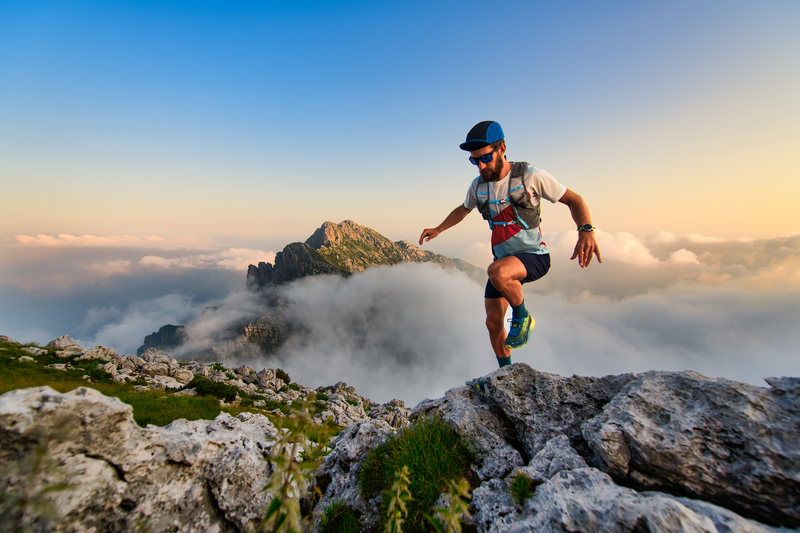
94% of researchers rate our articles as excellent or good
Learn more about the work of our research integrity team to safeguard the quality of each article we publish.
Find out more
ORIGINAL RESEARCH article
Front. Endocrinol. , 16 September 2024
Sec. Endocrinology of Aging
Volume 15 - 2024 | https://doi.org/10.3389/fendo.2024.1394252
This article is part of the Research Topic Telomeres and Epigenetics in Endocrinology: Volume II View all 4 articles
Background: Stress urinary incontinence (SUI) is a common condition characterized by urethral sphincter failure and urine leakage. Its prevalence in women is higher than in men, and estimates of crude prevalence rates vary widely due to factors such as research methodologies, study populations, and underreporting by patients. This variability hinders research and impacts patient diagnosis, treatment, and quality of life. The complex etiology of SUI is not fully understood, and previous studies have primarily focused on non-invasive indicators. While emerging observational research suggests a correlation between SUI in women and abnormalities in lipid and blood metabolism, the underlying biological mechanisms and causal relationships require further investigation. This study aims to explore the causalities between SUI in women and lipid and blood metabolism.
Methods: Using bidirectional univariate Mendelian randomization (MR), we investigated the causal association between SUI liability in women (case/control = 5,924/399,509) from UK Biobank and lipid and glucose metabolism, indicated by total cholesterol (TC, N = 61,166), low-density lipoproteins (LDL, N = 58,381), high-density lipoproteins (HDL, N = 60,812), triglycerides (TG, N = 60,027), fasting glucose (FG, N = 19,745), and fasting insulin (FI, N = 38,238) from ENGAGE consortium. To account for potential confounding effects, multivariable MR (MVMR) analyses were performed, adjusting for body mass index (BMI) and separately among lipid and glucose metabolism.
Results: We found that increased genetically proxied TC, LDL, and HDL levels were associated with an elevated risk of SUI in women (OR: 1.090–1.117, all P < 0.05), These associations were further supported by MVMR analyses with adjustment for BMI (OR: 1.087–1.114, all P < 0.05). Conversely, increased FG and FI were associated with reduced SUI reliability in women (OR: 0.731–0.815, all P < 0.05). When adjusting among lipid and glucose metabolism, only HDL and FI demonstrated causal effects. Reverse MR analyses provided no genetic evidence supporting the causal effect of SUI in women on lipid and blood metabolism (all P > 0.05).
Conclusions: Our results reported that increased TC, LDL, and HDL are linked to higher SUI susceptibility in women, while higher FG and FI levels have a protective effect. In overweight/obese women with metabolic abnormalities, the positive associations between TC, LDL, and HDL levels and SUI indicate a higher risk.
Urinary incontinence (UI) is characterized by the frequent urge to urinate or involuntary leakage of urine. Stress urinary incontinence (SUI) is a common form of urinary incontinence characterized by the failure of the urethral sphincter to close completely, resulting in urine leakage (1). Due to factors such as pregnancy, childbirth, and hormonal changes during menopause, the incidence of SUI in women is higher than in men (2). It was reported that SUI occurs at least weekly in one-third of adult women (3). Estimates of its crude prevalence rate in the female population range from 10% to 40%, which have reported significant variations (4, 5). On the one hand, it attributed to differences in research methodologies, study populations, and definitions of UI (6). On the other hand, given the sensitive and potential nature of UI, patients may tend to underreport their symptoms, resulting in an underestimated prevalence and a reluctance to seek medical assistance (7). This wide variability not only hinders relevant research but also has significant implications for the diagnosis, treatment, management, health status, and overall quality of life of patients.
The etiology and pathogenesis of SUI are complex and not yet completely clear. Prior research on the etiology or risk factors of SUI have primarily focused on indicators that are available by easy screening methods, such as BMI, weight, pregnancy/childbirth history, and history of uterine/bladder disease treatment (8–10). Notably, a growing number of researchers are finding that SUI frequently accompanies abnormalities in blood lipid and glucose-related biomarkers. Among women with obesity, hyperlipidemia was associated with a higher risk of SUI, and there was a significant synergistic effect of hyperlipidemia and obesity on SUI (11). Furthermore, a cross-sectional study showed that the levels of blood glucose were positively correlated with SUI (12). However, the precise biological mechanism underlying this reciprocal association and the establishment of causal inference remain inadequately understood due to the effect of confounding factors and the absence of randomized controlled trial (RCT) studies.
Mendelian randomization (MR) is an epidemiological technique to estimate the causal associations, utilizing genetic variants as instrumental variables which are not expected to be subject to biases from confounding and reverse causation seen in other types of observational epidemiology (13, 14). To investigate a clearer causal effect of lipid and glucose metabolism on SUI in women, we performed bidirectional two-sample MR analysis and multivariable MR (MVMR) analysis using 405,433 European ancestry participants. Furthermore, we also adjusted the confounding effect of BMI in the causal inference of lipid and glucose metabolism on SUI in women using MVMR.
We utilized bidirectional two-sample Mendelian randomization (MR) (15) to assess the causal association between lipid and glucose metabolism and SUI in female patients. We ensured that the study sample is derived from a relatively genetically homogenous population (European ancestry). Figure 1 shows an overview of the study design.
Figure 1. Flow chart. TC, total cholesterol; LDL, low-density lipoproteins; HDL, high-density lipoproteins; TG, triglycerides; FG, fasting glucose; FI, fasting insulin; MR, Mendelian randomization; IVW, inverse-variance weighted method; ConMix, contamination mixture; RAPS, robust adjusted profile score; MBE, mode-based estimate; PRESSO, pleiotropy residual sum and outlier; LCV, latent causal variable.
Regarding lipid and glucose metabolism biomarkers, all the summary statistical data were continuous biomarkers from the European Network for Genetic and Genomic Epidemiology (ENGAGE) consortium (16, 17), including total cholesterol (TC, N = 61,166), low-density lipoproteins (LDL, N = 58,381), high-density lipoproteins (HDL, N = 60,812), triglycerides (TG, N = 60,027), fasting glucose (FG, N = 19,745), and fasting insulin (FI, N = 38,238). According to summary statistics, SUI in women was found in a total of 405,433 individuals (case/control = 5,924/399,509), derived from UK Biobank/PheWeb (18). Moreover, genetic association estimates for BMI were also obtained from 346,393 European ancestry individuals in UK Biobank (19). All data of this study are publicly available GWAS summary statistics. Therefore, additional ethical approvals for the GWAS were not required.
For forward MR, instrumental variables were identified as genetic variants associated with the abovementioned six lipid and glucose metabolism biomarkers. To meet the first assumption of MR that the instrumental variables are strongly associated with exposures (lipid and glucose metabolism), conditionally independent SNPs at a level of genome-wide significance threshold (p < 5 × 10−8) were selected. For FI, only one SNP was selected with the threshold of p < 5 × 10−8, so we set the p-value to 5 × 10−5 to get adequate SNPs for analysis. All genetic variants were clumped using PLINK to ensure that a list of lipid and glucose metabolism SNPs was from an independent set of variants (parameters: –clump-p1 5 × 10−8 –clump-p2 1 × 10−5 –clump-r2 0.1 –clump-kb 1000). For reverse MR (testing the effect of genetic predictors of SUI in women on lipid and glucose metabolism), we applied the p-value threshold of p < 5 × 10−6 to SUI in women. The independent instruments were determined by clumping (parameters: –clump-p1 5 × 10−6 –clump-p2 1 × 10−5 –clump-r2 0.1 –clump-kb 1000).
For the third assumption, there should be no SNP associated with unobserved confounding variable and outcomes (20). We performed a sensitivity analysis with a p-value threshold of p < 5 × 10−10 in lipid and glucose metabolism SNPs (set p < 5 × 10−6 for FI). In addition, we set r2 to 0.001, a more stringent threshold, to reduce the risk of horizontal pleiotropy. For the reverse analysis, to include adequate SNPs for the analysis, we applied a more relaxed p-value threshold of p < 5 × 10−5.
In order to provide unbiased estimates for Mendelian randomization, three key assumptions should be met: (1) genetic instrumental variants should be significantly associated with the exposure (i.e., lipid and glucose metabolism), (2) the genetic instrumental variants affect the outcome (i.e., SUI in women) only through the effect of exposure (i.e., lipid and glucose metabolism) indirectly and not through other biological pathways (14), and (3) genetic instrumental variants should be independent of any confounders. This study adhered to the Strengthening the Reporting of Observational Studies in Epidemiology—Mendelian Randomization guidelines (STROBE—MR) (21).
To investigate the potential causal relationship between lipid and glucose metabolism and SUI in women, we applied several MR methods. First, inverse-variance weighted (IVW) was performed. It is a common MR approach, which assumed that all instruments were valid and could be liable to be biased with any instrument exhibiting horizontal pleiotropy (22). Thus, for the second assumption, the primary analysis method in this study was the mode-based estimate (MBE) method, which allows the majority of instruments to be pleiotropic (23). To minimize the effect of horizontal pleiotropy, weighted median analysis (24) and MR-Egger regression (25) were also performed. Contamination mixture (ConMix) (24) and robust adjusted profile score (RAPS) (26) were performed to detect the causal effect of invalid instruments’ bias, pleiotropy, and extreme outliers. In addition, we used the pleiotropy residual sum and outlier (PRESSO) method to detect horizontal pleiotropy and correct for horizontal pleiotropy via outlier removal (27).
We introduce a latent causal variable (LCV) model, which is robust to sample overlap. Under this model, the genetic correlation between two traits is mediated by a latent variable having a causal effect on each trait (28). Moreover, MVMR is an MR extension analogue to estimate the effect of two or more correlated exposure variables (29). Thus, we applied MVMR based on the IVW methods separately for lipid and glucose metabolism biomarkers, which are closely related risk factors on the same molecular level. As BMI was reported to be a factor that affects the metabolic levels of lipid and glucose, we additionally applied MVMR based on the IVW methods to adjust BMI-associated variants.
All analyses were performed with R software 4.2.2. ConMix, IVW, weighted median, MBE, MR–Egger methods, and multivariable MR were performed using the “MendelianRandomization” package. The RAPS model was performed using the “mr. raps” package. The MR-PRESSO approach was performed using the “MR-PRESSO” package. We used the mRnd tool (https://shiny.cnsgenomics.com/mRnd/) to calculate the F-statistics to assess the strength of the instruments (30). F-statistic greater than 10 was considered a strong instrument (31).
This MR study was based on publicly available GWAS data, obviating the need for ethical approvals.
In the MR analysis of lipid and glucose metabolism on SUI in women, the total number of genetic instruments retained in terms of genome-wide threshold (p < 5 × 10−8, r2 < 0.1) from the ENGAGE consortium was 151, 149, 133, 93, and 28 SNPs for TC, LDL, HDL, TG, and FG, respectively. Setting the p-value to 5 × 10−5, we obtained 152 instrumental SNPs for FI. F-statistics across SNPs ranged from 997 to 7,223 among lipid and glucose metabolism biomarkers, which are displayed in Supplementary Table S1.
For lipid metabolism biomarkers, IVW, MBE, ConMix, RAPS, and weighted median analyses all identified significant positive associations between TC/LDL/HDL and SUI in women. MR-Egger regression also suggested the positive effect of TC and LDL with no significant intercept (all OR >1, P < 0.05). There is no evidence of causal relationship between TG and SUI in women (all P > 0.05). For glucose metabolism biomarkers, a significant negative causal effect of FG on the risk of SUI was identified in female patients (IVW OR = 0.815, 95%CI: 0.676–0.981, P = 0.032; the results of MR-Egger, ConMix, and RAPS are aligned), while we found no significant causal relationship between FI and SUI in women when using the threshold of p < 5 × 10−5, r2 < 0.1 to select SNPs of FI (all P > 0.05) (Figure 2 and Supplementary Table S2).
Figure 2. Forest plots of main forward and reverse Mendelian randomization analyses investigating the causal relationships between both lipid and glucose metabolism biomarkers and SUI in women. The p-value threshold of forward Mendelian randomization for fasting insulin is 5E-5. Asterisk denotes that the intercepts of MR–Egger were significant (p < 0.05). IVW, inverse-variance weighted method; ConMix, contamination mixture; RAPS, robust adjusted profile score; MBE, mode-based estimate.
As we adjusted the genome-wide threshold of p < 5 × 10−10 and r2 < 0.1, a total of 99, 112, 87, 56, and 15 instrumental SNPs was selected for TC, LDL, HDL, TG, and FG, respectively. For lipid metabolism biomarkers, we also found some similar causal effect of TC, LDL, and HDL on SUI in women (all OR >1, P < 0.05). Furthermore, the ConMix method found an additional causal role of genetically decreased risk of SUI in female patients with elevated TG (OR = 0.798, P = 0.01). For glucose metabolism biomarkers, in addition to the negative causal association between FG and SUI in women (IVW OR = 0.7, 95%CI: 0.538–0.9112, P = 0.008; RAPS: OR = 0.699, 95%CI: 0.5358–0.9129, P = 0.009), we also found a negative effect of FI on SUI in women with 24 instrumental SNPs selected by the threshold of p < 5 × 10−6 and r2 < 0.1 (ConMix OR = 0.460, 95%CI: 0.248–0.909, P = 0.030; the results of IVW and RAPS are aligned) (Supplementary Table S2).
After setting the R2 as 0.001, we obtained 59 SNPs for TC, 53 SNPs for LDL, 56 SNPs for HDL, 36 SNPs for TG, 17 SNPs for FG (genome-wide threshold p < 5 × 10−8, r2 < 0.001), and 143 SNPs for FI (genome-wide threshold p < 5 × 10−5, r2 < 0.001). Genetically predicted higher TC/HDL was associated with an increased risk of SUI in women. With the more stringent threshold (TC, LDL, HDL, TG, and FG: p < 5 × 10−10/FI: p < 5 × 10−6, r2 < 0.001), we found that a positive causal effect of genetically predicted TC (45 SNPs)/LDL (41 SNPs)/HDL (33 SNPs) (all OR >1, P < 0.05) and a negative causal effect of FG (25 SNPs)/FI (24 SNPs) (all OR <1, P < 0.05) were also significant. For significant TG-related instrumental SNPs, we did not find evidence of a causal effect on SUI in women under any genome-wide thresholds (p < 5 × 10−8/p < 5 × 10−10, r2 < 0.001) (Supplementary Table S3).
The MR-PRESSO process had verified all the causal associations mentioned above (Supplementary Table S4).
We obtain 21 SNPs for SUI in women without effects of linkage disequilibrium (r2 < 0.1), which reached the primary genome-wide significant level (p < 5 × 10−6). To retain more instrumental SNPs and avoid over-inflated type I errors in these reverse MR analyses, we also performed analysis by setting genome-wide significance of p < 5 × 10−5. Non-significant causal association was found in all analyses (all P > 0.05) (Figure 2 and Supplementary Table S5). Furthermore, MR-PRESSO analyses also did not find any significant associations (Supplementary Table S6).
The LCV method was employed to investigate if the causal effect of lipid and glucose metabolism on SUI in women could be fully explained by shared underlying genetic etiology. Our finding identified evidence for genetic positive correlations between TC, LDL, and HDL and SUI (Rho = 0.11–0.22, all P < 0.05) with partial causal effect (GCP = 0.68–0.77). For glucose metabolism biomarkers, we only obtained one negative genetic effect of FI through LCV analysis [Rho = -0.12 (0.17), GCP = 0.77 (0.08), P <0.001] (Table 1).
Table 1. Results of LCV modeling for the genetic relationships between both lipid and glucose metabolism biomarkers and SUI in women.
We performed MVMR separately for lipid and glucose metabolism biomarkers to disentangle the causal effect on SUI in women. Only HDL retained a positive causal relationship with SUI in women while controlling TC, LDL, and TG in the model (BETA = 0.232, 95%CI: 0.054–0.411, P = 0.011). When the independent causal effects of FG and FI were evaluated together in one MVMR, only FI showed a negatively causal effect on SUI in women (BETA = -0.383, 95%CI: -0.678–0.089, P = 0.011) (Table 2).
Table 2. Causal relationships of lipid and glucose metabolism biomarkers on SUI in women estimated by multivariable MR, adjusting for BMI and mutual adjustments among lipid biomarkers and among glucose biomarkers.
As BMI could affect lipid and glucose metabolism, as well as SUI in women, we established MVMR models to account for the influence of BMI-associated variants. Regarding lipid metabolism biomarkers, the positive associations for genetically predicted levels of TC/LDL/HDL remained significant in multivariable MR analyses with adjustment for genetically predicted levels of BMI (TC BETA = 0.097, 95%CI: 0.021–0.174, P = 0.013; LDL BETA = 0.083, 95%CI: 0.018–0.148, P = 0.012; HDL BETA = 0.108, 95%CI: 0.037–0.180, P = 0.003). However, in contrast to univariable MR analyses, multivariable MR presented no corresponding associations between both FG and FI and SUI in women after adjustment for BMI (FG BETA = -0.155, 95%CI: -0.418–0.107, P = -0.245; FI BETA = -0.088, 95%CI: -0.323–0.147, P = 0.464) (Table 2).
In this study, we applied bidirectional two-sample MR method to explore the causal association of lipid (TC, LDL, HDL, and TG) and glucose (FG and T2D) metabolism traits with SUI in women based on summary-level data from large-scale datasets. The primary analyses revealed that genetically predicted SUI in women was associated with a wide range of lipid and glucose metabolism biomarkers. Specifically, we observed significant positive associations between the levels of TC, LDL, and HDL and the risk of SUI in women. Furthermore, we observed a protective causal effect of FG and FI levels on SUI liability in women. Reverse MR analyses provided genetic evidence supporting a deleterious causal effect of SUI in women on increased levels of TG. Additionally, after adjusting for BMI, the causal effect of TC, LDL, and HDL on SUI in women was supported in the multivariable MR analysis, while separate MVMR analyses among lipid and glucose metabolism biomarkers only demonstrated significant effects of HDL and FI.
Standard lipid blood tests consist of four basic parameters: TC, LDL, HDL, and TG. In our study, we found that the higher levels of TC, LDL, and HDL had a genetic causal effect on SUI liability in women, while there was no significant relationship between TG and SUI in women, which was also supported by LCV analysis and MVMR analysis adjusting BMI. However, this finding differed from the conclusions of previous observational studies. A study had shown that cholesterol and triglycerides have no significant effect on the risk of urinary incontinence in women with diabetes mellitus (32), while another had reported that TG was higher in older women with SUI than in age- and sex-matched control groups without clinical SUI (33). We believe that these differences may originate from the lack of adjustment for certain covariates. Therefore, we conducted MVMR analysis, mutually adjusting among lipid biomarkers that are closely related risk factors at the same molecular level. After adjustment, only HDL retained a positive causal correlation with SUI.
Generally, it is believed that high levels of LDL, which are associated with an increased risk of multisystem disorders, can cause cholesterol to stick to the walls of blood vessels and contribute to plaque formation (34). In contrast, HDL carries cholesterol from the blood vessels to the liver for absorption and can counter the effects of LDL (35). Interestingly, in our analyses, elevated LDL and HDL levels were both associated with SUI liability in women. On the one hand, as we have discussed above, we believed that some potentially complex confounding factors in the causal relationship between LDL and SUI are important reasons for this unusual result (36). On the other hand, extreme high HDL concentrations may paradoxically have deleterious effects on health status (37)—for instance, certain genetic variants associated with extreme high HDL cholesterol concentrations have been paradoxically related with the risk of cardiovascular disease (38, 39). Further targeted investigations and clinical trials are warranted to explore the different effects on SUI in women across a range of HDL concentrations as well as to identify the specific pathways and molecular targets that mediate the relationship between different HDL levels and SUI, which could inform additional therapeutic development.
Some literatures have discussed the physiological mechanisms by which lipid metabolism affects pelvic floor structure and function. A study on animals demonstrated that prolonged exposure to the dyslipidemic environment triggers epigenetic changes, resulting in aberrant global transcriptional signatures of genes and microRNAs (40, 41). This process impairs the reparative capacity of muscle-derived stem cells, consequently impacting the recovery of pelvic floor function, leading to SUI in women (41, 42). Mohamed Kamal Mesregah et al. found that the rate of incontinence was higher in patients with chronic hyperlipidemia with significantly increased nerve root injury (43). Therefore, we thought that lipid metabolism biomarkers could play an important role in the identification of pelvic floor nerve injury. It suggested that clinicians could include these lipid biomarkers in examination when assessing the risk of SUI in women. Additionally, for women with a family history of SUI (44), clinicians should monitor their lipid levels more frequently and intervene in cases of abnormalities to prevent the occurrence of SUI earlier.
Regarding glucose metabolism, there were some evidence supporting a causal effect of a lower level of FG on high risk of SUI in women, which was opposite to the conclusion that Jeanette S. Brown et al. reported. In their investigation of 1,461 nonpregnant adult women, they found that the prevalence of incontinence was significantly higher in women with high glucose levels than those with normal FG (45). Moreover, we observed a significantly low level of FI in SUI female patients with a more relaxed p-value threshold (p < 5 × 10−6), which was supported by our LCV analysis and MVMR analysis adjusting for FG. As BMI or obesity, often accompanied by hyperglycemia and insulin resistance (46, 47), is widely recognized as a significant risk factor for SUI in women, we also conducted MVMR analysis adjusting BMI for glucose biomarkers. However, the results revealed no significant association between SUI in women and FG and FI levels. This underscores the limitations of using glucose-related biomarkers as independent predictors of SUI (51) despite high glucose being considered a risk factor for UI. Considering BMI as a potential modifiable risk factor, amenable to lifestyle modifications, underscores the importance of integrating clinical and lifestyle interventions in the future management of SUI in women (48). By employing comprehensive interventions, such as combining dietary control, increased physical activity, and medication therapy, along with improving patients’ weight and blood glucose levels, the risk of SUI may be more effectively reduced.
This present study was the first to demonstrate the causal associations between lipid and glucose metabolism and SUI in women using MR analysis based on largest GWAS data. The various MR methods that we utilized guaranteed the reliability of results by eliminating residual confounding as much as possible. Meanwhile, given the effect of BMI and mutual interaction of metabolism traits, we applied MVMR to adjust these exposures. In addition, the F-statistics of the genetic variants were all more than 10 with a large sample size, which indicated that the genetic variants were strong enough to be IVs for exposure.
However, there were some limitations that need to be acknowledged. Firstly, we did not differentiate between the levels of lipid and glucose metabolism biomarkers in our analyses. Despite the fact that the potential impact on our results is unclear, we assumed the paradoxically causal direction of extreme high HDL and proposed a new and significant research aspect for SUI in women. Secondly, our study population was limited to individuals of European ancestry, and thus caution should be taken when generalizing the results to populations of other ancestries (49).
In conclusion, our MR estimates supported an adverse effect of increased TC/LDL/HDL and a protective effect of increased FG and FI on SUI susceptibility in women. The positive associations between TC/LDL/HDL and SUI in women were also verified through MVMR models with adjusted BMI, which revealed the risk of SUI in obese/overweight women with metabolic abnormalities. The potential mechanism of the causal relationship between lipid and glucose metabolism and SUI warrants further investigations.
The original contributions presented in the study are included in the article/Supplementary Material. Further inquiries can be directed to the corresponding authors.
NX: Visualization, Validation, Software, Methodology, Formal Analysis, Writing – original draft. SS: Writing – review & editing, Writing – original draft, Methodology, Formal Analysis. YY: Writing – review & editing, Software, Methodology, Data curation. YRL: Writing – original draft, Visualization, Validation, Software. TF: Writing – original draft, Validation, Software. LW: Writing – original draft, Validation. YFL: Writing – review & editing, Resources, Methodology, Data curation, Conceptualization. JH: Writing – review & editing, Writing – original draft.
The author(s) declare financial support was received for the research, authorship, and/or publication of this article. This study was supported by the National Natural Science Foundation of China (Grant No. 32101206), Key Program of Science and Technology Department of Sichuan Province (Grant Nos. 2023YFS0025 and 2023YFS0102); 1·3·5 project for disciplines of excellence–Clinical Research Fund, West China Hospital, Sichuan University (2023HXFH044).
We would like to thank ENGAGE consortium, UK Biobank, and PheWeb.
The authors declare that the research was conducted in the absence of any commercial or financial relationships that could be construed as a potential conflict of interest.
All claims expressed in this article are solely those of the authors and do not necessarily represent those of their affiliated organizations, or those of the publisher, the editors and the reviewers. Any product that may be evaluated in this article, or claim that may be made by its manufacturer, is not guaranteed or endorsed by the publisher.
The Supplementary Material for this article can be found online at: https://www.frontiersin.org/articles/10.3389/fendo.2024.1394252/full#supplementary-material
1. Gaspari A. Pelvic Floor Disorders: Surgical Approach. Berlin/Heidelberg, Germany: Springer, (2014).
2. Wu JM. Stress incontinence in women. New Engl J Med. (2021) 384:2428–36. doi: 10.1056/NEJMcp1914037
3. Nygaard IE, Heit M. Stress urinary incontinence. Obstetrics gynecology. (2004) 104:607–20. doi: 10.1097/01.AOG.0000137874.84862.94
4. Capobianco G, Madonia M, Morelli S, Dessole F, De Vita D, Cherchi PL, et al. Management of female stress urinary incontinence: A care pathway and update. Maturitas. (2018) 109:32–8. doi: 10.1016/j.maturitas.2017.12.008
5. Cerruto MA, D’Elia C, Aloisi A, Fabrello M, Artibani W. Prevalence, incidence and obstetric factors’ impact on female urinary incontinence in Europe: a systematic review. Urologia internationalis. (2013) 90:1–9. doi: 10.1159/000339929
6. Schreiber Pedersen L, Lose G, Høybye MT, Elsner S, Waldmann A, Rudnicki M. Prevalence of urinary incontinence among women and analysis of potential risk factors in Germany and Denmark. Acta Obstetricia Gynecologica Scandinavica. (2017) 96:939–48. doi: 10.1111/aogs.13149
7. Bardsley A. An overview of urinary incontinence. Br J Nurs. (2016) 25(18). doi: 10.12968/bjon.2016.25.18.S14
8. Rortveit G, Hannestad YS, Daltveit AK, Hunskaar S. Age- and type-dependent effects of parity on urinary incontinence: the Norwegian EPINCONT study. Obstetrics gynecology. (2001) 98:1004–10. doi: 10.1097/00006250-200112000-00004
9. Subak LL, Richter HE, Hunskaar S. Obesity and urinary incontinence: epidemiology and clinical research update. J urology. (2009) 182:S2–7. doi: 10.1016/j.juro.2009.08.071
10. Lukacz ES, Lawrence JM, Contreras R, Nager CW, Luber KM. Parity, mode of delivery, and pelvic floor disorders. Obstetrics gynecology. (2006) 107:1253–60. doi: 10.1097/01.AOG.0000218096.54169.34
11. Zhu F, Chen M, Xiao Y, Huang X, Chen L, Hong L. Synergistic interaction between hyperlipidemia and obesity as a risk factor for stress urinary incontinence in Americans. Sci Rep. (2024) 14:7312. doi: 10.1038/s41598-024-56744-5
12. Liu N, Xing L, Mao W, Chen S, Wu J, Xu B, et al. Relationship between blood glucose and hemoglobin A1c levels and urinary incontinence in women. Int J Gen Med. (2021) 14:4105–16. doi: 10.2147/IJGM.S324332
13. Davey Smith G, Hemani G. Mendelian randomization: genetic anchors for causal inference in epidemiological studies. Hum Mol Genet. (2014) 23:R89–98. doi: 10.1093/hmg/ddu328
14. Davies NM, Holmes MV, Davey Smith G. Reading Mendelian randomisation studies: a guide, glossary, and checklist for clinicians. BMJ (Clinical Res ed). (2018) 362:k601. doi: 10.1136/bmj.k601
15. Pierce BL, Burgess S. Efficient design for Mendelian randomization studies: subsample and 2-sample instrumental variable estimators. Am J Epidemiol. (2013) 178:1177–84. doi: 10.1093/aje/kwt084
16. Horikoshi M, Mägi R, van de Bunt M, Surakka I, Sarin AP, Mahajan A, et al. Discovery and fine-mapping of glycaemic and obesity-related trait loci using high-density imputation. PloS Genet. (2015) 11:e1005230. doi: 10.1371/journal.pgen.1005230
17. Surakka I, Horikoshi M, Mägi R, Sarin AP, Mahajan A, Lagou V, et al. The impact of low-frequency and rare variants on lipid levels. Nat Genet. (2015) 47:589–97. doi: 10.1038/ng.3300
18. PheWeb stress incontinence, female . Available online at: https://pheweb.org/UKB-SAIGE/pheno/624.9. [April 25, 2023].
19. Wang H, Zhang F, Zeng J, Wu Y, Kemper KE, Xue A, et al. Genotype-by-environment interactions inferred from genetic effects on phenotypic variability in the UK Biobank. Sci Adv. (2019) 5:eaaw3538. doi: 10.1126/sciadv.aaw3538
20. VanderWeele TJ, Tchetgen Tchetgen EJ, Cornelis M, Kraft P. Methodological challenges in mendelian randomization. Epidemiol (Cambridge Mass). (2014) 25:427–35. doi: 10.1097/EDE.0000000000000081
21. Skrivankova VW, Richmond RC, Woolf BAR, Yarmolinsky J, Davies NM, Swanson SA, et al. Strengthening the reporting of observational studies in epidemiology using mendelian randomization: the STROBE-MR statement. Jama. (2021) 326:1614–21. doi: 10.1001/jama.2021.18236
22. Mounier N, Kutalik Z. Bias correction for inverse variance weighting Mendelian randomization. Genet Epidemiol. (2023) 47:314–31. doi: 10.1002/gepi.22522
23. Hartwig FP, Davey Smith G, Bowden J. Robust inference in summary data Mendelian randomization via the zero modal pleiotropy assumption. Int J Epidemiol. (2017) 46:1985–98. doi: 10.1093/ije/dyx102
24. Burgess S, Foley CN, Allara E, Staley JR, Howson JMM. A robust and efficient method for Mendelian randomization with hundreds of genetic variants. Nat Commun. (2020) 11:376. doi: 10.1038/s41467-019-14156-4
25. Burgess S, Thompson SG. Interpreting findings from Mendelian randomization using the MR-Egger method. Eur J Epidemiol. (2017) 32:377–89. doi: 10.1007/s10654-017-0255-x
26. Qingyuan Z JW, Gibran H, Jack B, Dylan SS. Statistical inference in two-sample summary-data Mendelian randomization using robust adjusted profile score. Ann Stat. (2020) 48:1742–69. doi: 10.48550/arXiv.1801.09652
27. Verbanck M, Chen CY, Neale B, Do R. Detection of widespread horizontal pleiotropy in causal relationships inferred from Mendelian randomization between complex traits and diseases. Nat Genet. (2018) 50:693–8. doi: 10.1038/s41588-018-0099-7
28. O’Connor LJ, Price AL. Distinguishing genetic correlation from causation across 52 diseases and complex traits. Nat Genet. (2018) 50:1728–34. doi: 10.1038/s41588-018-0255-0
29. Sanderson E, Davey Smith G, Windmeijer F, Bowden J. An examination of multivariable Mendelian randomization in the single-sample and two-sample summary data settings. Int J Epidemiol. (2019) 48:713–27. doi: 10.1093/ije/dyy262
30. Sanderson E, Windmeijer F. A weak instrument [Formula: see text]-test in linear IV models with multiple endogenous variables. J econometrics. (2016) 190:212–21. doi: 10.1016/j.jeconom.2015.06.004
31. Burgess S. Sample size and power calculations in Mendelian randomization with a single instrumental variable and a binary outcome. Int J Epidemiol. (2014) 43:922–9. doi: 10.1093/ije/dyu005
32. Ebbesen MH, Hannestad YS, Midthjell K, Hunskaar S. Diabetes related risk factors did not explain the increased risk for urinary incontinence among women with diabetes. The Norwegian HUNT/EPINCONT study. BMC urology. (2009) 9:11. doi: 10.1186/1471-2490-9-11
33. Wang W, Peng L, Gao X, Luo D. The frequency of metabolic syndrome in aged female patients (Older than 65 years) with and without stress urinary incontinence: A case-control study. Female pelvic Med reconstructive surgery. (2022) 28:e11–e5. doi: 10.1097/SPV.0000000000001122
34. Mortensen MB, Nordestgaard BG. Elevated LDL cholesterol and increased risk of myocardial infarction and atherosclerotic cardiovascular disease in individuals aged 70-100 years: a contemporary primary prevention cohort. Lancet (London England). (2020) 396:1644–52. doi: 10.1016/S0140-6736(20)32233-9
35. Mahdy Ali K, Wonnerth A, Huber K, Wojta J. Cardiovascular disease risk reduction by raising HDL cholesterol–current therapies and future opportunities. Br J Pharmacol. (2012) 167:1177–94. doi: 10.1111/j.1476-5381.2012.02081.x
36. Lukacz ES, Santiago-Lastra Y, Albo ME, Brubaker L. Urinary incontinence in women: A review. Jama. (2017) 318:1592–604. doi: 10.1001/jama.2017.12137
37. Madsen CM, Varbo A, Nordestgaard BG. Extreme high high-density lipoprotein cholesterol is paradoxically associated with high mortality in men and women: two prospective cohort studies. Eur Heart J. (2017) 38:2478–86. doi: 10.1093/eurheartj/ehx163
38. Johannsen TH, Frikke-Schmidt R, Schou J, Nordestgaard BG, Tybjærg-Hansen A. Genetic inhibition of CETP, ischemic vascular disease and mortality, and possible adverse effects. J Am Coll Cardiol. (2012) 60:2041–8. doi: 10.1016/j.jacc.2012.07.045
39. Zanoni P, Khetarpal SA, Larach DB, Hancock-Cerutti WF, Millar JS, Cuchel M, et al. Rare variant in scavenger receptor BI raises HDL cholesterol and increases risk of coronary heart disease. Sci (New York NY). (2016) 351:1166–71. doi: 10.1126/science.aad3517
40. Kovanecz I, Gelfand R, Lin G, Sharifzad S, Ohanian A, Ricks R, et al. Stem cells from a female rat model of type 2 diabetes/obesity and stress urinary incontinence are damaged by in vitro exposure to its dyslipidemic serum, predicting inadequate repair capacity in vivo. Int J Mol Sci. (2019) 20(16):4044. doi: 10.3390/ijms20164044
41. Kovanecz I, Gelfand R, Sharifzad S, Ohanian A, DeCastro WB, Cooper C, et al. Evaluation of the in vitro damage caused by lipid factors on stem cells from a female rat model of type 2 diabetes/obesity and stress urinary incontinence. Int J Mol Sci. (2020) 21(14):5045. doi: 10.3390/ijms21145045
42. Zhou Y, Li H, Wang L. Mechanism of miR-34a in the metabolism of extracellular matrix in fibroblasts of stress urinary incontinence via Nampt-mediated autophagy. Cell Stress chaperones. (2022) 27:369–81. doi: 10.1007/s12192-022-01278-w
43. Mesregah MK, Mgbam P, Fresquez Z, Wang JC, Buser Z. Impact of chronic hyperlipidemia on perioperative complications in patients undergoing lumbar fusion: a propensity score matching analysis. Eur Spine J. (2022) 31:2579–86. doi: 10.1007/s00586-022-07333-5
44. Gari AM, Alamer EHA, Almalayo RO, Alshaddadi WA, Alamri SA, Aloufi RS, et al. Prevalence of stress urinary incontinence and risk factors among saudi females. Medicina (Kaunas Lithuania). (2023) 59(5):940. doi: 10.3390/medicina59050940
45. Brown JS, Vittinghoff E, Lin F, Nyberg LM, Kusek JW, Kanaya AM. Prevalence and risk factors for urinary incontinence in women with type 2 diabetes and impaired fasting glucose: findings from the National Health and Nutrition Examination Survey (NHANES) 2001-2002. Diabetes Care. (2006) 29:1307–12. doi: 10.2337/dc05-2463
46. Lu Q, Guo P, Liu A, Ares I, Martínez-Larrañaga MR, Wang X, et al. The role of long noncoding RNA in lipid, cholesterol, and glucose metabolism and treatment of obesity syndrome. Medicinal Res Rev. (2021) 41:1751–74. doi: 10.1002/med.21775
47. Martyn JA, Kaneki M, Yasuhara S. Obesity-induced insulin resistance and hyperglycemia: etiologic factors and molecular mechanisms. Anesthesiology. (2008) 109:137–48. doi: 10.1097/ALN.0b013e3181799d45
48. Weinberg AE, Leppert JT, Elliott CS. Biochemical measures of diabetes are not independent predictors of urinary incontinence in women. J urology. (2015) 194:1668–74. doi: 10.1016/j.juro.2015.06.074
Keywords: stress urinary incontinence, lipid, glucose, Mendelian randomization, genetic epidemiology
Citation: Xiang N, Su S, Yang Y, Luo Y, Fu T, Wang L, Lin Y and Huang J (2024) Genetic support of causal association between lipid and glucose metabolism and stress urinary incontinence in women: a bidirectional Mendelian randomization and multivariable-adjusted study. Front. Endocrinol. 15:1394252. doi: 10.3389/fendo.2024.1394252
Received: 01 March 2024; Accepted: 23 July 2024;
Published: 16 September 2024.
Edited by:
Jiahui Si, Peking University, ChinaReviewed by:
Danielle Cristina Honorio França, São Paulo State University, BrazilCopyright © 2024 Xiang, Su, Yang, Luo, Fu, Wang, Lin and Huang. This is an open-access article distributed under the terms of the Creative Commons Attribution License (CC BY). The use, distribution or reproduction in other forums is permitted, provided the original author(s) and the copyright owner(s) are credited and that the original publication in this journal is cited, in accordance with accepted academic practice. No use, distribution or reproduction is permitted which does not comply with these terms.
*Correspondence: Jin Huang, bWljaGFlbF9odWFuZ2ppbkAxNjMuY29t; Yifei Lin, eWxpbkBoc3BoLmhhcnZhcmQuZWR1
Disclaimer: All claims expressed in this article are solely those of the authors and do not necessarily represent those of their affiliated organizations, or those of the publisher, the editors and the reviewers. Any product that may be evaluated in this article or claim that may be made by its manufacturer is not guaranteed or endorsed by the publisher.
Research integrity at Frontiers
Learn more about the work of our research integrity team to safeguard the quality of each article we publish.