- 1School of Clinical Medicine, Chengdu University of Traditional Chinese Medicine, Chengdu, Sichuan, China
- 2Department of Communication Sciences and Disorders, MGH Institute of Health Professions, Boston, MA, United States
- 3General Practice, Chengdu Integrated Traditional Chinese Medicine (TCM) & Western Medicine Hospital, Chengdu, China
Objective: Stroke risk factors often exert long-term effects, and Mendelian randomization (MR) offers significant advantages over traditional observational studies in evaluating the causal impact of these factors on stroke. This study aims to consolidate and evaluate the relationships between potential causal factors and stroke risk, drawing upon existing MR research.
Methods: A comprehensive search for MR studies related to stroke was conducted up to August 2023 using databases such as PubMed, Web of Science, Embase, and Scopus. This meta-analysis examines the relationships between potential causative factors and stroke risk. Both random-effects and fixed-effects models were utilized to compile the dominance ratios of various causative elements linked to stroke. The reliability of the included studies was assessed according to the Strengthening the Reporting of Observational Studies in Epidemiology incorporating Mendelian Randomization (STROBE-MR) guidelines.
Results: The analysis identified several risk factors for stroke, including obesity, hypertension, low-density lipoprotein cholesterol (LDL-C), chronic kidney disease (CKD), and smoking. Protective factors included high-density lipoprotein cholesterol (HDL-C), estimated glomerular filtration rate (eGFR), and educational attainment. Subgroup analysis revealed that type 2 diabetes mellitus (T2DM), diastolic blood pressure (DBP) are risk factors for ischemic stroke (IS).
Conclusion: This study confirms that variables such as obesity, hypertension, elevated LDL-C levels, CKD, and smoking are significantly linked to the development of stroke. Our findings provide new insights into genetic susceptibility and potential biological pathways involved in stroke development.
Systematic review registration: https://www.crd.york.ac.uk/PROSPERO, identifier CRD42024503049.
1 Introduction
Stroke, a severe neurological condition, is often triggered by decreased blood flow or breakdown of vascular structures, leading to irreversible damage to neurons in the cerebrum (1). Stroke is the second most common cause of death worldwide and a significant contributor to severe disability, impacting more than 150,000 individuals each year. According to the Global Burden of Disease (GBD) study, the annual number of strokes and stroke-related deaths increased substantially between 1990 and 2019, with incident strokes rising by 70% and stroke-related deaths by 43%. Notably, it is anticipated that by 2030, the incidence of ischemic stroke (IS), one of the two major subtypes of stroke, will escalate to 4.90 million globally (2–5). Especially in low-income countries, the disease burden of stroke is even higher (6–11). In recent years, studies on the etiology of stroke have gradually revealed connections between numerous life-style and physiologic factors and their likelihood of leading to the disease (12–15). Lower educational attainment is linked to an increased risk of stroke. Obesity, especially increased body mass index (BMI) and waist circumference (WC), is an independent predictor of stroke occurrence (16). Furthermore, smoking may result in the emergence of additional cardiovascular risk factors such as dyslipidemia, and hypertension through metabolic and hemodynamic changes (17). Importantly, the presence of chronic kidney disease (CKD) dramatically increases death risk in individuals suffering from a stroke (18). However, establishing a direct causal connection between a particular cause and its associated disease is difficult due to inherent limitations in observation-based studies, like potential confounding factors and skewed information. These hindrances make proving causality a tricky task. Mendelian Randomization (MR) is a method in human genetics, using single nucleotide polymorphisms (SNPs) as instrumental variables (IVs) in genome-wide association study(GWAS) focus on exposure events (19). It is specifically aimed at elucidating the causal connections between exposure and outcomes (20). Recent research undertaking MR has turned its attention towards identifying how specific risk factors could potentially contribute to a stroke. Given the diverse nature of these studies, it becomes overwhelmingly essential to carry out comprehensive systematic reviews coupled with meta-analysis. Such evaluations are vital in bolstering the evidence that supports effective strategies in both the prevention and treatment of stroke.
This paper presents a systematic review and meta-analysis of MR studies related to stroke. We evaluate a spectrum of stroke-related risk factors, including obesity, lipid profiles, blood pressure (BP), renal function, and environmental factors. The primary outcome of this study is total stroke. Additionally, we focus on ischemic stroke (IS), defined as an episode of neurological dysfunction caused by focal cerebral, spinal, or vascular infarction, assessed in subgroup analysis (21, 22).
2 Materials and method
2.1 Literature search
The configuration and documentation of this systematic evaluation were conducted following the recommendations of the Preferred Reporting Items for Systematic Reviews and Meta-Analyses (PRISMA) guideline (23, 100) and pre-registered with PROSPERO(CRD42024503049).
In pursuit of a comprehensive scholarly investigation, a thorough search was carried out through four databases: PubMed, Web of Science, Embase, and Scopus. The databases were searched for relevant citations published from their inception to August 20, 2023, using the search terms “Mendelian randomization analysis” combined with “stroke.” The strategies used to search the databases are described in Supplementary Table 1. To ensure a comprehensive literature review, we carefully reviewed the references in the selected studies to identify relevant articles potentially missed in the preliminary search. Each of these articles was manually verified for relevance. When discrepancies arose among the reviewers, they engaged in deliberative discussions until a unanimous consensus was reached. Prior to the assessment using the predefined inclusion and exclusion criteria, articles were initially screened to ensure relevance and de-emphasize any irrelevant ones.
The following criteria guided the selection of studies for inclusion in the screening process:
1. International studies published up to August 2023 that utilized the MR method to investigate causal links between stroke or related phenotypes and various risk factors;
2. All studies using genetic variation to establish causal relationships regarding the impact of exposure factors on stroke outcomes;
3. All studies that includes MR in their analysis using GWAS or phenotype-wide association studies (PheWAS);
4. Studies reporting outcomes as 95% confidence intervals (CI), odds ratios (OR), and relative risks (RR), or providing raw data that can be converted to these metrics;
5. Original research articles.
The following criteria guide the exclusion of studies in the screening process:
1. Diagnosis of other types of cerebrovascular disease, such as cerebral atherosclerosis, cerebral arteritis, cerebral aneurysm, cerebral artery injury, intracranial vascular malformation, thrombosis, and cerebral arteriovenous fistula;
2. Relevant outcome indicators were not reported or data were incomplete (101);
3. Repeatedly published studies;
4. Editorials, letters to the editor, review articles, conference coverage, systematic reviews, case reports, and experimental animal studies.
2.2 Literature screening and data extraction
All identified literature was imported into EndNote 20 for systematic review. Two researchers independently conducted the screening process, initially reviewing titles and abstracts, followed by full-text assessments in accordance with predefined inclusion and exclusion criteria. Articles selected during this initial phase underwent a more detailed full-text review. In cases of disagreement about eligibility, a third researcher was consulted to make the final decision.
Data extraction involved several key parameters, including the first author’s name, publication year, study ethnicity, consortium responsible for stroke genomics research, exposure variables, outcome sample size, and major findings. Additionally, OR and 95% CI were extracted using various MR methods, including inverse variance weighted (IVW), weighted median estimator (WME), MR-Egger regression, simple mode, and weighted mode (see Supplementary Table 2).
2.3 Assessment of methodological quality
The methodological quality of the studies included in the meta-analysis was evaluated using a modified version of the Strengthening the Reporting of Observational Studies in Epidemiology for Mendelian Randomization (STROBE-MR) guidelines (24). We converted quality scores into percentages: studies scoring below 75% were classified as poor quality, those scoring between 75% and 85% were considered moderate quality, and studies scoring above 85% were regarded as high quality (25, 26). Two researchers independently conducted the quality assessment, and any discrepancies were resolved through consultation with a third researcher.
2.4 Statistical analysis
To qualify for the meta-analysis, each study had to meet specific criteria. It needed to be among at least two independent investigations focusing on the etiology of stroke or examining the causal relationships between stroke and various genetic or contributing factors. Statistical analysis was conducted using Stata 17.0 software. Outcome measures included OR values and 95% CI as effect size metrics, with a significance threshold of α=0.05. Inter-study heterogeneity was assessed using the χ2 test, with the p-value and I² as indicators. For I²< 50% and p > 0.1, a fixed-effects model was applied. For I² ≥ 50%, indicating substantial heterogeneity, sensitivity and subgroup analyses were conducted to identify sources of heterogeneity. If sources of heterogeneity could not be resolved, a random-effects model was employed (27, 28). Sensitivity analysis was performed to assess the stability of the results by comparing the combined effect estimates from both random-effects and fixed-effects models. Significant differences indicated high sensitivity and potential instability in the outcomes of the meta-analysis. Egger’s test was utilized for funnel plot analysis to evaluate publication bias, with a p-value < 0.05 considered statistically significant.
3 Results
3.1 Literature screening and selection
The database search initially resulted in 2,089 documents. Following the use of EndNote 20 and manual verification by the researchers, this number was narrowed down to 881 articles. During the preliminary review of titles and abstracts, two researchers identified 30 pertinent articles. Subsequent full-text reviews further reduced the selection down to 11 articles for inclusion. The literature screening process and its outcomes are illustrated in Figure 1.
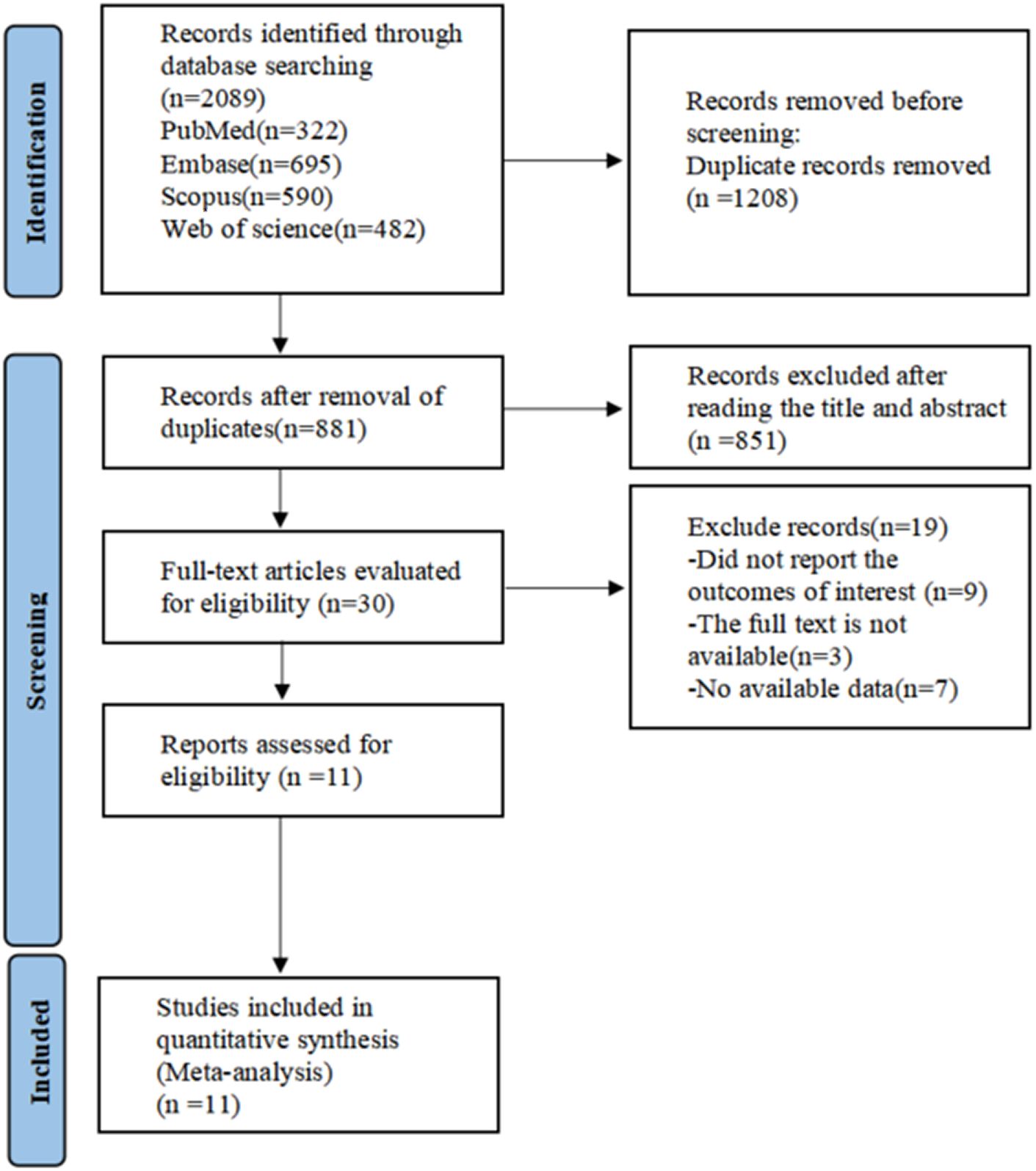
Figure 1. PRISMA flow diagram of the study process. PRISMA, Preferred Reporting Items for Systematic review and Meta-analysis.
3.2 Characteristics and quality of included studies
The meta-analysis included 11 MR studies (29–39). All studies were evaluated as high quality (Supplementary Table 3). These studies included subjects from multiple datasets (29–32, 34, 37), covering Europeans (31–36, 38, 39), Africans (29, 37), Asians (29, 30, 37), and Latin Americans (29, 37). Most MR studies used a strict linkage disequilibrium (LD) threshold (R2 < 0.001) and restricted the genetic distance to a maximum of 10,000 kilobases (kb) to select independent SNPs as IVs for exposure. However, some studies opted to identify all conditionally independent SNPs in GWAS. The number of SNPs used in the studies varied from tens to thousands, with one study failing to report the number of SNPs used in MR (30). One study did not report the sample size for the outcome variable (39). The most frequently utilized cohort was the UK Biobank, which was featured in seven studies (29, 32, 34, 36, 37, 39). Additionally, four of the cohorts focused on stroke outcomes and all utilized the MEGASTROKE consortium (31, 32, 36–38). Two studies considered stratification by arterial gender (39), and population (30) respectively. All studies were statistically analyzed for MR, with one study reporting results based on IVW, MR-Egger regression, WME, simple mode, and weighted mode (34). Due to the limited number of included studies, funnel plots were not generated for all phenotypes.
3.3 Meta-analysis results
A total of four studies on type 2 diabetes mellitus (T2DM) (29, 35–37), two studies on waist-hip ratio (WHR) (29, 32), five studies on BMI (32, 33, 35, 37, 39), five studies on triglycerides (TGs) (six datasets) (29–31, 35, 37), four studies on high-density lipoprotein cholesterol (HDL-C) (five datasets) (30, 31, 35, 37), four studies on low-density lipoprotein cholesterol (LDL-C) (five datasets) (30, 31, 35, 37), four studies on systolic blood pressure (SBP) (29, 32, 35, 36), three studies on diastolic blood pressure (DBP) (29, 35, 36), two studies on hypertension (35–37), two studies on estimated glomerular filtration rate (eGFR) (three datasets) (34, 38), two studies on CKD (three datasets) (34, 38), two studies on smoking (35, 37), and two studies on educational level (35, 36).
3.3.1 Obesity related indicators
The study demonstrated that genetic tendencies towards two obesity-related measures correlated with an increased stroke risk, although this correlation was not observed for one of the measures (Figure 2). Specifically, T2DM [1.11(1.07-1.14)], WHR [1.14, (0.98-1.33)], BMI [1.08(1.05-1.12)]. There was heterogeneity between T2DM and stroke (I2 = 62.5%, p=0.046), which we attribute to variations in the demographics of the study populations. Therefore, we utilized a random-effects model. Heterogeneity was also observed in the correlation between WHR and stroke (I2 = 64.4%, p=0.094). It is possible that differences in the populations used for the exposure factors contributed to this, while the outcome metrics were based on the same population. There was no heterogeneity between BMI and stroke risk when using a fixed-effects model.
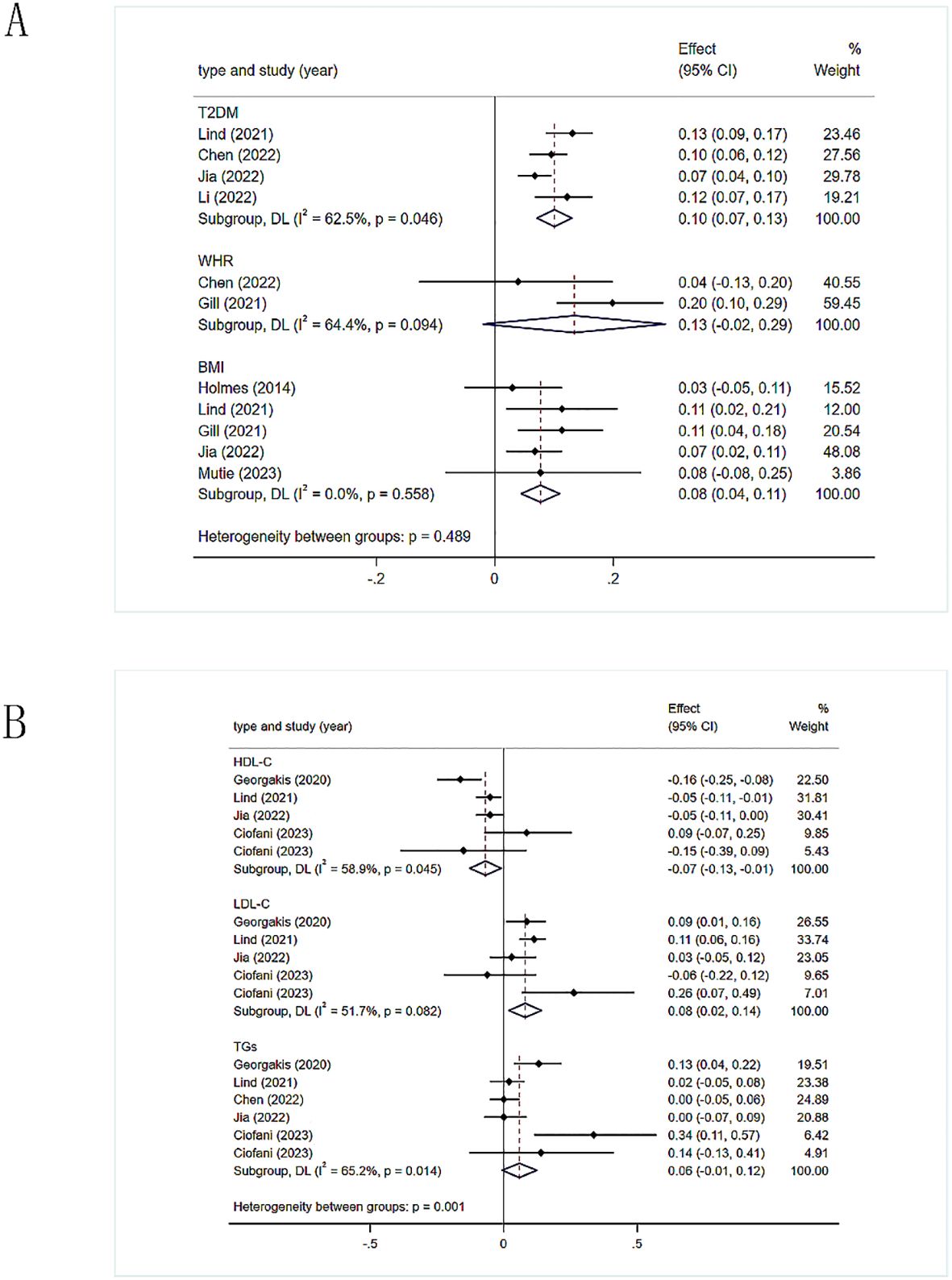
Figure 2. (A) Meta-analysis results of the association between genetic liability to obesity-related indicators and risk of stroke. T2DM, type 2 diabetes mellitus; WHR, waist-hip ratio; BMI, body mass index. (B) Meta-analysis results of the association between genetic liability to lipid related indicators and risk of stroke. HDL-C, high-density lipoprotein cholesterol; LDL-C, low-density lipoprotein cholesterol; TGs, triglycerides.
3.3.2 Lipid-related Indicators
The IVW method was used to calculate the values, which were then utilized to evaluate the overall causal effect of the three lipid-related indicators on stroke (Figure 2). HDL-C has a protective effect against stroke [0.94, (0.97-0.97)], and there was slight heterogeneity in the results (I2 = 58.9%, p=0.045). The heterogeneity was possibly due to the fact that HDL-C data was derived from five different populations, necessitating the use of a random-effects model. Elevated levels of LDL-C might correlate with an increased likelihood of stroke [1.08(1.05-1.12)], with slight heterogeneity in the results (I2 = 51.7%, p = 0.082), possibly due to the inclusion of LDL-C data from five different populations, thus requiring a random-effects model. There was no evidence of a causal relationship between TGs and stroke [1.06 (0.99-1.13)].
3.3.3 Blood pressure-related indicators
Genetic susceptibility to two blood pressure-related indices correlated with a heightened likelihood of developing stroke (Figure 3). Specifically, DBP [1.04 (1.03 -1.05)], SBP [1.03 (1.03 -1.03)], and hypertension [2.25(0.49-10.40)]. There was heterogeneity in the statistical results between DBP(I2 = 66%), SBP(I2 = 0%), hypertension(I2 = 99.7%) and stroke. The heterogeneity in the results was attributed to the use of different populations and strata as exposure factors. All analyses were conducted using a random-effects model.
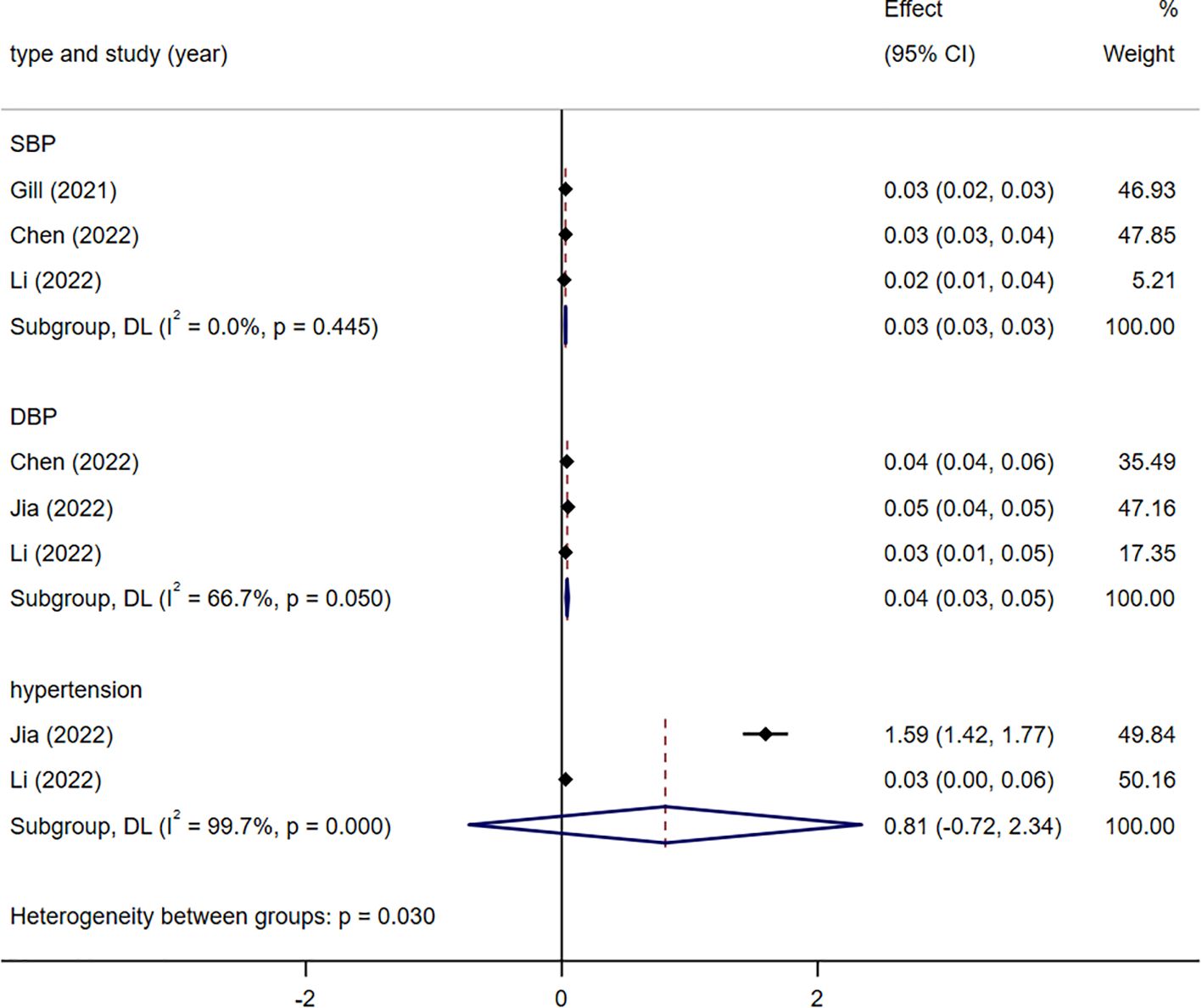
Figure 3. Meta-analysis results of the association between genetic liability to blood pressure-related indicators and risk of stroke. SBP, systolic blood pressure; DBP, diastolic blood pressure.
3.3.4 Renal function related indicators
Values obtained using the IVW method were used to assess the overall causal impact of two renal function-related indicators on stroke: eGFR [0.92 (0.87-0.98)] and CKD [1.07 (1.03-1.10)]. The results suggest a causal effect for both indicators. There was no heterogeneity (I2 = 0%; I2 = 0%) in the statistical results for the onset of stroke using fixed-effects models (Figure 4, Supplementary Figure 1).
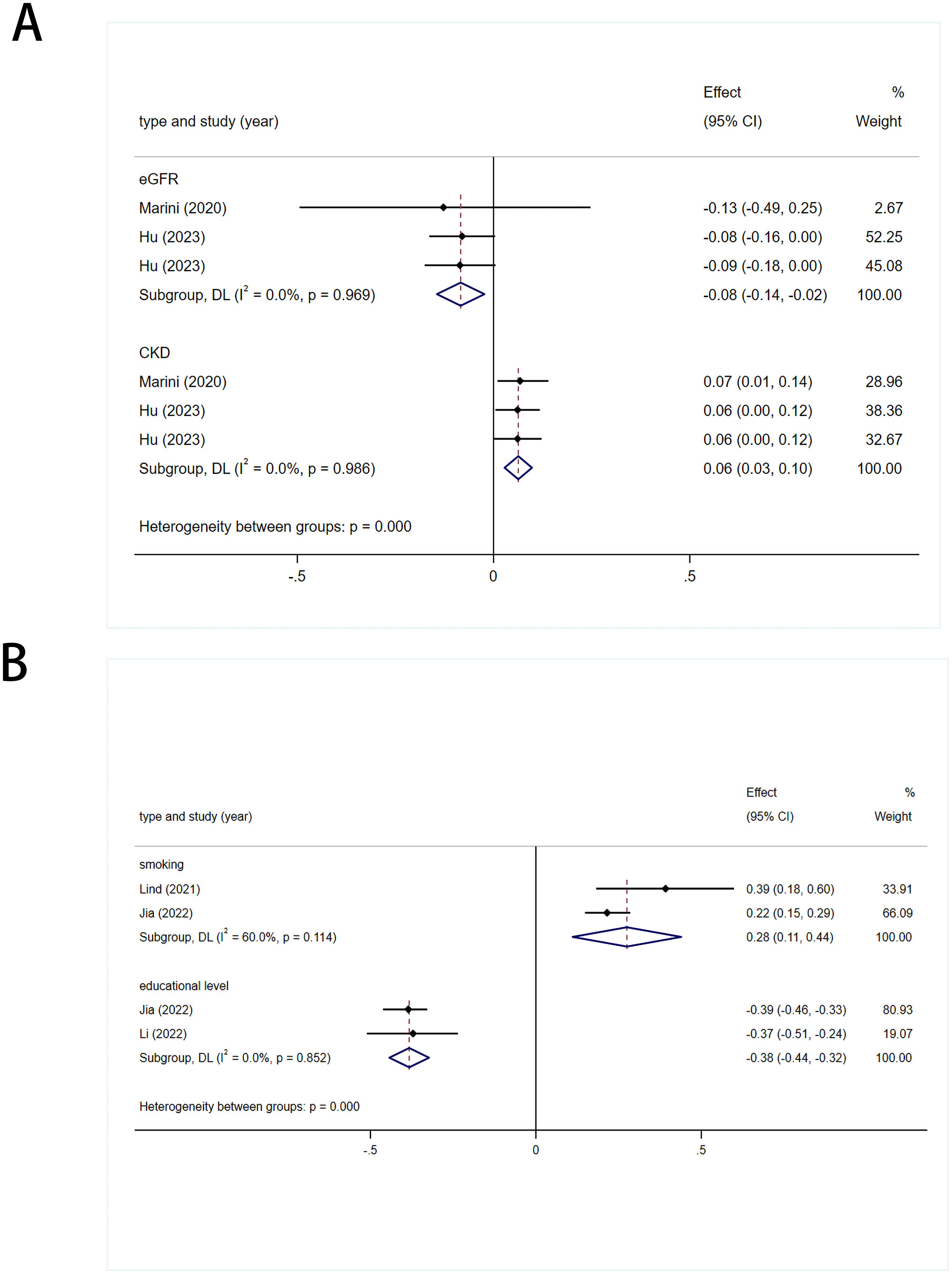
Figure 4. (A) Meta analysis results of the association between genetic liability to renal function related indicators and risk of stroke. eGFR, estimated glomerular filtration rate; CKD, chronic kidney disease. (B) Meta analysis results of the association between genetic liability to living environment related indicators and risk of stroke.
3.3.5 Living environment-related indicators
A causal link was established between stroke and smoking [1.32 (1.12-1.55)], with slight heterogeneity in the results (I2 = 60%, p= 0.114), which was attributed to different populations. Therefore, a random-effects model was used. Educational level had a protective effect against stroke [0.68 (0.64-0.72)]. There was no heterogeneity in the results (I2 = 0%, p= 0.852), so a fixed-effects model was used (Figure 4).
3.3.6 Subgroup analoysis of indicators related to ischemic stroke
The two indicators associated with IS are T2DM [1.10 (1.04-1.15)], DBP [1.04 (1.02-1.06)]. They all exhibit heterogeneity (I2 = 72.2%, I2 = 71.8%), attributed to differences in populations and stratification (Figure 5).
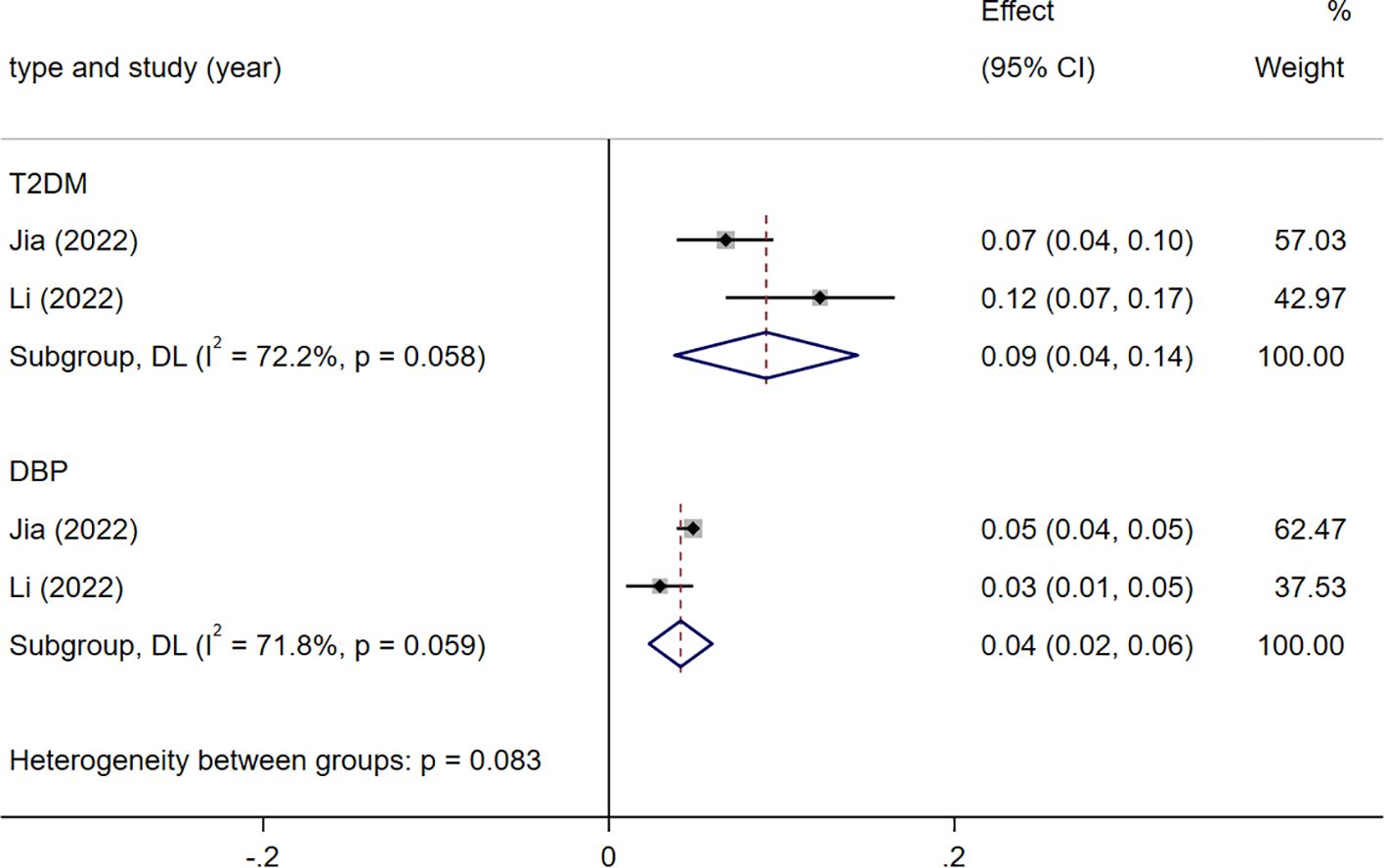
Figure 5. Meta analysis results of indicators related to ischemic stroke(IS). T2DM, type 2 diabetes mellitus; DBP, diastolic blood pressure.
4 Discussion
This review is a meta-analysis of published MR findings related to stroke. Genetic evidence suggests that T2DM, BMI, LDL-C, SBP, DBP, CKD, and smoking contribute to a higher likelihood of stroke, while HDL-C, eGFR, and educational level are protective factors against stroke. Conversely, no causal link was found between WHR, TGs, and stroke. In subgroup analyses, T2DM and DBP showed a correlation with a higher risk of IS.
4.1 Obesity related indicators
Obesity markedly elevates the likelihood of developing cardiovascular disease by inducing metabolic syndrome, which involves insulin resistance, elevated blood glucose levels, substantial body fat accumulation, irregular cholesterol profiles, and hypertension (40, 41). Specifically, in visceral obesity, the combined effects of adipocyte hypertrophy and proliferation lead to an elevated release of pro-inflammatory cytokines (42), exacerbating the systemic and vascular inflammatory response (43–45). Further supporting this, our meta-analysis revealed that obesity-related indicators, such as BMI and T2DM, are risk factors for stroke, consistent with experimental findings. Notably, a higher BMI was correlated with a heightened overall risk of stroke, with combined RR values of 1.25 (95% CI 1.16-1.34, I2 = 84.8%, p = 0.00) and 1.47 (95% CI 1.02-2.11, I2 = 99.4%, p = 0.04) (46–48). Moreover, T2DM was found to be causal for stroke in Europeans (49) (95% CI 1.06-1.09). While studies have shown that WHR is a risk factor for stroke (50–52), intriguingly, our meta-analysis indicated no direct causal relationship between WHR and stroke. This might be due to gender differences in how visceral fat relates to calcified atherosclerosis (53–57). The differing findings between our study and Riaz H et al. may be due to differences in study populations and genetic instruments used (58). Our analysis includes a broader population and more recent studies, which may provide updated data. Methodological variations, such as inclusion criteria and statistical approaches, could also contribute to the discrepancies. Therefore, we propose that for a more comprehensive anthropometric assessment of cardiovascular disease(CVD) risk, a combination of measurements including WHR, WC, and Waist-to-Height Ratio (WHtR) should be utilized (59–61).
4.2 Lipid-related indicators
According to our systematic meta-analysis and comprehensive observational studies, elevated levels of HDL-C are significantly associated with the prevention of IS. Concurrently, LDL-C has been identified as the most useful biomarker for predicting the risk of stroke (62–65). HDL-C facilitates the uptake of cholesterol from peripheral tissues through the reverse cholesterol transport (RCT) pathway. It influences macrophage activity and function, triggers local aggregation of pro-inflammatory cells (66–68), and its antioxidant properties prevent the oxidation of LDL into ox-LDL, thus inhibiting the process of atherosclerosis formation (69). However, our meta-analysis indicates no definitive evidence supporting a causal relationship between TGs and stroke. Research indicates arteriosclerosis-inducing dyslipidemia is attributed to other lipid components (70, 71), notably triglyceride-rich lipoproteins (TRLs) and remnant cholesterol (72), which are crucial in atherosclerotic processes (70, 73). Therefore, further investigation into the collective influence of TGs, TRLs, and remnant cholesterol on atherosclerotic cardiovascular disease (ASCVD) is warranted.
4.3 Blood pressure-related indicators
Studies have confirmed that SBP, DBP, and hypertension are risk factors for stroke, a finding supported by our meta-analysis (74–77). In endothelial cells, a deficiency in Piezo1 can impair flow-mediated vasodilation and elevate SBP (78). Additionally, endothelial damage (79), proliferation of vascular smooth muscle cells (VSMCs) (80, 81), and infiltration of immune cells (82), may induce hypertension (83). Reduced expression of transmembrane member 16A (TMEM16A) may promote cellular proliferation and brain vascular remodeling induced by hypertension (84). Furthermore, the China Stroke Primary Prevention Trial (CSPPT) demonstrated that the risk of stroke was lowest in patients with an average SBP of 120‐130 mm Hg, increasing in those with SBP <120 mm Hg and SBP 130-140 mmHg (85). Therefore, we suggest enhancing awareness and improving treatment compliance for hypertension to effectively prevent stroke.
4.4 Renal function related indicators
Our meta-analysis and systematic review indicate CKD as a significant risk factor for stroke (86–89). This is further supported by studies demonstrating CKD’s independent impact on the risk of stroke, especially when characterized by reduced eGFR (34, 89). Moreover, eGFR is crucial for assessing renal function, with levels < 15, 15-29, and 30-44 mL/min/1.73m2 closely linked to increased likelihoods of adverse clinical outcomes in stroke patients (90–93). However, research on the categorization of eGFR in relation to stroke prognosis (90). Therefore, our study emphasizes the importance of monitoring renal function in these patients (93). We advocate for further research to investigate the temporal relationship between eGFR and stroke over time, and we recommend eGFR as the preferred marker for assessing stroke risk.
4.5 Living environment-related indicators
Previous observational studies, along with our meta-analysis, indicate that smoking and lower educational levels increase the risk of stroke (74, 94, 95). Delgado et al. discovered elevated levels of soluble Intercellular Adhesion Molecule-1 (sICAM-1), soluble Vascular Cell Adhesion Molecule-1 (sVCAM-1), as well as sE-selectin, sP-selectin, and sL-selectin in smokers. These elements are produced by both endothelial cells and leukocytes (96). Consequently, we believe that smoking cessation should be a primary intervention. Additionally, since higher educational attainment is linked to a decreased risk of stroke (97, 98), we recommend improving the overall education and cultural literacy of the general population.
4.6 Indicators related to ischemic stroke
Subgroup analysis of IS indicated that T2DM, DBP significantly elevate IS risk, consistent with clinical observations. However, our analysis did not find evidence of a causal relationship between SBP and IS, this result we view with caution. Considering clinical studies affirming the association of IS with SBP (74), we still advise IS patients to diligently monitor both SBP and DBP, engage in moderate exercise, and actively manage blood glucose and BP. Although limited clinical studies exist in this area, some research does support a causal relationship between BP and SV-Stroke (36, 99). Therefore, we recommend observational studies analyzing risk factors for stroke subtypes (large artery, cardioembolic, SV-Stroke) to further understand these associations.
5 Clinical implications and future research
Given the heightened risk of stroke associated with obesity, abnormal BP, and smoking, it is crucial to prioritize the promotion of healthy dietary habits, regular physical activity, and stress reduction. Additionally, the implementation of smoking cessation programs is essential for primary prevention and non-pharmacological interventions. Enhancing educational and literacy levels is also key in reducing the occurrence of strokes. While our findings show no clear association between WHR and stroke, we remain skeptical of these results. Therefore, it is still recommended to maintain a healthy body weight and closely monitor metrics such as WHR, WC, and WHtR.
This paper summarizes current MR research on risk factors for stroke but identifies several issues that need further study. Specifically, it emphasizes the importance of incorporating novel SNPs as IVs in stroke etiology research through MR studies. This method leverages recent genetic markers to more precisely determine the influence of diverse risk factors on stroke. Further research should expand to encompass diverse cohorts, especially those exposed to different environmental factors, for a more comprehensive understanding of stroke risk factors. The MR method is crucial in providing new insights into epidemiological studies and elucidating complex diseases such as stroke, including their pathophysiology and pharmacological treatments. Future efforts should focus on integrating MR into clinical settings to improve treatment protocols and reduce medication side effects. This advancement could significantly enhance stroke prevention and therapy.
This meta-analysis has several limitations. Firstly, the limited number of existing MR studies on stroke restricts our ability to assess publication bias through funnel plot symmetry analysis and the application of Egger’s and Begg’s tests. Additionally, we were unable to perform subgroup analyses based on region, age, and sex, limiting our exploration of the potential effects of these variables on the consolidated results. Secondly, the significant heterogeneity observed across studies necessitates careful interpretation of the results. This heterogeneity is somewhat expected given the variations in study methods, participant characteristics, and locations. In summary, although MR methods offer advantages over traditional meta-analysis and provide strong evidence linking stroke with its risk factors, they still have limitations. MR studies may be affected by measurement errors in exposure and outcomes, as well as limited ability to capture longitudinal causal relationships.
6 Conclusion
In conclusion, risk factors for stroke include obesity, dyslipidemia, abnormal BP, CKD, and smoking. Conversely, HDL-C, eGFR, and higher levels of education serve as protective factors against stroke. Therefore, the likelihood of stroke can be significantly reduced by quitting smoking, maintaining a healthy body weight, addressing CKD treatment, and improving educational attainment, particularly in individuals predisposed to stroke, such as hereditary susceptibility.
Data availability statement
The original contributions presented in the study are included in the article/Supplementary Material. Further inquiries can be directed to the corresponding author.
Author contributions
YT: Writing – original draft, Writing – review & editing. XT: Data curation, Methodology, Writing – review & editing. YL: Formal analysis, Methodology, Writing – review & editing. SL: Writing – review & editing, Conceptualization.
Funding
The author(s) declare that no financial support was received for the research, authorship, and/or publication of this article.
Acknowledgments
We thank to YT, XT, YL, and SL for their contribution to this article.
Conflict of interest
The authors declare that the research was conducted in the absence of any commercial or financial relationships that could be construed as a potential conflict of interest.
Publisher’s note
All claims expressed in this article are solely those of the authors and do not necessarily represent those of their affiliated organizations, or those of the publisher, the editors and the reviewers. Any product that may be evaluated in this article, or claim that may be made by its manufacturer, is not guaranteed or endorsed by the publisher.
Supplementary material
The Supplementary Material for this article can be found online at: https://www.frontiersin.org/articles/10.3389/fendo.2024.1379516/full#supplementary-material
Supplementary Figure 1 | (A) Meta-analysis results of the association between genetic liability to chronic kidney disease (CKD) and risk of stroke. CKD: chronic kidney disease; IVW: inverse variance weighted, MR-Egger: MR-Egger regression; simple mode; weighted mode; WME: weighted median estimator. (B) Meta-analysis results of the association between genetic liability to estimated glomerular filtration rate (eGFR) and risk of stroke. CKD: chronic kidney disease; IVW: inverse variance weighted, MR-Egger: MR-Egger regression; simple mode; weighted mode; WME: weighted median estimator.
References
2. GBD 2019 Stroke Collaborators. Global, regional, and national burden of stroke and its risk factors, 1990-2019: a systematic analysis for the Global Burden of Disease Study 2019. Lancet Neurol. (2021) 20:795–820. doi: 10.1016/S1474-4422(21)00252-0
3. Bonkhoff AK, Schirmer MD, Bretzner M, Hong S, Regenhardt RW, Brudfors M, et al. Outcome after acute ischemic stroke is linked to sex-specific lesion patterns. Nat Commun. (2021) 12:3289. doi: 10.1038/s41467-021-23492-3
4. Ma Q, Li R, Wang L, Yin P, Wang Y, Yan C, et al. Temporal trend and attributable risk factors of stroke burden in China, 1990-2019: an analysis for the Global Burden of Disease Study 2019. Lancet Public Health. (2021) 6:e897–906. doi: 10.1016/S2468-2667(21)00228-0
5. Fan J, Li X, Yu X, Liu Z, Jiang Y, Fang Y, et al. Global burden, risk factor analysis, and prediction study of ischemic stroke, 1990-2030. Neurology. (2023) 101:e137–50. doi: 10.1212/WNL.0000000000207387
6. Krishnamurthi RV, Ikeda T, Feigin VL. Global, regional and country-specific burden of ischaemic stroke, intracerebral haemorrhage and subarachnoid haemorrhage: A systematic analysis of the global burden of disease study 2017. Neuroepidemiology. (2020) 54:171–9. doi: 10.1159/000506396
7. Pandian JD, Padma MV Srivastava, Aaron S, Ranawaka UK, Venketasubramanian N, Sebastian IA, et al. The burden, risk factors and unique etiologies of stroke in South-East Asia Region (SEAR). Lancet Regional Health Southeast Asia. (2023) 17:100290. doi: 10.1016/j.lansea.2023.100290
8. Pandian JD, Kalkonde Y, Sebastian IA, Felix C, Urimubenshi G, Bosch J. Stroke systems of care in low-income and middle-income countries: challenges and opportunities. Lancet. (2020) 396:1443–51. doi: 10.1016/S0140-6736(20)31374-X
9. Owolabi MO, Thrift AG, Martins S, Johnson W, Pandian J, Abd-Allah F, et al. The state of stroke services across the globe: Report of World Stroke Organization-World Health Organization surveys. Int J Stroke. (2021) 16:889–901. doi: 10.1177/17474930211019568
10. Kayola G, Mataa MM, Asukile M, Chishimba L, Chomba M, Mortel D, et al. Stroke rehabilitation in low- and middle-income countries: challenges and opportunities. Am J Phys Med Rehabil. (2023) 102:S24–32. doi: 10.1097/PHM.0000000000002128
11. Gerstl JVE, Blitz SE, Qu QR, Yearley AG, Lassarén P, Lindberg R, et al. Global, regional, and national economic consequences of stroke. Stroke. (2023) 54:2380–9. doi: 10.1161/STROKEAHA.123.043131
12. Yao M, Ren Y, Jia Y, Xu J, Wang Y, Zou K, et al. Projected burden of stroke in China through 2050. Chin Med Journal/Chinese Med J. (2022) 136:1598–605. doi: 10.1097/CM9.0000000000002060
13. Tsao CW, Aday AW, Almarzooq ZI, Anderson CAM, Arora P, Avery CL, et al. Heart disease and stroke statistics-2023 update: A report from the american heart association. Circulation. (2023) 147:e93–e621. doi: 10.1161/CIR.0000000000001123
14. Potter TBH, Tannous J, Vahidy FS. A contemporary review of epidemiology, risk factors, etiology, and outcomes of premature stroke. Curr Atheroscl Rep. (2022) 24:939–48. doi: 10.1007/s11883-022-01067-x
15. Harshfield EL, Georgakis MK, Malik R, Dichgans M, Markus HS. Modifiable lifestyle factors and risk of stroke: A mendelian randomization analysis. Stroke. (2021) 52:931–6. doi: 10.1161/STROKEAHA.120.031710
16. Blüher M. Obesity: global epidemiology and pathogenesis. Nat Rev Endocrinol. (2019) 15:288–98. doi: 10.1038/s41574-019-0176-8
17. Haidar A, Horwich T. Obesity, cardiorespiratory fitness, and cardiovascular disease. Curr Cardiol Rep. (2023) 25:1565–71. doi: 10.1007/s11886-023-01975-7
18. Wyld M, Webster AC. Chronic kidney disease is a risk factor for stroke. J Stroke Cerebrovascular Dis. (2021) 30:105730. doi: 10.1016/j.jstrokecerebrovasdis.2021.105730
19. Boehm FJ, Zhou X. Statistical methods for Mendelian randomization in genome-wide association studies: A review. Comput Struct Biotechnol J. (2022) 20:2338–51. doi: 10.1016/j.csbj.2022.05.015
20. Yang J, Ferreira T, Morris AP, Medland SE, Genetic Investigation of ANthropometric Traits (GIANT) Consortium, collabDIAbetes Genetics Replication And Meta-analysis (DIAGRAM) Consortium, et al. Conditional and joint multiple-SNP analysis of GWAS summary statistics identifies additional variants influencing complex traits. Nat Genet. (2012) 44:369–375, S1-3. doi: 10.1038/ng.2213
21. Amarenco P, Bogousslavsky J, Caplan LR, Donnan GA, Hennerici MG. Classification of stroke subtypes. Cerebrovascular Dis. (2009) 27:493–501. doi: 10.1159/000210432
22. Sacco RL, Kasner SE, Broderick JP, Caplan LR, Connors JJ, Culebras A, et al. An updated definition of stroke for the 21st century. Stroke. (2013) 44:2064–89. doi: 10.1161/STR.0b013e318296aeca
23. Page MJ, Moher D, Bossuyt PM, Boutron I, Hoffmann TC, Mulrow CD, et al. PRISMA 2020 explanation and elaboration: updated guidance and exemplars for reporting systematic reviews. BMJ (Clinical Res ed.). (2021) 372:n160. doi: 10.1136/bmj.n160
24. Skrivankova VW, Richmond RC, Woolf BAR, Yarmolinsky J, Davies NM, Swanson SA, et al. Strengthening the reporting of observational studies in epidemiology using mendelian randomization: the STROBE-MR statement. JAMA. (2021) 326:1614–21. doi: 10.1001/jama.2021.18236
25. Boef AGC, Dekkers OM, le Cessie S. Mendelian randomization studies: a review of the approaches used and the quality of reporting. Int J Epidemiol. (2015) 44:496–511. doi: 10.1093/ije/dyv071
26. Davies NM, Holmes MV, Davey Smith G. Reading Mendelian randomisation studies: a guide, glossary, and checklist for clinicians. BMJ (Clinical Res ed.). (2018) 362:k601. doi: 10.1136/bmj.k601
27. Higgins JPT, Thompson SG, Deeks JJ, Altman DG. Measuring inconsistency in meta-analyses. BMJ (Clinical Res ed.). (2003) 327:557–60. doi: 10.1136/bmj.327.7414.557
28. DerSimonian R, Laird N. Meta-analysis in clinical trials. Controlled Clin Trials. (1986) 7:177–88. doi: 10.1016/0197-2456(86)90046-2
29. Chen K, Zhuang Z, Shao C, et al. Roles of cardiometabolic factors in mediating the causal effect of type 2 diabetes on cardiovascular diseases: A two-step, two-sample multivariable mendelian randomization study. Front Cardiovasc Med. (2022) 9:813208. doi: 10.3389/fcvm.2022.813208
30. Ciofani JL, Han D, Allahwala UK, Woolf B, Gill D, Bhindi R. Lipids, blood pressure, and diabetes mellitus on risk of cardiovascular diseases in east asians: A mendelian randomization study. Am J Cardiol. (2023) 205:329–37. doi: 10.1016/j.amjcard.2023.08.007
31. Georgakis MK, Malik R, Anderson CD, Parhofer KG, Hopewell JC, Dichgans M. Genetic determinants of blood lipids and cerebral small vessel disease: role of high-density lipoprotein cholesterol. Brain. (2020) 143:597–610. doi: 10.1093/brain/awz413
32. Gill D, Zuber V, Dawson J, Pearson-Stuttard J, Carter AR, Sanderson E, et al. Risk factors mediating the effect of body mass index and waist-to-hip ratio on cardiovascular outcomes: Mendelian randomization analysis. Int J Obes. (2021) 45:1428–38. doi: 10.1038/s41366-021-00807-4
33. Holmes MV, Lange LA, Palmer T, Lanktree MB, North KE, Almoguera B, et al. Causal effects of body mass index on cardiometabolic traits and events: A mendelian randomization analysis. Am J Hum Genet. (2014) 94:198–208. doi: 10.1016/j.ajhg.2013.12.014
34. Hu C, Li Y, Qian Y, Wu Z, Hu B, Peng Z. Kidney function and cardiovascular diseases: a large-scale observational and Mendelian randomization study. Front Immunol. (2023) 14:1190938. doi: 10.3389/fimmu.2023.1190938
35. Jia Y, Wang R, Guo D, Sun L, Shi M, Zhang K, et al. Contribution of metabolic risk factors and lifestyle behaviors to cardiovascular disease: A mendelian randomization study. Nutrition Metab Cardiovasc Dis. (2022) 32:1972–81. doi: 10.1016/j.numecd.2022.04.019
36. Li HQ, Feng YW, Yang YX, Leng XY, Zhang PC, Chen SD, et al. Causal relations between exposome and stroke: A mendelian randomization study. J Stroke. (2022) 24:236–44. doi: 10.5853/jos.2021.01340
37. Lind L, Ingelsson M, Sundstrom J, Ärnlöv J. Impact of risk factors for major cardiovascular diseases: a comparison of life-time observational and Mendelian randomisation findings. Open Heart. (2021) 8:e001735. doi: 10.1136/openhrt-2021-001735
38. Marini S, Georgakis MK, Chung J, Henry JQA, Dichgans M, Rosand J, et al. Genetic overlap and causal inferences between kidney function and cerebrovascular disease. Neurology. (2020) 94:e2581–91. doi: 10.1212/WNL.0000000000009642
39. Mutie PM, Pomares-Milan H, Atabaki-Pasdar N, Coral D, Fitipaldi H, Tsereteli N, et al. Investigating the causal relationships between excess adiposity and cardiometabolic health in men and women[. Diabetologia. (2023) 66:321–35. doi: 10.1007/s00125-022-05811-5
40. Powell-Wiley TM, Poirier P, Burke LE, Després JP, Gordon-Larsen P, Lavie CJ, et al. Obesity and cardiovascular disease: A scientific statement from the american heart association. Circulation. (2021) 143:e984–e1010. doi: 10.1161/CIR.0000000000000973
41. Katta N, Loethen T, Lavie CJ, Alpert MA. Obesity and coronary heart disease: epidemiology, pathology, and coronary artery imaging. Curr Problems Cardiol. (2021) 46:100655. doi: 10.1016/j.cpcardiol.2020.100655
42. de A Boleti AP, de O Cardoso PH, F Frihling BE, E Silva PS, de Moraes LFRN, Migliolo L. Adipose tissue, systematic inflammation, and neurodegenerative diseases. Neural Regeneration Res. (2023) 18:38–46. doi: 10.4103/1673-5374.343891
43. Neeland IJ, Ross R, Després JP, Matsuzawa Y, Yamashita S, Shai I, et al. Visceral and ectopic fat, atherosclerosis, and cardiometabolic disease: a position statement. Lancet Diabetes Endocrinol. (2019) 7:715–25. doi: 10.1016/S2213-8587(19)30084-1
44. Mallick R, Basak S, Das RK, Banerjee A, Paul S, Pathak S, et al. Fatty acids and their proteins in adipose tissue inflammation. Cell Biochem Biophysics. (2023) 82:35–51. doi: 10.1007/s12013-023-01185-6
45. Pestel J, Blangero F, Watson J, Pirola L, Eljaafari A. Adipokines in obesity and metabolic-related-diseases. Biochimie. (2023) 212:48–59. doi: 10.1016/j.biochi.2023.04.008
46. Wang X, Huang Y, Chen Y, Yang T, Su W, Chen X, et al. The relationship between body mass index and stroke: a systemic review and meta-analysis. J Neurol. (2022) 269:6279–89. doi: 10.1007/s00415-022-11318-1
47. Kim MS, Kim WJ, Khera AV, Kim JY, Yon DK, Lee SW, et al. Association between adiposity and cardiovascular outcomes: an umbrella review and meta-analysis of observational and Mendelian randomization studies. Eur Heart J. (2021) 42:3388–403. doi: 10.1093/eurheartj/ehab454
48. Shiozawa M, Kaneko H, Itoh H, Morita K, Okada A, Matsuoka S, et al. Association of body mass index with ischemic and hemorrhagic stroke. Nutrients. (2021) 13:2343. doi: 10.3390/nu13072343
49. Zhang W, Zhang L, Zhu J, Xiao C, Cui H, Yang C, et al. Additional evidence for the relationship between type 2 diabetes and stroke through observational and genetic analyses. Diabetes. (2023) 72:1671–81. doi: 10.2337/db22-0954
50. Onwuakagba IU, Okoye EC, Kanu FC, Kalu CM, Akaeme DC, Obaji OC, et al. Population-based stroke risk profile from a West-African community. eNeurologicalSci. (2023) 33:100483. doi: 10.1016/j.ensci.2023.100483
51. Haberka M, Kubicius A, Starzak M, Partyka M, Gąsior Z. Adiposity, fat depots and the prediction of stroke. Cardiol J. (2023) 30:810–6. doi: 10.5603/CJ.a2021.0134
52. Pillay P, Lewington S, Taylor H, Lacey B, Carter J. Adiposity, body fat distribution, and risk of major stroke types among adults in the United Kingdom. JAMA network Open. (2022) 5:e2246613. doi: 10.1001/jamanetworkopen.2022.46613
53. van Dam-Nolen DHK, van Egmond NCM, Koudstaal PJ, van der Lugt A, Bos D. Sex differences in carotid atherosclerosis: A systematic review and meta-analysis. Stroke. (2023) 54:315–26. doi: 10.1161/STROKEAHA.122.041046
54. Lam CSP. How to incorporate sex and gender into the design of cardiovascular clinical trials. Circulation. (2022) 145:499–501. doi: 10.1161/CIRCULATIONAHA.121.058771
55. Scovelle AJ, Milner A, Beauchamp A, Byrnes J, Norton R, Woodward M, et al. The importance of considering sex and gender in cardiovascular research. Heart Lung Circ. (2020) 29:e7–8. doi: 10.1016/j.hlc.2019.08.011
56. Gauci S, Cartledge S, Redfern J, Gallagher R, Huxley R, Lee CMY, et al. Biology, bias, or both? The contribution of sex and gender to the disparity in cardiovascular outcomes between women and men. Curr Atheroscl Rep. (2022) 24:701–8. doi: 10.1007/s11883-022-01046-2
57. Suman S, Pravalika J, Manjula P, Farooq U. Gender and CVD- does it really matters? Curr Problems Cardiol. (2023) 48:101604. doi: 10.1016/j.cpcardiol.2023.101604
58. Riaz H, Khan MS, Siddiqi TJ, Usman MS, Shah N, Goyal A, et al. Association between obesity and cardiovascular outcomes: A systematic review and meta-analysis of mendelian randomization studies. JAMA Netw Open. (2018) 1:e183788. doi: 10.1001/jamanetworkopen.2018.3788
59. Ke JF, Wang JW, Lu JX, Zhang ZH, Liu Y, Li LX, et al. Waist-to-height ratio has a stronger association with cardiovascular risks than waist circumference, waist-hip ratio and body mass index in type 2 diabetes. Diabetes Res Clin Pract. (2022) 183:109151. doi: 10.1016/j.diabres.2021.109151
60. Zhang S, Fu X, Du Z, Guo X, Li Z, Sun G, et al. Is waist-to-height ratio the best predictive indicator of cardiovascular disease incidence in hypertensive adults? A cohort study. BMC Cardiovasc Disord. (2022) 22:214. doi: 10.1186/s12872-022-02646-1
61. Pasdar Y, Moradi S, Moludi J, Saiedi S, Moradinazar M, Hamzeh B, et al. Waist-to-height ratio is a better discriminator of cardiovascular disease than other anthropometric indicators in Kurdish adults. Sci Rep. (2020) 10:16228. doi: 10.1038/s41598-020-73224-8
62. Su L, Mittal R, Ramgobin D, Jain R, Jain R. Current management guidelines on hyperlipidemia: the silent killer. J Lipids. (2021) 2021:9883352. doi: 10.1155/2021/9883352
63. Chang Y, Eom S, Kim M, Song TJ. Medical management of dyslipidemia for secondary stroke prevention: narrative review. Medicina (Kaunas Lithuania). (2023) 59:776. doi: 10.3390/medicina59040776
64. Su X, Cheng Y, Chang D. Lipid-lowering therapy: Guidelines to precision medicine. Clinica Chimica Acta; Int J Clin Chem. (2021) 514:66–73. doi: 10.1016/j.cca.2020.12.019
65. Crismaru I, Pantea Stoian A, Bratu OG, Gaman MA, Stanescu AMA, Bacalbasa N, et al. Low-density lipoprotein cholesterol lowering treatment: the current approach. Lipids Health Dis. (2020) 19:85. doi: 10.1186/s12944-020-01275-x
66. Cheng W, Rosolowski M, Boettner J, Desch S, Jobs A, Thiele H, et al. High-density lipoprotein cholesterol efflux capacity and incidence of coronary artery disease and cardiovascular mortality: a systematic review and meta-analysis. Lipids Health Dis. (2022) 21:47. doi: 10.1186/s12944-022-01657-3
67. Maguire EM, Pearce SWA, Xiao Q. Foam cell formation: A new target for fighting atherosclerosis and cardiovascular disease. Vasc Pharmacol. (2019) 112:54–71. doi: 10.1016/j.vph.2018.08.002
68. Liu X, Wu J, Tian R, Su S, Deng S, Meng X. Targeting foam cell formation and macrophage polarization in atherosclerosis: The Therapeutic potential of rhubarb. Biomedicine Pharmacotherapy = Biomedecine Pharmacotherapie. (2020) 129:110433. doi: 10.1016/j.biopha.2020.110433
69. Luo M, Zhang Z, Peng Y, Wang S, Peng D. The negative effect of ANGPTL8 on HDL-mediated cholesterol efflux capacity. Cardiovasc Diabetol. (2018) 17:142. doi: 10.1186/s12933-018-0785-x
70. Duran EK, Aday AW, Cook NR, Buring JE, Ridker PM, Pradhan AD. Triglyceride-rich lipoprotein cholesterol, small dense LDL cholesterol, and incident cardiovascular disease. J Am Coll Cardiol. (2020) 75:2122–35. doi: 10.1016/j.jacc.2020.02.059
71. Reiner Ž. Hypertriglyceridaemia and risk of coronary artery disease. Nature Reviews. Cardiology. (2017) 14:401–11. doi: 10.1038/nrcardio.2017.31
72. Li W, Huang Z, Fang W, Wang X, Cai Z, Chen G, et al. Remnant cholesterol variability and incident ischemic stroke in the general population. Stroke. (2022) 53:1934–41. doi: 10.1161/STROKEAHA.121.037756
73. Watts GF, Chan DC. Atherogenic dyslipoproteinemia and management of ASCVD: will new indices untie the gordian knot? J Am Coll Cardiol. (2020) 75:2136–9. doi: 10.1016/j.jacc.2020.03.032
74. Blomstrand A, Blomstrand C, Hakeberg M, Sundh V, Lissner L, Björkelund C. Forty-four-year longitudinal study of stroke incidence and risk factors - the Prospective Population Study of Women in Gothenburg. Scandinavian J Primary Health Care. (2022) 40:139–47. doi: 10.1080/02813432.2022.2057040
75. Wang YX, Wei WB, Xu L, Jonas JB. Prevalence, risk factors and associated ocular diseases of cerebral stroke: the population-based Beijing Eye Study. BMJ Open. (2020) 10:e024646. doi: 10.1136/bmjopen-2018-024646
76. Chen TY, Uppuluri A, Aftab O, Zarbin M, Agi N, Bhagat N. Risk factors for ischemic cerebral stroke in patients with acute amaurosis fugax. Can J Ophthalmol. (2022) 59::50–6:. doi: 10.1016/j.jcjo.2022.10.010. S0008-4182(22)00323-4.
77. Khalfallah M, Elsheikh A, Eissa A, Elnagar B. Prevalence, predictors, and outcomes of resistant hypertension in Egyptian population. Global Heart. (2023) 18:31. doi: 10.5334/gh.1211
78. Xu J, Mathur J, Vessières E, Hammack S, Nonomura K, Favre J, et al. GPR68 senses flow and is essential for vascular physiology. Cell. (2018) 173:762–75.e16. doi: 10.1016/j.cell.2018.03.076
79. Picca A, Mankowski RT, Burman JL, Donisi L, Kim JS, Marzetti E, et al. Mitochondrial quality control mechanisms as molecular targets in cardiac ageing. Nat Rev Cardiol. (2018) 15:543–54. doi: 10.1038/s41569-018-0059-z
80. Kim CW, Pokutta-Paskaleva A, Kumar S, Timmins LH, Morris AD, Kang DW, et al. Disturbed flow promotes arterial stiffening through thrombospondin-1 (TSP-1). Circulation. (2017) 136:1217–32. doi: 10.1161/CIRCULATIONAHA.116.026361
81. Fang Q, Tian M, Wang F, Zhang Z, Du T, Wang W, et al. Amlodipine induces vasodilation via Akt2/Sp1-activated miR-21 in smooth muscle cells. Br J Pharmacol. (2019) 176:2306–20. doi: 10.1111/bph.14679
82. Sumida T, Naito AT, Nomura S, Nakagawa A, Higo T, Hashimoto A, et al. Complement C1q-induced activation of β-catenin signalling causes hypertensive arterial remodelling. Nat Commun. (2015) 6:6241. doi: 10.1038/ncomms7241
83. Turana Y, Tengkawan J, Chia YC, Nathaniel M, Wang J, Sukonthasarn A, et al. Hypertension and stroke in Asia: A comprehensive review from HOPE Asia. J Clin Hypertension. (2020) 23:513–21. doi: 10.1111/jch.14099
84. Lv XF, Zhang YJ, Liu X, Zheng HQ, Liu CZ, Zheng XL, et al. TMEM16A ameliorates vascular remodeling by suppressing autophagy via inhibiting Bcl-2-p62 complex formation. Theranostics. (2020) 10:3980–93. doi: 10.7150/thno.41028
85. Fan F, Yuan Z, Qin X, Li J, Zhang Y, Li Y, et al. Optimal systolic blood pressure levels for primary prevention of stroke in general hypertensive adults: findings from the CSPPT (China stroke primary prevention trial). Hypertension (Dallas Tex.: 1979). (2017) 69:697–704. doi: 10.1161/HYPERTENSIONAHA.116.08499
86. Bruce SS, Parikh NS. Chronic kidney disease and stroke outcomes: beyond serum creatinine. Stroke. (2023) 54:1278–9. doi: 10.1161/STROKEAHA.123.042965
87. Kourtidou C, Tziomalos K. Epidemiology and risk factors for stroke in chronic kidney disease: A narrative review. Biomedicines. (2023) 11:2398. doi: 10.3390/biomedicines11092398
88. Bobot M, Suissa L, Hak JF, Burtey S, Guillet B, Hache G. Kidney disease and stroke: epidemiology and potential mechanisms of susceptibility. Nephrology Dialysis Transplant. (2023) 38:1940–51. doi: 10.1093/ndt/gfad029
89. Kelly DM, Georgakis MK, Franceschini N, Blacker D, Viswanathan A, Anderson CD. Interplay between chronic kidney disease, hypertension, and stroke: insights from a multivariable mendelian randomization analysis. Neurology. (2023) 101:e1960–9. doi: 10.1212/WNL.0000000000207852
90. Vart P, Barlas RS, Bettencourt-Silva JH, Metcalf AK, Bowles KM, Potter JF, et al. Estimated glomerular filtration rate and risk of poor outcomes after stroke. Eur J Neurol. (2019) 26:1455–63. doi: 10.1111/ene.14026
91. Nugroho AW, Arima H, Miyazawa I, Fujii T, Miyamatsu N, Sugimoto Y, et al. The association between glomerular filtration rate estimated on admission and acute stroke outcome: the shiga stroke registry. J Atheroscl Thromb. (2018) 25:570–9. doi: 10.5551/jat.42812
92. Liao J, Xiao F, Yang L, Wei Y, Song C, Li J, et al. Cystatin C–based estimated glomerular filtration rate and risk of stroke in the general population: a prospective cohort study. Clin Kidney J. (2023) 16:2059–71. doi: 10.1093/ckj/sfad188
93. Turin TC, Jun M, James MT, Tonelli M, Coresh J, Manns BJ, et al. Magnitude of rate of change in kidney function and future risk of cardiovascular events. Int J Cardiol. (2016) 202:657–65. doi: 10.1016/j.ijcard.2015.09.090
94. Larsson SC, Burgess S, Michaëlsson K. Smoking and stroke: A mendelian randomization study. Ann Neurol. (2019) 86:468–71. doi: 10.1002/ana.25534
95. Abraham G, Malik R, Yonova-Doing E, Salim A, Wang T, Danesh J, et al. Genomic risk score offers predictive performance comparable to clinical risk factors for ischaemic stroke. Nat Commun. (2019) 10:5819. doi: 10.1038/s41467-019-13848-1
96. Delgado GE, Krämer BK, Siekmeier R, Yazdani B, März W, Leipe J, et al. Influence of smoking and smoking cessation on biomarkers of endothelial function and their association with mortality. Atherosclerosis. (2020) 292:52–9. doi: 10.1016/j.atherosclerosis.2019.11.017
97. Jørgensen TSH, Osler M, Fors S, Nilsson CJ, Meyer A, Modig K. Adult offspring and their socioeconomic resources for development and survival of stroke: A Swedish and Danish nationwide register-based study. Scandinavian J Public Health. (2023) 52:216–24. doi: 10.1177/14034948231152352
98. Zhang B, Sun Q, Lv Y, Sun T, Zhao W, Yan R, et al. Influencing factors for decision-making delay in seeking medical care among acute ischemic stroke patients in rural areas. Patient Educ Couns. (2023) 108:107614. doi: 10.1016/j.pec.2022.107614
99. Georgakis MK, Gill D, Webb AJS, Evangelou E, Elliott P, Sudlow CLM, et al. Genetically determined blood pressure, antihypertensive drug classes, and risk of stroke subtypes. Neurology. (2020) 95:e353–61. doi: 10.1212/WNL.0000000000009814
100. Page MJ, McKenzie JE, Bossuyt PM, Boutron I, Hoffmann TC, Mulrow CD, et al. The PRISMA 2020 statement: an updated guideline for reporting systematic reviews. BMJ (Clinical Res ed.). (2021) 372:n71. doi: 10.1136/bmj.n71
101. Lawlor DA, Wade KH, Borges MC, Palmer T, Hartwig FP, Hemani G, et al. A Mendelian Randomization dictionary: Useful definitions and descriptions for undertaking, understanding and interpreting Mendelian Randomization studies. A Mendelian Randomization Dictionary. (2019). doi: 10.31219/osf.io/6yzs7
Keywords: stroke, ischemic stroke, small vessel stroke, mendelian randomization analysis, genetic epidemiology
Citation: Tian Y, Tang X, Liu Y and Liu SY (2024) Mendelian randomization studies of lifestyle-related risk factors for stroke: a systematic review and meta-analysis. Front. Endocrinol. 15:1379516. doi: 10.3389/fendo.2024.1379516
Received: 31 January 2024; Accepted: 14 October 2024;
Published: 04 November 2024.
Edited by:
Hwi Seung Kim, Chung-Ang University Gwangmyeong Hospital, Republic of KoreaReviewed by:
JoonNyung Heo, Severance Hospital, Republic of KoreaLingli Sun, Peking University, China
Copyright © 2024 Tian, Tang, Liu and Liu. This is an open-access article distributed under the terms of the Creative Commons Attribution License (CC BY). The use, distribution or reproduction in other forums is permitted, provided the original author(s) and the copyright owner(s) are credited and that the original publication in this journal is cited, in accordance with accepted academic practice. No use, distribution or reproduction is permitted which does not comply with these terms.
*Correspondence: Shu Yi Liu, 13693476096@163.com