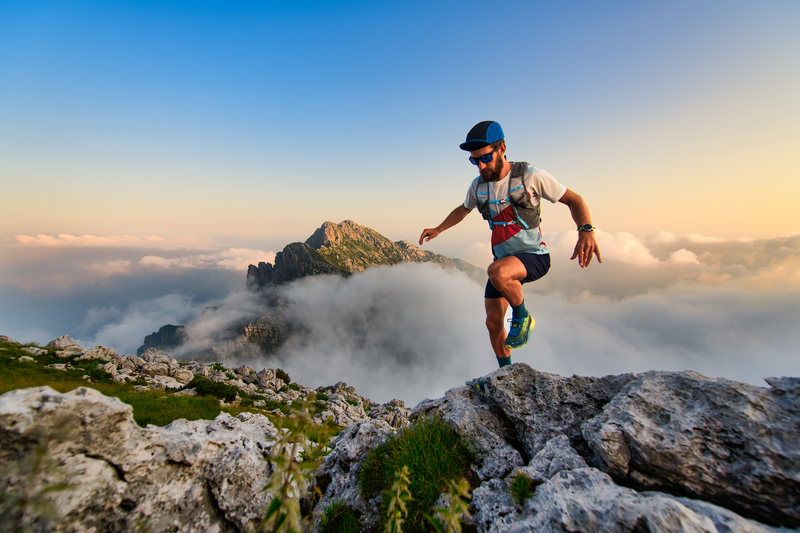
94% of researchers rate our articles as excellent or good
Learn more about the work of our research integrity team to safeguard the quality of each article we publish.
Find out more
ORIGINAL RESEARCH article
Front. Endocrinol. , 10 May 2024
Sec. Endocrinology of Aging
Volume 15 - 2024 | https://doi.org/10.3389/fendo.2024.1372518
Background: Aging has always been considered as a risk factor for neurodegenerative diseases, but there are individual differences and its mechanism is not yet clear. Epigenetics may unveil the relationship between aging and neurodegenerative diseases.
Methods: Our study employed a bidirectional two-sample Mendelian randomization (MR) design to assess the potential causal association between epigenetic aging and neurodegenerative diseases. We utilized publicly available summary datasets from several genome-wide association studies (GWAS). Our investigation focused on multiple measures of epigenetic age as potential exposures and outcomes, while the occurrence of neurodegenerative diseases served as potential exposures and outcomes. Sensitivity analyses confirmed the accuracy of the results.
Results: The results show a significant decrease in risk of Parkinson’s disease with GrimAge (OR = 0.8862, 95% CI 0.7914–0.9924, p = 0.03638). Additionally, we identified that HannumAge was linked to an increased risk of Multiple Sclerosis (OR = 1.0707, 95% CI 1.0056–1.1401, p = 0.03295). Furthermore, we also found that estimated plasminogen activator inhibitor-1(PAI-1) levels demonstrated an increased risk for Alzheimer’s disease (OR = 1.0001, 95% CI 1.0000–1.0002, p = 0.04425). Beyond that, we did not observe any causal associations between epigenetic age and neurodegenerative diseases risk.
Conclusion: The findings firstly provide evidence for causal association of epigenetic aging and neurodegenerative diseases. Exploring neurodegenerative diseases from an epigenetic perspective may contribute to diagnosis, prognosis, and treatment of neurodegenerative diseases.
Neurodegenerative diseases are a class of multifactorial diseases characterized by progressive loss of neuronal structure and function (1). Neurodegenerative diseases can affect cognitive function, mental state and motor function of patients, which lead to constant loss of essence of being. World Health Organization (WHO) reports that neurodegenerative diseases will overtake cancer and become the second leading cause of death by 2040 (2). Although there is no unified standard for the classification of neurodegenerative diseases, Alzheimer’s disease (AD), Parkinson’s disease (PD), and Multiple Sclerosis (MS) are predominantly common neurodegenerative diseases (3).
However, effective treatment and prevention measures of neurodegenerative disorders confront considerable hurdles due to unclear pathogenesis. Among heterogenous risk factors for neurodegeneration diseases, aging has by far the greatest impact (3). Of interest, although the rate of chronological aging is constant across individuals, there are individual variances in risk of neurodegenerative diseases. Even in aging adults with neurodegenerative disorders, disease progression and clinical phenotype still varies. Variability was also observed among family members who possessed identical pathogenic characteristic for neurodegenerative diseases, even among individuals with a strong hereditary background (4). Aging may involve more than a change in chronological age.
The measurement of biological age can be conducted at the epigenetic molecular level, particularly through utilization of DNA methylation (DNAm) age (5). The epigenetic clock was established by integration of DNAm values derived from a wide range of cytosine-phosphate-guanine base pairs (CpGs), which were chosen by supervised machine learning methodologies. Horvath developed the first epigenetic clock based on DNAm, which exhibited robust associations with chronological age. Hannum established a blood-based DNAm age algorithm consisting of 71 CpGs, which effectively recorded alterations in chronological age (6). Subsequently, many other DNA methylation-based ‘clocks’ have been developed. A variety of studies have identified associations between epigenetic aging and mortality, and other characteristics associated to health (7–9).
Some studies found that there is association with disease progression and clinical outcome of neurodegenerative diseases (10–16). Compared with age-matched controls, cortical tissue from patients with AD show modest DNA methylation changes in genes related in beta-amyloid proteins. A systematic review has shown that several epigenetic clocks were used to evaluate progression and pathology of AD and PD (17). Primarily, the Horvath clock was used for evaluating disease progression (18). A recent research of gene co-expression networks in patients with AD revealed the presence of epigenetic clocks-related genes, with enrichment of 50 distinct pathways (15). In general, there is a correlation between rapid DNAm age and a younger age of onset as well as quicker progression in several diseases, including AD (17).
Epigenetic aging may explain heterogeneity and homogeneity of various types of neurodegenerative diseases that will eventually dominate the aging brain. However, it remains unclear how epigenetic aging influences such an association. Thus, developing specific mechanisms between epigenetic aging and neurodegenerative disease is conducive to develop patient-tailored preventions and interventions. Furthermore, it may be useful in differentiating and diagnosing various forms of neurodegenerative disorders.
According to the latest study about polygenic risk for biomarkers of aging, the second-generation epigenetic clocks, GrimAge and PhenoAge, DNAm plasminogen activator inhibitor-1 (PAI1) levels and granulocyte proportion have a strong association with aging (19). A study has also found epigenetic factors are associated with the aging-related cognitive decline (20). They found that cognitive dysfunction had association with older Pheno Age progression and a faster Dunedin PACE (20). Therefore, we propose a hypothesis that these epigenetic genes may reveal specific mechanisms between aging and various kinds of neurodegenerative diseases.
As for causality between exposures and outcomes in diseases, mendelian randomization employs genetic variants as bridge variables to determine whether a risk factor causally influences the occurrence of diseases (21). Research of MR in neurodegenerative diseases is gradually receiving attention. Further studies on these heterogeneities may give insights to a specific mechanism between aging and neurodegenerative diseases, perhaps leading to early prevention, timely diagnosis, and efficient therapy.
Therefore, we conducted an MR study to examine causal effects between epigenetic aging and neurodegenerative diseases, such as AD, MS and PD.
Our study employed a bidirectional two-sample Mendelian randomization (MR) design to assess the potential impact of epigenetic age on neurodegenerative diseases susceptibility. To acquire primary data, we utilized publicly available summary datasets from several genome-wide association studies (GWAS). Our investigation focused on multiple measures of epigenetic age as potential exposures, while the occurrence of neurodegenerative diseases served as the outcome of interest. In order to establish instrumental variables (IVs), we carefully selected specific single-nucleotide polymorphisms (SNPs) that exhibited strong associations with various aspects of epigenetic age. By ensuring adherence to the three key assumptions of the MR framework - (i) the genetic instrumental variables are correlated with the exposure (epigenetic age), (ii) the genetic instrumental variables are independent of potential confounders, and (iii) the genetic instrumental variables solely impact the outcome (neurodegenerative diseases) through the exposure (epigenetic age) - our objective was to examine the bidirectional causal association between epigenetic age and the risk of neurodegenerative diseases (Figure 1).
The study encompassed a diverse range of participants, with 57.3% being female, and the findings provide valuable insights into the methods employed for analyzing biological aging through genome-wide association studies. Age-adjusted DNA methylation-based estimates of HannumAge, Intrinsic Horvath age, PhenoAge, GrimAge, plasminogen activator inhibitor-1 levels were calculated using the Horvath epigenetic age calculator software https://www.ebi.ac.uk/gwas/publications/34187551 (19).
For neurodegenerative diseases, a recent PD GWAS meta-analysis from the International Parkinson’s Disease Genomics Consortium (IPDGC) including 3 previously reported GWAS studies, 13 new datasets, as well as UKB proxy-case data (excluding 23and Me) was used as the PD source (33,674 cases, and 449,056 controls).
For AD, we used the recently published GWAS data, which is an International Genomics of Alzheimer’s Project (IGAP) meta-analysis of stage 1 as AD data in which 63,926 individuals were included (21,982 cases and 41,944 controls) from four consortiums as primary data for analysis. In addition, summary-level data for MS was retrieved from the shared data set by the most recent publication of the International Multiple Sclerosis Genetics Consortium(IMSGC) which included 47,429 MS cases and 68,374 controls. In this GWAS, 233 statistically independent genome-wide significant SNPs were found to be associated with MS susceptibility, explaining about 39% of the genetic predisposition to MS.
In order to identify genetic predictors associated with epigenetic age characteristics, we implemented a stringent quality control procedure. We applied a strict threshold of genome-wide significance (P < 5 × 10 -8) to identify highly significant SNPs that are associated with both epigenetic age and neurodegenerative diseases. To ensure compliance with the assumptions of MR, we conducted a linkage disequilibrium (LD) analysis using data from the 1,000 Genomes Project, focusing on individuals of European ancestry. SNPs that did not meet the criteria (R2 < 0.001, clumping distance = 10,000 kb) were excluded from further analysis. Furthermore, we removed palindromic SNPs due to uncertainties regarding their alignment in the same direction for both the exposure and outcome variables in the neurodegenerative diseases genome-wide association studies. Additionally, SNPs with a minor allele frequency (MAF) below 0.01 were excluded from the analysis. In cases where SNPs associated with the exposure variable were missing in the outcome GWAS dataset, we selected proxy SNPs with a high level of linkage disequilibrium (r2 > 0.80) to ensure comprehensive coverage. To assess the strength of the instrumental variables, we calculated the F statistic using the formula F = R2(n-1-k)/(k*(1-R2)), where K is the number of instrumental variables, n is the sample size, R2 represents the proportion of variance explained by the instrumental variables, and n represents the sample size. An F statistic value below 10 indicates a higher likelihood of weak instrument bias, which highlights the need for cautious interpretation of the findings (Figure 2).
We employed inverse variance weighted (IVW) method as the primary approach for analyzing the MR data. In order to ensure the robustness of our findings, we also conducted several sensitivity analyses using alternative methodologies. These included maximum likelihood, MR-Egger regression, weighted median method, simple mode, and weighted mode method. Although some of these methods did not yield statistically significant results, we considered the findings positive if the IVW method produced significant results (p < 0.05) and the direction of the beta values remained consistent. To assess the impact on neurodegenerative diseases, we calculated odds ratios (OR) along with 95% confidence intervals (CIs), using a significance threshold of p < 0.05. Heterogeneity was evaluated using Cochran’s Q test for the IVW and MR-Egger estimates. To investigate potential pleiotropic bias, we utilized the MR-Egger regression technique. Furthermore, we performed a systematic “leave-one-out” analysis to evaluate the stability of our results, sequentially excluding each single-nucleotide polymorphism (SNP) to assess its influence on the overall findings. Additionally, a reverse MR analysis was performed to examine potential causal effects of neurodegenerative diseases on epigenetic age, following the same protocol as the two-sample MR. All statistical analyses were conducted using the Two Sample MR package (version 0.5.5) in the R software environment (version 4.0.3). These rigorous analytical approaches were employed to ensure the reliability and validity of the study’s outcomes (Figure 2).
To investigate the association between epigenetic age and the risk of neurodegenerative diseases, we conducted an MR analysis involving six specific epigenetic age traits. We ensured the use of robust genetic instruments (p< 5 × 10-8) to establish the independence of these traits (r2 < 0.01) by excluding palindromic single nucleotide polymorphisms (SNPs). The instrumental variables exhibited F-statistics that were all significantly greater than 10, indicating the absence of weak instrument bias. These measures were implemented to ensure the reliability and validity of our findings (Supplementary Table 3).
Using an IVW approach, we observed a significant decrease in the risk of PD with GrimAge (OR = 0.8862, 95% CI 0.7914–0.9924, p = 0.03638) (Figure 3A). Consistently, negative associations between GrimAge and PD risk were also found using other methods such as maximum likelihood, weighted median, simple mode, and weighted mode (Figure 3D, Table 1, Supplementary Table 1).
Figure 3 Causal effect of epigenetic age on neurodegenerative diseases. (A) Forest figure of DNA methylation GrimAge for PD risk. (B) Forest figure of DNA methylation HannumAge for MS risk. (C) Forest figure of plasminogen activator inhibitor-1 for Alzheimer’s disease risk. (D) SNP effect on exposure of GrimAge on Parkinson’s disease. (E) SNP effect on exposure of HannumAge on MS. (F) SNP effect on exposure of plasminogen activator inhibitor-1 on AD.
Additionally, we identified that HannumAge was linked to an increased risk of MS (OR = 1.0707, 95% CI 1.0056–1.1401, p = 0.03295) (Figure 3B). Similarly, the maximum likelihood, MR-Egger, weighted median, simple mode, and weighted mode methods also indicated positive associations between HannumAge and MS (Figure 3E, Table 1).
Furthermore, we also found that estimated plasminogen activator inhibitor-1(PAI-1) levels demonstrated an increased risk for AD (OR = 1.0001, 95% CI 1.0000–1.0002, p = 0.04425) (Figures 3C, 3F). Beyond that, we did not observe any causal associations between epigenetic age and neurodegenerative diseases.
Using an IVW approach, genetically determined AD was found to have causal effect on GrimAge (OR = 0.9057, 95% CI 0.8265–0.9925, p = 0.03386) (Figures 4A, C), while genetically determined MS has a causal effect on HannumAge (OR = 1.0653, 95% CI 1.0031–1.1314, p = 0.03932) (Figures 4B, D, Table 2, Supplementary Table 1).
Figure 4 Causal effect of neurodegenerative diseases on epigenetic age. (A) Forest figure of AD for GrimAge. (B) Forest figure of MS for HannumAge. (C) SNP effect on exposure of AD on GrimAge. (D) SNP effect on exposure of MS on HannumAge.
The estimates of causal effects obtained from multiple analytical methods, including maximum likelihood, MR-Egger regression, weighted median method, simple mode, and weighted model methods, consistently yielded similar results in terms of both magnitude and direction. This consistent pattern enhances the reliability and confidence in our findings. Our analysis did not uncover substantial evidence of horizontal pleiotropy, suggesting that the instrumental variables utilized in the study were not influenced by factors other than the specific exposure of interest. This was supported by p-values greater than 0.05 when applying the MR-Egger regression intercept approach. Furthermore, the assessment of heterogeneity using Cochrane’s Q statistics did not reveal statistically significant differences among the estimates (p > 0.05). This indicates that the genetic variants employed as instruments for epigenetic age did not have significant differential effects on the outcome (Figures 5A–E, Table 3). Additionally, the leave-one-out analysis, where individual variants were systematically excluded, demonstrated the stability of the effect estimates and minimal influence from any single variant (Figures 6A–E). These findings further reinforce the robustness of our results. Taken together, these findings provide consistent and reliable evidence of a causal relationship between epigenetic age and neurodegenerative diseases, while also suggesting the absence of significant confounding factors or outliers affecting the observed effects (Supplementary Table 2).
Figure 5 MR-Egger regression between epigenetic age and neurodegenerative diseases. (A) MR-Egger regression about risk of PD with GrimAge. (B) MR-Egger regression about risk of MS with HannumAge. (C) MR-Egger regression about risk of AD with plasminogen activator inhibitor-1. (D) MR-Egger regression about causal effect of AD on GrimAge. (E) MR-Egger regression about causal effect of MS on HannumAge.
Table 3 Sensitivity analyses of causal association between epigenetic aging and neurodegenerative diseases.
Figure 6 Leave-one-out analysis between epigenetic age and neurodegenerative diseases. (A) Leave-one-out analysis about risk of PD with GrimAge. (B) Leave-one-out analysis about risk of MS with HannumAge. (C) Leave-one-out analysis about risk of AD with plasminogen activator inhibitor-1. (D) Leave-one-out analysis about causal effect of AD on GrimAge. (E) Leave-one-out analysis about causal effect of MS on HannumAge.
Our study is the first to elucidate underlying association between aging and neurodegenerative diseases from an epigenetic aging perspective by bidirectional MR. Although there have been many studies exploring relationship of epigenetic aging on neurodegenerative disease, few studies focused on causal effect between them. The causal relationship between epigenetics and neurodegenerative diseases may contribute to identifying targets for treatment and prevention of neurodegenerative diseases (3). Our study selected PD, AD, and MS as representative neurodegenerative diseases and conducted an MR study in combination with the latest published epigenetic aging biomarkers. Our study involves a PD source (33,674 cases, and 449,056 controls),AD data (21,982 cases and 41,944 controls),and an MS source (47,429 cases and 68,374 controls). We found evidence to support the claim that there is causality between epigenetic aging and neurodegenerative diseases. Epigenetic aging may act as an one of the triggers to determine the incidence and prevalence of neurodegenerative diseases.
It is commonly known that aging is a major hazard for neurodegenerative diseases, a deteriorating brain is an arbiter of neurodegenerative diseases (3). And significant progress has been made in gene discovery and gene therapy for neurodegenerative diseases. However, neurodegenerative diseases may not be simply viewed as results of accelerated aging or gene disorder (3). There is growing evidence that epigenetic age and neurodegenerative diseases are related. Several studies have shown that epigenetic age is accelerated in brains and blood of patients with neurodegenerative diseases, compared to healthy controls (22). One possible mechanism for this relationship is that epigenetic aging may affect the expression of genes that are involved in neuronal function, survival, and repair. For example, epigenetic aging may alter the expression of genes that regulate inflammation (23), oxidative stress (24), synaptic plasticity (25), and neurogenesis (26). Additionally, neurodegenerative diseases may also cause acceleration of epigenetic aging. For example, neurodegenerative diseases may induce DNA damage (27), oxidative stress (28), inflammation (29), and metabolic dysfunction (30), which can affect DNA methylation patterns. However, various calculation methods of epigenetic aging and different kinds of neurodegenerative diseases have both homogeneity and heterogeneity.
As for PD, we observed a negative association between GrimAge and risk of PD. GrimAge is the second of epigenetic clocks, which is calculated based on various biomarkers, such as DNA methylation, telomere length, and blood biochemistry (31). GrimAge is considered to be a more accurate predictor of mortality and health outcomes than chronological age (31). One study has confirmed that GrimAge is associated with grip strength, walking speed, polypharmacy, and all-cause mortality (31). Even though PD is classified as a type of neurodegenerative disorder, the mortality rate is increasing annually (32). The cause of death from PD has long been debatable and elusive, and there are significant individual variances in mortality rates among patients. According to one recent research, late onset age, dementia, cardiac abnormalities and autonomic dysfunction have been viewed as triggers leading to an increase in mortality rate (33). Our results showed a negative associations between GrimAge and PD risk. In neurodegenerative diseases, this may mean that GrimAge has specific predictive ability for mortality of PD. Predicting the mortality rate of PD can contribute to personalized treatment and prevention strategies for PD.
MS is a chronic disease causing demyelinating, neurodegenerative lesions to the central nervous system (34). MS is believed to have an autoimmune disorder in pathology and progressive mechanism, with polygenic and environmental susceptibility factors (35). However, pathology and progressive mechanism of MS cannot be solely attributed to genetic or environmental factors.
From view of the progressive mechanism, the clinical stage of MS is better explained as a continuum that may vary according to the amount of individuals (36). MS has always been categorized by distinct clinical stage—relapsing/remitting(RRMS), secondary/progressive(SPMS), and primary/progressive(PPMS) (36). Personalized progression continuum may be related to a transition from predominantly localized injury to widespread inflammation and neurodegeneration (36, 37).According to recent research, aging mainly affects the progression of MS in conjunction with the immune system and microbiota (38, 39). Deregulation of the immune system caused by aging directly hinders the repair pathways in progressive MS. Like concentrations of the chemokine eotaxin (CCL11) in blood and CSF, which are associated with clinical disability and radiological lesion burden in patients with MS (40). The microbiota and immune system reciprocally affect each another. Aging amplifies the process in which immunoregulatory microbial products diffuse into circulation from the gut through increased intestinal epithelial permeability (40). Similarly, aging is associated with a reduction in fecal concentrations of short chain fatty acids, which might exacerbate the immune system and MS progression. The process of aging is linked to a decrease in levels of short chain fatty acids in feces, perhaps leading to changes in the immune system and worsening the progress of MS (41). Comparing biological changes with the risk of aging-related diseases and mortality by measuring epigenetic clocks has been viewed as promising method. Compared with healthy control participants, one study showed that epigenetics can unveil higher age acceleration in patients with MS (42). But the association of this metric with progression of MS was not clear.
Of interest, we identified that HannumAge was linked to an increased risk of MS. This may support the idea that HannumAge is a specific risk factor for MS in different types of multiple sclerosis. Hannum et al. analyzed DNA methylation patterns from two cohorts’ whole blood data to discover 71 CpG sites that might be used to calculate age, which is called HannumAge (43). HannumAge estimator, the first epigenetic clock, is considered to track aspects of immunosenescence. HannumAge has been described as a biomarker of immune system aging (44). One study found that Hannum is positively correlated with estimated abundance of exhausted, plasmablast cells, and is negatively correlated with naive CD8+ T cell types (45). Since pathogenic B and T cells are prone to enter the central nervous system, interacting B and T cells drive the pathogenesis of MS (46). Genetic burden and environmental factors may contribute to the CNS-infiltrating (46, 47). HannumAge has been found to be associated with sensitivity to variations in environment and lifestyle (48).
Current evidence indicates that MS pathogenesis should integrate underlying risk related to genetic susceptibility with epigenetic age (49). Our result may indicate that HannumAge may affect pathogenesis and progression of MS by regulation of immunity and inflammation, which is consistent with the previous point. Epigenetics emerges as a crucial intermediary factor that bridges the gap between genes and the environment factors. Therefore, Hannum’s age may be a potential research direction for preventing progression and treatment of MS in response to epigenetic aging and environmental factors.
Significantly, we also found that MS can in turn affect acceleration of the Hannum clock. There is currently a lack of research on acceleration of Hannum’s age. According to the present study, possible factors that can accelerate Hannum epigenetic age mainly include smoking, obesity and inflammation. Inflammatory markers in blood were always associated with higher Hannum epigenetic age (50). As mentioned before, progression of MS is gradual and can be divided into three stages(RRMS,SPMS,PPMS). Inflammation plays an important role in progression of MS to varying degrees (51). Therefore, results shows there is mutual causality in a continuous process of onset and progression of MS. Hannum clock may lead to onset of MS through immune system disorders. Afterwards, diffusion of inflammatory reactions leads to an acceleration in the Hannum clock as MS progresses.
According to numerous research works, several age-related diseases are linked to a dysfunctional fibrinolytic system (52). Plasminogen activator inhibitor-1 (PAI-1) is the primary physiological inhibitor of both tissue type and urokinase type plasminogen activators. Therefore, it is the main regulator of plasminogen activation system activators (53). According to recent research, PAI-1 has both direct and indirect effects in the development of AD (54). Directly, accumulation of amyloid beta (Aβ) peptide was considered as the main pathogenesis of AD. Elevated expression of PAI-1 has been shown to interfere with plasmin-mediated clearance and degradation of Aβ, thereby contributing to neurotoxic plaques in AD (55–57). Additionally, inhibition of PAI-1 activity can reduce accumulation of Aβ and affect synaptic function in the brain, which promotes memory in AD model mice (58). A study has shown that Aβ might impair BDNF proteolytic processing through regulation of PAI‐1, which unveils potential mechanisms.
Indirectly, plasminogen activation system activators exhibit multiple functions that are potential risk factors for AD onset and progression, like diabetes, cardiovascular health, and chronic inflammation (52). Additionally, some studies further showed that increased PAI-1 expression may drive astrocyte senescence and that senescent astrocytes can promote neuron apoptosis by secreting PAI-1. In conclusion, there are increasing studies exploring the relationship between PAI-1 and AD (58–60). The present mendelian randomization study, in vitro and in vivo experiments have confirmed that PAI-1 is a biomarker of cellular aging (54, 61). PAI-1 may have a potential effect between AD and aging.
Our study is the first to confirm casual effect between AD and PAI-1 by MR, and we only found that estimated PAI-1 levels demonstrated an increased risk of AD. By contrast, we found no effect of AD on PAI-1. Therefore, our study suggests that PAI-1 may be a pathogenic factor of AD, instead of a result of AD onset. According to the present study, PAI-1 expression increases in plasma and brain of both AD patients and AD model mice (54, 62–64).
There are some kinds of molecules that were proven to be efficient PAI-1 inhibitors both in vitro and in vivo (57, 58, 65–67). However, no PAI-1 inhibitor is presently licensed for use in humans. Our research is consistent with current research results and is the first to further confirm from a genetic perspective.
As significant DNAm-based surrogate markers, PAI-1 were causally influenced by lifestyle factors and had a causal effect on aging and disease outcomes (68–70). One study has shown that lifestyle intervention can reverse insulin-induced vascular dysfunction in parallel with decreased PAI-1 level (68). Another study has shown that exercise ameliorates PAI-1 mediated cardiovascular inflammation in renal insufficiency (71). However, there is currently no research focusing on how lifestyle can influence AD by reversing PAI-1. Our research findings indicate that it may be advisable to explore interventions aimed at reversing PAI-1 expression in order to achieve therapeutic or preventative effects on AD.
Although we found no effect of AD on PAI-1, our results show that AD had a causal effect of on GrimAge. Unlike other epigenetic ages, GrimAge is calculated from a formula that uses the methylation levels of 103 CpG sites, which are regions of DNA where a cytosine nucleotide is followed by a guanine nucleotide (31). GrimAge is also calculated based on various biomarkers, such as DNA methylation (31), telomere length(TL) (72, 73), and blood biochemistry (73–75). Among various triggers that affect GrimAge, DNA methylation measures of aging have offered promise, but their relation to AD has been equivocal. One cohort study showed that candidate CpG sites and regions in peripheral blood were identified as associated with the rate of cognitive decline (75). However, based on existing studies, one systematic review showed that DNA methylation is not associated with risk of dementia (17).
These equivocal findings, together with our results, imply that GrimAge may not be the factor causing AD. More likely, onset and development of AD may cause alterations in DNA methylation, resulting in an acceleration of GrimAge.
TL is a potential indicator of biological aging and age-related consequences (76, 77). Shorter telomeres are associated with higher overall risk of developing AD (78). However, previous literature showed inconsistent findings regarding TL effect on AD. Hägg et al. reported an association between longer TL and cognitive performance (79). Fani et al. found a kind of U-shaped association between TL and risk of AD (80), which means that both shorter TL and longer TL were associated with risk of AD. Blanca et al. did not find a statistically significant association between genetically longer TL and cognitive function (81). Differences between these results may be due to characteristics of cross-sectional and cohort studies. As one of the calculation tools for GrimAge, TL may be related to acceleration of GrimAge caused by AD onset. However, our study did not make specific analysis of causal relationship between TL and AD, further studies are needed to explore whether onset and progression of AD will lead to TL changes.
Besides TL and DNA methylation, GrimAge incorporates plasma protein levels into a composite measure of biological age. Several plasma proteins have association with GrimAge: beta-2-microglobulin (β2M) (82), adrenomedullin (83), cystatin C(Cys C) (84), PAI-1 (54). These proteins not only serve as the basis for calculating biological aging in GrimAge, but are also highly correlated with pathological mechanisms of AD. β2M (85), and Cys C (86) has been verified to be related to deposition of Aβ, the most discussed pathogenesis of AD.
β2M has been viewed as a coaggregation factor with Aβ (85). Increased soluble β2M has been detected in plasma with AD patients (73). Additionally, one study showed that targeting peripheral β2M can effectively decrease deposition of β-amyloid in brain of AD model mice and improve learning and memory abilities (87).
Cystatins are a family of cysteine protease inhibitors, which play a significant role in regulating abnormal accumulation of Aβ in AD (88). Wang et al. have shown that plasma Cys C levels were higher in patients with AD than in healthy subjects (84). And there is correlations between plasma Cys C levels and severity scores in AD patients (84).
Adrenomedullin has also been shown to have diagnostic value for AD (83). In addition, Buerger et al. shows that plasma concentrations of adrenomedullin have predictive value in progression from MCI to clinical AD (89), which may indicate adrenomedullin is a biomarker of AD progression. As we have shown, AD has causal effect on GrimAge, and it is suggested that calculating GrimAge with adrenomedullin may help predict progression of AD.
Changes of GrimAge related proteins in plasma of AD patients may be one of the reasons for GrimAge increase caused by progression of AD. Therefore, calculating GrimAge as a result in future studies may be useful in predicting prognosis and progression of AD.
Additionally, a clinical study found that the GrimAge predictor of human morbidity and mortality showed a 2-year decrease in epigenetic vs. chronological age after intervention (90). These preliminary results confirmed that epigenetic GrimAge aging is reversible, which sheds light on potential epigenetic therapy for AD.
In conclusion, our study is the first to elucidate underlying association between aging and neurodegenerative diseases from an epigenetic aging perspective by using bidirectional MR. We found negative associations between GrimAge and PD, PAI-1 and AD. We also found a casual effect between HannumAge and MS, as well as GrimAge and AD. This suggests that epigenetic age and neurodegenerative diseases may have a bidirectional relationship, where each one influences the other. Therefore, understanding this relationship may help to identify biomarkers for early diagnosis, prognosis, and treatment. It may also reveal novel targets for interventions that can slow down or reverse epigenetic aging and prevent or delay neurodegeneration.
Our study is not free of limitations. From the perspective of clinical significance, neurodegenerative diseases are multifaceted, involving genetic, epigenetic, and environmental factors. Epigenetic clocks, although offering valuable insights, provide but just one piece of the puzzle in understanding the complex mechanisms. Further research is needed to determine whether epigenetic clocks lead to the occurrence of neurodegenerative diseases through interactions with other causes. Methodologically, there are many determining factors for each generation epigenetic clock. Although this study has confirmed correlation between some epigenetic clocks and neurodegenerative diseases in a broad sense, it has not targeted specific determining factors, which also highlights a need for further studies. Types of neurodegenerative diseases are not limited to PD, AD, and MS. Our study did not include all types of neurodegenerative diseases. Further research on the correlation between other types of neurodegenerative diseases and epigenetic aging are conducive to find promising candidate for diagnosis and prognosis.
Cancer and aging are accompanied by changes to epigenetic clocks, including progressive loss of DNA methylation over gene-poor genomic regions (91). Much evidence demonstrated that modifications in genetic and epigenetic characteristics may drive tumor metastasis (92). The presence of intricate inter- and intratumor heterogeneity is a characteristic observed in several cancers, frequently leading to limited efficacy of treatment interventions (93). Epigenetic changes, due to their dynamic and reversible character, have great potential as a target for innovative anticancer treatments. In future research, the study of cancer incidence, metastasis, and treatment resistance through epigenetic perspectives may provide more profound implications. Besides, epigenetics is a crucial factor in the pathogenesis of several metabolic disorders, such as diabetes, obesity, and osteoporosis (94). Enhanced comprehension of epigenetic regulatory systems in metabolic illnesses facilitates a comprehensive understanding of these conditions, consequently enabling the development of innovative therapeutic approaches.
The research subjects included in this study were mostly from the United States and Europe. In subsequent research, it will be necessary to promptly follow up on data updates from multiple races for analysis, in order to obtain more representative conclusions.
Unlike genetics, epigenetic changes are mostly reversible. Therefore, epigenetics has a great potential for clinical application. We provided primary evidence that epigenetic aging might be a potential diagnostic or patient-tailored therapeutic approach for neurodegenerative diseases through MR research, but further extensive research is still required.
The original contributions presented in the study are included in the article/Supplementary Material. Further inquiries can be directed to the corresponding author.
JF: Conceptualization, Methodology, Software, Writing – original draft. QL: Conceptualization, Writing – review & editing. XL: Conceptualization, Data curation, Methodology, Software, Writing – original draft. MG: Conceptualization, Investigation, Software, Writing – review & editing. IL: Investigation, Software, Writing – review & editing. YT: Software, Writing – review & editing. XX: Data curation, Methodology, Writing – review & editing. LS: Data curation, Writing – review & editing. LD: Software, Writing – review & editing. YZ: Methodology, Writing – review & editing. ML: Formal analysis, Project administration, Writing – review & editing. LZ: Funding acquisition, Validation, Writing – review & editing.
The author(s) declare financial support was received for the research, authorship, and/or publication of this article. This work was supported by National Natural Science Foundation of China(No.82174486), San Ming Project Medicine in Shenzhen Nanshan(No. SZSM202103010), Scientific Research Project of Guangdong Provincial(No.20221135).
The authors declare that the research was conducted in the absence of any commercial or financial relationships that could be construed as a potential conflict of interest.
All claims expressed in this article are solely those of the authors and do not necessarily represent those of their affiliated organizations, or those of the publisher, the editors and the reviewers. Any product that may be evaluated in this article, or claim that may be made by its manufacturer, is not guaranteed or endorsed by the publisher.
The Supplementary Material for this article can be found online at: https://www.frontiersin.org/articles/10.3389/fendo.2024.1372518/full#supplementary-material
Supplementary Table 1 | Causal effect between epigenetic age and neurodegenerative diseases.
Supplementary Table 2 | Sensitive analysis_Causal effect between epigenetic age and neurodegenerative diseases.
Supplementary Table 3 | Instrumental variables_Causal effect of epigenetic age on neurodegenerative diseases.
1. Dugger BN, Dickson DW. Pathology of neurodegenerative diseases. Cold Spring Harb Perspect Biol. (2017) 9:a028035. doi: 10.1101/cshperspect.a028035
2. Mensah-Kane P, Sumien N. The potential of hyperbaric oxygen as a therapy for neurodegenerative diseases. GeroScience. (2023) 45:747–56. doi: 10.1007/s11357-022-00707-z
3. Wyss-Coray T. Ageing, neurodegeneration and brain rejuvenation. Nature. (2016) 539:180–6. doi: 10.1038/nature20411
4. Liu H, Lutz M, Luo S. Genetic association between epigenetic aging-acceleration and the progression of mild cognitive impairment to alzheimer's disease. J Gerontol A Biol Sci Med Sci. (2022) 77:1734–42. doi: 10.1093/gerona/glac138
5. McCartney DL, Hillary RF, Stevenson AJ, Ritchie SJ, Walker RM, Zhang Q, et al. Epigenetic prediction of complex traits and death. Genome Biol. (2018) 19:136. doi: 10.1186/s13059-018-1514-1
6. Horvath S, Raj K. DNA methylation-based biomarkers and the epigenetic clock theory of ageing. Nat Rev Genet. (2018) 19:371–84. doi: 10.1038/s41576-018-0004-3
7. Marioni RE, Shah S, McRae AF, Chen BH, Colicino E, Harris SE, et al. DNA methylation age of blood predicts all-cause mortality in later life. Genome Biol. (2015) 16:25. doi: 10.1186/s13059-015-0584-6
8. Horvath S, Erhart W, Brosch M, Ammerpohl O, von Schönfels W, Ahrens M, et al. Obesity accelerates epigenetic aging of human liver. Proc Natl Acad Sci U S A. (2014) 111:15538–43. doi: 10.1073/pnas.1412759111
9. Wolf EJ, Logue MW, Hayes JP, Sadeh N, Schichman SA, Stone A, et al. Accelerated DNA methylation age: Associations with ptsd and neural integrity. Psychoneuroendocrinology. (2016) 63:155–62. doi: 10.1016/j.psyneuen.2015.09.020
10. Akbarian S, Beeri MS, Haroutunian V. Epigenetic determinants of healthy and diseased brain aging and cognition. JAMA Neurol. (2013) 70:711–8. doi: 10.1001/jamaneurol.2013.1459
11. Prudencio M, Belzil VV, Batra R, Ross CA, Gendron TF, Pregent LJ, et al. Distinct brain transcriptome profiles in C9orf72-associated and sporadic als. Nat Neurosci. (2015) 18:1175–82. doi: 10.1038/nn.4065
12. Levine ME, Lu AT, Bennett DA, Horvath S. Epigenetic age of the pre-frontal cortex is associated with neuritic plaques, amyloid load, and alzheimer's disease related cognitive functioning. Aging (Albany NY). (2015) 7:1198–211. doi: 10.18632/aging.100864
13. Yang T, Xiao Y, Cheng Y, Huang J, Wei Q, Li C, et al. Epigenetic clocks in neurodegenerative diseases: A systematic review. J Neurol Neurosurg Psychiatry. (2023) 94:1064–70. doi: 10.1136/jnnp-2022-330931
14. Sato K, Mano T, Suzuki K, Toda T, Iwatsubo T, Iwata A. Attempt to predict a/T/N-based alzheimer's disease cerebrospinal fluid biomarkers using a peripheral blood DNA methylation clock. J Alzheimers Dis Rep. (2020) 4:287–96. doi: 10.3233/ADR-200205
15. Kim BH, Vasanthakumar A, Li QS, Nudelman KNH, Risacher SL, Davis JW, et al. Integrative analysis of DNA methylation and gene expression identifies genes associated with biological aging in alzheimer's disease. Alzheimers Dement (Amst). (2022) 14:e12354. doi: 10.1002/dad2.12354
16. Paul KC, Binder AM, Horvath S, Kusters C, Yan Q, Rosario ID, et al. Accelerated hematopoietic mitotic aging measured by DNA methylation, blood cell lineage, and parkinson's disease. BMC Genom. (2021) 22:696. doi: 10.1186/s12864-021-08009-y
17. Zhou A, Wu Z, Zaw Phyo AZ, Torres D, Vishwanath S, Ryan J. Epigenetic aging as a biomarker of dementia and related outcomes: A systematic review. Epigenomics. (2022) 14:1125–38. doi: 10.2217/epi-2022-0209
18. Tang X, Gonzalez-Latapi P, Marras C, Visanji NP, Yang W, Sato C, et al. Epigenetic clock acceleration is linked to age at onset of parkinson's disease. Mov Disord. (2022) 39:1831–40. doi: 10.1002/mds.29157
19. McCartney DL, Min JL, Richmond RC, Lu AT, Sobczyk MK, Davies G, et al. Genome-wide association studies identify 137 genetic loci for DNA methylation biomarkers of aging. Genome Biol. (2021) 22:194. doi: 10.1186/s13059-021-02398-9
20. Sugden K, Caspi A, Elliott ML, Bourassa KJ, Chamarti K, Corcoran DL, et al. Association of pace of aging measured by blood-based DNA methylation with age-related cognitive impairment and dementia. Neurology. (2022) 99:e1402–e13. doi: 10.1212/WNL.0000000000200898
21. Smith GD, Ebrahim S. 'Mendelian randomization': Can genetic epidemiology contribute to understanding environmental determinants of disease? Int J Epidemiol. (2003) 32:1–22. doi: 10.1093/ije/dyg070
22. Mohd Murshid N, Aminullah Lubis F, Makpol S. Epigenetic changes and its intervention in age-related neurodegenerative diseases. Cell Mol Neurobiol. (2022) 42:577–95. doi: 10.1007/s10571-020-00979-z
23. Zhu X, Chen Z, Shen W, Huang G, Sedivy JM, Wang H, et al. Inflammation, epigenetics, and metabolism converge to cell senescence and ageing: the regulation and intervention. Signal Transduct Target Ther. (2021) 6:245. doi: 10.1038/s41392-021-00646-9
24. Silva-Palacios A, Ostolga-Chavarría M, Zazueta C, Königsberg M. Nrf2: molecular and epigenetic regulation during aging. Ageing Res Rev. (2018) 47:31–40. doi: 10.1016/j.arr.2018.06.003
25. Li Y, Rong J, Zhong H, Liang M, Zhu C, Chang F, et al. Prenatal stress leads to the altered maturation of corticostriatal synaptic plasticity and related behavioral impairments through epigenetic modifications of dopamine D2 receptor in mice. Mol Neurobiol. (2021) 58:317–28. doi: 10.1007/s12035-020-02127-6
26. Delgado-Morales R, Agís-Balboa RC, Esteller M, Berdasco M. Epigenetic mechanisms during ageing and neurogenesis as novel therapeutic avenues in human brain disorders. Clin Epigenetics. (2017) 9:67. doi: 10.1186/s13148-017-0365-z
27. Maiuri T, Suart CE, Hung CLK, Graham KJ, Barba Bazan CA, Truant R. DNA damage repair in huntington's disease and other neurodegenerative diseases. Neurotherapeutics. (2019) 16:948–56. doi: 10.1007/s13311-019-00768-7
28. Lin MT, Beal MF. Mitochondrial dysfunction and oxidative stress in neurodegenerative diseases. Nature. (2006) 443:787–95. doi: 10.1038/nature05292
29. Kwon HS, Koh SH. Neuroinflammation in neurodegenerative disorders: the roles of microglia and astrocytes. Transl Neurodegener. (2020) 9:42. doi: 10.1186/s40035-020-00221-2
30. Gómez-Gómez ME, Zapico SC. Frailty, cognitive decline, neurodegenerative diseases and nutrition interventions. Int J Mol Sci. (2019) 20:2842. doi: 10.3390/ijms20112842
31. McCrory C, Fiorito G, Hernandez B, Polidoro S, O'Halloran AM, Hever A, et al. GrimAge outperforms other epigenetic clocks in the prediction of age-related clinical phenotypes and all-cause mortality. J Gerontol A Biol Sci Med Sci. (2021) 76:741–9. doi: 10.1093/gerona/glaa286
32. Scorza FA, de Almeida AG, Scorza CA, Finsterer J. Parkinson's disease, premature mortality, and amygdala. Mov Disord. (2022) 37:1110–1. doi: 10.1002/mds.29014
33. Diederich NJ, Goldman JG, Stebbins GT, Goetz CG. Failing as doorman and disc jockey at the same time: Amygdalar dysfunction in parkinson's disease. Mov Disord. (2016) 31:11–22. doi: 10.1002/mds.26460
34. Correale J, Gaitán MI, Ysrraelit MC, Fiol MP. Progressive multiple sclerosis: From pathogenic mechanisms to treatment. Brain. (2017) 140:527–46. doi: 10.1093/brain/aww258
35. Lovato L, Willis SN, Rodig SJ, Caron T, Almendinger SE, Howell OW, et al. Related B cell clones populate the meninges and parenchyma of patients with multiple sclerosis. Brain. (2011) 134:534–41. doi: 10.1093/brain/awq350
36. Kuhlmann T, Moccia M, Coetzee T, Cohen JA, Correale J, Graves J, et al. Multiple sclerosis progression: time for a new mechanism-driven framework. Lancet Neurol. (2023) 22:78–88. doi: 10.1016/s1474-4422(22)00289-7
37. Magliozzi R, Howell OW, Nicholas R, Cruciani C, Castellaro M, Romualdi C, et al. Inflammatory intrathecal profiles and cortical damage in multiple sclerosis. Ann Neurol. (2018) 83:739–55. doi: 10.1002/ana.25197
38. Fernández A, Quintana E, Velasco P, Moreno-Jimenez B, de Andrés B, Gaspar ML, et al. Senescent accelerated prone 8 (Samp8) mice as a model of age dependent neuroinflammation. J Neuroinflammation. (2021) 18:75. doi: 10.1186/s12974-021-02104-3
39. Rothhammer V, Borucki DM, Tjon EC, Takenaka MC, Chao CC, Ardura-Fabregat A, et al. Microglial control of astrocytes in response to microbial metabolites. Nature. (2018) 57:724–8. doi: 10.1038/s41586-018-0119-x
40. Huber AK, Giles DA, Segal BM, Irani DN. An emerging role for eotaxins in neurodegenerative disease. Clin Immunol. (2018) 189:29–33. doi: 10.1016/j.clim.2016.09.010
41. Duscha A, Gisevius B, Hirschberg S, Yissachar N, Stangl GI, Dawin E, et al. Propionic acid shapes the multiple sclerosis disease course by an immunomodulatory mechanism. Cell. (2020) 180:1067–80. doi: 10.1016/j.cell.2020.02.035
42. Theodoropoulou E, Alfredsson L, Piehl F, Marabita F, Jagodic MX. Different epigenetic clocks reflect distinct pathophysiological features of multiple sclerosis. Epigenomics. (2019) 11:1429–39. doi: 10.2217/epi-2019-0102
43. Hannum G, Guinney J, Zhao L, Zhang L, Hughes G, Sadda S, et al. Genome-wide methylation profiles reveal quantitative views of human aging rates. Mol Cell. (2013) 49:359–67. doi: 10.1016/j.molcel.2012.10.016
44. Chen BH, Marioni RE, Colicino E, Peters MJ, Ward-Caviness CK, Tsai PC, et al. DNA methylation-based measures of biological age: Meta-analysis predicting time to death. Aging (Albany NY). (2016) 8:1844–65. doi: 10.18632/aging.101020
45. Stevenson AJ, McCartney DL, Harris SE, Taylor AM, Redmond P, Starr JM, et al. Trajectories of inflammatory biomarkers over the eighth decade and their associations with immune cell profiles and epigenetic ageing. Clin Epigenetics. (2018) 10:159. doi: 10.1186/s13148-018-0585-x
46. van Langelaar J, Rijvers L, Smolders J, van Luijn MM. B and T cells driving multiple sclerosis: Identity, mechanisms and potential triggers. Front Immunol. (2020) 11:760. doi: 10.3389/fimmu.2020.00760
47. Huang J, Khademi M, Fugger L, Lindhe Ö, Novakova L, Axelsson M, et al. Inflammation-related plasma and csf biomarkers for multiple sclerosis. Proc Natl Acad Sci U S A. (2020) 117:12952–60. doi: 10.1073/pnas.1912839117
48. Gibson J, Russ TC, Clarke TK, Howard DM, Hillary RF, Evans KL, et al. A meta-analysis of genome-wide association studies of epigenetic age acceleration. PLoS Genet. (2019) 15:e1008104. doi: 10.1371/journal.pgen.1008104
49. Iridoy Zulet M, Pulido Fontes L, Ayuso Blanco T, Lacruz Bescos F, Mendioroz Iriarte M. Epigenetic changes in neurology: DNA methylation in multiple sclerosis. Neurologia. (2017) 32:463–8. doi: 10.1016/j.nrl.2015.03.011
50. Oblak L, van der Zaag J, Higgins-Chen AT, Levine ME, Boks MP. A systematic review of biological, social and environmental factors associated with epigenetic clock acceleration. Ageing Res Rev. (2021) 69:101348. doi: 10.1016/j.arr.2021.101348
51. Dendrou CA, Fugger L, Friese MA. Immunopathology of multiple sclerosis. Nat Rev Immunol. (2015) 15:545–58. doi: 10.1038/nri3871
52. Eren M, Boe AE, Klyachko EA, Vaughan DE. Role of plasminogen activator inhibitor-1 in senescence and aging. Semin Thromb Hemostasis. (2014) 40:645–51. doi: 10.1055/s-0034-1387883
53. Campisi J, d'Adda di Fagagna F. Cellular senescence: when bad things happen to good cells. Nat Rev Mol Cell Biol. (2007) 8:729–40. doi: 10.1038/nrm2233
54. Jiang CS, Rana T, Jin LW, Farr SA, Morley JE, Qin H, et al. Aging, plasminogen activator inhibitor 1, brain cell senescence, and alzheimer's disease. Aging Dis. (2023) 14:515–28. doi: 10.14336/ad.2022.1220
55. Angelucci F, Čechová K, Průša R, Hort J. Amyloid beta soluble forms and plasminogen activation system in alzheimer's disease: Consequences on extracellular maturation of brain-derived neurotrophic factor and therapeutic implications. CNS Neurosci Ther. (2019) 25:303–13. doi: 10.1111/cns.13082
56. Tucker HM, Kihiko M, Caldwell JN, Wright S, Kawarabayashi T, Price D, et al. The plasmin system is induced by and degrades amyloid-beta aggregates. J Neurosci. (2000) 20:3937–46. doi: 10.1523/jneurosci.20-11-03937.2000
57. Tucker HM, Kihiko-Ehmann M, Wright S, Rydel RE, Estus S. Tissue plasminogen activator requires plasminogen to modulate amyloid-beta neurotoxicity and deposition. J Neurochem. (2000) 75:2172–7. doi: 10.1046/j.1471-4159.2000.0752172.x
58. Akhter H, Huang WT, van Groen T, Kuo HC, Miyata T, Liu RM. A small molecule inhibitor of plasminogen activator inhibitor-1 reduces brain amyloid-Β Load and improves memory in an animal model of alzheimer's disease. J Alzheimers Dis. (2018) 64:447–57. doi: 10.3233/jad-180241
59. Liu RM, van Groen T, Katre A, Cao D, Kadisha I, Ballinger C, et al. Knockout of plasminogen activator inhibitor 1 gene reduces amyloid beta peptide burden in a mouse model of alzheimer's disease. Neurobiol Aging. (2011) 32:1079–89. doi: 10.1016/j.neurobiolaging.2009.06.003
60. Jacobsen JS, Comery TA, Martone RL, Elokdah H, Crandall DL, Oganesian A, et al. Enhanced clearance of abeta in brain by sustaining the plasmin proteolysis cascade. Proc Natl Acad Sci U S A. (2008) 105:8754–9. doi: 10.1073/pnas.0710823105
61. Yamamoto K, Takeshita K, Saito H. Plasminogen activator inhibitor-1 in aging. Semin Thromb Hemost. (2014) 40:652–9. doi: 10.1055/s-0034-1384635
62. Aillaud MF, Pignol F, Alessi MC, Harle JR, Escande M, Mongin M, et al. Increase in plasma concentration of plasminogen activator inhibitor, fibrinogen, von willebrand factor, factor viii:C and in erythrocyte sedimentation rate with age. Thromb Haemost. (1986) 55:330–2. doi: 10.1055/s-0038-1661557
63. Angelucci F, Veverova K, Katonová A, Vyhnalek M, Hort J. Serum pai-1/bdnf ratio is increased in alzheimer's disease and correlates with disease severity. ACS Omega. (2023) 8:36025–31. doi: 10.1021/acsomega.3c04076
64. Ziliotto N, Bernardi F, Piazza F. Hemostasis components in cerebral amyloid angiopathy and alzheimer's disease. Neurol Sci. (2021) 42:3177–88. doi: 10.1007/s10072-021-05327-7
65. Bi Oh S, Suh N, Kim I, Lee JY. Impacts of aging and amyloid-Β Deposition on plasminogen activators and plasminogen activator inhibitor-1 in the tg2576 mouse model of alzheimer's disease. Brain Res. (2015) 1597:159–67. doi: 10.1016/j.brainres.2014.11.042
66. Cho H, Joo Y, Kim S, Woo RS, Lee SH, Kim HS. Plasminogen activator inhibitor-1 promotes synaptogenesis and protects against Aβ(1-42)-induced neurotoxicity in primary cultured hippocampal neurons. Int J Neurosci. (2013) 123:42–9. doi: 10.3109/00207454.2012.724127
67. Rodriguez G, Eren M, Haupfear I, Viola KL, Cline EN, Miyata T, et al. Pharmacological inhibition of plasminogen activator inhibitor-1 prevents memory deficits and reduces neuropathology in app/ps1 mice. Psychopharmacol (Berl). (2023) 240:2641–55. doi: 10.1007/s00213-023-06459-8
68. Vinet A, Obert P, Dutheil F, Diagne L, Chapier R, Lesourd B, et al. Impact of a lifestyle program on vascular insulin resistance in metabolic syndrome subjects: the resolve study. J Clin Endocrinol Metab. (2015) 100:442–50. doi: 10.1210/jc.2014-2704
69. Hoekstra T, Geleijnse JM, Schouten EG, Kluft C. Plasminogen activator inhibitor-type 1: Its plasma determinants and relation with cardiovascular risk. Thromb Haemost. (2004) 91:861–72. doi: 10.1160/th03-08-0546
70. Sasaki A, Kurisu A, Ohno M, Ikeda Y. Overweight/obesity, smoking, and heavy alcohol consumption are important determinants of plasma pai-1 levels in healthy men. Am J Med Sci. (2001) 322:19–23. doi: 10.1097/00000441-200107000-00004
71. Bai Y, Sigala W, Adams GR, Vaziri ND. Effect of exercise on cardiac tissue oxidative and inflammatory mediators in chronic kidney disease. Am J Nephrol. (2009) 29:213–21. doi: 10.1159/000156715
72. Pearce EE, Alsaggaf R, Katta S, Dagnall C, Aubert G, Hicks BD, et al. Telomere length and epigenetic clocks as markers of cellular aging: A comparative study. Geroscience. (2022) 44:1861–9. doi: 10.1007/s11357-022-00586-4
73. Shinko Y, Okazaki S, Otsuka I, Horai T, Kim S, Tanifuji T, et al. Accelerated epigenetic age and shortened telomere length based on DNA methylation in nicolaides-baraitser syndrome. Mol Genet Genomic Med. (2022) 10:e1876. doi: 10.1002/mgg3.1876
74. Tanifuji T, Okazaki S, Otsuka I, Mouri K, Horai T, Shindo R, et al. Epigenetic clock analysis reveals increased plasma cystatin C levels based on DNA methylation in major depressive disorder. Psychiatry Res. (2023) 322:115103. doi: 10.1016/j.psychres.2023.115103
75. Li QS, Vasanthakumar A, Davis JW, Idler KB, Nho K, Waring JF, et al. Association of peripheral blood DNA methylation level with alzheimer's disease progression. Clin Epigenetics. (2021) 13:191. doi: 10.1186/s13148-021-01179-2
76. López-Otín C, Blasco MA, Partridge L, Serrano M, Kroemer G. The hallmarks of aging. Cell. (2013) 153:1194–217. doi: 10.1016/j.cell.2013.05.039
77. Demanelis K, Jasmine F, Chen LS, Chernoff M, Tong L, Delgado D, et al. Determinants of telomere length across human tissues. Science. (2020) 369:eaaz6876. doi: 10.1126/science.aaz6876
78. Honig LS, Kang MS, Schupf N, Lee JH, Mayeux R. Association of shorter leukocyte telomere repeat length with dementia and mortality. Arch Neurol. (2012) 69:1332–9. doi: 10.1001/archneurol.2012.1541
79. Hägg S, Zhan Y, Karlsson R, Gerritsen L, Ploner A, van der Lee SJ, et al. Short telomere length is associated with impaired cognitive performance in european ancestry cohorts. Transl Psychiatry. (2017) 7:e1100. doi: 10.1038/tp.2017.73
80. Fani L, Hilal S, Sedaghat S, Broer L, Licher S, Arp PP, et al. Telomere length and the risk of alzheimer's disease: The rotterdam study. J Alzheimers Dis. (2020) 73:707–14. doi: 10.3233/jad-190759
81. Rodríguez-Fernández B, Vilor-Tejedor N, Arenaza-Urquijo EM, Sánchez-Benavides G, Suárez-Calvet M, Operto G, et al. Genetically predicted telomere length and alzheimer's disease endophenotypes: A mendelian randomization study. Alzheimers Res Ther. (2022) 14:167. doi: 10.1186/s13195-022-01101-9
82. Smith LK, He Y, Park JS, Bieri G, Snethlage CE, Lin K, et al. Β2-microglobulin is a systemic pro-aging factor that impairs cognitive function and neurogenesis. Nat Med. (2015) 21:932–7. doi: 10.1038/nm.3898
83. Ferrero H, Larrayoz IM, Martisova E, Solas M, Howlett DR, Francis PT, et al. Increased levels of brain adrenomedullin in the neuropathology of alzheimer's disease. Mol Neurobiol. (2018) 55:5177–83. doi: 10.1007/s12035-017-0700-6
84. Wang R, Chen Z, Fu Y, Wei X, Liao J, Liu X, et al. Plasma cystatin C and high-density lipoprotein are important biomarkers of alzheimer's disease and vascular dementia: A cross-sectional study. Front Aging Neurosci. (2017) 9:26. doi: 10.3389/fnagi.2017.00026
85. Zhao Y, Zheng Q, Hong Y, Gao Y, Hu J, Lang M, et al. Β(2)-microglobulin coaggregates with Aβ and contributes to amyloid pathology and cognitive deficits in alzheimer's disease model mice. Nat Neurosci. (2023) 26:1170–84. doi: 10.1038/s41593-023-01352-1
86. Sheikh AM, Wada Y, Tabassum S, Inagaki S, Mitaki S, Yano S, et al. Aggregation of cystatin C changes its inhibitory functions on protease activities and amyloid Β Fibril formation. Int J Mol Sci. (2021) 22:9682. doi: 10.3390/ijms22189682
87. Keren-Shaul H, Spinrad A, Weiner A, Matcovitch-Natan O, Dvir-Szternfeld R, Ulland TK, et al. A unique microglia type associated with restricting development of alzheimer's disease. Cell. (2017) 169:1276–90.e17. doi: 10.1016/j.cell.2017.05.018
88. Yang DS, Stavrides P, Mohan PS, Kaushik S, Kumar A, Ohno M, et al. Reversal of autophagy dysfunction in the tgcrnd8 mouse model of alzheimer's disease ameliorates amyloid pathologies and memory deficits. Brain. (2011) 134:258–77. doi: 10.1093/brain/awq341
89. Buerger K, Uspenskaya O, Hartmann O, Hansson O, Minthon L, Blennow K, et al. Prediction of alzheimer's disease using midregional proadrenomedullin and midregional proatrial natriuretic peptide: A retrospective analysis of 134 patients with mild cognitive impairment. J Clin Psychiatry. (2011) 72:556–63. doi: 10.4088/JCP.09m05872oli
90. Clement J, Yan Q, Agrawal M, Coronado RE, Sturges JA, Horvath M, et al. Umbilical cord plasma concentrate has beneficial effects on DNA methylation grimAge and human clinical biomarkers. Aging Cell. (2022) 21:e13696. doi: 10.1111/acel.13696
91. Johnstone SE, Gladyshev VN, Aryee MJ, Bernstein BE. Epigenetic clocks, aging, and cancer. Science. (2022) 378:1276–7. doi: 10.1126/science.abn4009
92. Banerjee R, Smith J, Eccles MR, Weeks RJ, Chatterjee A. Epigenetic basis and targeting of cancer metastasis. Trends Cancer. (2022) 8:226–41. doi: 10.1016/j.trecan.2021.11.008
93. Chatterjee A, Rodger EJ, Eccles MR. Epigenetic drivers of tumourigenesis and cancer metastasis. Semin Cancer Biol. (2018) 51:149–59. doi: 10.1016/j.semcancer.2017.08.004
Keywords: epigenetic aging, neurodegenerative diseases, Mendelian randomization study, Alzheimer’s disease, Parkinson’s disease, Multiple Sclerosis
Citation: Fan J, Liu Q, Liu X, Gong M, Leong II, Tsang Y, Xu X, Lei S, Duan L, Zhang Y, Liao M and Zhuang L (2024) The effect of epigenetic aging on neurodegenerative diseases: a Mendelian randomization study. Front. Endocrinol. 15:1372518. doi: 10.3389/fendo.2024.1372518
Received: 22 January 2024; Accepted: 19 April 2024;
Published: 10 May 2024.
Edited by:
Marco Segatto, University of Molise, ItalyReviewed by:
Ernesto G. Miranda-Morales, University of Texas Medical Branch at Galveston, United StatesCopyright © 2024 Fan, Liu, Liu, Gong, Leong, Tsang, Xu, Lei, Duan, Zhang, Liao and Zhuang. This is an open-access article distributed under the terms of the Creative Commons Attribution License (CC BY). The use, distribution or reproduction in other forums is permitted, provided the original author(s) and the copyright owner(s) are credited and that the original publication in this journal is cited, in accordance with accepted academic practice. No use, distribution or reproduction is permitted which does not comply with these terms.
*Correspondence: Lixing Zhuang, emh1YW5nbGl4aW5nQDE2My5jb20=
Disclaimer: All claims expressed in this article are solely those of the authors and do not necessarily represent those of their affiliated organizations, or those of the publisher, the editors and the reviewers. Any product that may be evaluated in this article or claim that may be made by its manufacturer is not guaranteed or endorsed by the publisher.
Research integrity at Frontiers
Learn more about the work of our research integrity team to safeguard the quality of each article we publish.