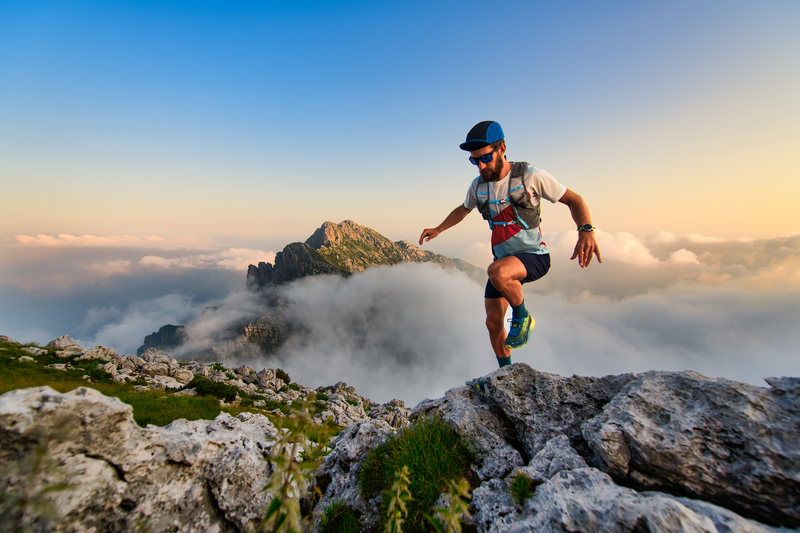
95% of researchers rate our articles as excellent or good
Learn more about the work of our research integrity team to safeguard the quality of each article we publish.
Find out more
ORIGINAL RESEARCH article
Front. Endocrinol. , 26 November 2024
Sec. Clinical Diabetes
Volume 15 - 2024 | https://doi.org/10.3389/fendo.2024.1397423
This article is part of the Research Topic Women in Clinical Diabetes View all 15 articles
Background: Although the association between HHEX, IGF2BP2, and FTO polymorphisms and the risk of GDM has been investigated in several studies, the findings have been inconsistent across different populations. The study aimed to investigate the association between genetic polymorphisms and GDM risk in a Chinese population.
Methods: 502 control volunteers and 500 GDM patients were enrolled. IGF2BP2 rs11705701 and rs4402960, FTO rs9939609, and HHEX rs1111875 and rs5015480 were all genotyped using the SNPscan™ genotyping assay. The independent sample t-test, logistic regression, and chi-square test were used to assess the variations in genotype and allele and their relationships with the risk of GDM. The blood glucose level, gestational week of delivery, and newborn weight were compared using a one-way ANOVA.
Results: After adjusting for confounding factors, the results show that the rs1111875 heterozygous (OR=1.370; 95% CI: 1.040-1.805; P = 0.025) and overdominant (OR=1.373; 95% CI: 1.049-1.796; P = 0. 021) models are significantly associated with an increased risk of GDM, especially for the age ≥ 30 years group: heterozygote (OR=1.646; 95% CI: 1.118-2.423; P=0.012) and overdominant (OR=1.553; 95% CI: 1.064-2.266; P = 0.022) models. In the age ≥ 30 years, the rs5015480 overdominant model (OR=1.595; 95% CI: 1.034-2.459; P = 0.035) and the rs9939609 heterozygote model (OR=1.609; 95% CI: 1.016-2.550; P=0.043), allele (OR=1. 504; 95% CI: 1.006-2.248; P = 0.047), dominant model (OR=1.604; 95% CI: 1.026-2.505; P = 0.038), and overdominant model (OR=1.593; 95% CI: 1.007-2.520; P = 0.047) were associated with a significantly increased risk of GDM; Additionally, people with the TC genotype of rs1111875 had a substantially higher 1-hour blood glucose level than TT genotype (P < 0.05). The results of the meta-analysis showed that the A allele of rs11705701 was associated with an increased risk of diabetes mellitus (P < 0.05).
Conclusion: The study indicates that the TC genotype of rs1111875 is linked to a higher risk of GDM, particularly in women aged 30 years or older. Additionally, rs5015480 and rs9939609 were significantly associated with GDM in the same age group. These SNPs may therefore be more closely linked to GDM in older mothers.
Gestational diabetes mellitus (GDM) as diabetes diagnosed during pregnancy that is not clearly overt diabetes. It is a common disease in pregnancy that is determined by the first diagnosis of hyperglycemia (1, 2). It is associated with hypertensive disorders of pregnancy, macrosomia, cesarean section, and neonatal complications (3). The prevalence of GDM ranges from 1.8% to 25.1% globally (4). GDM is a multifactorial complex metabolic disorder influenced by genetic and environmental factors, similar to type 2 diabetes mellitus (T2DM). Genetics is crucial to GDM and is not easily modifiable through intervention (5). Studies have demonstrated that several genes linked to T2DM risk are also related to GDM, and polymorphisms in HHEX, IGF2BP2, and FTO have been linked to decreased β-cell function and diabetes risk (6–11).
The hematopoietically expressed homeobox (HHEX) gene is situated in the 270 kb linkage disequilibrium (LD) region of human chromosome 10, q23.33. It plays a regulatory role in insulin secretion and diabetes mellitus. The LD block comprises three genes: the kinase family member 11 gene, the insulin- degrading enzyme gene, and HHEX. The regions rs1111875 and rs5015480 are closest to HHEX, which has been linked to diabetes mellitus (DM), and are situated close to the LD region (12–15). Moreover, previous studies have shown that polymorphisms in the insulin-like growth factor 2 mRNA binding protein 2 (IGF2BP2) gene may be a risk factor for obesity and T2DM (10, 16). IGF2BP2 belongs to a family of messenger ribonucleic acid-binding proteins that regulate the translation of IGF2 (17). IGF2BP2 promotes the release of insulin and is essential for the growth and development of pancreatic β-cells. Alpha-ketoglutarate-dependent dioxygenase, or FTO, is involved in energy balance, and lipid and carbohydrate metabolism. T2DM and obesity have been associated with variations in the FTO gene (11, 18–20).
Some research works have investigated the relationship between the risk of GDM and HHEX rs1111875 and rs5015480, IGF2BP2 rs11705701 and rs4402960, and FTO rs9939609 (21, 22). Nevertheless, the outcomes have displayed variability. The objective of this study was to examine the relationship between gene polymorphisms (rs1111875 and rs5015480, rs11705701 and rs4402960, and rs9939609) and the risk of GDM in the Chinese population. Additionally, our objective was to examine the connections between gene polymorphisms and clinical parameters, such as glycemia, week of gestation, and newborn weight.
With a total of 1002 participants, the study involved 500 patients with GDM and 502 healthy pregnant women as controls. The study protocol for this research was approved by the Ethics Committee of Shunde Women and Children’s Hospital at Guangdong Medical University (the ethical approval number: 2020072), and subjects were selected based on specific criteria: (i) Han ethnicity; (ii) age ≥ 18 years; (iii) voluntary informed consent; (iv) never diagnosed with diabetes; (v) no glucose-lowering medication; and (vi) no pregnancy complications. Participants were excluded if they had previously been diagnosed with diabetes, were under 18 years of age, had pregnancy complications, or were taking glucose-lowering medication. A total of 1002 pregnant Chinese Han women provided voluntary informed consent. According to the diagnostic criteria established by the International Association of Diabetes and Pregnancy Study Groups (IADPSG), pregnant women underwent an oral glucose tolerance test (OGTT) between weeks 24 and 28 of gestation. In cases where at least one glucose level measurement equals or exceeds the threshold value, the subject was considered positive for GDM. Subjects with GDM were identified through the assessment of their blood glucose levels: fasting blood glucose (FBG) ≥ 5.1 mmol/L, or 1-hour postprandial glucose (1h-PG) ≥ 10.0 mmol/L, or 2-hour postprandial glucose (2h-PG) ≥ 8.5 mmol/L. Healthy controls were defined as individuals with normoglycemic levels. The study was carried out in compliance with the principles of the Declaration of Helsinki.
In the study, general clinical information was compiled, encompassing age, ethnicity, height, systolic blood pressure (SBP), diastolic blood pressure (DBP), prepregnancy weight, and parity (primipara or multipara). The prepregnancy body mass index (pre-BMI, Kg/m2) was computed as the ratio of prepregnancy weight (Kg) to the square of the height (m2). The pre-BMI categorizes Chinese individuals into the following groups based on their weight: Obesity is defined as having a BMI equal to or greater than 28 Kg/m2, overweight falls within the range of 24 Kg/m2 to less than 28 Kg/m2, normal weight is classified between 18.5 Kg/m2 and less than 24 Kg/m2, and underweight is indicated by a BMI of less than 18.5 Kg/m2.
Extraction of genomic DNA was conducted utilizing the QIAamp DNA blood kit (Qiagen, Germany), followed by genotyping of individual SNPs through the SNPscan method. The resulting raw data were acquired using an ABI3730XL sequencer and processed using GeneMapper 4.1 software (Applied Biosystems, USA) by Genesky Technologies Inc. (Shanghai, China). Rigorous quality control protocols were enforced to ensure the precision of the genotyping results.
Statistical analyses were conducted using SPSS 20.0 software (SPSS, Chicago, IL, USA). Continuous variables were compared using an independent sample t-test, presenting the results as mean ± standard deviation. Discontinuous variables, such as Hardy-Weinberg equilibrium (HWE) in the control group, were analyzed using chi-square tests. The association between SNP and GDM risk was evaluated through binary logistic regression analysis, adjusting for potential confounders like pre-BMI, age, parity, and blood pressure. The results were expressed as a odds ratio (OR) with a 95% confidence interval (CI). We used one-way ANOVA to analyze the correlation between SNP and blood glucose levels, gestational week of labor, and neonatal weight. For significant one-way ANOVA results, we continued with the least significant difference (LSD) comparisons. Subgroup analyses for age and pre-BMI were also performed. Heterogeneity was estimated using Q-test and I2 test. No heterogeneity was defined as I2 < 50% and P > 0.1, STATA v.16.0 software (Stata Corporation, Texas, United States) was used to perform heterogeneity analyses. A statistically significant result was observed for bilateral P < 0.05.
A systematic search was conducted across PubMed, Chinese National Knowledge Infrastructure, and Google Scholar databases using various combinations of the terms rs11705701, Gestational diabetes mellitus (GDM), type 2 diabetes mellitus (T2DM), type 1 diabetes mellitus (T1DM), and prediabetes mellitus (Pre-DM, without any restrictions. Inclusion criteria encompassed case-control or cohort studies investigating the relationship between rs11705701 and GDM, T2DM, T1DM, or Pre-DM, and providing adequate raw data. Studies not meeting diagnostic criteria or exhibiting data deviating from HWE were excluded. Data extraction was overseen by two authors. The meta-analysis, utilizing fixed or random effects models based on heterogeneity levels, was conducted across six genetic models. Publication bias was evaluated through Egger’s and Begg’s tests. All statistical analyses were performed using STATA v.16.0 software (Stata Corporation, TX, USA).
The online tool PROMO (https://alggen.lsi.upc.es/home.html) was utilized to investigate whether rs11705701 located in the promoter region of the IGF2BP2 gene impacts the binding sites of transcription factors (23, 24).
In this case-control research, 502 healthy controls and 500 GDM patients were examined. The genotypes of FTO rs9939609, IGF2BP2 rs11705701 and rs4402960, and HHEX rs1111875 and rs5015480 were studied. The clinical baseline data is shown in Table 1. In comparison to the control group, the GDM group exhibited substantially higher mean age, pre-BMI, SBP, DBP, and blood glucose levels (P < 0.05). Furthermore, there was a significant difference (P < 0.05) in the parity between the GDM group and the control group.
The HWE analysis and minor allele frequencies (MAF) for the five SNPs in the control group are shown in Table 2. Except rs11705701, the results were consistent with HWE (P > 0.05), so no comparative analyses were carried out for this SNP. Using six models (homozygous, heterozygous, allele, dominant, recessive, and overdominant) the correlation between genotype and GDM was assessed, and the (unadjusted and adjusted) OR and 95% CI were computed for each SNP. Before adjustment, the results indicated that the rs1111875 heterozygous (TC vs. TT: OR=1.349; 95% CI: 1.039-1.753; P = 0.025) and overdominant (TC vs. TT+CC: OR=1.352; 95% CI: 1.047-1.744; P = 0.021) models were associated with an increased risk of GDM. After adjusting for pre-BMI, age, DBP, SBP, and parity, the results showed that the rs1111875 heterozygous (TC vs. TT: OR=1.370; 95% CI: 1.040-1.805; P = 0.025) and overdominant (TC vs. TT+CC: OR=1.373; 95% CI: 1.049-1.796; P = 0.021) models were significantly associated with an increased risk of GDM (Figure 1). Nevertheless, rs5015480, rs4402960, and rs9939609 did not significantly correlate with the GDM risk (P > 0.05, Figures 2, 3; Supplementary Table S1).
The relationship between the four SNPs and the risk of GDM was next examined using stratified analysis based on age or pre-BMI. In the group with an age of 30 or above, before adjustment, the GDM risk was significantly higher in the rs1111875 dominant (CC+TC vs. TT: OR=1.685; 95% CI: 01.173-2.421; P = 0.005), overdominant (TC vs. TT+CC: OR=1.566; 95% CI: 1.082-2.269; P = 0.018), heterozygote (TC vs. TT: OR=1.669; 95% CI: 1.143-2.438; P = 0.008) and allele (C vs. T: OR=1.481; 95% CI: 1.106-1.983; P = 0.008) models (Figure 1); A significantly higher risk of GDM was seen in the rs5015480 heterozygote (TC vs. TT: OR=1.581; 95% CI: 1.031-2.425; P = 0.036) and overdominant (TC vs. TT+CC: OR=1.631; 95% CI: 1.066-2.494; P = 0.024) models (Figure 2); There was no discernible association between rs9939609, rs4402960 and the risk of GDM (P > 0.05, Figure 3; Supplementary Table S1). After adjusting for pre-BMI, age, SBP, the results of the rs1111875 heterozygote (TC vs. TT: OR=1.646; 95%CI:1.118-2.423; P = 0.012) and overdominant (TC vs. TT+CC: OR=1.553; 95% CI: 1.064-2.266; P = 0.022), allele (C vs. T: OR=1.454; 95% CI: 1.079-1.958; P = 0.014) and dominant (CC+TC vs. TT: OR=1.653; 95% CI: 1.142-2.392; P = 0.008) models remained significantly associated with increased GDM risk (Figure 1); The rs5015480 overdominant (TC vs. TT+CC: OR=1.595; 95% CI: 1.034-2.459; P = 0.035) model showed a significantly increased GDM risk (Figure 2). The rs9939609 heterozygote (TA vs. TT: OR=1.609; 95% CI: 1.016-2.550; P = 0.043), allele (A vs. T: OR=1.504; 95% CI: 1.006-2.248; P = 0.047), dominant (AA+TA vs. TT: OR=1.604; 95% CI: 1.026-2.505; P = 0.038) and overdominant (TA vs. TT+AA: OR=1.593; 95% CI: 1.007-2.520; P = 0.047) models showed a significantly increased GDM risk (Figure 3); Nevertheless, rs4402960 did not significantly correlate with the risk of GDM (P > 0.05, Supplementary Table S1). In additon, In subjects less than 30 years of age, pre-BMI < 18.5, 18.5 ≤ pre-BMI <24, and pre-BMI ≥ 24 groups, no significant correlation with GDM risk was found for any SNP (P > 0.05, Figures 1-3; Supplementary Table S1; Supplementary Figures S1–S3).
To confirm that the aforementioned associations were related to age rather than differences between different age groups, we conducted a heterogeneity analysis across different age groups. We found that after adjusting for confounding factors, the heterogeneity among different age groups in various genetic models for rs1111875 and rs5015480 was reduced compared to before adjustment (Figures 4, 5), especially for the overdominant (TC Vs. CC+TT: I2 = 0.0%, P = 0.379) and heterozygous (TC Vs. TT: I2 = 40.9%, P = 0.193) models of rs1111875 (Figure 4), where no significant heterogeneity was observed. This strongly suggests that the association of rs1111875 with an increased risk of GDM is related to age, particularly in pregnant women aged 30 or older. The high heterogeneity observed across different age groups for other SNPs genetic models may be related to insufficient sample size or other confounding factors that were not fully adjusted (Figures 5, 6).
Figure 4. Heterogeneity analysis of various genetic models of rs1111875 among different age groups. (A) Unadjusted results, (B) Adjusted results.
Figure 5. Heterogeneity analysis of various genetic models of rs5015480 among different age groups. (A) Unadjusted results, (B) Adjusted results.
Figure 6. Heterogeneity analysis of various genetic models of rs9939609 among different age groups. (A) Unadjusted results, (B) Adjusted results.
In overall, no significant association was found between any genotype and blood glucose levels in any SNP (Supplementary Table S2). The 1-hour PG level of the TC genotype of rs1111875, however, were considerably greater than those of the TT genotype in participants over 30 years of age (P < 0.05, Table 3). In the pre-BMI < 18.5 group, those with the TC genotype of rs1111875 had a substantially higher 2-hour postprandial glucose level than people with the CC genotype (P < 0.05). Furthermore, people with the CC genotype of rs5015480 had a substantially lower 2-hour postprandial glucose level than people with the TT and TC genotypes (P < 0.05, Table 3). Individuals with the CC genotype of rs5015480 had a lower FBG level in the pre-BMI ≥ 24 group than those with the TC and TT genotypes (P < 0.05, Table 3). Furthermore, PG levels at 1 and 2 hours were considerably greater in persons with the TA genotype of rs9939609 than in those with the TT genotype (P < 0.05, Table 3). No significant association was found between genotype and blood glucose levels in other groups (P > 0.05, Supplementary Table S2).
Table 3. Association between genotype and blood glucose level, gestational week of labor and neonatal weight.
In all groups, there was no discernible relationship between any genotype and the gestational week of labor (P > 0.05, Table 3; Supplementary Table S2).
In all groups, there were no significant differences between genotypes and newborn weight (P > 0.05, Table 3; Supplementary Table S2).
We performed a meta-analysis of published research to gain a better understanding of the relationship between rs11705701 and diabetes because the control group in our study did not follow HWE. Four studies were included in the final analysis: two studies about rs11705701 and T2DM, one study about rs11705701 and GDM, and one study about rs11705701 and prediabetes mellitus (Pre-DM). The features of the research are displayed in Supplementary Table S3. Figure 7 illustrates the associations found in the overall analysis between the various models and increased risk of diabetes mellitus. The dominant (AA+GA vs. GG: OR=1.218; 95% CI: 1.088-1.364; P = 0.001), homozygous (AA vs. GG: OR=1.472; 95% CI: 1.023-2.119; P = 0.037), heterozygous (GA vs. GG: OR = 1.153; 95% CI: 1.024-1.298; P = 0.019), and allele (A vs. G: OR=1.202; 95% CI: 1.106-1.307; P < 0.001) models demonstrated associations with increased risk of diabetes. In other groups, there was no discernible difference (P > 0.05, Figure 7). The funnel plot was shown to be symmetrical (P > 0.05, Figure 8). Egger’s tests yielded consistent results (all P > 0.05), indicating the absence of publication bias.
Figure 7. Meta-analysis for the association between the IGF2BP2 rs11705701 and GDM susceptibility. (A) Dominant model, AA+GA vs.GG (fixed effects mode); (B) Recessive model, AA vs.GA+GG (random effects model); (C) Overdominant model, GA vs.AA+GG (fixed effects model); (D) Homozygote model: AA vs. GG (random effects model); (E) Heterozygote model: GA vs. GG (fixed effects model); (F) Allele model, A vs. G (fixed effects model). OR, odds ratio; CI, confidence interval; I-squared, measure to quantify the degree of heterogeneity in meta-analyses.
Figure 8. Funnel plot of the odds ratios in the meta-analysis. (A) Dominant model, AA+GA vs.GG; (B) Recessive model, AA vs.GA+GG; (C) Overdominant model, GA vs.AA+GG; (D) Homozygote model: AA vs. GG; (E) Heterozygote model: GA vs. GG; (F) Allele model, A vs. G.
To investigate if SNPs in the IGF2BP2 gene’s promoter affect particular transcription factor binding locations, the PROMO database was consulted. SNPs have an impact on the binding of pertinent transcription factors, as seen in Figure 9. It was discovered that the binding of GR-alpha and E2F-1 transcription factors was impacted by rs11705701 G > A. It was discovered that the rs11705701 A allele binds exclusively to the GR-alpha transcription factor, while the rs11705701 G allele preferentially binds to the E2F-1 transcription factor.
Figure 9. IGF2BP2 rs11705701 G > A transcription factor prediction. rs11705701 G > A affects the binding of GR-alpha and E2F-1 transcription factors. (A) rs11705701 (reference); (B) rs11705701 (mutant).
Variants in genetic composition can alter how encoded proteins are expressed and function, which can have a broad range of physiological effects. Thus, polymorphisms might be clinically significant for a range of diseases (25). A higher risk of GDM has been linked to alleles in HHEX, IGF2BP2, and FTO (22, 26). We studied the Chinese population’s susceptibility to GDM using HHEX rs1111875 and rs5015480, IGF2BP2 rs11705701 and rs4402960, and FTO rs9939609. Figure 1 shows that there was a considerable increase in the likelihood of developing GDM due to the rs1111875 TC in HHEX. According to a recent meta-analysis, the rs1111875 CC and CT genotype group had a 50% and 29% higher risk of GDM than the TT genotype population, respectively (27). Similar to our results, Benny et al. discovered that HHEX rs1111875 was substantially related to GDM susceptibility (28). A meta-analysis revealed that the HHEX rs5015480 C allele was linked to GDM susceptibility, and the same rs5015480 polymorphism was found to be similarly associated with T2DM in a study of T2DM (29). Furthermore, we discovered that the overdominant model in HHEX rs5015480 was significantly associated with GDM in the age ≥ 30 years group.
The HHEX gene rs1111875 and rs5015480 have been identified as typical loci linked with diabetes since GWAS started to validate candidate gene research in various ethnic groups (12–15). The IDE, KIF11, and HHEX genes are found on human chromosome 10 and are positioned in the LD region at q23.33, which is home to the HHEX gene. The HHEX-KIF-IDE region, which is closest to the HHEX gene, has the C/T variants rs1111875 and rs5015480. These variants have been linked to pancreatic embryonic development and may have an impact on future insulin secretion. In this study, we observed a significant association between HHEX SNPs and susceptibility to GDM, particularly among individuals aged 30 years and older. Furthermore, individuals with the TC genotype exhibited significantly higher 1-hour glucose levels compared to the TT genotype. This finding suggests that genetic variations in the HHEX gene may contribute to β-cell dysfunction, thereby influencing the onset of diabetes mellitus. The HHEX rs1111875 is implicated in the Wingless-type MMTV integration site (WNT) signaling pathway, impacting susceptibility to diabetes. This SNP encodes transcription factors that modulate gene expression in cellular processes, influencing cell development and growth (30, 31). Similarly, among the Greek Cypriots, the HHEX rs5015480 has been linked to altered insulin secretion, cellular function, and diabetes susceptibility. The HHEX rs1111875 and rs5015480, have been associated with diabetes risk across diverse populations, primarily due to their role in reducing β-cell response and insulin secretion (30, 32–35). Importantly, HHEX gene polymorphisms are believed to influence insulin production and secretion (36, 37).
Dysregulation of IGF2BP2 has been linked to various metabolic diseases and cancers (38, 39). Notably, the IGF2BP2 SNP is associated with both T2DM and cancer (38). In 2007, Grarup et al. reported no association between IGF2BP2 gene variants and pancreatic cell dysfunction in a Danish cohort (40). Subsequent research revealed that IGF2BP2 variants diminish glucose-stimulated insulin secretion in the initial phase of diabetes progression, indicating an impact on pancreatic β-cell function (41–43). The IGF2BP2 rs11705701 G/A variant, situated in the -1479 locus of the promoter region, has been linked to reduced body fat and insulin resistance in Mexican Americans, elevating the susceptibility to T2DM (44). Moreover, this variant is associated with a heightened T2DM risk in the Russian population, with allele A correlating with a truncated IGF2BP2 protein in the adipose tissue of non-obese individuals (45). Furthermore, the rs11705701 variant exhibits a strong association with female prediabetic patients (46). While no significant association was observed between IGF2BP2 rs11705701 and the risk of GDM in the Polish population, it is linked to prolonged gestation and enhanced neonatal health, as indicated by Apgar scores (47). Collectively, these findings suggest a potential role for IGF2BP2 rs11705701 in diabetes development. Comparative analyses were not conducted with the control group in our study due to non-compliance with Hardy-Weinberg equilibrium. Nonetheless, through a meta-analysis of relevant previously published studies, we identified a significant association between the rs11705701 A variant and an increased risk of developing diabetes mellitus. Utilizing a transcription factor prediction analysis website, we further investigated the impact of rs11705701 variants on promoter activity. Our analysis revealed specific binding patterns, with rs11705701 G binding E2F1 and rs11705701 A binding GR-alpha transcription factor. This suggests that IGF2BP2 rs11705701 may regulate its transcriptional activity by modulating transcription factor binding. Subsequent molecular biology experiments are warranted to confirm these findings. Additionally, our results suggested that IGF2BP2 rs4402960 was not associated with the risk of GDM, consistent with recent studies and comprehensive quantitative meta-analyses (48–50).
FTO proteins function within the nucleus to remove N6-methyladenosine modifications from mRNA, thereby influencing the splicing of genes crucial for adipogenesis (51). Notably, variations located in the initial intron of the FTO gene have been linked to elevated BMI and T2DM, with a 47 kb genomic segment identified to harbor multiple SNPs associated with these conditions. Among these, the rs9939609 variant has been extensively researched (52). The precise mechanism through which this SNP contributes to obesity remains elusive. Nevertheless, individuals heterozygous for rs9939609 exhibit heightened levels of primary FTO transcripts in the risk A allele compared to the T allele (53), potentially resulting in increased FTO expression that promotes adipogenesis. Notably, this latter association has not been documented in existing literature. The FTO rs9939609, situated in the first intron of the gene, has been linked to a heightened risk of GDM in Caucasian populations. Our study revealed a significant association between carriers of the A allele and an increased risk of GDM in individuals aged ≥ 30. Furthermore, the A allele was found to be correlated with accelerated weight gain during pregnancy. Additionally, our investigation demonstrated elevated 1-hour and 2-hour glucose levels in the OGTT among individuals with the TA genotype compared to the TT genotype in the pre-BMI ≥ 24 group. But some studies describing the absence of association of FTO (rs9939609) and GDM risk (49, 50), this was consistent with our overall analysis results. These genetic variations may potentially impact FTO expression or enzyme activity, resulting in metabolic alterations that disrupt glucose metabolism and induce insulin resistance, consequently heightening the susceptibility to GDM.
The modest sample size of the GDM and control groups necessitates validation of our observations in a larger cohort in future studies. The scope of the study was limited to Chinese individuals, underscoring the need for further research to confirm our findings in diverse populations.
In conclusion, our study revealed an elevated risk of GDM associated with the TC genotype of the HHEX gene rs1111875, particularly among individuals aged ≥ 30. Additionally, rs5015480 and rs9939609 showed significant correlations with GDM in the same age group. These findings suggest a potentially stronger link between these specific SNPs and GDM among women of advanced maternal age.
The datasets presented in this study can be found in online repositories. The names of the repository/repositories and accession number(s) can be found in the article/Supplementary Material.
The studies involving humans were approved by Shunde Women and Children’s Hospital of Guangdong Medical University (Maternity and Child Healthcare Hospital of Shunde Foshan). The studies were conducted in accordance with the local legislation and institutional requirements. The participants provided their written informed consent to participate in this study.
QZ: Writing – review & editing, Writing – original draft. JL: Writing – original draft. XL: Writing – original draft. NL: Writing – original draft. WW: Writing – original draft. RGy: Writing – original draft. DZ: Writing – original draft. YW: Writing – original draft. RGu: Writing – review & editing, Writing – original draft.
The author(s) declare that financial support was received for the research, authorship, and/or publication of this article. Support from the National Natural Science Foundation of China (81873649); Doctoral scientific research Initiate funding project of Shunde Women and Children’s Hospital of Guangdong Medical University (Maternity and Child Healthcare Hospital of Shunde Foshan) (2020BSQD007); Self-financing science and technology project of Foshan (2320001006049).
The authors declare that the research was conducted in the absence of any commercial or financial relationships that could be construed as a potential conflict of interest.
All claims expressed in this article are solely those of the authors and do not necessarily represent those of their affiliated organizations, or those of the publisher, the editors and the reviewers. Any product that may be evaluated in this article, or claim that may be made by its manufacturer, is not guaranteed or endorsed by the publisher.
The Supplementary Material for this article can be found online at: https://www.frontiersin.org/articles/10.3389/fendo.2024.1397423/full#supplementary-material
Supplementary Figure 1 | The associations between rs1111875 and GDM risk in different pre-BMI subgroups. *adjusted.
Supplementary Figure 2 | The associations between rs5015480 and GDM risk in different pre-BMI subgroups. *adjusted.
Supplementary Figure 3 | The associations between rs9939609 and GDM risk in different pre-BMI subgroups. *adjusted.
1. Popova P, Castorino K, Grineva E, Kerr D. Gestational diabetes mellitus diagnosis and treatment goals: Measurement and measures. Minerva Endocrinologica. (2016) 41:421–32. doi: 10.1055/a-1284-6011
2. American Diabetes Association Professional Practice Committee. 2. Diagnosis and classification of diabetes: standards of care in diabetes-2024. Diabetes Care. (2024) 47:S20–42. doi: 10.2337/dc24-S002
3. Kim C. Gestational diabetes: risks, management, and treatment options. Int J Women’s Health. (2010) 2:339–51. doi: 10.2147/IJWH.S13333
4. Zhu Y, Zhang C. Prevalence of gestational diabetes and risk of progression to type 2 diabetes: a global perspective. Curr Diabetes Rep. (2016) 16:7. doi: 10.1007/s11892-015-0699-x
5. Chen P, Wang S, Ji J, Ge A, Chen C, Zhu Y, et al. Risk factors and management of gestational diabetes. Cell Biochem Biophysics. (2015) 71:689–94. doi: 10.1007/s12013-014-0248-2
6. Kwak SH, Choi SH, Jung HS, Cho YM, Lim S, Cho NH, et al. Clinical and genetic risk factors for type 2 diabetes at early or late post partum after gestational diabetes mellitus. J Clin Endocrinol Metab. (2013) 98:E744–52. doi: 10.1210/jc.2012-3324
7. Wang X, Li W, Ma L, Ping F, Liu J, Wu X, et al. Investigation of miRNA-binding site variants and risk of gestational diabetes mellitus in Chinese pregnant women. Acta Diabetologica. (2017) 54:309–16. doi: 10.1007/s00592-017-0969-y
8. Palmer ND, Goodarzi MO, Langefeld CD, Ziegler J, Norris JM, Haffner SM, et al. Quantitative trait analysis of type 2 diabetes susceptibility loci identified from whole genome association studies in the Insulin Resistance Atherosclerosis Family Study. Diabetes. (2008) 57:1093–100. doi: 10.2337/db07-1169
9. Rodriguez S, Eiriksdottir G, Gaunt TR, Harris TB, Launer LJ, Gudnason V, et al. IGF2BP1, IGF2BP2 and IGF2BP3 genotype, haplotype and genetic model studies in metabolic syndrome traits and diabetes. Growth Hormone IGF Res. (2010) 20:310–8. doi: 10.1016/j.ghir.2010.04.002
10. Rao P, Wang H, Fang H, Gao Q, Zhang J, Song M, et al. Association between IGF2BP2 polymorphisms and type 2 diabetes mellitus: A case-control study and meta-analysis. Int J Environ Res Public Health. (2016) 13:574. doi: 10.3390/ijerph13060574
11. Shabana, Ullah Shahid S, Wah Li K, Acharya J, Cooper JA, Hasnain S, et al. Effect of six type II diabetes susceptibility loci and an FTO variant on obesity in Pakistani subjects. Eur J Hum Genetics: EJHG. (2016) 24:903–10. doi: 10.1038/ejhg.2015.212
12. Sladek R, Rocheleau G, Rung J, Dina C, Shen L, Serre D, et al. A genome-wide association study identifies novel risk loci for type 2 diabetes. Nature. (2007) 445:881–5. doi: 10.1038/nature05616
13. Uma Jyothi K, Reddy BM. Gene-gene and gene-environment interactions in the etiology of type 2 diabetes mellitus in the population of Hyderabad, India. Meta Gene. (2015) 5:9–20. doi: 10.1016/j.mgene.2015.05.001
14. Verma AK, Goyal Y, Bhatt D, Beg MMA, Dev K, Alsahli MA, et al. Association between CDKAL1, HHEX, CDKN2A/2B and IGF2BP2 gene polymorphisms and susceptibility to type 2 diabetes in Uttarakhand, India. Diabetes Metab Syndrome Obesity: Targets Ther. (2021) 14:23–36. doi: 10.2147/DMSO.S284998
15. Lee YH, Kang ES, Kim SH, Han SJ, Kim CH, Kim HJ, et al. Association between polymorphisms in SLC30A8, HHEX, CDKN2A/B, IGF2BP2, FTO, WFS1, CDKAL1, KCNQ1 and type 2 diabetes in the Korean population. J Hum Genet. (2008) 53:991–8. doi: 10.1007/s10038-008-0341-8
16. Wu HH, Liu NJ, Yang Z, Tao XM, Du YP, Wang XC, et al. IGF2BP2 and obesity interaction analysis for type 2 diabetes mellitus in Chinese Han population. Eur J Med Res. (2014) 19:40. doi: 10.1186/2047-783X-19-40
17. Christiansen J, Kolte AM, Hansen TV, Nielsen FC. IGF2 mRNA-binding protein 2: biological function and putative role in type 2 diabetes. J Mol Endocrinol. (2009) 43:187–95. doi: 10.1677/JME-09-0016
18. Sevgi M, Rigoux L, Kühn AB, Mauer J, Schilbach L, Hess ME, et al. An obesity-predisposing variant of the FTO gene regulates D2R-dependent reward learning. J Neurosci. (2015) 35:12584–92. doi: 10.1523/JNEUROSCI.1589-15.2015
19. Xiao S, Zeng X, Quan L, Zhu J. Correlation between polymorphism of FTO gene and type 2 diabetes mellitus in Uygur people from northwest China. Int J Clin Exp Med. (2015) 8:9744–50.
20. Elouej S, Belfki-Benali H, Nagara M, Lasram K, Attaoua R, Sallem OK, et al. Association of rs9939609 polymorphism with metabolic parameters and FTO risk haplotype among Tunisian metabolic syndrome. Metab Syndrome Related Disord. (2016) 14:121–8. doi: 10.1089/met.2015.0090
21. Lauenborg J, Grarup N, Damm P, Borch-Johnsen K, Jørgensen T, Pedersen O, et al. Common type 2 diabetes risk gene variants associate with gestational diabetes. J Clin Endocrinol Metab. (2009) 94:145–50. doi: 10.1210/jc.2008-1336
22. Cho YM, Kim TH, Lim S, Choi SH, Shin HD, Lee HK, et al. Type 2 diabetes-associated genetic variants discovered in the recent genome-wide association studies are related to gestational diabetes mellitus in the Korean population. Diabetologia. (2009) 52:253–61. doi: 10.1007/s00125-008-1196-4
23. Messeguer X, Escudero R, Farré D, Núñez O, Martínez J, Albà MM. PROMO: detection of known transcription regulatory elements using species-tailored searches. Bioinf (Oxford England). (2002) 18:333–4. doi: 10.1093/bioinformatics/18.2.333
24. Farré D, Roset R, Huerta M, Adsuara JE, Roselló L, Albà MM, et al. Identification of patterns in biological sequences at the ALGGEN server: PROMO and MALGEN. Nucleic Acids Res. (2003) 31:3651–3. doi: 10.1093/nar/gkg605
25. Ramírez-Bello J, Jiménez-Morales M. Implicaciones funcionales de los polimorfismos de un solo nucleótido (SNP) en genes codificantes de proteínas y no codificantes en enfermedades multifactoriales [Functional implications of single nucleotide polymorphisms (SNPs) in protein-coding and non-coding RNA genes in multifactorial diseases. Gaceta Med Mexico. (2017) 153:238–50.
26. Lin Z, Wang Y, Zhang B, Jin Z. Association of type 2 diabetes susceptible genes GCKR, SLC30A8, and FTO polymorphisms with gestational diabetes mellitus risk: a meta-analysis. Endocrine. (2018) 62:34–45. doi: 10.1007/s12020-018-1651-z
27. Xie W, Zhang L, Wang J, Wang Y. Association of HHEX and SLC30A8 gene polymorphisms with gestational diabetes mellitus susceptibility: A meta-analysis. Biochem Genet. (2023) 61:2203–21. doi: 10.1007/s10528-023-10385-x
28. Benny P, Ahn HJ, Burlingame J, Lee MJ, Miller C, Chen J, et al. Genetic risk factors associated with gestational diabetes in a multi-ethnic population. PloS One. (2021) 16:e0261137. doi: 10.1371/journal.pone.0261137
29. Klimentidis YC, Lemas DJ, Wiener HH, O’Brien DM, Havel PJ, Stanhope KL, et al. CDKAL1 and HHEX are associated with type 2 diabetes-related traits among Yup’ik people. J Diabetes. (2014) 6:251–9. doi: 10.1111/1753-0407.12093
30. Li C, Shen K, Yang M, Yang Y, Tao W, He S, et al. Association between single nucleotide polymorphisms in CDKAL1 and HHEX and type 2 diabetes in Chinese population. Diabetes Metab Syndrome Obesity: Targets Ther. (2021) 13:5113–23. doi: 10.2147/DMSO.S288587
31. Alfaifi M. Contribution of genetic variant identified in HHEX gene in the overweight Saudi patients confirmed with type 2 diabetes mellitus. Saudi J Biol Sci. (2022) 29:804–8. doi: 10.1016/j.sjbs.2021.10.028
32. Votsi C, Toufexis C, Michailidou K, Antoniades A, Skordis N, Karaolis M, et al. Type 2 diabetes susceptibility in the Greek-Cypriot population: replication of associations with TCF7L2, FTO, HHEX, SLC30A8 and IGF2BP2 polymorphisms. Genes. (2017) 8:16. doi: 10.3390/genes8010016
33. Hindy G, Mollet IG, Rukh G, Ericson U, Orho-Melander M. Several type 2 diabetes-associated variants in genes annotated to WNT signaling interact with dietary fiber in relation to incidence of type 2 diabetes. Genes Nutr. (2016) 11:6. doi: 10.1186/s12263-016-0524-4
34. Galavi H, Mollashahee-Kohkan F, Saravani R, Sargazi S, Noorzehi N, Shahraki H. HHEX gene polymorphisms and type 2 diabetes mellitus: A case-control report from Iran. J Cell Biochem. (2019) 120:16445–51. doi: 10.1002/jcb.28788
35. Aka TD, Saha U, Shati SA, Aziz MA, Begum M, Hussain MS, et al. Risk of type 2 diabetes mellitus and cardiovascular complications in KCNJ11, HHEX and SLC30A8 genetic polymorphisms carriers: A case-control study. Heliyon. (2021) 7:e08376. doi: 10.1016/j.heliyon.2021.e08376
36. Ortega-Contreras B, Armella A, Appel J, Mennickent D, Araya J, González M, et al. Pathophysiological role of genetic factors associated with gestational diabetes mellitus. Front Physiol. (2022) 13:769924. doi: 10.3389/fphys.2022.769924
37. Zhang J, McKenna LB, Bogue CW, Kaestner KH. The diabetes gene Hhex maintains δ-cell differentiation and islet function. Genes Dev. (2014) 28:829–34. doi: 10.1101/gad.235499.113
38. Cao J, Mu Q, Huang H. The roles of insulin-like growth factor 2 mRNA-binding protein 2 in cancer and cancer stem cells. Stem Cells Int. (2018) 2018:4217259. doi: 10.1155/2018/4217259
39. Dai N, Zhao L, Wrighting D, Krämer D, Majithia A, Wang Y, et al. IGF2BP2/IMP2-Deficient mice resist obesity through enhanced translation of Ucp1 mRNA and Other mRNAs encoding mitochondrial proteins. Cell Metab. (2015) 21:609–21. doi: 10.1016/j.cmet.2015.03.006
40. Xu CX, Zhu HH, Zhu YM. Diabetes and cancer: Associations, mechanisms, and implications for medical practice. World J Diabetes. (2014) 5:372–80. doi: 10.4239/wjd.v5.i3.372
41. Vigneri R, Goldfine ID, Frittitta L. Insulin, insulin receptors, and cancer. J Endocrinological Invest. (2016) 39:1365–76. doi: 10.1007/s40618-016-0508-7
42. Grarup N, Rose CS, Andersson EA, Andersen G, Nielsen AL, Albrechtsen A, et al. Studies of association of variants near the HHEX, CDKN2A/B, and IGF2BP2 genes with type 2 diabetes and impaired insulin release in 10,705 Danish subjects: validation and extension of genome-wide association studies. Diabetes. (2007) 56:3105–11. doi: 10.2337/db07-0856
43. Groenewoud MJ, Dekker JM, Fritsche A, Reiling E, Nijpels G, Heine RJ, et al. Variants of CDKAL1 and IGF2BP2 affect first-phase insulin secretion during hyperglycaemic clamps. Diabetologia. (2008) 51:1659–63. doi: 10.1007/s00125-008-1083-z
44. Li X, Allayee H, Xiang AH, Trigo E, Hartiala J, Lawrence JM, et al. Variation in IGF2BP2 interacts with adiposity to alter insulin sensitivity in Mexican Americans. Obes (Silver Spring Md.). (2009) 17:729–36. doi: 10.1038/oby.2008.593
45. Chistiakov DA, Nikitin AG, Smetanina SA, Bel’chikova LN, Suplotova LA, Shestakova MV, et al. The rs11705701 G>A polymorphism of IGF2BP2 is associated with IGF2BP2 mRNA and protein levels in the visceral adipose tissue - a link to type 2 diabetes susceptibility. Rev Diabetic Studies: RDS. (2012) 9:112–22. doi: 10.1900/RDS.2012.9.112
46. Omori S, Tanaka Y, Takahashi A, Hirose H, Kashiwagi A, Kaku K, et al. Association of CDKAL1, IGF2BP2, CDKN2A/B, HHEX, SLC30A8, and KCNJ11 with susceptibility to type 2 diabetes in a Japanese population. Diabetes. (2008) 57:791–5. doi: 10.2337/db07-0979
47. Almgren P, Lehtovirta M, Isomaa B, Sarelin L, Taskinen MR, Lyssenko V, et al. Heritability and familiality of type 2 diabetes and related quantitative traits in the Botnia Study. Diabetologia. (2011) 54:2811–9. doi: 10.1007/s00125-011-2267-5
48. Liu J, Song G, Zhao G, Meng T. Lack of association between IGF2BP2 rs4402960 polymorphism and gestational diabetes mellitus: a case-control study, meta-analysis and trial sequential analysis. Biosci Rep. (2020) 40:BSR20200990. doi: 10.1042/BSR20200990
49. Popova PV, Klyushina AA, Vasilyeva LB, Tkachuk AS, Bolotko YA, Gerasimov AS, et al. Effect of gene-lifestyle interaction on gestational diabetes risk. Oncotarget. (2017) 8:112024–35. doi: 10.18632/oncotarget.22999
50. Popova PV, Klyushina AA, Vasilyeva LB, Tkachuk AS, Vasukova EA, Anopova AD, et al. Association of common genetic risk variants with gestational diabetes mellitus and their role in GDM prediction. Front Endocrinol. (2021) 12:628582. doi: 10.3389/fendo.2021.628582
51. Bartosovic M, Molares HC, Gregorova P, Hrossova D, Kudla G, Vanacova S. N6-methyladenosine demethylase FTO targets pre-mRNAs and regulates alternative splicing and 3’-end processing. Nucleic Acids Res. (2017) 45:11356–70. doi: 10.1093/nar/gkx778
52. Frayling TM, Timpson NJ, Weedon MN, Zeggini E, Freathy RM, Lindgren CM, et al. A common variant in the FTO gene is associated with body mass index and predisposes to childhood and adult obesity. Sci (New York N.Y.). (2007) 316:889–94. doi: 10.1126/science.1141634
Keywords: gestational diabetes mellitus, rs1111875, rs5015480, rs11705701, rs4402960, rs9939609, case-control study
Citation: Zeng Q, Liu J, Liu X, Liu N, Wu W, Watson RG, Zou D, Wei Y and Guo R (2024) Association between genetic polymorphisms and gestational diabetes mellitus susceptibility in a Chinese population. Front. Endocrinol. 15:1397423. doi: 10.3389/fendo.2024.1397423
Received: 07 March 2024; Accepted: 13 November 2024;
Published: 26 November 2024.
Edited by:
Ana Maria Ramos-Levi, Princess University Hospital, SpainReviewed by:
Polina Popova, Almazov National Medical Research Centre, RussiaCopyright © 2024 Zeng, Liu, Liu, Liu, Wu, Watson, Zou, Wei and Guo. This is an open-access article distributed under the terms of the Creative Commons Attribution License (CC BY). The use, distribution or reproduction in other forums is permitted, provided the original author(s) and the copyright owner(s) are credited and that the original publication in this journal is cited, in accordance with accepted academic practice. No use, distribution or reproduction is permitted which does not comply with these terms.
*Correspondence: Yue Wei, d2VpeXVlMTM4QDE2My5jb20=; Runmin Guo, cnVubWluLmd1b0BnZG11LmVkdS5jbg==
Disclaimer: All claims expressed in this article are solely those of the authors and do not necessarily represent those of their affiliated organizations, or those of the publisher, the editors and the reviewers. Any product that may be evaluated in this article or claim that may be made by its manufacturer is not guaranteed or endorsed by the publisher.
Research integrity at Frontiers
Learn more about the work of our research integrity team to safeguard the quality of each article we publish.