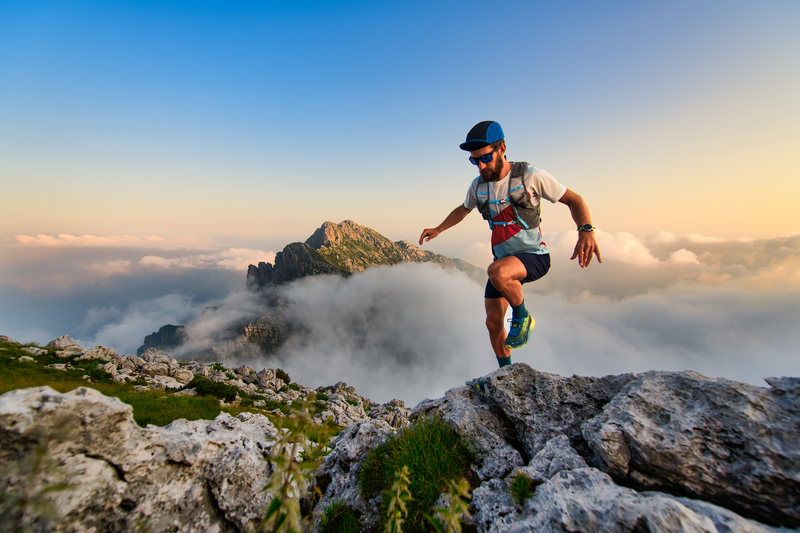
94% of researchers rate our articles as excellent or good
Learn more about the work of our research integrity team to safeguard the quality of each article we publish.
Find out more
ORIGINAL RESEARCH article
Front. Endocrinol. , 21 August 2024
Sec. Thyroid Endocrinology
Volume 15 - 2024 | https://doi.org/10.3389/fendo.2024.1391014
Background: Radioactive iodine (RAI) therapy is a widely used treatment for Graves’ Hyperthyroidism (GH). However, various factors can impact the non-remission rate of GH after single RAI therapy. This study aimed to develop an online dynamic nomogram to assist physicians in providing personalized therapy for GH.
Methods: Data from 454 GH patients who received RAI therapy were retrospectively reviewed and included in the present study. The univariate and multivariate analysis were conducted to investigate and identify independent influencing factors. The nomogram was developed based on the training cohort to explore non-remission rates. Finally, the reliability and accuracy of the constructed nomogram model were verified in the validation cohort via the calibration, receiver operating characteristic (ROC) curve, and decision curve analysis (DCA).
Results: 24-hours radioactive iodine uptake (RAIU24h), effective half-life (Teff), total iodine dose (TID) and iodine dose per gram of thyroid tissue (IDPG) were independent predictors. The nomogram had a high C-index 0.922 (95% CI: 0.892–0.953), for predicting non-remission. The calibration curves demonstrated excellent consistency between the predicted and the actual probability of non-remission. ROC analysis showed that the AUC of the nomogram model and the four independent factors in the training cohort were 0.922, 0.673, 0.760, 0.761, and 0.786, respectively. The optimal cutoff value for the total nomogram scores was determined to be 155. A total score of ≥155 indicates a higher likelihood of non-remission after a single RAI therapy for GH, whereas a score below 155 suggests a greater likelihood of remission. Additionally, the DCA curve indicated that this nomogram had good clinical utility in predicting non-remission.
Conclusion: An online nomogram was constructed with good predictive performance, which can be used as a practical approach to predict and assist physicians in making personalized therapy decisions for GH patients.
Graves’ Hyperthyroidism (GH), caused by Graves’ disease (GD), is a common endocrine disorder characterized by excessive thyroid hormone production (1–3). The treatment options for GH include antithyroid drugs, surgery, and Radioactive iodine (RAI) therapy. Antithyroid drugs (ATD), particularly methimazole, are currently considered the preferred treatment option (4–6). However, in certain circumstances such as liver disease, congestive heart failure, advanced age with comorbidities, or periodic paralysis, RAI may be preferred (4, 5).
For both patients and clinicians, the non-remission rate is a crucial factor in determining the choice of treatment. The relapse rates are 52-53% following ATD, 8-15% following RAI, and 0-10% following thyroidectomy (7, 8). Although, RAI therapy is a widely used treatment due to its effectiveness, convenience, and low price, various factors can impact the non-remission rate of GH. Therefore, it is important to identify the risk factors for non-remission and to develop strategies to minimize the probability of non-remission. However, to our knowledge, there is lack of study to develop a dynamic prediction model for non-remission in GH treated with RAI.
Nomogram is a valuable statistical tool that can transform predictive models into visual graphs, providing a numerical probability of a specific clinical event (9). Therefore, this study aimed to develop an online dynamic nomogram to assist physicians in providing a personalized therapy for GH.
This retrospective analysis includes 129 patients treated at Xijing Hospital (from September 2017 to April 2022) and 325 patients treated at Tianjin Medical University General Hospital (from June 2017 to February 2019), all of whom were referred for RAI therapy for GH. GH was diagnosed based on a combination of clinical and laboratory criteria, including thyrotoxicosis, moderately diffuse goiter, elevated thyroid hormones, decreased thyrotropin (TSH), positive thyrotropin receptor antibody (TRAb), elevated radioactive iodine uptake (RAIU), or accompanied with exophthalmos or pretibial myxedema (4, 10). Thyroid glands >80 grams in size were not represented. The study cohort consisted of 454 GH patients, with a female-to-male ratio of 3.3:1. The average age was 41.8 ± 11.8 years.
All patients were explained the procedure and precautions, including maintaining a low-iodine diet and avoiding iodide-containing medications for 7-14 days prior to treatment. Pregnant and breastfeeding patients were excluded. ATD was to be discontinued at least 3 days prior to RAI therapy.
Laboratory investigations including TSH, triiodothyronine (T3), thyroxine (T4), Free triiodothyronine (FT3), free thyroxin (FT4), Thyroglobulin antibody (TgAb), thyroid peroxidase (TPOAb) and TRAb levels with normal reference ranges as follow: TSH: 0.27-4.2 uIU/mL, T3:1.2-3.1 nmol/L, T4: 66-181 nmol/L, FT3: 3.1-6.8 pmol/L, FT4: 12-22 pmol/L, TgAb: 0-40 IU/mL, TPOAb: 0-35 IU/mL, TRAb: 0-1.5 IU/mL. Thyroid ultrasonography was done to detect the presence of nodules and measure the depth (D, cm), width (W, cm), and length (L, cm) of each thyroid lobe. The total thyroid volume (TV) was determined by summing the calculated products for both lobes, where the volume of one lobe was calculated as D×W×L. The thyroid mass (TM) was calculated with the formula (11): TM (g)=0.479×TV (cm3). Thyroid scan with 99mTechnetium-pertechnetate (99mTc) was done for all patients to exclude the presence of subacute thyroiditis. The RAIU of the thyroid was measured dynamically at 6, 24, 48, 72, and 144 hours to determine the values of 24-hours radioactive iodine uptake (RAIU24h), maximal radioactive iodine uptake (RAIUmax), and effective half-life (Teff).
After consultation with more than two nuclear medicine specialists, personalized 131I doses were calculated by following formula (11): . Where: A— therapeutic radioactivity (mCi); 0.67—correction coefficient; TM—estimated thyroid mass (g); pAD—prediction absorption dose=100Gy/g.
All 454 patients were followed for 6 months to 1 year after receiving RAI therapy. The therapeutic efficacy was evaluated based on the following criteria (10). Euthyroidism was determined by the absence of symptoms or signs of GH and normal levels of FT3, FT4, and TSH in the serum. The diagnosis was hypothyroidism if a patient exhibited symptoms or signs of hypothyroidism (or without), with FT3 and FT4 levels below normal and TSH levels above average. Partial remission was characterized by alleviation of GH symptoms, partial resolution of signs, and reduction in serum levels of FT3 and FT4, although not reaching normal levels. No response was defined as either no significant improvement or an increase in symptoms and signs of hyperthyroidism, with no decrease in serum levels of FT3 and FT4. We categorized euthyroidism and hypothyroidism as “remission” (remission group) and persistent hyperthyroidism (including partial remission and no response) as “non-remission” (non-remission group).
The patients’ clinical records, including age, gender, disease course, RAIU24h, RAIUmax, Teff, Thyroid weight, FT3, FT4, FT3/FT4, TSH, TRAb, TgAb, and TPOAb, total iodine dose (TID), iodine dose per gram of thyroid tissue (IDPG), were thoroughly reviewed. It should be noted that we transformed the continuous variables TRAb, TgAb, and TPOAb into more easily observable categorical variables based on the upper limits of their normal reference ranges and the upper measurable limits.
To ensure the accuracy and clinical application efficiency of the established model, all patients were randomly divided into training and validation cohorts at a 7:3 ratio using R software. The patients were categorized based on their therapeutic efficacy and divided into two groups. Consequently, the training cohort was separated into group 1 (remission, n=217) and group 2 (non-remission, n=101). The validation cohort was separated into group A (remission, n=87) and group B (non-remission, n=49). Subsequently, univariate and multivariate analyses were conducted to investigate the effects of the variables. Moreover, a nomogram was developed based on the training cohort to predict the non-remission rates for GH patients, serving as a valuable tool in clinical practice. Finally, the predictive capability of the constructed nomogram was validated in the validation cohort.
The T-test was used to compare differences in age, Teff, RAIU24h, RAIUmax, TSH, TID, and IDPG, while the Mann-Whitney U test was used to compare differences in thyroid weight, FT3, FT4, and FT3/FT4. The chi-squared test was used to analyze differences in categorical variables. Multivariate analysis was used to identify independent factors associated with non-remission of Graves’ hyperthyroidism after RAI therapy. The performance of prediction and discrimination was evaluated using Harrell’s concordance index (C-index) (12), and the predictive power of the nomogram was shown using the ROC curve, with the area under the curve (AUC) values listed. The calibration curve was generated using the rms package in R language, which reflected the relationship between the predicted and actual incidence. The DCA curve was used to evaluate the clinical application value of the nomogram model by showing the net benefit of the observers. The nomogram was established using R software (version 4.0.5; http://www.rproject.org/), and all statistical analyses were performed using SPSS software version 25.0 (SPSS Inc Chicago, IL, USA).
During the study period, 454 patients with GH who met the inclusion criteria were included, with 318 and 136 patients assigned to the training and validation cohorts, respectively (Figure 1).
Table 1 lists the clinical characteristics of the patients, which were similar between the training and validation cohorts. Non-remission after RAI therapy was observed in 103 (32.4%) and 47 (34.6%) patients in the two cohorts, respectively.
Upon analyzing the relationship between clinical characteristics and non-remission of GH after single RAI therapy, a total of 16 factors were involved in the univariate analyses (Table 2). The results showed that patients with shorter Teff, lower RAIU24h, higher FT3/FT4, higher TRAb level, lower TID and IDPG would have a higher probability of non-remission of GH (P<0.001, P<0.001, P=0.044, P<0.001, P<0.001, P<0.001, respectively). However, we found no statistically significant differences in age (P =0.398), gender (P =0.566), disease course (P =0.721), RAIUmax (P =0.146), thyroid weight (P =0.391), FT3 (P =0.631), FT4 (P =0.899), TSH (P =0.138), TgAb (P =0.110), and TPOAb (P =0.331).
Multivariate logistic regression analysis using a stepwise method was performed based on related factors from the univariate analysis. The results revealed that a total of 4 factors, including RAIU24h, Teff, TID, IDPG, were verified as independent risk factors that predicted non-remission of GH after RAI therapy. On multivariate analysis, with results reported as odds ratio (95% CI), subjects with RAIU24h (0.910 [0.874-0.947]), Teff (0.362 [0.262-0.501]), TID (0.777 [0.702-0.959]) and IDPG (0.982 [0.976-0.987]) had a higher probability of non-remission of GH after RAI therapy (Table 3).
Based on the multivariate analysis results, the independently associated risk factors were used to form a simple-to-use nomogram model for predicting non-remission probability, which is illustrated in Figure 2A and available online (https://yufengyxlxt.shinyapps.io/DynNom_model/) and presented in Figure 2B. Each variable was assigned a score on a scale. A total score was obtained by adding scores for each selected variable. A vertical line was then drawn along the total points series to estimate the risk of non-remission of GH after RAI therapy. The prediction model showed that IDPG was the top 1 contributor to non-remission, followed by TID, Teff and RAIU24h (Figure 2A).
Figure 2. Nomogram prediction model for non-remission probability. (A) Established nomogram in the training cohort incorporating the following four parameters: Teff, RAIU24h, TID and IDPG. (B) Online dynamic nomogram accessible at https://yufengyxlxt.shinyapps.io/DynNom_model/.
The nomogram exhibited a high C-index of 0.922 (95% CI: 0.892–0.953) for predicting non-remission of GH following RAI therapy, indicating a high level of accuracy in the model’s predictions. Calibration curves demonstrated excellent consistency between the predicted results and the actual probability of non-remission in GH patients after RAI therapy (Figure 3A). ROC analysis revealed that the AUC of the nomogram model and the four independent factors (including RAIU24h, Teff, TID, and IDPG) in the training cohort were 0.922 (95% CI: 0.891–0.953), 0.673 (95% CI:0.610-0.735), 0.760 (95% CI: 0.702-0.817), 0.760 (95% CI: 0.694-0.825), and 0.786 (95% CI: 0.731-0.840), respectively. The five models could be ranked from best to worst as follows: nomogram model > IDPG > TID > Teff > RAIU24h (Figure 3B). Similar results were observed in the validation cohort. Additionally, the DCA curve indicated that this nomogram had good clinical utility in predicting non-remission of GH after RAI therapy (Figure 3C). The optimal cutoff value for the total nomogram scores was determined to be 155, with specificity and sensitivity of 91.2% and 83.5% in the training cohort, and 84.3% and 80.9% in the validation cohort, respectively (Figure 3B).
Figure 3. (A) Calibration curves showing the agreement between predicted and observed probabilities of non-remission, for both training and validation cohorts. The closer the curve aligns with the diagonal dashed line (ideal calibration line), the better the predictive accuracy. (B) ROC curves of the nomogram prediction for non-remission probability of the training and validation cohorts. (C) Decision curve analysis (DCA) illustrating the clinical usefulness of the nomogram for predicting non-remission probability in the training and validation cohorts. DCA assesses the net benefit of using the nomogram across a range of threshold probabilities, aiding clinical decision-making.
Over the years, RAI therapy has been one of the most important methods for treating GH (4). Many physicians prefer to use a large dose of the isotope to achieve early hypothyroidism and avoid the need for another dose of iodine-131. However, there are still 8-15% of patients who do not achieve complete remission after the initial treatment and require a second round of RAI therapy 3-6 months later. Numerous studies have been conducted to determine the ideal dosage of iodine-131 that would increase the likelihood of achieving euthyroid in patients. However, these studies often overlooked other predictive factors that could influence the treatment outcome (13–15). In our study, we proposed four predictive factors: RAIU24h, Teff, TID, and IDPG, and used these four factors to construct a nomogram model for predicting non-remission after the first RAI therapy.
RAIU24h plays a crucial role in determining the iodine-131 dose and is included in various iodine-131 dose calculation formulas. Numerous studies have been conducted to investigate the relationship between RAIU24h and the efficacy of RAI therapy, both domestically and internationally. In our study, we observed patients with high RAIU24h had better outcomes with RAI therapy, which aligns with the conclusions of studies such as NordykeRA and NordykeRA, where higher RAIU24h was associated with higher cure rates (16–18). Contrary to our findings, some studies have shown a negative correlation between the cure rate of RAI therapy for GH and RAIU24h, indicating that higher RAIU24h is associated with lower cure rates (15, 19–21). There are several possible explanations for this phenomenon. Firstly, there may be an inverse relationship between the sensitivity of thyroid tissue to radiation and RAIU24h, as demonstrated in the study by Di Martino F et al (22). Secondly, the iodine-131 dose for treatment decreases as RAIU24h increases, and low therapeutic dose of iodine-131 may reduce the uptake of iodine-131 in thyroid tissue (23, 24). Despite the inconsistency in results, RAIU24h is still considered an important factor influencing the success of RAI therapy.
Teff refers to the time required for the radioactive nuclide iodine-131 to decrease to half of its original amount in the body, due to the combined effects of physical decay and biological activity. It reflects the duration of iodine-131 residence in thyroid tissue, which directly affects the actual absorbed dose of beta radiation in thyroid tissue, thereby influencing the extent of damage to the thyroid tissue by iodine-131. Yu et al. (11) found that a longer Teff increases the likelihood of developing hypothyroidism, while other study (25) reported no significant difference in Teff between the remission and non-remission groups after RAI therapy. In this study, both univariate and multivariate logistic regression analyses found that shorter Teff is associated with non-remission, which is consistent with the current general view. Shorter Teff reflects a high metabolic state of thyroid cells, resulting in a shorter residence time and lower absorbed dose of thyroid tissue (26).
The IDPG and TID can impact the actual absorbed dose of the thyroid tissue and therapeutic effect of RAI. Previous studies have shown that lower do IDPG result in higher failure rates (27). In both the univariate and multivariate logistic regression analyses in this study, the TID and IDPG identified as positive risk factors affecting treatment outcomes. This is consistent with the general consensus (28). We speculate that when higher TID, especially higher IDPG, are administered, it ensures sufficient beta radiation within the thyroid tissue to exert a destruction, resulting in satisfactory treatment outcomes. Currently, the typical IDPG in clinical practice is 70-150 µCi/g, but evidence suggests that in order to achieve a hypothyroid state in patients with GH, the IDPG needs to be greater than 150 µCi/g (29, 30). The ATA guideline recommends an IDPG of 50-200µCi in clinical practice, while the Chinese guideline suggests 70-150µCi for IDPG (4, 10). However, there is evidence suggesting that in order to achieve hypothyroid state, the IDPG needs to be greater than 15 MBq (405 µCi) or dosimetry aiming at delivering at least 300 Gy to the thyroid tissue for functional ablation (31, 32). Peters’ research has shown that RAI efficacy is demonstrated for glands up to 80 ml, and it is important to note that the patients presented in our study fit within previously shown gland sizes for RAI efficacy (<80 gm). In this study, glands were in the modestly enlarged range, and glands >80 gm were generally excluded. These findings are in agreement with those reported by Peters (31, 32). It is worth noting that in Table 2, the TID range in the validation group was 9.1 ± 5.0 mCi, which is notably lower than the commonly used fixed dose of 555 MBq (15 mCi). This preference for lower treatment doses is influenced by patient-centered or cultural considerations, aiming to restore euthyroidism rather than induce hypothyroidism in the initial management of GH using single RAI therapy. The choice of treatment target may differ from practices in other regions, such as the United States, where the standard approach is to use RAI doses that induce hypothyroidism. It should be emphasized that using lower doses aimed at restoring euthyroidism may result in lower long-term remission rates and could potentially limit the applicability of the model.
Through univariate and multivariate analyses, these four factors were ultimately identified as independent factors affecting the non-remission rate after initial RAI therapy in GH patients. However, in order to more accurately predict the probability of non-remission, we did not choose to use a single independent factor for prediction, but instead combined these four factors to construct a more predictive nomogram model. As shown in ROC curve analysis, it can be clearly seen that the nomogram model performed better in terms of predictive ability compared to single independent factor, both in the training set and validation set. Additionally, the nomogram model also demonstrated excellent consistency and clinical net benefit in calibration curve and DCA curve analysis. By applying this model, we can guide the adjustment of dosage before RAI administration to achieve a lower rate of non-remission and reduce the chances of a second RAI therapy.
However, it is important to acknowledge the limitations of our study. Firstly, this was a retrospective study with some inevitable bias. Secondly, the sample size of two center was small. The novel nomogram model was only validated internally. Therefore, a multicenter clinical study with a larger sample size could be conducted for external validation to verify the clinical benefits in the subsequent research. Thirdly, the patients included in this study had moderately enlarged thyroid glands, not markedly enlarged glands. This may limit the applicability of our model in treating GH patients with very large goiters. Nevertheless, this novel nomogram model does have the potential to improve clinical practice of personalized therapy.
This study was the first to develop and validate online nomogram based on the independent risk factors to dynamically predict non-remission probability in individuals with GH after initial RAI therapy. This novel model demonstrated superior performance and discriminative power, providing vital information for nuclear medicine physicians to design customized clinical treatments for their patients.
The raw data supporting the conclusions of this article will be made available by the authors, without undue reservation.
The studies involving humans were approved by Ethical Committee of Xijing Hospital. The studies were conducted in accordance with the local legislation and institutional requirements. Written informed consent for participation was not required from the participants or the participants’ legal guardians/next of kin in accordance with the national legislation and institutional requirements.
FY: Formal analysis, Writing – original draft, Investigation, Software, Writing – review & editing. WM: Validation, Writing – review & editing, Software. XL: Data curation, Writing – review & editing. RZ: Methodology, Writing – review & editing. FK: Supervision, Writing – review & editing. WY: Investigation, Writing – review & editing. RW: Project administration, Writing – review & editing, Supervision. JW: Funding acquisition, Writing – review & editing, Supervision.
The author(s) declare financial support was received for the research, authorship, and/or publication of this article. The present study was supported by grants from the National Natural Science Foundation of China (Nos. 81971646 and 91959208), the Natural Science Foundation of Shaanxi Province (No. 2023-JC-YB-757) and the Medical Research Project of the Xi’an Innovation Capacity Support Plan (No. 23YXYJ0169).
The authors declare that the research was conducted in the absence of any commercial or financial relationships that could be construed as a potential conflict of interest.
All claims expressed in this article are solely those of the authors and do not necessarily represent those of their affiliated organizations, or those of the publisher, the editors and the reviewers. Any product that may be evaluated in this article, or claim that may be made by its manufacturer, is not guaranteed or endorsed by the publisher.
1. Burch HB, Burman KD, Cooper DS. A 2011 survey of clinical practice patterns in the management of Graves' disease. J Clin Endocrinol Metab. (2012) 97:4549–58. doi: 10.1210/jc.2012-2802
2. Smith TJ, Hegedüs L. Graves' Disease. New Engl J Med. (2016) 375:1552–65. doi: 10.1056/NEJMra1510030
3. Wiersinga WM, Poppe KG, Effraimidis G. Hyperthyroidism: aetiology, pathogenesis, diagnosis, management, complications, and prognosis. Lancet Diabetes Endocrinol. (2023) 11:282–98. doi: 10.1016/s2213-8587(23)00005-0
4. Ross DS, Burch HB, Cooper DS, Greenlee MC, Laurberg P, Maia AL, et al. 2016 American thyroid association guidelines for diagnosis and management of hyperthyroidism and other causes of thyrotoxicosis. Thyroid. (2016) 26:1343–421. doi: 10.1089/thy.2016.0229
5. Kahaly GJ, Bartalena L, Hegedüs L, Leenhardt L, Poppe K, Pearce SH. 2018 European thyroid association guideline for the management of Graves' Hyperthyroidism. Eur Thyroid J. (2018) 7:167–86. doi: 10.1159/000490384
6. Brito JP, Schilz S, Singh Ospina N, Rodriguez-Gutierrez R, Maraka S, Sangaralingham LR, et al. Antithyroid drugs-the most common treatment for Graves' Disease in the United States: A nationwide population-based study. Thyroid. (2016) 26:1144–5. doi: 10.1089/thy.2016.0222
7. Sundaresh V, Brito JP, Wang Z, Prokop LJ, Stan MN, Murad MH, et al. Comparative effectiveness of therapies for Graves' hyperthyroidism: a systematic review and network meta-analysis. J Clin Endocrinol Metab. (2013) 98:3671–7. doi: 10.1210/jc.2013-1954
8. Sundaresh V, Brito JP, Thapa P, Bahn RS, Stan MN. Comparative effectiveness of treatment choices for Graves' Hyperthyroidism: A historical cohort study. Thyroid. (2017) 27:497–505. doi: 10.1089/thy.2016.0343
9. Iasonos A, Schrag D, Raj GV, Panageas KS. How to build and interpret a nomogram for cancer prognosis. J Clin Oncol. (2008) 26:1364–70. doi: 10.1200/jco.2007.12.9791
10. Medicine CSoN. Clinical guidelines for 131I treatment of Graves' hyperthyroidism (2021 edition). Chin J Nucl Med Mol Imaging. (2021) 41:242–53. doi: 10.3760/cma.j.cn321828-20201109-00405
11. Yu F, Zhang R, Zhang G, Meng Z, Liu X, He Y, et al. Predictive value of a thyroid-absorbed dose with a shorter effective half-life on efficacy in Graves disease patients receiving iodine-131 therapy. Med Sci monitor. (2021) 27:e928796. doi: 10.12659/msm.928796
12. Harrell FE Jr, Califf RM, Pryor DB, Lee KL, Rosati RA. Evaluating the yield of medical tests. JAMA. (1982) 247:2543–6. doi: 10.1001/jama.1982.03320430047030
13. Chen DY, Schneider PF, Zhang XS, He ZM, Jing J, Chen TH. Striving for euthyroidism in radioiodine therapy of Graves' disease: a 12-year prospective, randomized, open-label blinded end point study. Thyroid. (2011) 21:647–54. doi: 10.1089/thy.2010.0348
14. Piccardo A, Ugolini M, Altrinetti V, Righi S, Fiz F, Foppiani L, et al. Radioiodine therapy of Graves' disease. Q J Nucl Med Mol Imaging. (2021) 65:132–7. doi: 10.23736/s1824-4785.21.03337-9
15. Liu M, Jing D, Hu J, Yin S. Predictive factors of outcomes in personalized radioactive iodine ((131)I) treatment for Graves' disease. Am J Med Sci. (2014) 348:288–93. doi: 10.1097/maj.0000000000000288
16. Nordyke RA, Gilbert FI Jr. Optimal iodine-131 dose for eliminating hyperthyroidism in Graves' disease. J Nucl Med. (1991) 32:411–6.
17. Sabri O, Zimny M, Schreckenberger M, Reinartz P, Nowak B, Ostwald E, et al. [Characterization of therapy failures in radioiodine therapy of Graves' disease without simultaneous antithyroid agents]. Nuklearmedizin Nucl Med. (2001) 40:1–6.
18. Xing YZ, Zhang K, Jin G. Predictive factors for the outcomes of Graves' disease patients with radioactive iodine (131I) treatment. Bioscience Rep. (2020) 40:BSR20191609. doi: 10.1042/bsr20191609
19. de Jong JA, Verkooijen HM, Valk GD, Zelissen PM, de Keizer B. High failure rates after (131)I therapy in Graves hyperthyroidism patients with large thyroid volumes, high iodine uptake, and high iodine turnover. Clin Nucl Med. (2013) 38:401–6. doi: 10.1097/RLU.0b013e3182817c78
20. Walter MA, Christ-Crain M, Eckard B, Schindler C, Nitzsche EU, Müller-Brand J, et al. Radioiodine therapy in hyperthyroidism: inverse correlation of pretherapeutic iodine uptake level and post-therapeutic outcome. Eur J Clin Invest. (2004) 34:365–70. doi: 10.1111/j.1365-2362.2004.01349.x
21. Kristoffersen US, Hesse B, Rasmussen AK, Kjaer A. Radioiodine therapy in hyperthyroid disease: poorer outcome in patients with high 24 hours radioiodine uptake. Clin Physiol Funct Imaging. (2006) 26:167–70. doi: 10.1111/j.1475-097X.2006.00666.x
22. Di Martino F, Traino AC, Brill AB, Stabin MG, Lazzer M. A theoretical model for prescription of the patient-specific therapeutic activity for radioiodine therapy of Graves' disease. Phys Med Biol. (2002) 47:1493–9. doi: 10.1088/0031-9155/47/9/305
23. Sabri O, Zimny M, Schreckenberger M, Meyer-Oelmann A, Reinartz P, Buell U. Does thyroid stunning exist? A model with benign thyroid disease. Eur J Nucl Med. (2000) 27:1591–7. doi: 10.1007/s002590000348
24. Happel C, Kranert WT, Ackermann H, Binse I, Bockisch B, Gröner D, et al. Thyroid stunning in radioiodine-131 therapy of benign thyroid diseases. Endocrine. (2019) 63:537–44. doi: 10.1007/s12020-018-01833-5
25. Sabri O, Zimny M, Schreckenberger M, Reinartz P, Ostwald E, Buell U. Radioiodine therapy in Graves' disease patients with large diffuse goiters treated with or without carbimazole at the time of radioiodine therapy. Thyroid. (1999) 9:1181–8. doi: 10.1089/thy.1999.9.1181
26. Bonnema SJ, Hegedüs L. Radioiodine therapy in benign thyroid diseases: effects, side effects, and factors affecting therapeutic outcome. Endocrine Rev. (2012) 33:920–80. doi: 10.1210/er.2012-1030
27. Alexander EK, Larsen PR. High dose of (131)I therapy for the treatment of hyperthyroidism caused by Graves' disease. J Clin Endocrinol Metab. (2002) 87:1073–7. doi: 10.1210/jcem.87.3.8333
28. Mizokami T, Hamada K, Maruta T, Higashi K, Tajiri J. Long-term outcomes of radioiodine therapy for juvenile Graves disease with emphasis on subsequently detected thyroid nodules: A single institution experience from Japan. Endocrine Pract. (2020) 26:729–37. doi: 10.4158/ep-2019-0468
29. Peters H, Fischer C, Bogner U, Reiners C, Schleusener H. Reduction in thyroid volume after radioiodine therapy of Graves' hyperthyroidism: results of a prospective, randomized, multicentre study. Eur J Clin Invest. (1996) 26:59–63. doi: 10.1046/j.1365-2362.1996.98243.x
30. Peters H, Fischer C, Bogner U, Reiners C, Schleusener H. Treatment of Graves' hyperthyroidism with radioiodine: results of a prospective randomized study. Thyroid. (1997) 7:247–51. doi: 10.1089/thy.1997.7.247
31. Stokkel MP, Handkiewicz Junak D, Lassmann M, Dietlein M, Luster M. EANM procedure guidelines for therapy of benign thyroid disease. Eur J Nucl Med Mol Imaging. (2010) 37:2218–28. doi: 10.1007/s00259-010-1536-8
Keywords: Graves’ hyperthyroidism, radioactive iodine therapy, non-remission, prediction model, nomogram
Citation: Yu F, Ma W, Li X, Zhang R, Kang F, Yang W, Wang R and Wang J (2024) Nomogram construction and evaluation for predicting non-remission after a single radioactive iodine therapy for Graves’ hyperthyroidism: a retrospective cohort study. Front. Endocrinol. 15:1391014. doi: 10.3389/fendo.2024.1391014
Received: 24 February 2024; Accepted: 07 August 2024;
Published: 21 August 2024.
Edited by:
Paul B. Kaplowitz, Children’s National Hospital, United StatesReviewed by:
Jason Wexler, MedStar Washington Hospital Center, United StatesCopyright © 2024 Yu, Ma, Li, Zhang, Kang, Yang, Wang and Wang. This is an open-access article distributed under the terms of the Creative Commons Attribution License (CC BY). The use, distribution or reproduction in other forums is permitted, provided the original author(s) and the copyright owner(s) are credited and that the original publication in this journal is cited, in accordance with accepted academic practice. No use, distribution or reproduction is permitted which does not comply with these terms.
*Correspondence: Jing Wang, MTM5MDkyNDU5MDJAMTYzLmNvbQ==; Renfei Wang, Um9zbHluX2VuQDE2My5jb20=
†These authors share first authorship
Disclaimer: All claims expressed in this article are solely those of the authors and do not necessarily represent those of their affiliated organizations, or those of the publisher, the editors and the reviewers. Any product that may be evaluated in this article or claim that may be made by its manufacturer is not guaranteed or endorsed by the publisher.
Research integrity at Frontiers
Learn more about the work of our research integrity team to safeguard the quality of each article we publish.