.1007/s10654-017-0255-x
36. Feldman EL, Callaghan BC, Pop-Busui R, Zochodne DW, Wright DE, Bennett DL, et al. Diabetic neuropathy. Nat Rev Dis primers. (2019) 5:41. doi: 10.1038/s41572-019-0092-1
37. Li J, Zhang X, Zhang Y, Dan X, Wu X, Yang Y, et al. Increased systemic immune-inflammation index was associated with type 2 diabetic peripheral neuropathy: A cross-sectional study in the chinese population. J Inflammation Res. (2023) 16:6039–53. doi: 10.2147/JIR.S433843
38. Li J, Wang X, Jia W, Wang K, Wang W, Diao W, et al. Association of the systemic immuno-inflammation index, neutrophil-to-lymphocyte ratio, and platelet-to-lymphocyte ratio with diabetic microvascular complications. Front endocrinology. (2024) 15:1367376. doi: 10.3389/fendo.2024.1367376
39. Rumora AE, Kim B, Feldman EL. A role for fatty acids in peripheral neuropathy associated with type 2 diabetes and prediabetes. Antioxid Redox Signal. (2022) 37:560–77. doi: 10.1089/ars.2021.0155
40. Liu K, Zou J, Fan H, Hu H, You Z. Causal effects of gut microbiota on diabetic retinopathy: A Mendelian randomization study. Front Immunol. (2022) 13:930318. doi: 10.3389/fimmu.2022.930318
41. Seah JYH, Hong Y, Cichońska A, Sabanayagam C, Nusinovici S, Wong TY, et al. Circulating metabolic biomarkers are consistently associated with type 2 diabetes risk in asian and european populations. J Clin Endocrinol Metab. (2022) 107:e2751–e61. doi: 10.1210/clinem/dgac212
42. Andreasson R, Ekelund C, Landin-Olsson M, Nilsson C. HbA1c levels in children with type 1 diabetes and correlation to diabetic retinopathy. J Pediatr Endocrinol Metab. (2018) 31:369–74. doi: 10.1515/jpem-2017-0417
43. Salardi S, Porta M, Maltoni G, Bassi M, Minuto N, D'Annunzio G, et al. Decreasing prevalence of retinopathy in childhood-onset type 1 diabetes over the last decade: A comparison of two cohorts diagnosed 10 years apart. Diabetes Obes Metab. (2021) 23:1950–5. doi: 10.1111/dom.14438
44. Wang H, Guo Z, Xu Y. Association of monocyte-lymphocyte ratio and proliferative diabetic retinopathy in the U.S. population with type 2 diabetes. J Transl Med. (2022) 20:219. doi: 10.1186/s12967-022-03425-4
45. Yue T, Shi Y, Luo S, Weng J, Wu Y, Zheng X. The role of inflammation in immune system of diabetic retinopathy: Molecular mechanisms, pathogenetic role and therapeutic implications. Front Immunol. (2022) 13:1055087. doi: 10.3389/fimmu.2022.1055087
46. Andersson K, Halling A, Agvall B. Factors associated with development of retinopathy in patients with type 2 diabetes mellitus at onset and within three years after diagnosis. Scand J Prim Health Care. (2024) 22:1–7. doi: 10.1080/02813432.2024.2329215
47. Gholami Chahkand MS, Esmaeilpour Moallem F, Qezelgachi A, Seifouri K, Pesaran Afsharian A, Sheikhzadeh F, et al. Lipoprotein (a) as a predictor of diabetic retinopathy in patients with type 2 diabetes: A systematic review. Diabetes Vasc Dis Res. (2023) 20:14791641231197114. doi: 10.1177/14791641231197114
48. Wang Q, Zeng N, Tang H, Yang X, Yao Q, Zhang L, et al. Diabetic retinopathy risk prediction in patients with type 2 diabetes mellitus using a nomogram model. Front endocrinology. (2022) 13:993423. doi: 10.3389/fendo.2022.993423
49. Catherine Prater M, Polley KR, Cooper JA. Improvements in markers of inflammation and coagulation potential following a 5-day high-fat diet rich in cottonseed oil vs. Olive Oil healthy males. Cytokine. (2024) 175:156494. doi: 10.1016/j.cyto.2023.156494
50. Belury MA. Linoleic acid, an omega-6 fatty acid that reduces risk for cardiometabolic diseases: premise, promise and practical implications. Curr Opin Clin Nutr Metab Care. (2023) 26:288–92. doi: 10.1097/MCO.0000000000000919
51. Innes JK, Calder PC. Omega-6 fatty acids and inflammation. Prostaglandins Leukot Essent Fatty Acids. (2018) 132:41–8. doi: 10.1016/j.plefa.2018.03.004
52. Hussein N, Ah-Sing E, Wilkinson P, Leach C, Griffin BA, Millward DJ. Long-chain conversion of [13C]linoleic acid and alpha-linolenic acid in response to marked changes in their dietary intake in men. J Lipid Res. (2005) 46:269–80. doi: 10.1194/jlr.M400225-JLR200
53. Sarkkinen ES, Agren JJ, Ahola I, Ovaskainen ML, Uusitupa MI. Fatty acid composition of serum cholesterol esters, and erythrocyte and platelet membranes as indicators of long-term adherence to fat-modified diets. Am J Clin Nutr. (1994) 59:364–70. doi: 10.1093/ajcn/59.2.364
54. Virtanen JK, Mursu J, Voutilainen S, Tuomainen TP. The associations of serum n-6 polyunsaturated fatty acids with serum C-reactive protein in men: the Kuopio Ischaemic Heart Disease Risk Factor Study. Eur J Clin Nutr. (2018) 72:342–8. doi: 10.1038/s41430-017-0009-6
55. Takala R, Ramji DP, Andrews R, Zhou Y, Farhat M, Elmajee M, et al. Pinolenic acid exhibits anti-inflammatory and anti-atherogenic effects in peripheral blood-derived monocytes from patients with rheumatoid arthritis. Sci Rep. (2022) 12:8807. doi: 10.1038/s41598-022-12763-8
56. Aryan Z, Ghajar A, Faghihi-Kashani S, Afarideh M, Nakhjavani M, Esteghamati A. Baseline high-sensitivity C-reactive protein predicts macrovascular and microvascular complications of type 2 diabetes: A population-based study. Ann Nutr Metab. (2018) 72:287–95. doi: 10.1159/000488537
57. Atlı H, Onalan E, Yakar B, Duzenci D, Dönder E. Predictive value of inflammatory and hematological data in diabetic and non-diabetic retinopathy. Eur Rev Med Pharmacol Sci. (2022) 26:76–83. doi: 10.26355/eurrev_202201_27750
58. Riaz A, Asghar S, Shahid S, Tanvir H, Ejaz MH, Akram M. Prevalence of metabolic syndrome and its risk factors influence on microvascular complications in patients with type 1 and type 2 diabetes mellitus. Cureus. (2024) 16:e55478. doi: 10.7759/cureus.55478
59. Rus M, Crisan S, Andronie-Cioara FL, Indries M, Marian P, Pobirci OL, et al. Prevalence and risk factors of metabolic syndrome: A prospective study on cardiovascular health. Medicina (Kaunas Lithuania). (2023) 59:1711. doi: 10.3390/medicina59101711
60. Hajihashemi P, Feizi A, Heidari Z, Haghighatdoost F. Association of omega-6 polyunsaturated fatty acids with blood pressure: A systematic review and meta-analysis of observational studies. Crit Rev Food Sci Nutr. (2023) 63:2247–59. doi: 10.1080/10408398.2021.1973364
61. Mazidi M, Shekoohi N, Katsiki N, Banach M. Omega-6 fatty acids and the risk of cardiovascular disease: insights from a systematic review and meta-analysis of randomized controlled trials and a Mendelian randomization study. Arch Med Sci. (2022) 18:466–79. doi: 10.5114/aoms/136070
62. Beyene HB, Olshansky G, Giles C, Huynh K, Cinel M, Mellett NA, et al. Lipidomic signatures of changes in adiposity: A large prospective study of 5849 adults from the Australian diabetes, obesity and lifestyle study. Metabolites. (2021) 11:646. doi: 10.3390/metabo11090646
63. Pandit S, Ho AC, Yonekawa Y. Recent advances in the management of proliferative diabetic retinopathy. Curr Opin Ophthalmol. (2023) 34:232–6. doi: 10.1097/ICU.0000000000000946
64. Burgess S, Bowden J, Fall T, Ingelsson E, Thompson SG. Sensitivity analyses for robust causal inference from mendelian randomization analyses with multiple genetic variants. Epidemiol (Cambridge Mass). (2017) 28:30–42. doi: 10.1097/EDE.0000000000000559
Keywords: diabetic microvascular complications, polyunsaturated fatty acids, Mendelian randomization, omega-6, omega-3, diabetic neuropathy, diabetic retinopathy, diabetic kidney disease
Citation: Liu B, Liu R, Gu Y, Shen X, Zhou J and Luo C (2024) Polyunsaturated fatty acids and diabetic microvascular complications: a Mendelian randomization study. Front. Endocrinol. 15:1406382. doi: 10.3389/fendo.2024.1406382
Received: 25 March 2024; Accepted: 25 July 2024;
Published: 07 August 2024.
Edited by:
John D. Imig, University of Arkansas for Medical Sciences, United StatesReviewed by:
Kangcheng Liu, Affiliated Eye Hospital of Nanchang University, ChinaCosmin Mihai Vesa, University of Oradea, Romania
Copyright © 2024 Liu, Liu, Gu, Shen, Zhou and Luo. This is an open-access article distributed under the terms of the Creative Commons Attribution License (CC BY). The use, distribution or reproduction in other forums is permitted, provided the original author(s) and the copyright owner(s) are credited and that the original publication in this journal is cited, in accordance with accepted academic practice. No use, distribution or reproduction is permitted which does not comply with these terms.
*Correspondence: Chun Luo, lhlluochun@nbu.edu.cn