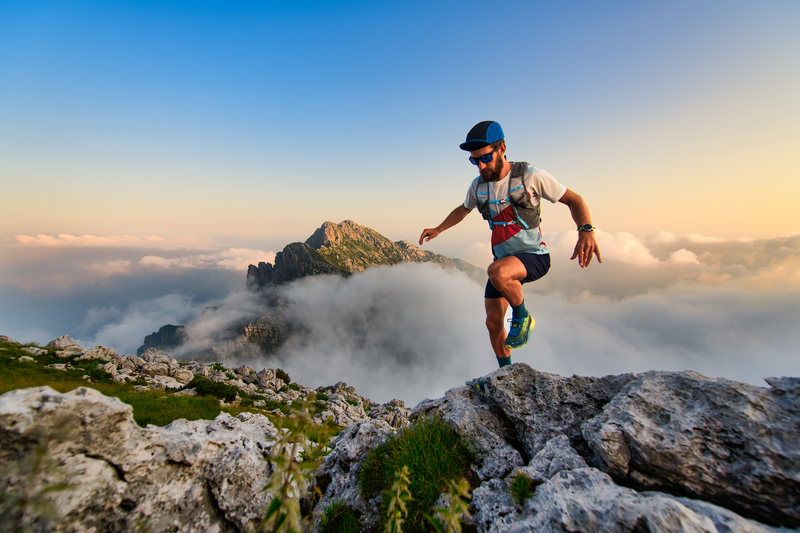
95% of researchers rate our articles as excellent or good
Learn more about the work of our research integrity team to safeguard the quality of each article we publish.
Find out more
ORIGINAL RESEARCH article
Front. Endocrinol. , 10 June 2024
Sec. Diabetes: Molecular Mechanisms
Volume 15 - 2024 | https://doi.org/10.3389/fendo.2024.1366290
This article is part of the Research Topic Personalized Therapies for Monogenic Diabetes View all 5 articles
Purpose: Diabetes and its complications cause a heavy burden of disease worldwide. In recent years, Mendelian randomization (MR) has been widely used to discover the pathogenesis and epidemiology of diseases, as well as to discover new therapeutic targets. Therefore, based on systematic “druggable” genomics, we aim to identify new therapeutic targets for diabetes and analyze its pathophysiological mechanisms to promote its new therapeutic strategies.
Material and method: We used double sample MR to integrate the identified druggable genomics to evaluate the causal effect of quantitative trait loci (eQTLs) expressed by druggable genes in blood on type 1 and 2 diabetes (T1DM and T2DM). Repeat the study using different data sources on diabetes and its complications to verify the identified genes. Not only that, we also use Bayesian co-localization analysis to evaluate the posterior probabilities of different causal variations, shared causal variations, and co-localization probabilities to examine the possibility of genetic confounding. Finally, using diabetes markers with available genome-wide association studies data, we evaluated the causal relationship between established diabetes markers to explore possible mechanisms.
Result: Overall, a total of 4,477 unique druggable genes have been gathered. After filtering using methods such as Bonferroni significance (P<1.90e-05), the MR Steiger directionality test, Bayesian co-localization analysis, and validation with different datasets, Finally, 7 potential druggable genes that may affect the results of T1DM and 7 potential druggable genes that may affect the results of T2DM were identified. Reverse MR suggests that C4B may play a bidirectional role in the pathogenesis of T1DM, and none of the other 13 target genes have a reverse causal relationship. And the 7 target genes in T2DM may each affect the biomarkers of T2DM to mediate the pathogenesis of T2DM.
Conclusion: This study provides genetic evidence supporting the potential therapeutic benefits of targeting seven druggable genes, namely MAP3K13, KCNJ11, REG4, KIF11, CCNE2, PEAK1, and NRBP1, for T2DM treatment. Similarly, targeting seven druggable genes, namely ERBB3, C4B, CD69, PTPN22, IL27, ATP2A1, and LT-β, has The potential therapeutic benefits of T1DM treatment. This will provide new ideas for the treatment of diabetes and also help to determine the priority of drug development for diabetes.
Diabetes is a chronic metabolic disorder that is characterized by an increase in blood sugar levels caused by absolute or relative insulin deficiency (1). It is estimated that one in every 10 people in the world has diabetes, and this number will continue to increase in the coming decades. The International Diabetes Federation estimates that the number of diabetes patients is expected to increase from 425 million adults in 2017 to 629 million in 2045. This will affect approximately 9.9% of the global population, resulting in an increasingly unsustainable global health burden (2, 3). Moreover, diabetes complications also lead to a heavy burden of disease worldwide. Acute complications of diabetes (such as diabetes ketoacidosis [DKA], hypertonic hyperglycemic coma [HHS]) and vascular complications of diabetes macrovascular and microvascular systems (such as cardiovascular disease [CVD], diabetes nephropathy [DKD], diabetes retinopathy [DR], neuropathy, and diabetes foot) are the main causes of quality of life impairment and death in diabetes patients, which has brought a serious burden to global public health (4, 5).
However, the current treatment of diabetes often relies on two main ways: changing lifestyles (such as diet, exercise, and weight loss) and lifelong use of hypoglycemic drugs. Since the 1950s, eight types of hypoglycemic drugs have been approved and widely used by the global Food and Drug Administration. This includes insulin preparations, traditional oral hypoglycemic drugs (metformin, thiazolidinediones (TZDs), sulfonylureas (SUs), glinides, and α-glucosidase inhibitors), modern therapies based on intestinal proinsulin (such as dipeptidyl peptidase-4 inhibitors (DPP4is) and glucagon-like peptide-1 receptor agonists (GLP-1RA), and sodium glucose cotransporter-2 inhibitors (SGLT2is) (6, 7). However, in the case of traditional hypoglycemic drugs, their adverse events (hypoglycemia, weight gain, gastrointestinal disorders, psychological insulin resistance, and edema) can negatively affect the acceptability of treatment, thereby reducing patient satisfaction and adherence to the therapy (8). Compared with traditional oral drugs, incretin-based therapies (DPP4is and GLP-1RA) and SGLT2is are new drugs for the treatment of diabetes. They play an important role in delaying the complications of diabetes and controlling weight (9). SGLT2is (engagliflozin and dapagliflozin) has a strong role in reducing the risk of hospitalization for heart failure, slowing down the progression of diabetes nephropathy, and protecting the kidney. However, due to the mechanism of action of SGLT2is (promoting urination and sodium excretion by inhibiting glucose reabsorption in proximal renal tubules), it cannot be used in patients with renal insufficiency. Meanwhile, SGLT2is can lead to accelerated loss of bone mineral density, an increased risk of fractures, and an increased risk of urinary and reproductive system infections (10, 11). DPP4is (Sigliptin) and GLP-1RA (Lilalutide) are incretin-based therapies that are helpful to control and reduce the weight of diabetes patients in the treatment of diabetes, but their side effects include severe nausea, vomiting, dizziness, and gastrointestinal discomfort, which make some patients intolerable (12). In summary, both traditional drugs that focus on insulin secretion and insulin sensitization, as well as modern therapies based on incretin-based therapies, can have unnecessary side effects on patients, leading to non-compliance and treatment failure (13). Moreover, these treatment methods cannot reverse this process. Therefore, evaluating the common genomic basis between diabetes and diabetes complications is very important for better understanding its potential pathophysiology and possibly controlling disease progression. In the past few decades, “druggable” genes or their encoded proteins have been targeted by drugs or may be used as proteins targeting small molecules or monoclonal antibodies. The “druggable” genes that simultaneously encode protein or gene expression can serve as drug targets and provide strong clues for disease treatment (14, 15).
Mendelian randomization (MR) analysis provides a valuable alternative to randomized clinical trials by utilizing genetic variations associated with specific exposures. By adopting this method, the causal relationship of the disease can be evaluated and potential therapeutic targets can be identified, which can be validated in subsequent clinical trials (16). Therefore, through MR analysis, there should be many disease-specific drug targets that have not yet been developed among the thousands of other loci identified in GWAS and similar high-quality genetic associations (expression quantitative trait loci, eQTL) studies. In this study (Figure 1), we conducted systematic “druggable” genome-wide MR to identify therapeutic targets for diabetes. Secondly, we conducted a reverse MR analysis to assess the existence of a reverse causal relationship. Thirdly, we conducted a Steiger directionality test to eliminate SNPs in the opposite direction so as to ensure the directionality of the association between the “druggable” gene and diabetes.Fourthly, we conducted co location analysis to verify the robustness of the expression tool variable (IV), and repeated validation studies were conducted using different diabetes data sources to verify the stability of the identified druggable genes. Finally, we evaluated the causal relationship between the identified druggable genes and the established biomarkers of diabetes to explore the possible mechanisms of these genes and the pathogenesis of diabetes.
The design of this study referred to the Mendelian Randomized Enhanced Epidemiological Observation Study Report (STROBE-MR) (17), and all participants in this study were subjects of European ancestry to reduce population stratification bias. In order to obtain the necessary data, publicly accessible datasets from eQTLGen, derived from genome-wide association studies (GWAS) and expression quantitative trait loci (eQTL) studies, were used (https://eqtlgen.org/). In addition, all the data used in this work came from studies with subject consent and ethical recognition; therefore, our study does not require ethical approval from the institutional review committee.
Druggable genes were obtained from the Drug-Gene Interaction Database (DGIdb V.4.2.0, https://www.dgidb.org/) and a recent review on the ‘druggability’ of genes (14). The DGIdb provides information on drug-gene interactions and druggable genes from publications, databases, and other web-based sources. Obtained a total of approximately 4,477 “patent drug genes” (Supplementary Table 1).
Organizational-specific eQTL data from eQTLGen (https://eqtlgen.org/). The eQTLGen data includes both cis and trans eQTLs (16,987 cis eQTLs and 6,298 trans eQTLs) from 31,684 blood samples, with the majority being healthy individuals of European ancestry (18).
The data set for type 2 diabetes (T2DM) comes from a meta-analysis that combines GWAS data sets of three European pedigrees (62,892 cases of T2DM and 59,6424 controls) to determine the genetic locus of T2DM (19). The genotype data of 9,358 type 1 diabetes cases and 15,705 control subjects from 12 European cohorts were deeply interpolated in the type 1 diabetes (T1DM) dataset, and then the genome-wide association test and meta-analysis were conducted (20). All validation sets of type 1 and type 2 diabetes and its 12 complications, namely, acute complications (diabetes ketoacidosis), diabetes coma, chronic microvascular complications (diabetes retinopathy, diabetes nephropathy, and diabetes neuropathy), and chronic macrovascular complications (diabetes peripheral circulation complications), are derived from the FinnGen R9 study (https://www.finngen.fi/en/). We have listed all the data sources and detailed information in Table 1.
Select druggable genome eQTLs as exposure data. In order to generate IV, meet the assumption of MR, and obtain reliable IV, we conducted a series of strict standards on the systematic druggable genome eQTLs for screening IV: (1) Select SNPs with genome-wide significance (p<5×10–8) and an acceptable mutation probability (secondary allele frequency>1%); (2) Execute clump (r^2<0.001, kb=10,000kb) to eliminate linkage imbalance between genetic instruments; (3) The F-statistic is used to estimate the strength of each genetic instrument and select all strong instrumental variables (F > 10) (21). The formula is R^ 2×(N − 2)/(1 − R^ 2), where R^ 2 is the cumulative explained variance of selected SNPs in exposure that used (2×EAF×(1 − EAF)×beta^ 2)/[(2×EAF×(1 − EAF) ×beta^ 2) + (2×EAF×(1 − EAF)×N×SE(beta) ^ 2)], where N is the sample size of research, EAF is the effect allele frequency, beta is the estimated genetic effect, and SE(beta) is the standard error of the beta. Under strict screening conditions, a total of 2,619 “druggable” genes (8,904 significant SNPs) were included in the preliminary analysis.
In this study, we used the “druggable” gene eQTLs as exposure data and used type 1 diabetes and type 2 diabetes as outcomes data for dual-sample MR analysis. If there is only one SNP for a given gene, eQTL, use the Wald ratio. When two or more genetic instruments (SNPs) are available, inverse variance weighting (Re IVW) is the main analytical method. This method is used to combine the causal effects of individual SNPs, allowing for heterogeneity between SNPs and returning unbiased estimates of causal relationships when all IVs are valid and pleiotropy levels are balanced. For preliminary analysis, we used Bonferroni correction to adjust for multiple tests, with a threshold P-value of 0.05/2619 (P<1.90e-05) to determine the priority of the results for further analysis.
For the preliminary validation results, we first conducted an MR Steiger directionality test, which effectively removed SNPs with opposite causal directions. The MR-Steiger filtering hypothesis suggests that effective genetic variations should explain more exposure differences than results. If the genetic tool does not meet this criterion, the genetic variation is determined to have bidirectional effects. After removing SNPs with bidirectional effects, we perform a double-sample MR analysis again.
For the preliminary significant results obtained, we selected significant genetic instruments (SNPs) from the original exposure data of T2DM and T1DM according to the same screening criteria for “druggable” gene eQTLs and used “druggable” gene eQTL as the outcome data for bidirectional MR analysis to detect potential reverse causal relationships. The results have statistical significance at P<0.05.
Bayesian co-localization analysis uses the “coloc” software package to evaluate the probability of two features sharing the same causal variable. This analysis helps to examine the possibility of genetic confounding by evaluating the posterior probabilities of different causal variations, shared causal variations, and co-localization probabilities. This analysis provides several outputs of interest. Co-localization generates a posterior probability corresponding to one of the following five assumptions: PPH0, no association with either trait; PPH1, association with the expression of the gene but not the diabetes risk; PPH2, association with the diabetes risk but not the expression of the gene; PPH3, association with the diabetes risk and expression of the gene, with distinct causal variants; and PPH4, association with the diabetes risk and expression of the gene, with a shared causal variant. We restricted our analysis to genes reaching PPH3+PPH4 of ≥0.8 owing to limited power in the co-localization analysis. We think that there is evidence of a common localization of this gene.
Due to differences in genetic effects between different datasets. We use the same-variant and significant-variant strategies in different datasets to replicate the primary findings and further determine the stability of our results. T2DM and its complications, as well as T1DM and its complications, are all from the FinnGen R9 study. For external validation, we still use the Bonferroni correction to adjust for multiple tests. The threshold P-value for T2DM and its complications is 0.05/13 (P<0.0038), while the threshold P-value for T1DM and its complications is 0.05/10 (P<0.005). We believe the results are significant.
To determine whether the potential “druggable” gene targets identified by our MR analysis are involved in the hypothetical pathological mechanism of diabetes (mainly T2DM). We conducted another set of dual-sample MR. Among them, we used the identified potential “druggable” gene eQTL as exposure data and T2DM-related biomarkers as outcome data. To further explore the influence of “druggable” gene expression on diabetes biomarkers.
All MR analyses were conducted using the R (version 4.2.2) TwoSampleMR, MRPRESSO, Mendelian Randomization, and Coloc software packages. All DNA positions were constructed based on the human reference genome using hg19 (GRCh37).
At Bonferroni significance (P<1.90e-05), preliminary MR analysis revealed a total of 23 “druggable” genes associated with T2DM and T1DM (Figure 2). Our preliminary results confirm that 13 potential drug targets have significant genetic effects on T2DM (Table 2). Specifically, the overexpression of Dopamine Receptor D4 (DRD4) gene, Mitogen-Activated protein kinase 13 (MAP3K13) gene, Mannosidase beta (MANBA) gene, Baculoviral IAP repeat containing 2 (BIRC2) gene, Nuclear receptor Binding protein 1 (NRBP1) gene, Kinesin family member 11 (KIF11) gene, Cyclin E2 (CCNE2) gene and Hyaluronidase 3 (HYAL3) gene is negatively correlated with the risk of developing T2DM (OR values all<1). In contrast, the overexpression of the MAX dimerization protein MLX (MLX) gene, regenerative family member 4 (REG4) gene, pseudopodium-rich atypical kinase 1 (PEAK1) gene, potassium inward rectifying channel subfamily J member 11 (KCNJ11) gene, and major histocompatibility complex class II DRβ5 (HLA-DRB5) gene is positively correlated with the risk of T2DM (OR values all>1). Moreover, our preliminary results also confirm that 10 potential drug targets have significant genetic effects on T1DM (Table 3). Specifically, the Erb-b2 receptor tyrosine kinase 3 (ERBB3) gene, Tyrosine kinase 2 (TYK2) gene, ATPase sarcoplasmic/endoplasmic reticulum Ca2+ transporting 1 (ATP2A1) gene, Interleukin-27 (IL-27) geneand Lymphotoxin β (LT- β) gene overexpression is negatively correlated with the risk of developing T1DM (OR values all<1). In contrast, the overexpression of the Endoplasmic reticulum protein 29 (ERP29) gene, CD69 molecule (CD69), protein tyrosine phosphatase non-receptor type 22 (PTPN22) gene, Cytochrome B-245 beta chain (CYBβ) gene, and complement C4B gene is positively correlated with the risk of T1DM (OR values all > 1).
Figure 2 Forest map of 23 potential drug targets for diabetes prevention identified in the dual sample MR phase.
In the preliminary analysis of MR, we first identified 23 “druggable” gene targets as potential therapeutic targets for T1DM and T2DM. Including 13 T2DM targets and 10 T1DM targets. To verify the stability of the results, we conducted a series of sensitivity analyses on 23 “druggable” genes (Tables 4, 5). Firstly, we conducted Steiger directionality tests on 23 potential “druggable” gene targets to further ensure directionality. For SNPs with opposite directions, we excluded them to consolidate the stability of the results. Secondly, we conducted a pleiotropy test and found that the P-values for pleiotropy testing were all greater than 0.05 among the 23 “druggable” genes (Supplementary Table 2). Thirdly, Bayesian co-localization strongly suggests that in T2DM, CCNE2, HLA-DRB5, KCNJ11, KIF11, MAMBA, MAP3K13, MLX, NRBP1, PEAK1, and REG4 shared the same variant with T2DM (PPH3+PPH4 ≥0.8). Similarly, in T1DM, Bayesian co-localization strongly suggests that ATP2A1, C4B, CD69, ERBB3, IL27, ERP29, PTPN22, TYK2, and LT-β shared the same variant with T1DM (PPH3+PPH4 ≥0.8). It is worth noting that the CYBβ gene serves as a trans-eQTL gene; therefore, Bayesian co-localization analysis was not performed on the CYBβ gene. Fourth, in order to avoid the reverse causal relationship between the 23 “druggable” gene targets and diabetes (T1DM and T2DM), we conducted dual sample reverse MR analysis using T1DM and T2DM as exposure data and 23 eQTLs of “druggable” genes as outcome data, with the IVW method as the main analysis method. We found that the DRD4 (p = 0.021) gene has a bidirectional causal relationship in T2DM, with P values of all other genes > 0.05. In T1DM, we found that the C4B gene (p = 0.000179) had a bidirectional causal relationship, with P values of all other genes > 0.05. This suggests that the DRD4 gene and C4B gene play a bidirectional role in the pathogenesis of T2DM and T1DM, respectively.
After a series of quality control steps such as sensitivity, co-localization, and reverse MR analysis, we deem that 21 gene targets have the most reliable MR evidence for T1DM and T2DM risk. C4B gene has a bidirectional causal relationship in T1DM risk. However, due to differences in genetic effects between different datasets. However, due to differences in genetic effects between different datasets, Therefore, we use the same-variant and significant-variant strategies in different datasets to replicate the primary findings and further determine the stability of our results (Table 6; Supplementary Table 3). We selected T1DM, T2DM, and their six related complications from the FinnGen R9 study as the external validation set. In external validation, we found that seven target genes (P<0.0038) were replicated in T2DM, namely MAP3K13, NRBP1, REG4, KIF11, PEAK1, CCNE2, and KCNJ11 (Figure 3). Among them, REG4 has a strong correlation with T2DM and can be replicated in all six complications of T2DM; KIF11 is replicated in four complications of T2DM; and KCNJ11 is replicated in three complications of T2DM (Supplementary Table 4). Same, Not only that, we also found a strong correlation between C4B, LT-β, and T1DM. C4B and LT-β were replicated in six complications of T1DM; ERBB3 and PTPN22 were replicated in five complications of T1DM; and IL27 was replicated in five complications of T1DM (Supplementary Table 5).
Figure 3 Forest diagram of 14 potential drug targets confirmed through external validation of FinnGen R9T1DM and T2DM.
The primary pathophysiological features of T2MD are impaired insulin secretion and insulin resistance, with insulin resistance being the principal cause of T2MD (22). Certain biomarkers, lifestyle, environmental, dietary, and socio-psychological factors contribute to the risk of insulin resistance and are also non-genetic risk factors for T2DM (23). Previous studies have determined that certain clinical characteristics (age, body mass index (BMI), waist-to-hip ratio, and hypertension) are associated with the risk of T2DM and a series of metabolic characteristics related to diabetes (24). There is a causal relationship between BMI and a 26% increase in T2DM risk, and a causal relationship between BMI adjusted waist to hip ratio and a 38% increase in T2DM risk (25). The coexistence of T2DM and hypertension significantly increases the risk of cardiovascular disease, end-stage renal disease, and death (26). Secondly, in a large-scale, 10-year prospective study of T2DM patients (the METSIM study), it was observed that vitamin D, branched-chain amino acids, low-density cholesterol, triglycerides, proinsulin levels, fatty acids, glycerol, mannose, glycoprotein acetyl (GlycA), and acetyl acetate are associated with an increased risk of T2DM and also serve as T2DM biomarkers (27, 28). Mannose is a hexose required for glycoprotein synthesis, which is significantly elevated in insulin-resistant subjects (29). Acetoacetate (ketone body) is an important marker of T2DM ketoacidosis (30). GlycA is associated with chronic inflammation of the pancreas and serves as a marker for predicting impaired insulin secretion (31). Insulin regulates the concentration of glycerol, triglycerides, and fatty acids in the serum by inhibiting fat breakdown. Therefore, the concentration of lipids in plasma also serves as a marker for predicting T2DM (32). Vitamin D may regulate insulin resistance and the pancreas β Cellular function plays a role in the pathogenesis of T2DM (33). The ratio of apolipoprotein to low-density cholesterol (LDL) is a predictor of worsening blood glucose and the incidence rate of T2DM (34).
In order to explore the potential role of identified drug targets in the pathogenesis of T2DM, we used the previously identified 7 potential “druggable” targets eQTL for T2DM as exposure data and 10 T2DM biomarkers (vitamin D, branched-chain amino acids, low-density cholesterol, triglycerides, proinsulin levels, fatty acids, glycerol, mannose, glycoprotein acetyl, and acetoacetate) and 3 clinical features (BMI, hypertension, and waist-to-hip ratio) as outcome data to further evaluate the genetic effects of potential “druggable” targets and T2DM biomarkers (Supplementary Table 6). We found that under P-values <0.05 (P<0.05), the level of vitamin D is only related to the KCNJ11 gene. The level of branched-chain amino acids is related to the NRBP1, KIF11, and CCNE2 genes. The level of LDL is related to the REG4, KIF11, and KCNJ11 genes. The level of triglycerides is related to the NRBP1, REG4, KIF11, and CCNE2 genes. The levels of proinsulin, mannose, and glycerol are only related to the NRBP1 gene. The level of total fatty acid is related to the NRBP1, MAP3K13, REG4, and KIF11 genes. The level of glycoprotein acetyl is related to the NRBP1, MAP3K13, REG4, KIF11, and PEAK1 genes. The level of acetoacetate is related to the REG4 and PEAK1 genes. The risk of obesity (BMI) is related to the NRBP1, KIF11, and KCNJ11 genes. The risk of hypertension is related to the NRBP1, REG4, CCNE2, and KCNJ11 genes. The waist-to-hip ratio is related to the MAP3K13, NRBP1, and KIF11 genes. (Supplementary Table 7).
To our knowledge, this work is the first to combine eQTL data from “druggable” genomics. Through the use of double-sample MR, Bayesian co-localization, and external validation of different data sets, we explored the potential pathophysiological mechanisms of T1DM and T2DM and specifically sought to identify new drug targets for diabetes treatment. In this study, we finally found 14 potential drug targets to prevent diabetes, including 7 “druggable” genes that may affect the expression of T1DM results and 7 “druggable” genes that may affect the expression of T2DM results.
Mitogen-activated protein kinase kinase 13 (MAP3K13), also known as leucine zipper kinase (LZK), has a high degree of homology with leucine zipper kinase (DLK). The common feature of both is the presence of a double leucine zipper (LZ) domain after the kinase domain, which can activate JNK and, to some extent, activate p38 MAPK to exert biological activity (35). The human DLK kinase domain consists of 127–375 amino acid residues, including four characteristic domains: the catalytic domain, the double leucine zipper domain, the glycine serine proline rich domain, and the glycine proline rich domain (36). In many previous studies, it has been confirmed that DLK is related to the occurrence of type 2 diabetes, and DLK is widely present in pancreatic islets. In the experiment of E. Oetjen et al., it was found that the overexpression of DLK in pancreatic β cells decreased the transcriptional activity of CBP/CERB, leading to decreased insulin secretion and the occurrence of diabetes (37). In the study by M.J. Stahnke et al., it was also found that overexpression of DLK not only inhibits the transcriptional activity of the human insulin gene promoter but also leads to a decrease in the function and quality of pancreatic islet β cells. Not only that, they also found that overexpression of DLK can induce the degradation of the insulin gene transcription factor v-Maf musculoaponeurotic fibrosarcoma oncogene family, protein A (MafA) (38). Mafa is an alkaline leucine zipper family transcription factor that can activate the expression of insulin in β-cells with PDX1 and NEUROD1. Therefore, MafA is essential for maintaining both adult β cells and β cellular function (39). Moreover, inhibiting DLK kinase may protect β cells from the influence of β cytotoxic pre-diabetes signals and prevent the development of diabetes (40). Therefore, MAP3K13/DLK is expected to become a new target for the treatment of T2DM.
Potassium inwardly rectifying channel subfamily J member 11 (KCNJ11) is a target most closely related to T2DM in our study and also an achievement based on the precise treatment of monogenic insulin deficiency and insulin resistance diabetes in the new century (41). The potassium inward rectifying 6.2 subunit (Kir6.2) of the ATP-sensitive potassium (K (ATP)) channel encoded by the KCNJ11 gene is a therapeutic target for sulfonylurea drugs and plays an important role in regulating glucose homeostasis. At present, sulfonylurea drugs targeting KCNJ11 (glibenclamide, gliclazide) are widely used as first-line treatment drugs for T2DM in clinical treatment (42).
Regenerating family member 4 (REG4) is a member of the calcium-dependent (C-type) lectin superfamily, mainly expressed in gastrointestinal tissues, including the colon, small intestine, stomach, and pancreas (43). Previous studies have found that REG4 plays an important role in pancreatic cell and duct regeneration. In Hu et al.’s experiment, it was found that the expression levels of Reg4 mRNA and protein were significantly increased during acute pancreatitis (AP). In addition, Reg4 upregulates the expression of Bcl-2 or Bcl-xL by activating the EGFR/Akt pathway, which can prevent arginine-induced acinar cell necrosis both in vivo and in vitro (44). This was also confirmed in another study (45). Moreover, in the animal experiment constructed by Wang et al. using rats, it was found that Reg4 may mediate intestinal fatty acid extraction and absorption through AMPK. The expression of Reg4 can reduce intestinal fat absorption to protect mice from high-fat diet-induced hepatic steatosis, increased fat accumulation, and insulin resistance (46). It is worth noting that although Reg4 plays a certain role in pancreatic regeneration, it also plays an important role in the occurrence, development, and invasion of pancreatic cancer as a serum marker of cancer (47).
Kinesin family member 11 (KIF11) belongs to the kinesin-5 family and is a protein-coding gene that plays an important role in cell mitosis, cell cycle, and differentiation. Previous studies have found that KIF11 is closely associated with familial exudative vitreoretinopathy, lymphedema, intellectual disability, chorioretinopathy, and an increased risk of T2DM (48, 49). Four single nucleotide polymorphisms (SNPs) of the KIF11 gene identified in the GWAS catalogue are associated with diabetes, including RS2153827, RS6583826, and RS7087591, which are all associated with the risk of T2DM, while RS7096101 is associated with insulin level. Moreover, KIF11 did not show significant racial differences in the risk of T2DM. KIF11 was found as a genetic variation associated with T2DM risk in large GWAS data from multiple ethnic cohorts, including Asian (Chinese, Japanese), European, and Southeast Asian populations (50–52). KIF11 has also been confirmed to be related to type 2 diabetes retinopathy, which is consistent with our external verification results (53). In summary, KIF11 is also expected to become a potential target for the treatment of T2DM.
Cyclin E2 (CCNE2) belongs to the highly conserved cyclin family and is a protein-coding gene that functions as a regulator of cell cycle-dependent protein kinase (CDK). The cyclin box domain in CCNE2 is an essential structural motif for the formation of cyclin CDK complexes. CCNE2 binds to CDK2 in a functional kinase complex with catalytic activity and plays a role in the G1/S transition of the cell cycle, reaching its peak expression in the G1-S phase. Plays an important role in cell proliferation and regeneration (54, 55). Therefore, the function of CCNE2 affects the cell cycle kinase CDK2 and the progression of the cell cycle. However, disruption of CDK2 and the cell cycle can lead to dysfunction of pancreatic β cells and a decrease in β cell mass, accelerating the progression of T2DM. In Yoon Kim et al.’s experiment, it was found that the absence of CDK2 can impair the function of adult β cells, affect the quality of pancreatic β cells, and lead to insulin secretion defects (56). This phenomenon was also confirmed in Jiang et al.’s experiment (57). In addition, CDK2 agonists may help to restore β cell function and restart β cell proliferation to combat β cell failure in diabetes patients. Therefore, CCNE2 is expected to become a potential therapeutic target for T2DM patients.
PEAK1 and NRBP1 are the two genes that we found to have the least correlation with reported T2DM. PEAK1 encodes a non-receptor tyrosine kinase, which is a member of the novel kinase family three (NFK3) family. This gene is associated with the actin cytoskeleton and adhesive plaques and plays a role in regulating cell migration, proliferation, and cancer metastasis (58). At present, the research on PEAK1 is more focused on its role in tumor metastasis and invasion, so the research on PEAK1 and diabetes, especially T2DM, needs further discussion. There are few reports about NRBP1 and diabetes. At present, studies believe that the expression of the NRBP1 gene is related to apo CIII sialylation and hypertriglyceridemia. However, apo CIII is involved in triglyceride-rich lipoprotein metabolism and is associated with β cell damage, insulin resistance, and cardiovascular disease (59).
T1DM is an autoimmune disease caused by complex interactions between multiple susceptible genes, environmental factors, and the immune system. Its characteristic is T-cell-mediated self-destruction of pancreatic β cells, leading to an absolute lack of insulin secretion (60). At present, the treatment of T1DM often relies on exogenous insulin. However, in our study, we found seven genes closely related to the pathophysiology of T1DM. They are ERBB3, C4B, CD69, PTPN22, IL27, ATP2A1, and LT -β respectively. ERBB3, CD69, PTPN22, and IL27 have a highly significant correlation with T1DM. These four genes are four of more than 50 loci significantly related to human non-HLA T1DM identified by the Genetic Alliance for T1DM (61). ERBB3 is located on chromosome 12q13, also known as Her3 (human epidermal growth factor receptor 3), and is a member of the epidermal growth factor receptor (EGFR) family of receptor tyrosine kinases (62). A study has found that the sites rs11171739 and rs2292239 associated with ERBB3 affect the occurrence and development of T1DM (63). Firstly, ERBB3 is considered a novel regulator of pancreatic β cell apoptosis, and downregulation of ERBB3 can reduce basal cell and cytokine-induced cell apoptosis. Secondly, ERBB3 can activate various downstream signaling pathways, such as phosphoinositol 3-kinase (PI3K), nuclear factor κB (NF-κB), and extracellular signal-regulated kinase (ERK), among which the PI3K pathway is an important survival pathway for pancreatic β cells (64, 65). There are also studies indicating that ERBB3 can regulate β-cellulose (BTC) to promote β cell regeneration (66). IL27 is a heterodimeric cytokine that plays a role in innate immunity, regulating helper T cell development, inhibiting T cell proliferation, and stimulating cytotoxic T cell activity. It has multiple effects on innate immune cells (67). IL27 can target CD4 helper T cells and promote the differentiation of type 1 effector cells (TH1) and type 2 effector cells (TH2). Previous studies have found that SNP rs4788084, located within 2kb upstream of IL27, serves as a T1D-related risk allele (61, 68). IL-27 plays a protective role in the development of T1DM and has anti-inflammatory properties by regulating T cell polarization and cytokine levels. Overexpression of IL-27 can reduce pancreatic blood glucose levels, immune cell infiltration, and the expression of the anti-inflammatory cytokine IL-1β mRNA, thereby alleviating inflammation of the pancreas (69). Secondly, IL-27 directly affects the differentiation and effector function of CD4 and CD8 T cells in the pancreas, enhancing the expression of T-bet and IFN-γ in the pancreas (70). IL-27 can also directly change the balance between islet regulatory T cells (Tregs) and helper T cells 1 (Th1), thereby regulating the diabetic activity of CD8 T cells (71).
The CD69 gene encodes a member of the type II transmembrane receptor calcium-dependent lectin superfamily and is expressed on various white blood cells, including newly activated lymphocytes, certain subtypes of memory T cells, regulatory T cells (Tregs), and natural killer (NK) T cells (72). CD69 is a marker of early lymphocyte activation, highly upregulated in T1DM lymphocytes. CD69 exerts its immune regulatory function by controlling the balance between Th/Treg cell differentiation and enhancing Treg inhibitory activity, thereby regulating immune tolerance and lymphocyte infiltration in pancreatic islet cells (73). The protein encoded by the protein tyrosine phosphatase non-receptor 22 (PTPN22) gene is a lymphoid-specific intracellular phosphatase that participates in regulating the function of the CBL protein in the T cell receptor signaling pathway by binding to the molecular adaptor protein CBL. Previous studies have shown that the allele variant PTPN22 R620W of the PTPN22 gene is the strongest non-HLA genetic risk factor for the development of T1DM. However, the pathogenesis of PTPN22 in T1DM is complex, and current research generally suggests that the expression of PTPN22 may play a role in β cell apoptosis and affect β cell function (74).
C4B is located in the major histocompatibility complex (MHC) class III region on chromosome 6, encoding the basic form of complement factor 4, and is part of the classical activation pathway along with the C4A gene. The C4B-related pathways include complement cascade response and regulation of insulin-like growth factor (IGF) transport and insulin-like growth factor binding protein (IGFBP) uptake. The immune deficiency caused by complement deficiency in the classical pathway plays an important role (75). This also indicates that the occurrence of T1DM is closely related to the complement pathway. The complement components C3 and C4 are highly expressed in the pancreas (including islets), and the copy number of the C4B gene or higher C4B protein concentration can affect the ability of endogenous insulin or C-peptide (76). In addition, studies have found that during insular encephalitis, the complement cascade reaction is activated, and complement factors deposit into the pancreas, thereby damaging the pancreas and pancreatic islet cells. Moreover, the increase in serum complement C4B level is also related to diabetes nephropathy (77). ATPase sarcoplasmic/endoplasmic reticulum Ca2+transporter 1 (ATP2A1) is a protein-coding gene that encodes a SERCA Ca2+ ATPase enzyme, which plays an important role in maintaining low concentrations of Ca2+ions in the cytoplasm (78). However, intracellular Ca2+ is an important coordinating agent in various aspects of cellular physiology, and changes in cellular Ca2+ dynamics help regulate normal and pathological signal transduction that controls cell growth and survival. The disturbance of Ca2+ concentration is closely related to ER stress and mitochondrial dysfunction, which are the two major defects leading to T1DM and T2DM (79). The changes in SERCA function, endoplasmic reticulum stress (ER), and Ca2+ cycle are the basis of the mechanism of pancreatic β cell apoptosis. A study has found that SERCA2 deficiency can lead to an increase in basal cytoplasmic Ca2+ levels, directly leading to a decrease in insulin secretion, a decrease in β cell mass, and an increase in β cell ER stress and death (80, 81). Lymphotoxin-β (LT-β) is a protein-coding gene that functions as a biological cousin of tumor necrosis factor α (TNFα) by binding to the lymphotoxin p receptor (LTβR) and participating in the normal development of lymphoid tissue. LT-β participates in the pathogenesis of T1D by promoting the formation of the tertiary lymphoid organ (TLO) around the pancreas and also enhances the autoimmune response by regulating the synergistic effect of multiple cytokines and inflammatory mediators, inducing insulinitis and peripancreatic inflammation, leading to β cell destruction (82).
Our research provides several significant advantages. Firstly, we used multiple sample datasets to validate our results, and the larger the sample size, the higher the ability to detect eQTL. Secondly, we used multiple methods such as multivariate MR analysis, MR Steiger filtering, reverse MR, co-localization analysis, and pleiotropy testing to validate our results, further ensuring the reliability of our results. As is well known, the success rate of drugs from phase 1 trials to approval is very low, requiring significant investment of manpower, material resources, and financial resources. Although MR analysis cannot replace in vitro and in vivo preclinical evaluations of drug targets, combined genomics methods can serve as an auxiliary tool for drug development, with the potential to better prioritize drug targets entering clinical trials. Therefore, this may have a significant impact on drug development costs. However, we have to admit that our research also has some limitations. First, the use of genetic variation to study and determine the effect of potential therapeutic drugs for diabetes is limited because the pathogenesis of diabetes is complex and involves a multi-gene, multi-factor, and multi-target pathogenic pathway. Therefore, drug MR analysis helps to determine the direction of causal relationships rather than quantifying the degree of correlation. Secondly, the number of IVs in eQTL MR is limited, with most not exceeding three SNPs, which limits the credibility of MR results. Thirdly, although we conducted detailed heterogeneity and pleiotropy tests, we cannot completely rule out the influence of horizontal pleiotropy. Fourthly, as our research findings mainly relate to individuals of European ancestry, these findings may not necessarily apply to other racial groups. Finally, despite the large sample size of our study, the several genetic tools used for the results are affected to varying degrees by low statistical power and incomplete phenotype definitions, which may render most of the explored association studies ineffective. Therefore, our results still need to be validated in larger queues. Even validated in clinical trials.
The raw data supporting the conclusions of this article will be made available by the authors, without undue reservation.
Ethical approval was not required for the study involving humans in accordance with the local legislation and institutional requirements. Written informed consent to participate in this study was not required from the participants or the participants’ legal guardians/next of kin in accordance with the national legislation and the institutional requirements.
HL: Conceptualization, Data curation, Formal analysis, Writing – original draft. WL: Software, Supervision, Writing – review & editing. DL: Writing – review & editing. LY: Writing – review & editing. YX: Writing – review & editing. PS: Writing – review & editing. LW: Writing – review & editing. ZZ: Funding acquisition, Project administration, Supervision, Writing – original draft, Writing – review & editing.
The author(s) declare financial support was received for the research, authorship, and/or publication of this article. This research was supported by the doctoral science fund of Binzhou Medical University(BY2016KYQD17) and Shandong Natural Science Foundation (ZR2019MH068).
We would like to thank all the research participants of this study.
The authors declare that the research was conducted in the absence of any commercial or financial relationships that could be construed as a potential conflict of interest.
All claims expressed in this article are solely those of the authors and do not necessarily represent those of their affiliated organizations, or those of the publisher, the editors and the reviewers. Any product that may be evaluated in this article, or claim that may be made by its manufacturer, is not guaranteed or endorsed by the publisher.
The Supplementary Material for this article can be found online at: https://www.frontiersin.org/articles/10.3389/fendo.2024.1366290/full#supplementary-material
1. DeFronzo RA, Ferrannini E, Groop L, Henry RR, Herman WH, Holst JJ, et al. Type 2 diabetes mellitus. Nat Rev Dis Primers. (2015) 1:15019. doi: 10.1038/nrdp.2015.19
2. Zimmet PZ, Magliano DJ, Herman WH, Shaw JE. Diabetes: a 21st century challenge. Lancet Diabetes Endocrinol. (2014) 2:56–64. doi: 10.1016/S2213-8587(13)70112-8
3. Cho NH, Shaw JE, Karuranga S, Huang Y, da Rocha Fernandes JD, Ohlrogge AW, et al. IDF Diabetes Atlas: Global estimates of diabetes prevalence for 2017 and projections for 2045. Diabetes Res Clin Pract. (2018) 138:271–81. doi: 10.1016/j.diabres.2018.02.023
4. Morrish NJ, Wang SL, Stevens LK, Fuller JH, Keen H. Mortality and causes of death in the WHO Multinational Study of Vascular Disease in Diabetes. Diabetologia. (2001) 44 Suppl 2:S14–21. doi: 10.1007/PL00002934
5. Cole JB, Florez JC. Genetics of diabetes mellitus and diabetes complications. Nat Rev Nephrol. (2020) 16:377–90. doi: 10.1038/s41581-020-0278-5
6. Bailey CJ, Day C. Treatment of type 2 diabetes: future approaches. Br Med Bull. (2018) 126:123–37. doi: 10.1093/brimed/ldy013
7. Taylor SI, Yazdi ZS, Beitelshees AL. Pharmacological treatment of hyperglycemia in type 2 diabetes. J Clin Invest. (2021) 131:e142243. doi: 10.1172/JCI142243
8. Pollack MF, Purayidathil FW, Bolge SC, Williams SA. Patient-reported tolerability issues with oral antidiabetic agents: associations with adherence; treatment satisfaction and health-related quality of life. Diabetes Res Clin Pract. (2010) 87:204–10. doi: 10.1016/j.diabres.2009.11.023
9. Rizzo MR, Di Meo I, Polito R, Auriemma MC, Gambardella A, di Mauro G, et al. Cognitive impairment and type 2 diabetes mellitus: focus of SGLT2 inhibitors treatment. Pharmacol Res. (2022) 176:106062. doi: 10.1016/j.phrs.2022.106062
10. D'Andrea E, Wexler DJ, Kim SC, Paik JM, Alt E, Patorno E. Comparing effectiveness and safety of SGLT2 inhibitors vs DPP-4 inhibitors in patients with type 2 diabetes and varying baseline hbA1c levels. JAMA Intern Med. (2023) 183:242–54. doi: 10.1001/jamainternmed.2022.6664
11. Bilezikian JP, Watts NB, Usiskin K, Polidori D, Fung A, Sullivan D, et al. Evaluation of bone mineral density and bone biomarkers in patients with type 2 diabetes treated with canagliflozin. J Clin Endocrinol Metab. (2016) 101:44–51. doi: 10.1210/jc.2015-1860
12. Padhi S, Nayak AK, Behera A. Type II diabetes mellitus: a review on recent drug based therapeutics. BioMed Pharmacother. (2020) 131:110708. doi: 10.1016/j.biopha.2020.110708
13. Tan SY, Mei Wong JL, Sim YJ, Wong SS, Mohamed Elhassan SA, Tan SH, et al. Type 1 and 2 diabetes mellitus: A review on current treatment approach and gene therapy as potential intervention. Diabetes Metab Syndr. (2019) 13:364–72. doi: 10.1016/j.dsx.2018.10.008
14. Finan C, Gaulton A, Kruger FA, Lumbers RT, Shah T, Engmann J, et al. The druggable genome and support for target identification and validation in drug development. Sci Transl Med. (2017) 9:eaag1166. doi: 10.1126/scitranslmed.aag1166
15. Nelson MR, Tipney H, Painter JL, Shen J, Nicoletti P, Shen Y, et al. The support of human genetic evidence for approved drug indications. Nat Genet. (2015) 47:856–60. doi: 10.1038/ng.3314
16. Sekula P, Del Greco MF, Pattaro C, Köttgen A. Mendelian randomization as an approach to assess causality using observational data. J Am Soc Nephrol. (2016) 27:3253–65. doi: 10.1681/ASN.2016010098
17. Skrivankova VW, Richmond RC, Woolf BAR, Davies NM, Swanson SA, VanderWeele TJ, et al. Strengthening the reporting of observational studies in epidemiology using mendelian randomisation (STROBE-MR): explanation and elaboration. BMJ. (2021) 375:n2233. doi: 10.1136/bmj.n2233
18. Võsa U, Claringbould A, Westra HJ, Bonder MJ, Deelen P, Zeng B, et al. Large-scale cis- and trans-eQTL analyses identify thousands of genetic loci and polygenic scores that regulate blood gene expression. Nat Genet. (2021) 53:1300–10. doi: 10.1038/s41588-021-00913-z
19. Xue A, Wu Y, Zhu Z, Zhang F, Kemper KE, Zheng Z, et al. Genome-wide association analyses identify 143 risk variants and putative regulatory mechanisms for type 2 diabetes. Nat Commun. (2018) 9:2941. doi: 10.1038/s41467-018-04951-w
20. Forgetta V, Manousaki D, Istomine R, Ross S, Tessier MC, Marchand L, et al. Rare genetic variants of large effect influence risk of type 1 diabetes. Diabetes. (2020) 69:784–95. doi: 10.2337/db19-0831
21. Pierce BL, Ahsan H, Vanderweele TJ. Power and instrument strength requirements for Mendelian randomization studies using multiple genetic variants. Int J Epidemiol. (2011) 40:740–52. doi: 10.1093/ije/dyq151
22. Bellou V, Belbasis L, Tzoulaki I, Evangelou E. Risk factors for type 2 diabetes mellitus: An exposure-wide umbrella review of meta-analyses. PloS One. (2018) 13:e0194127. doi: 10.1371/journal.pone.0194127
23. Laakso M. Biomarkers for type 2 diabetes. Mol Metab. (2019) 27S:S139–46. doi: 10.1016/j.molmet.2019.06.016
24. Corbin LJ, Richmond RC, Wade KH, Burgess S, Bowden J, Smith GD, et al. BMI as a modifiable risk factor for type 2 diabetes: refining and understanding causal estimates using Mendelian randomization. Diabetes. (2016) 65:3002–7. doi: 10.2337/db16-0418
25. Sun D, Zhou T, Heianza Y, Li X, Fan M, Fonseca VA, et al. Type 2 diabetes and hypertension. Circ Res. (2019) 124:930–7. doi: 10.1161/CIRCRESAHA.118.314487
26. Laakso M, Kuusisto J, Stančáková A, Kuulasmaa T, Pajukanta P, Lusis AJ, et al. The Metabolic Syndrome in Men study: a resource for studies of metabolic and cardiovascular diseases. J Lipid Res. (2017) 58:481–93. doi: 10.1194/jlr.O072629
27. Stančáková A, Kuulasmaa T, Kuusisto J, Mohlke KL, Collins FS, Boehnke M, et al. Genetic risk scores in the prediction of plasma glucose, impaired insulin secretion, insulin resistance and incident type 2 diabetes in the METSIM study. Diabetologia. (2017) 60:1722–30. doi: 10.1007/s00125-017-4313-4
28. Mardinoglu A, Stančáková A, Lotta LA, Kuusisto J, Boren J, Blüher M, et al. Plasma mannose levels are associated with incident type 2 diabetes and cardiovascular disease. Cell Metab. (2017) 26:281–3. doi: 10.1016/j.cmet.2017.07.006
29. Mahendran Y, Vangipurapu J, Cederberg H, Stancáková A, Pihlajamäki J, Soininen P, et al. Association of ketone body levels with hyperglycemia and type 2 diabetes in 9,398 Finnish men. Diabetes. (2013) 62:3618–26. doi: 10.2337/db12-1363
30. Fizelova M, Jauhiainen R, Kangas AJ, Soininen P, Ala-Korpela M, Kuusisto J, et al. Differential associations of inflammatory markers with insulin sensitivity and secretion: the prospective METSIM study. J Clin Endocrinol Metab. (2017) 102:3600–9. doi: 10.1210/jc.2017-01057
31. Mahendran Y, Cederberg H, Vangipurapu J, Kangas AJ, Soininen P, Kuusisto J, et al. Glycerol and fatty acids in serum predict the development of hyperglycemia and type 2 diabetes in Finnish men. Diabetes Care. (2013) 36:3732–8. doi: 10.2337/dc13-0800
32. Grammatiki M, Rapti E, Karras S, Ajjan RA, Kotsa K. Vitamin D and diabetes mellitus: Causal or casual association? Rev Endocr Metab Disord. (2017) 18:227–41. doi: 10.1007/s11154-016-9403-y
33. Fizelova M, Miilunpohja M, Kangas AJ, Soininen P, Kuusisto J, Ala-Korpela M, et al. Associations of multiple lipoprotein and apolipoprotein measures with worsening of glycemia and incident type 2 diabetes in 6607 non-diabetic Finnish men. Atherosclerosis. (2015) 240:272–7. doi: 10.1016/j.atherosclerosis.2015.03.034
34. Jin Y, Zheng B. Multitasking: dual leucine zipper-bearing kinases in neuronal development and stress management. Annu Rev Cell Dev Biol. (2019) 35:501–21. doi: 10.1146/annurev-cellbio-100617-062644
35. Ferraris D, Yang Z, Welsbie D. Dual leucine zipper kinase as a therapeutic target for neurodegenerative conditions. Future Med Chem. (2013) 5:1923–34. doi: 10.4155/fmc.13.150
36. Oetjen E, Lechleiter A, Blume R, Nihalani D, Holzman L, Knepel W. Inhibition of membrane depolarisation-induced transcriptional activity of cyclic AMP response element binding protein (CREB) by the dual-leucine-zipper-bearing kinase in a pancreatic islet beta cell line. Diabetologia. (2006) 49:332–42. doi: 10.1007/s00125-005-0087-1
37. Stahnke MJ, Dickel C, Schröder S, Kaiser D, Blume R, Stein R, et al. Inhibition of human insulin gene transcription and MafA transcriptional activity by the dual leucine zipper kinase. Cell Signal. (2014) 26:1792–9. doi: 10.1016/j.cellsig.2014.04.006
38. Nishimura W, Iwasa H, Tumurkhuu M. Role of the transcription factor MAFA in the maintenance of pancreatic β-cells. Int J Mol Sci. (2022) 23:4478. doi: 10.3390/ijms23094478
39. Oetjen E. Regulation of beta-cell function and mass by the dual leucine zipper kinase. Arch Pharm (Weinheim). (2016) 349:410–3. doi: 10.1002/ardp.201600053
40. Hattersley AT, Patel KA. Precision diabetes: learning from monogenic diabetes. Diabetologia. (2017) 60:769–77. doi: 10.1007/s00125-017-4226-2
41. Philla KQ, Bauer AJ, Vogt KS, Greeley SA. Successful transition from insulin to sulfonylurea therapy in a patient with monogenic neonatal diabetes owing to a KCNJ11 F333L [corrected] mutation. Diabetes Care. (2013) 36:e201. doi: 10.2337/dc13-1690
42. Hartupee JC, Zhang H, Bonaldo MF, Soares MB, Dieckgraefe BK. Isolation and characterization of a cDNA encoding a novel member of the human regenerating protein family: Reg IV. Biochim Biophys Acta. (2001) 1518:287–93. doi: 10.1016/S0167-4781(00)00284-0
43. Hu G, Shen J, Cheng L, Guo C, Xu X, Wang F, et al. Reg4 protects against acinar cell necrosis in experimental pancreatitis. Gut. (2011) 60:820–8. doi: 10.1136/gut.2010.215178
44. Dai J, He Y, Jiang M, Niu M, Li B, Wu Z, et al. Reg4 regulates pancreatic regeneration following pancreatitis via modulating the Notch signaling. J Cell Physiol. (2021) 236:7565–77. doi: 10.1002/jcp.30397
45. Wang Y, Yan W, Lu Y, Du J, Tian X, Wu B, et al. Intestinal Reg4 deficiency confers susceptibility to high-fat diet-induced liver steatosis by increasing intestinal fat absorption in mice. JHEP Rep. (2023) 5:100700. doi: 10.1016/j.jhepr.2023.100700
46. Takayama R, Nakagawa H, Sawaki A, Mizuno N, Kawai H, Tajika M, et al. Serum tumor antigen REG4 as a diagnostic biomarker in pancreatic ductal adenocarcinoma. J Gastroenterol. (2010) 45:52–9. doi: 10.1007/s00535-009-0114-y
47. Miki H, Okada Y, Hirokawa N. Analysis of the kinesin superfamily: insights into structure and function. Trends Cell Biol. (2005) 15:467–76. doi: 10.1016/j.tcb.2005.07.006
48. Balikova I, Robson AG, Holder GE, Ostergaard P, Mansour S, Moore AT. Ocular manifestations of microcephaly with or without chorioretinopathy, lymphedema or intellectual disability (MCLID) syndrome associated with mutations in KIF11. Acta Ophthalmol. (2016) 94:92–8. doi: 10.1111/aos.12759
49. Lewis JP, Palmer ND, Hicks PJ, Sale MM, Langefeld CD, Freedman BI, et al. Association analysis in african americans of European-derived type 2 diabetes single nucleotide polymorphisms from whole-genome association studies. Diabetes. (2008) 57:2220–5. doi: 10.2337/db07-1319
50. Grarup N, Rose CS, Andersson EA, Andersen G, Nielsen AL, Albrechtsen A, et al. Studies of association of variants near the HHEX, CDKN2A/B, and IGF2BP2 genes with type 2 diabetes and impaired insulin release in 10,705 Danish subjects: validation and extension of genome-wide association studies. Diabetes. (2007) 56:3105–11. doi: 10.2337/db07-0856
51. Hu C, Zhang R, Wang C, Wang J, Ma X, Lu J, et al. PPARG, KCNJ11, CDKAL1, CDKN2A-CDKN2B, IDE-KIF11-HHEX, IGF2BP2 and SLC30A8 are associated with type 2 diabetes in a Chinese population. PloS One. (2009) 4:e7643. doi: 10.1371/journal.pone.0007643
52. Peng D, Wang J, Zhang R, Jiang F, Tam CHT, Jiang G, et al. CDKAL1 rs7756992 is associated with diabetic retinopathy in a Chinese population with type 2 diabetes. Sci Rep. (2017) 7:8812. doi: 10.1038/s41598-017-09010-w
53. Payton M, Coats S. Cyclin E2, the cycle continues. Int J Biochem Cell Biol. (2002) 34:315–20. doi: 10.1016/S1357-2725(01)00137-6
54. Zariwala M, Liu J, Xiong Y. Cyclin E2, a novel human G1 cyclin and activating partner of CDK2 and CDK3, is induced by viral oncoproteins. Oncogene. (1998) 17:2787–98. doi: 10.1038/sj.onc.1202505
55. Kim SY, Lee JH, Merrins MJ, Gavrilova O, Bisteau X, Kaldis P, et al. Loss of cyclin-dependent kinase 2 in the pancreas links primary β-cell dysfunction to progressive depletion of β-cell mass and diabetes. J Biol Chem. (2017) 292:3841–53. doi: 10.1074/jbc.M116.754077
56. Jiang Z, Li H, Schroer SA, Voisin V, Ju Y, Pacal M, et al. Hypophosphorylated pRb knock-in mice exhibit hallmarks of aging and vitamin C-preventable diabetes. EMBO J. (2022) 41:e106825. doi: 10.15252/embj.2020106825
57. Ding C, Tang W, Fan X, Wang X, Wu H, Xu H, et al. Overexpression of PEAK1 contributes to epithelial-mesenchymal transition and tumor metastasis in lung cancer through modulating ERK1/2 and JAK2 signaling. Cell Death Dis. (2018) 9:802. doi: 10.1038/s41419-018-0817-1
58. Naber A, Demus D, Slieker R, Nicolardi S, Beulens JWJ, Elders PJM, et al. Apolipoprotein-CIII O-glycosylation, a link between GALNT2 and plasma lipids. Int J Mol Sci. (2023) 24:14844. doi: 10.3390/ijms241914844
59. Atkinson MA, Eisenbarth GS, Michels AW. Type 1 diabetes. Lancet. (2014) 383:69–82. doi: 10.1016/S0140-6736(13)60591-7
60. Winkler C, Krumsiek J, Buettner F, Angermüller C, Giannopoulou EZ, Theis FJ, et al. Feature ranking of type 1 diabetes susceptibility genes improves prediction of type 1 diabetes. Diabetologia. (2014) 57:2521–9. doi: 10.1007/s00125-014-3362-1
61. Ma J, Lyu H, Huang J, Liu B. Targeting of erbB3 receptor to overcome resistance in cancer treatment. Mol Cancer. (2014) 13:105. doi: 10.1186/1476-4598-13-105
62. Keene KL, Quinlan AR, Hou X, Hall IM, Mychaleckyj JC, Onengut-Gumuscu S, et al. Evidence for two independent associations with type 1 diabetes at the 12q13 locus. Genes Immun. (2012) 13:66–70. doi: 10.1038/gene.2011.56
63. Kaur S, Mirza AH, Brorsson CA, Fløyel T, Størling J, Mortensen HB, et al. Hvidoere International Study Group. The genetic and regulatory architecture of ERBB3-type 1 diabetes susceptibility locus. Mol Cell Endocrinol. (2016) 419:83–91. doi: 10.1016/j.mce.2015.10.002
64. Elghazi L, Bernal-Mizrachi E. Akt and PTEN: beta-cell mass and pancreas plasticity. Trends Endocrinol Metab. (2009) 20:243–51. doi: 10.1016/j.tem.2009.03.002
65. Lee YS, Song GJ, Jun HS. Betacellulin-induced α-cell proliferation is mediated by erbB3 and erbB4, and may contribute to β-cell regeneration. Front Cell Dev Biol. (2021) 8:605110. doi: 10.3389/fcell.2020.605110
66. Pflanz S, Timans JC, Cheung J, Rosales R, Kanzler H, Gilbert J, et al. IL-27, a heterodimeric cytokine composed of EBI3 and p28 protein, induces proliferation of naive CD4+ T cells. Immunity. (2002) 16:779–90. doi: 10.1016/S1074-7613(02)00324-2
67. Meka RR, Venkatesha SH, Dudics S, Acharya B, Moudgil KD. IL-27-induced modulation of autoimmunity and its therapeutic potential. Autoimmun Rev. (2015) 14:1131–41. doi: 10.1016/j.autrev.2015.08.001
68. Wang R, Han G, Wang J, Chen G, Xu R, Wang L, et al. The pathogenic role of interleukin-27 in autoimmune diabetes. Cell Mol Life Sci. (2008) 65:3851–60. doi: 10.1007/s00018-008-8540-1
69. Ciecko AE, Foda B, Barr JY, Ramanathan S, Atkinson MA, Serreze DV, et al. Interleukin-27 is essential for type 1 diabetes development and Sjögren syndrome-like inflammation. Cell Rep. (2019) 29:3073–3086.e5. doi: 10.1016/j.celrep.2019.11.010
70. Ciecko AE, Schauder DM, Foda B, Petrova G, Kasmani MY, Burns R, et al. Self-renewing islet TCF1+ CD8 T cells undergo IL-27-controlled differentiation to become TCF1- terminal effectors during the progression of type 1 diabetes. J Immunol. (2021) 207:1990–2004. doi: 10.4049/jimmunol.2100362
71. Sancho D, Gómez M, Sánchez-Madrid F. CD69 is an immunoregulatory molecule induced following activation. Trends Immunol. (2005) 26:136–40. doi: 10.1016/j.it.2004.12.006
72. Gorabi AM, Hajighasemi S, Kiaie N, Gheibi Hayat SM, Jamialahmadi T, Johnston TP, et al. The pivotal role of CD69 in autoimmunity. J Autoimmun. (2020) 111:102453. doi: 10.1016/j.jaut.2020.102453
73. Galvani G, Fousteri G. PTPN22 and islet-specific autoimmunity: What have the mouse models taught us? World J Diabetes. (2017) 8:330–6. doi: 10.4239/wjd.v8.i7.330
74. Blanchong CA, Chung EK, Rupert KL, Yang Y, Yang Z, Zhou B, et al. Genetic, structural and functional diversities of human complement components C4A and C4B and their mouse homologues, Slp and C4. Int Immunopharmacol. (2001) 1:365–92. doi: 10.1016/S1567-5769(01)00019-4
75. Kingery SE, Wu YL, Zhou B, Hoffman RP, Yu CY. Gene CNVs and protein levels of complement C4A and C4B as novel biomarkers for partial disease remissions in new-onset type 1 diabetes patients. Pediatr Diabetes. (2012) 13:408–18. doi: 10.1111/j.1399-5448.2011.00836.x
76. Sarkar S, Elliott EC, Henry HR, Ludovico ID, Melchior JT, Frazer-Abel A, et al. Systematic review of type 1 diabetes biomarkers reveals regulation in circulating proteins related to complement, lipid metabolism, and immune response. Clin Proteomics. (2023) 20:38. doi: 10.1186/s12014-023-09429-6
77. Odermatt A, Barton K, Khanna VK, Mathieu J, Escolar D, Kuntzer T, et al. The mutation of Pro789 to Leu reduces the activity of the fast-twitch skeletal muscle sarco(endo)plasmic reticulum Ca2+ ATPase (SERCA1) and is associated with Brody disease. Hum Genet. (2000) 106:482–91. doi: 10.1007/s004390000297
78. Chemaly ER, Troncone L, Lebeche D. SERCA control of cell death and survival. Cell Calcium. (2018) 69:46–61. doi: 10.1016/j.ceca.2017.07.001
79. Zarain-Herzberg A, García-Rivas G, Estrada-Avilés R. Regulation of SERCA pumps expression in diabetes. Cell Calcium. (2014) 56:302–10. doi: 10.1016/j.ceca.2014.09.005
80. Zhang IX, Raghavan M, Satin LS. The endoplasmic reticulum and calcium homeostasis in pancreatic beta cells. Endocrinology. (2020) 161:bqz028. doi: 10.1210/endocr/bqz028
81. Wu Q, Salomon B, Chen M, Wang Y, Hoffman LM, Bluestone JA, et al. Reversal of spontaneous autoimmune insulitis in nonobese diabetic mice by soluble lymphotoxin receptor. J Exp Med. (2001) 193:1327–32. doi: 10.1084/jem.193.11.1327
Keywords: diabetes, drug targets, genetics research, Mendelian randomization, genetic effect analysis
Citation: Li H, Li W, Li D, Yuan L, Xu Y, Su P, Wu L and Zhang Z (2024) Based on systematic druggable genome-wide Mendelian randomization identifies therapeutic targets for diabetes. Front. Endocrinol. 15:1366290. doi: 10.3389/fendo.2024.1366290
Received: 06 January 2024; Accepted: 28 May 2024;
Published: 10 June 2024.
Edited by:
Ming Liu, Tianjin Medical University General Hospital, ChinaReviewed by:
Rohit B. Sharma, NewYork-Presbyterian, United StatesCopyright © 2024 Li, Li, Li, Yuan, Xu, Su, Wu and Zhang. This is an open-access article distributed under the terms of the Creative Commons Attribution License (CC BY). The use, distribution or reproduction in other forums is permitted, provided the original author(s) and the copyright owner(s) are credited and that the original publication in this journal is cited, in accordance with accepted academic practice. No use, distribution or reproduction is permitted which does not comply with these terms.
*Correspondence: Liqiang Wu, YnlmeXdscUAxMjYuY29t; Zhiqiang Zhang, emhhbmd6cTY3OEBnbWFpbC5jb20=
†These authors have contributed equally to this work
Disclaimer: All claims expressed in this article are solely those of the authors and do not necessarily represent those of their affiliated organizations, or those of the publisher, the editors and the reviewers. Any product that may be evaluated in this article or claim that may be made by its manufacturer is not guaranteed or endorsed by the publisher.
Research integrity at Frontiers
Learn more about the work of our research integrity team to safeguard the quality of each article we publish.