- 1Department of Endocrinology, Jiangsu Province Hospital of Chinese Medicine, Affiliated Hospital of Nanjing University of Chinese Medicine, Nanjing, China
- 2The First Clinical Medical College, Nanjing University of Chinese Medicine, Nanjing, China
Background: Gut microbiota (GM) homeostasis in the human body is closely associated with health, which can be used as a regulator for preventing the onset and progression of disease. Diabetic microvascular complications bring about not only a huge economic burden to society, but also miserable mental and physical pain. Thus, alteration of the GM may be a method to delay diabetic microvascular complications.
Objective: A two-sample Mendelian randomization (MR) analysis was conducted to reveal the causal inference between GM and three core diabetic microvascular complications, namely, diabetic kidney disease (DKD), diabetic retinopathy (DR), and diabetic neuropathy (DNP).
Methods: First, genome-wide association study (GWAS) summary statistics for GM from the MiBioGen consortium and three main diabetic microvascular complications acquired from the FinnGen research project were assessed. Second, a forward MR analysis was conducted to assess the causality of GM on the risk of DKD, DR, and DNP. Third, a series of sensitivity studies, such as heterogeneity tests, pleiotropy evaluations, and leave-one-out analyses, were further conducted to assess the accuracy of MR analysis. Finally, Steiger tests and reverse MR analyses were performed to appraise the possibility of reverse causation.
Results: A total of 2,092 single-nucleotide polymorphisms related to 196 bacterial traits were selected as instrumental variables. This two-sample MR analysis provided strongly reasonable evidence that 28 genetically predicted abundance of specific GM that played non-negligible roles in the occurrence of DKD, DR, and DNP complications were causally associated with 23 GM, the odds ratio of which generally ranged from 0.9 to 1.1. Further sensitivity analysis indicated low heterogeneity, low pleiotropy, and high reliability of the causal estimates.
Conclusion: The study raised the possibility that GM may be a potential target to prevent and delay the progression of diabetic microvascular complications. Further experiments of GM therapy on diabetic microvascular complications are warranted to clarify their effects and specific mechanisms.
Introduction
Diabetic microvascular complications are characterized by the damage of small vessels or nerves as a result of chronic persistent hyperglycemic state in patients with diabetes mellitus (DM), manifested as abnormal structure and changes in functions of the corresponding targeted organs ultimately (1–3). It is known that diabetic kidney disease (DKD), diabetic retinopathy (DR), and diabetic neuropathy (DNP) are three major diabetic chronic microvascular complications that need to be screened comprehensively upon diagnosis of type 2 diabetes (T2D) and type 1 diabetes (T1D) in the fifth year and even at least annually thereafter because of the characteristics of insidious onset and irreversible progression, which leads to enormous economic burden and prolonged potential physical and mental suffering (4, 5). In spite of the large number of novel treatments available, the incidence and prevalence of DM continue to increase around the world and show a trend of younger generations being affected, which leads to an obvious rise in the corresponding microvascular complications (6–8).
Plenty of microbes are enriched in the gastrointestinal tract, the biggest microbiota habitat in the human body; meanwhile, these microbiota exist in a dynamic balanced state for health regulation purposes (9). The composition and metabolism of gut microbiota (GM) play an important role in DM and its complications (10, 11), which is affected by multiple factors such as diet (12), demographics (13), and use of medication (14). Recent studies have focused on the association between GM and DM and its microvascular complications and especially put forward the theory of “gut–kidney axis” (15), “gut–retina axis” (16), “gut–brain axis” (17), and “gut–peripheral nerve axis” (18). Therefore, focusing on the modulation of GM with probiotics, prebiotics, synbiotics, or even fecal microbial transplantation may be a promising breakthrough direction on DM and subsequent microvascular complications. Nonetheless, the link between GM and diabetic microvascular complications driven by causative mechanistic interactions or merely being correlative remains unclear.
Mendelian randomization (MR) is a complementary statistical approach that leverages the genetic variants associated with exposure factors such as instrumental variables (IVs) to imply the causal inference between exposure and disease outcomes (19). MR analyses for inferring the causal relationship of GM on multiple diseases have been widely applied due to various findings from large-scale genome-wide association studies (GWASs) to data conducted on GM (20, 21). Previous studies have indicated that some GM are causally associated with T1D (22) and T2D (23). However, there was no evidence that demonstrated whether GM became a potential causal factor on diabetic microvascular complications. Therefore, MR analysis allows us to assess the contribution of GM on diabetic microvascular complications in the present study. Furthermore, this study could facilitate drug discovery and obtain reliable surrogate biomarkers to predict the onset and progression of diabetic microvascular complications, including DKD, DR, and DNP.
Materials and methods
Data sources
Genetic variants for GM were obtained from the MiBioGen consortium, which performed the largest multi-ancestry genome-wide meta-analysis published to date (20). The study included 18,340 individuals, of whom over 70% were of European ancestry in 24 cohorts, targeting three distinct variable regions of the 16S rRNA gene to profile the microbial composition and utilizing direct taxonomic binning for conducting taxonomic classification. In addition, every sample was rarefied to 10,000 reads in all datasets on the interpretation of different sequencing depths. Microbiome quantitative trait loci mapping analysis, including 211 taxa (five levels, in the order of genus, family, order, class, and phylum), was conducted to identify the effect of host genetics on the relative abundance levels of microbial taxa. More details related to the GM data could be found elsewhere (20). All the GWAS summary statistics of diabetic complications in this study were acquired from the FinnGen research project (https://r9.finngen.fi/). Lastly, a total of 4,111 cases and 308,539 controls in DKD, 10,413 cases and 308,633 controls in DR, and 2,843 cases and 271,817 controls in DNP were included.
Ethics statement
The summary-level data involved in this study are free and publicly available for download. The respective institutions have approved the ethics statement of each GWAS in this study. There were no individual-level data in this study; thus, new ethical review board approval was unnecessary.
Instrumental variable selection
The selection of optimal IVs was vital for the robustness and accuracy of the causal association, which conformed to the MR’s three principal assumptions (24), specifically for relevance, independence, and exclusion assumption. To explore more relations, a relatively more comprehensive threshold (p < 1e−05) of single-nucleotide polymorphisms (SNPs) associated with GM was applied (25). Data of the European-based 1,000 Genome Projects were set as the reference panel for performing a linkage disequilibrium (LD) analysis, where SNPs had r2 < 0.001 and the window size was 10,000 kb. F statistics not less than 10 represented the notable strength of the selected SNPs for each bacterial taxon, the equation of which is R2(N − 2)/(1 − R2) (26), where R2 denotes the proportion in exposure variance of each selected IV interpretation and N represents the sample size (27). Minor allele frequency (MAF) <0.01 of SNPs was removed, aiming at clearing mutations in less than 1% of the population. Furthermore, palindromic SNPs were removed to prevent distortion of strand orientation or allele coding.
Statistical analysis
Inverse variance weighted (IVW) was the primary method to examine the causal association between GM and diabetic complications based on ratio estimates of each variant (28), which provided a more conservative but robust estimate (29), the p-value of which determined the criterion for the existence of a causal association between exposure and outcome. Cochran’s Q test was used for the assessment of the heterogeneity among IVs, and the random-effects model was applied in the presence of significant heterogeneity; otherwise, the fixed-effects model was used (30). A series of additional MR analyses were conducted for calculating the causal effect values, including the weighted median, MR-Egger regression, simple mode, and weighted mode methods. The weighted median method, the median of the weighted ratio estimates of valid variants as the total weight of the instrument, showed consistent results with IVW in the condition of even up to 50% of invalid IVs (28). The MR-Egger regression test employed a weighted linear regression instead of setting the intercept to zero in IVW and allowed the presence of over 50% of invalid IVs, intercept estimated by MR-Egger regression could serve to estimate the average horizontal effect of pleiotropy (31, 32). The largest cluster of SNPs was applied in simple mode and the weights were assigned to each SNP in weighted mode (33, 34). In scenarios where the beta values for exposure and outcome summary data exhibited significant disparity in distribution, the correct factor will be utilized.
Evaluation of overall horizontal pleiotropy was conducted by the MR pleiotropy residual sum and outlier (MR-PRESSO) global test, and then outlier removal could correct this pleiotropy (25). The intercept from the MR-Egger test further verified the sensitivity; pintercept < 0.05 indicated horizontal pleiotropy (31). Directional causal inference judged by the MR Steiger directionality test was made (35). Additionally, the leave-one-out analysis was performed to validate data robustness and avoid affecting significant results via a single SNP (36).
Reverse Mendelian randomized analysis
A reverse MR analysis was also conducted to explore whether the disease outcomes have any causal impact on the GM, especially the identified significant ones. Noteworthily, SNPs related to each genus of diabetic complications at the locus-wide significance threshold (p < 5e−08) were selected as potential IVs to obtain more comprehensive results (22), which were different from screening the IVs in the pre-MR analysis.
The threshold of statistical significance was identified as p < 0.05 and odds ratio (OR) with 95% confidence interval (CI) was regarded as the effect between GM and diabetic complications. False discovery rate (FDR) correction was conducted; a q-value of more than 0.1 means no suggestive causal association (37). R software (version 4.3.1) was used for all the above statistical analyses. We used the Strengthening the Reporting of Observational Studies in Epidemiology using Mendelian Randomization (STROBE-MR) checklist published in 2021 as reference (38).
R software (version 4.3.1. R Foundation for Statistical Computing 2023) was utilized in all of the analyses. The packages TwoSampleMR (version 0.5.7), MRPRESSO (version 1.0), vroom (version 1.6.4), grid (version 4.3.1), forestploter (version 1.1.1), data.table (version 1.14.8), phenoscanner (version 1.0), dplyr (version 1.1.2), fdrtool (version 1.2.17), plinkbinr (version 0.0.0.9000), and ieugwasr (version 0.1.5) were used.
Results
Causal effects of GM on diabetic microvascular diseases
A total of 196 bacterial taxa were identified for MR analysis after removing 15 unknown families or genera. All the F statistics of the IVs selected were more than 10, indicating no evidence of weak instrumental bias. Meanwhile, over three SNPs of each GM were included for a successful MR-PRESSO test. In addition, the MAFs were all more than 0.01. Lastly, a total of 2,092 IVs were identified, including 9 phyla (114 SNPs), 16 classes (179 SNPs), 20 orders (216 SNPs), 32 families (352 SNPs), and 119 genera (1,231 SNPs). Detailed information of IVs used in the MR analysis for the causal inference is presented in Supplementary Table S1. It was worth noting that the more taxonomically distinct GM was chosen when GM shared the same SNPs; in other words, we would pick the class Verrucomicrobiae instead of the order Verrucomicrobiales. An overall view of the MR analysis process and major hypotheses is shown in Figure 1.
Diabetic kidney disease
In the phylum level, only the genetic predicted Bacteroidetes (OR = 1.43, 95% CI = 1.09–1.88, p = 1.08e−02) was causally associated with DKD. As for the class level, we found that the higher genetically predicted Bacteroidia (OR = 1.45, 95% CI = 1.12–1.87, p = 4.57e−03) and Verrucomicrobiae (OR = 1.40, 95% CI = 1.13–1.73, p = 1.80e−03) were identified as higher risks of DKD. Meanwhile, the genetically predicted genera Catenibacterium (OR = 1.31, 95% CI = 1.08–1.59, p = 6.40e−03), Lachnoclostridium (OR = 1.43, 95% CI = 1.13–1.82, p = 3.11e−03), and Parasutterella (OR = 1.27, 95% CI = 1.07–1.51, p = 6.52e−03) were also causally associated with DKD. However, we found that family Bacteroidaceae (OR = 0.72, 95% CI = 0.52–0.99, p = 4.64e−02), family Victivallaceae (OR = 0.87, 95% CI = 0.77–0.98, p = 2.45e−02), genus Coprococcus2 (OR = 0.74, 95% CI = 0.58–0.96, p = 2.47e−02), and genus Lactococcus (OR = 0.85, 95% CI = 0.73–0.99, p = 3.93e−02) played protective roles in the causal inference between GM and DKD.
Diabetic retinopathy
We found that the genetically predicted class Bacteroidia was causally associated with DR (OR = 1.24, 95% CI = 1.05–1.46, p = 9.75e−03). In the family level, the higher genetically predicted BacteroidalesS24 (OR = 1.16, 95% CI = 1.02–1.33, p = 2.45e−02), ClostridialesvadinBB60group (OR = 1.18, 95% CI = 1.06–1.32, p = 2.62e−03), and Peptostreptococcaceae (OR = 1.17, 95% CI = 1.03–1.33, p = 1.90e−02) were related to the higher abundance of DR. In addition, IVW results demonstrated a harmful effect of the host-genetic-driven increase in the genera Eubacterium nodatum group (OR = 1.08, 95% CI = 1.01–1.17, p = 3.53e−02), Actinomyces (OR = 1.15, 95% CI = 1.01–1.32, p = 3.25e−02), Olsenella (OR = 1.10, 95% CI = 1.01–1.20, p = 2.16e−02), Parasutterella (OR = 1.12, 95% CI = 1.01–1.25, p = 3.87e−02), RuminococcaceaeUCG003 (OR = 1.15, 95% CI = 1.00–1.32, p = 4.54e−02), and RuminococcaceaeUCG011 (OR = 1.15, 95% CI = 1.04–1.28, p = 5.83e−03) on the risk of DKD, except for the genus Eisenbergiella (OR = 0.90, 95% CI = 0.82–0.99, p = 3.17e−02) acting as a protective factor.
Diabetic neuropathy
The genetic liability for the family Acidaminococcaceae (OR = 0.62, 95% CI = 0.46–0.84, p = 1.76e−03), family Peptococcaceae (OR = 0.70, 95% CI = 0.54–0.90, p = 5.65e−03), and genus Eubacterium coprostanoligenes group (OR = 0.68, 95% CI = 0.50-0.93, p = 1.61e−02) contributed to a decreased abundance of DNP in the results of IVW analyses. Nevertheless, the higher genetically predicted genera Alistipes (OR = 1.65, 95% CI = 1.18–2.31, p = 3.21e−03), ChristensenellaceaeR_7group (OR = 1.52, 95% CI = 1.03–2.23, p = 3.28e−02), Eggerthella (OR = 1.28, 95% CI = 1.05–1.55, p = 1.42e−02), and RuminococcaceaeUCG013 (OR = 1.35, 95% CI = 1.01–1.82, p = 4.57e−02) were causally associated with a higher abundance of DNP.
The significant results of the IVW analysis for the causal inference of GM on diabetic microvascular complications are presented in Figure 2, where fixed-effect models were applied. No significant q-value of the GM was discovered in FDR correction analysis. Results of all MR analyses (p-value of IVW method < 0.05) along with FDR correction for the IVW method are shown in Supplementary Tables S2–S4 and visual inspection of MR analyses is shown in Supplementary Figures S1–S3.
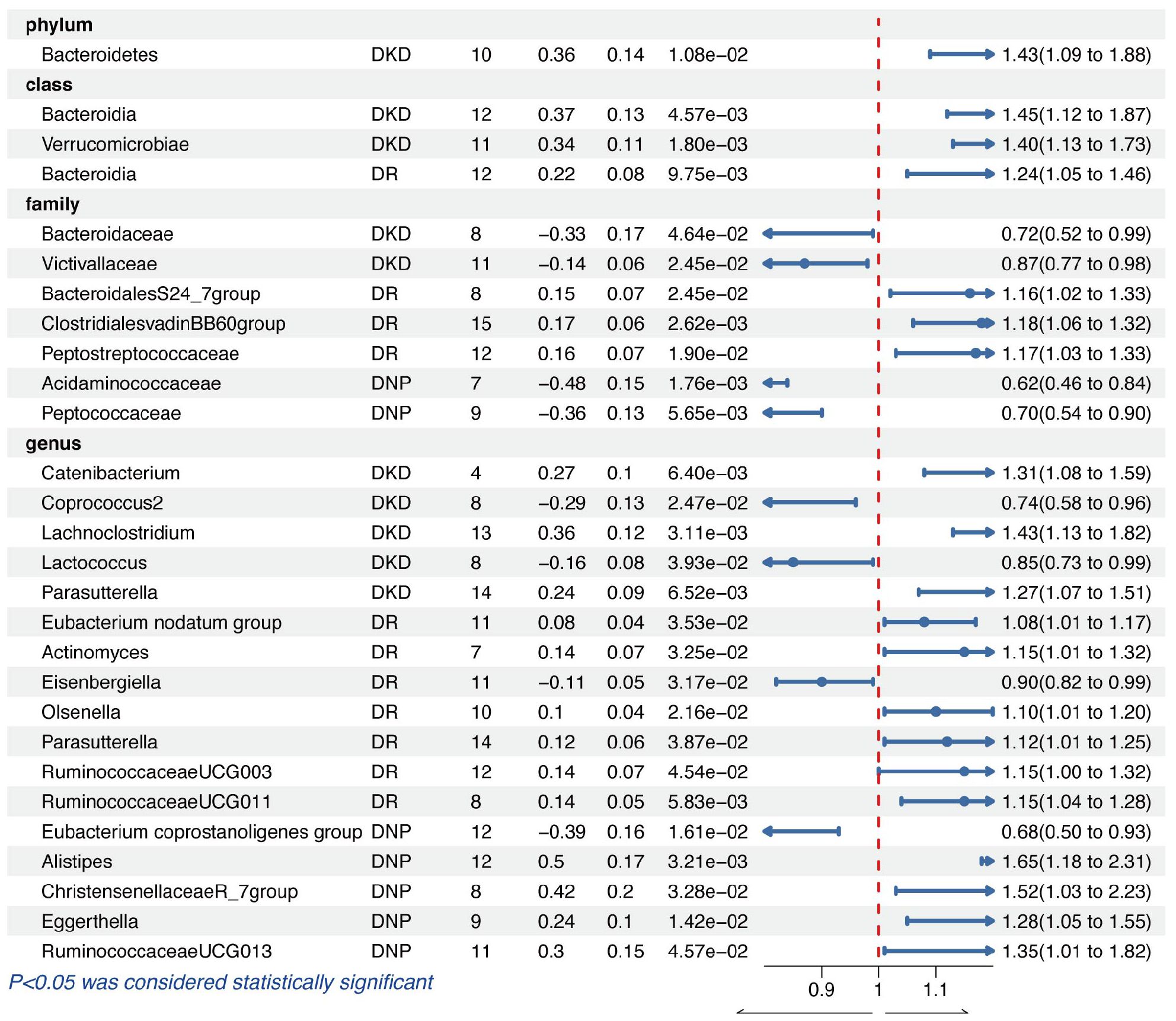
Figure 2 The results of the IVW method for causal inference of GM on diabetic microvascular complications.
Sensitivity analysis
No evidence of heterogeneity (p > 0.05) was observed in Cochran’s Q test, thus resulting in the fixed-effect models being used for the causal inference in the IVW analyses. Moreover, no significant horizontal pleiotropy existed in the MR-PRESSO analysis (global test p > 0.05), and MR-Egger regression intercept analysis was further verified (p > 0.05). Therefore, we could conclude that the results of IVW were authentic in the absence of pleiotropy and heterogeneity. At the same time, the Steiger test indicated no directional causal estimations between GM and diabetic microvascular complications. Summary data of heterogeneity, pleiotropy, and direction analyses are presented in Table 1. The leave-one-out analysis indicated that no single SNP affects the causal inference of GM on diabetic microvascular complications (Supplementary Figures S4–S6).
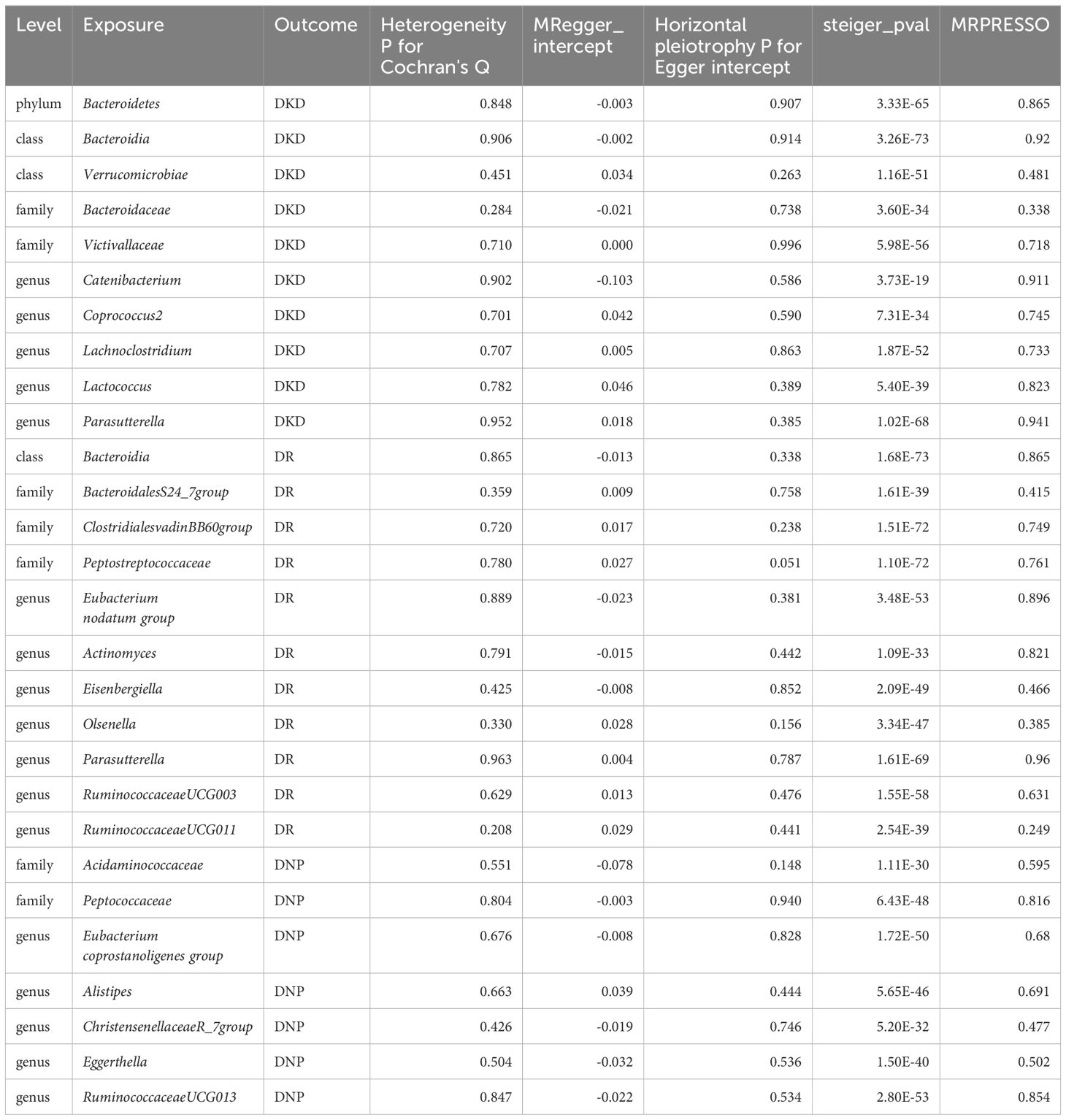
Table 1 Heterogeneity, pleiotropy and directional analyses of GM on diabetic microvascular complications.
Reverse causal effects of diabetic microvascular diseases on GM
When diabetic microvascular complications were set as exposure and GM as outcome, a total of 6 IVs on DKD, 17 IVs on DR, and 4 IVs on DNP were included according to the strict quality selection (Supplementary Table S5).
Figure 3 shows that genetically predicted diabetic microvascular complications were causally associated with some other GM in the IVW results. Summary data of MR analysis between DKD, DR, DNP, and GM are separately presented in Supplementary Tables S6 and S7. DKD was a protective factor for Eubacterium ventriosum group (OR = 0.96, 95% CI = 0.92–1.00, p = 3.73e−02) and a risk factor for Anaerofilum (OR = 1.09, 95% CI = 1.03–1.16, p = 4.84e−03) in the genus level.
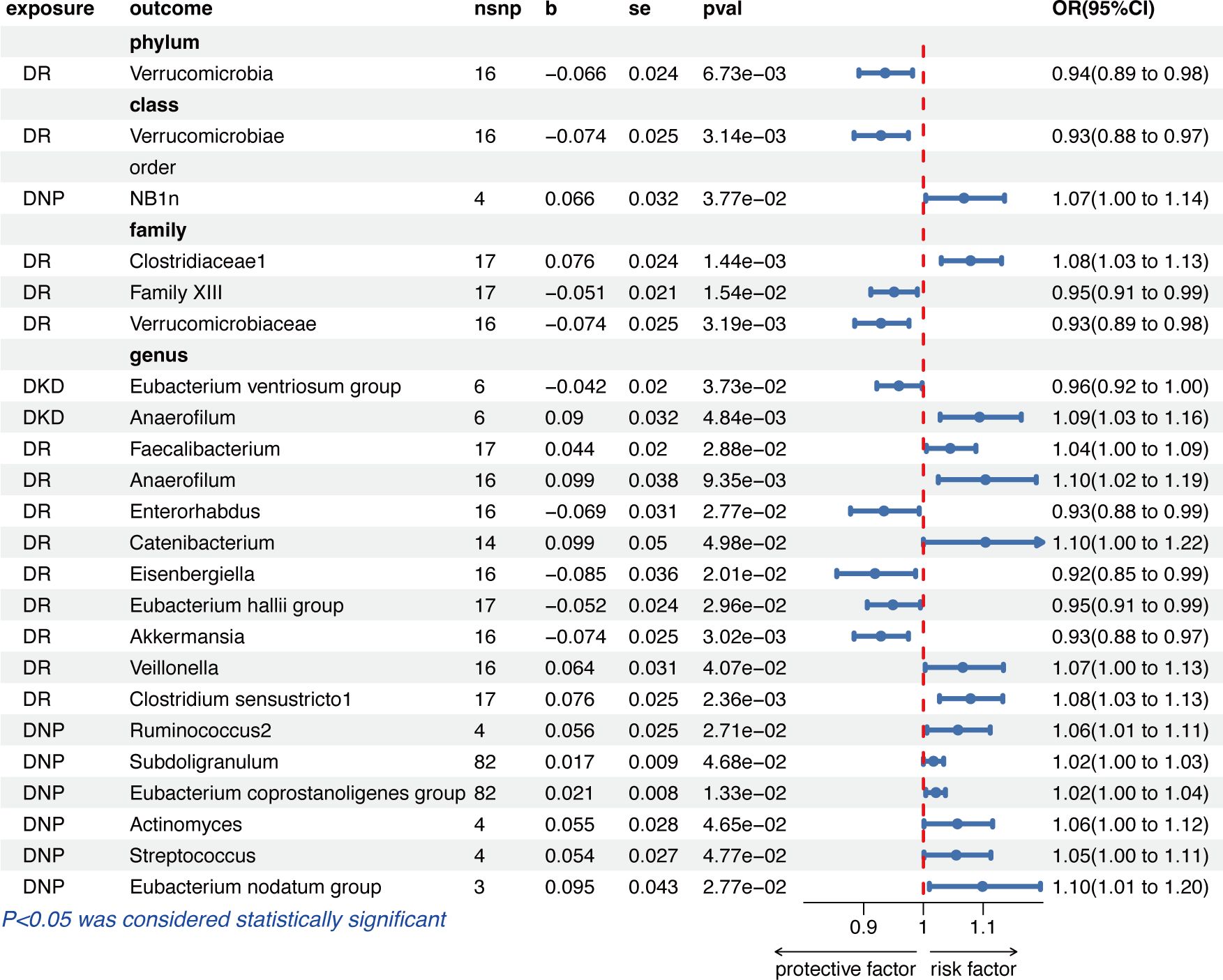
Figure 3 The results of the IVW method given as odds ratio (OR) and 95% confidence interval (CI) for causal inference of diabetic microvascular complications on GM. DR, diabetic retinopathy; DNP, diabetic neuropathy; DKD, diabetic kidney disease.
The IVW results indicated that a higher genetically predicted DR, on the one hand, had a beneficial role on the phylum Verrucomicrobia (OR = 0.94, 95% CI = 0.89–0.98, p = 6.73e−03), the class Verrucomicrobiae (OR = 0.93, 95% CI = 0.88–0.97, p = 3.14e−03), the families Family XIII (OR = 0.95, 95% CI = 0.91–0.99, p = 1.54e−02) and Verrucomicrobiaceae (OR = 0.93, 95% CI = 0.89-0.98, p = 3.19e−03), and the genera Enterorhabdus (OR = 0.93, 95% CI = 0.88–0.99, p = 2.77e−02), Eisenbergiella (OR = 0.92, 95% CI = 0.85–0.99, p = 2.01e−02), Eubacterium hallii group (OR = 0.95, 95% CI = 0.91–0.99, p = 2.96e−02), and Akkermansia (OR = 0.93, 95% CI = 0.88–0.97, p = 3.02e−03); on the other hand, DR had an adverse effect on the family Clostridiaceae1 (OR =1.08, 95% CI = 1.03-1.13, p = 1.44e−03) and the genera Faecalibacterium (OR =1.04, 95% CI = 1.00–1.09, p = 2.88e−02), Anaerofilum (OR =1.10, 95% CI = 1.02–1.19, p = 9.35e−03), Catenibacterium (OR =1.10, 95% CI = 1.00–1.22, p = 4.98e−02), Veillonella (OR =1.07, 95% CI = 1.00–1.13, p = 4.07e−02), and Clostridium sensustricto1 (OR =1.08, 95% CI = 1.03–1.13, p = 2.36e−03).
In the order level, DNP was causally associated with NB1n (OR =1.07, 95% CI = 1.00–1.14, p = 3.77e−02). As for the biological genus classifications, the IVW results indicated that DNP was causally associated with Ruminococcus2 (OR =1.06, 95% CI = 1.01–1.11, p = 2.71e−02), Subdoligranulum (OR =1.02, 95% CI = 1.00–1.03, p = 4.68e−02), Eubacterium coprostanoligenes group (OR =1.02, 95% CI = 1.00–1.04, p = 1.33e−02), Actinomyces (OR =1.06, 95% CI = 1.00–1.12, p = 4.65e−02), Streptococcus (OR =1.05, 95% CI = 1.00–1.11, p = 4.77e−02), and Eubacterium nodatum group (OR = 1.10, 95% CI = 1.01–1.20, p = 2.77e−02).
Cochrane’s Q test, MR-PRESSO, and MR-Egger regression intercept analysis further demonstrated that no evidence of heterogeneity and pleiotropy existed. Meanwhile, the Steiger test showed no directional causal estimations. Summary data of heterogeneity, pleiotropy, and direction analyses are presented in Supplementary Table S8.
Discussion
To the best of our knowledge, this is the first MR study to evaluate the causal inferences between diabetic microvascular complications and GM from a genetic perspective using the summary statistics of diseases from the FinnGen consortium R9 release data and GM from the largest GWAS meta-analysis conducted by the MiBioGen consortium. Our research mainly discussed the diabetic microvascular complications and the published article discussed six complications (including acute complications of diabetes). Although we chose the same GWAS summary data, we have more cases and controls with DR compared with those in the article of PMID 38481313 (39). Moreover, we conducted the Steiger test and reverse MR analysis to explore the genetically predicted GM on the diabetic microvascular complications.
This two-sample MR analysis provided reasonable evidence that 28 genetically predicted abundance of specific GM play non-negligible roles in the occurrence of DKD, DR, and DNP. Similarly, reverse MR analysis indicated that genetic liability to three chronic microvascular complications was causally associated with 23 GM. In particular, the IVW method indicated a bi-directional causal relationship between Eisenbergiella and DR. Amazingly, Eubacterium coprostanoligenes group and DNP presented contradictory effects on the causal inference via IVW analysis. These results could bring about implications for new effective treatments to counter DM-associated chronic microvascular complications. Visual results of the causality inference between GM and diabetic microvascular complications are shown in Figure 4.
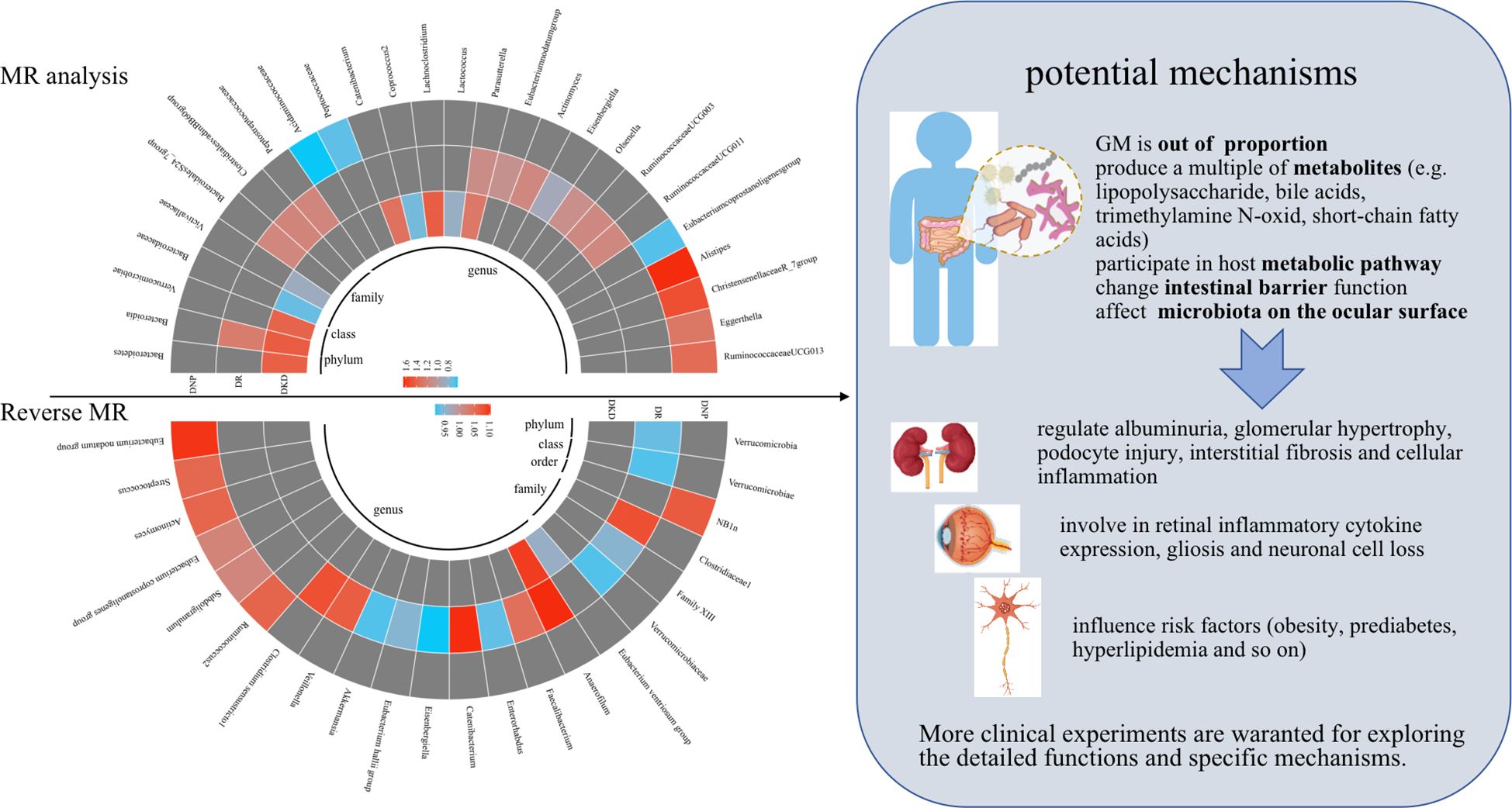
Figure 4 Visual results of the causality inference between GM and diabetic microvascular complications were shown on the left and potential mechanisms were presented on the right. Red represents risk factors, blue represents protective factors, and gray represents negative causal inference between disease and GM. MR, Mendelian randomization.
DKD, a devastating complication of T1D and T2D and a leading cause of end-stage renal disease, occurs in 20% to 50% of patients with DM (40). Few treatment options were available to better stop or delay the onset and progression of DKD. In our study, the family Bacteroidaceae showed an opposite causal inference from its phylum and class levels. Although Catenibacterium, Lachnoclostridium, Coprococcus2, and Lactococcus belonged to Firmicutes, they presented different causal relationships on DKD. Previous studies (41, 42) indicated that the relative abundance of Bacteroidetes decreased and that of Firmicutes increased in DKD compared with healthy individuals and in DM without kidney diseases, which was partly consistent with our results. Abnormal ratios of Bacteroidetes/Firmicutes were decreased in rats with chronic kidney disease compared to controls, which was related to increased acetate- and butyrate-producing bacteria (43). This discrepancy could be attributed to different species, complicated diet habits, and the living environment of people. Our results also found that Verrucomicrobiae and Parasutterella possibly played harmful roles on DKD, whereas Victivallaceae had suggestive protective effects on DKD. A translational human study (44) indicated that Parasutterella abundance was positively associated with obesity and T2D, where fatty acid biosynthesis pathway and L-cysteine might be relevant. Similar to the analysis of GM on patients with DKD, the abundance of Verrucomicrobia and Proteobacteria was relatively increased in patients with DKD in comparison with healthy individuals (45).
Furthermore, a multitude of metabolites produced by GM are essential mediators in the crosstalk between the microbial and host environment, such as lipopolysaccharide, bile acids, trimethylamine N-oxide, and short-chain fatty acids, regulating albuminuria, glomerular hypertrophy, podocyte injury, interstitial fibrosis, and cellular inflammation (46–48).
Bacteroides participated in the synthesis of fatty acids, such as acetate, propionate, and butyrate (49, 50). Olfr-78 receptors did not respond to butyrate but were more sensitive to propionate and acetate. Acetate was in the dysregulation of the dynamic homeostasis of fatty acid metabolism by activating Olfr-78 receptors, which led to tubulointerstitial injury in DKD (51). This might be a potential mechanism by which bacillus-like organisms affect diabetic nephropathy.
DR is the dominant cause of preventable blindness in adults and identified in a third of people with DM (52). Five years after the diagnosis of DM, the number of children and adolescents who progressed to DR increased rapidly (53); thus, annual screening with fundus photography and regulation of GM from diet or lifestyle are especially necessary. Substantial microorganisms are enriched not only on the intestinal but also on the ocular surface. The ocular flora plays an important role in the regulation of ocular immunity and prevention of pathogens, especially in the conjunctiva and cornea (54). Studies have shown that patients with diabetes with diabetic complications have higher conjunctival flora than patients with type 2 diabetes without complications (55). Moreover, studies discovered that the primary composition of microbiota on the ocular surface is Proteobacteria and Actinobacteria (56), accounting for over 87% of all microorganisms that exist in the eye along with Firmicutes (57). Modulation of microbiota through oral feeding of Lactobacillus paracasei secreting Ang-(1-7) could reduce retinal inflammatory cytokine expression and retinal gliosis and block neuronal cell loss (58).
Our results concluded that Bacteroidetes (Bacteroidia and BacteroidalesS24), Firmicutes (including ClostridialesvadinBB60group, Peptostreptococcaceae, and Eubacterium nodatum group), Actinobacteria (including Actinomyces and Olsenella), Parasutterella, and Verrucomicrobia (including RuminococcaceaeUCG011 and RuminococcaceaeUCG003) were the potentially detrimental bacteria, except for Eisenbergiella, acting as the latent protective bacteria on the occurrence and progression of DR via causal inference. Beli et al. discovered a significant increase of Bacteroidetes and Verrucomicrobia on DR with the decrease of acellular capillaries and leukocyte infiltration (59), which is similar to our results. Moreover, Firmicutes was a risk microbiota in the development of DR. The difference from our results was possibly attributed to the mouse models used, being kept in a sterile environment, and fixed diets. Different from a previously reported MR analysis (60), the results of our study were more comprehensive because we chose the updated data in the FinnGen research project and we all agreed that RuminococcaceaeUCG011 was a risk factor for the occurrence and progression of DR. Additionally, IVs are enough for us to conduct reverse MR analyses in our study. Interestingly, we discovered that Parasutterella played a harmful role on the progression of DKD and DR considering the possible reasons of change in fatty acid biosynthesis and L-cysteine in patients with DM (44). However, whether there is a specific reason for their progression other than DNP remains to be further explored.
DNP, which damages the diffuse and focal nervous system, is the most common complication occurring in up to 50% of individuals with DM, and distal symmetric polyneuropathy is the main characteristic (61). It can also affect other organs, resulting in cardiac autonomic neuropathy accompanied by weakness and orthostatic tachycardia, gastrointestinal autonomic dysfunction including early satiety with poor appetite and nausea, esophageal dysfunction with difficulty swallowing, bladder dysfunction, and sudomotor autonomic disturbance, among others (62). The current treatment of DNP is limited, and corresponding studies on GM that explore the ideal intervention targets and preventive strategies are on the rise. Our results indicated that the lower abundance of Acidaminococcaceae, Peptococcaceae, and Eubacterium coprostanoligenes group, and the higher abundance of Alistipes, ChristensenellaceaeR_7group, Eggerthella, and RuminococcaceaeUCG013 were causally associated with appearance and deterioration of DNP. Ma et al. discovered no significance of high blood glucose and painful hypersensitivity in animals induced by streptozotocin as major depletion of GM in comparison with controls (63). However, as far as we know, there are no relevant studies observed between our chosen GM and DNP, but they are associated with relative risk factors. The relationship between gut dysbiosis, neuronal damage, and dyskinesia is not yet fully understood, but GM clearly plays an important role in maintaining the function of the enteric nervous system (64). In a recent study, Nyavor et al. (65) found a reduction in inhibitory neuromuscular transmission and a loss of inhibitory motor neurons in muscles in rats fed a high-fat diet. High-fat diets also lead to microbiological imbalances in the bacterial flora, such as increased numbers of Aspergillus, Lactobacillus, and Bifidobacterium. These changes are associated with neuropathy and intestinal motility disorders.
Insulin resistance is closely linked to the onset and progression of DNP (66, 67). Yuan et al. indicated that Firmicutes was decreased and Bacteroidetes was increased in insulin-resistant subjects compared with insulin-sensitive individuals (68). Acidaminococcaceae and Peptococcaceae belonged to Firmicutes, whereas Alistipes was part of Bacteroidetes. Furthermore, Peptococcaceae was significantly more prevalent and Alistipes was less prevalent in individuals with insulin resistance via influencing serum concentrations of angiopoietin-like 4 and adropin, which was consistent with our causal inference of GM on DNP. ChristensenellaceaeR_7group was positively correlated with obesity and would be a potential therapeutic target of traditional Chinese medicine to relieve obesity (69). Although no research observed the relationship between RuminococcaceaeUCG013 and DNP, a study demonstrated that abnormalities of Ruminococcaceae caused cognitive dysfunction in DNP rats (70). In general, the causality of GM found in our study needs to be further verified.
The above positive strains showed no causal inference in reverse MR analyses except for Eisenbergiella and Eubacterium coprostanoligenes group. Eisenbergiella showed a bi-directional causal relationship in the reverse MR analysis. A plethora of studies revealed that alterations of the abundance of Eisenbergiella led to numerous diseases, including multiple sclerosis, rheumatoid arthritis, and autism (71–73). Upregulated Eisenbergiella affected fatty acid metabolism by the production of short-chain fatty acids to reduce obesity, an important risk factor for DM. Therefore, we can conclude that it could be a non-invasive biomarker or a potential target for the treatment of patients with DR. Our results implied an opposite mutual causal relationship between Eubacterium coprostanoligenes group and DNP.
Although no studies of Eubacterium coprostanoligenes group were relevant to neuropathy, some studies indicated improvement of dyslipidemia induced by high-fat diet (74) and amelioration of fasting blood glucose, hemoglobin, serum levels of endotoxin, interlukin-6, tumor necrosis factor-α, and interlukin-1β in prediabetes (75). Hyperlipidemia and prediabetes are risk factors for DNP (66, 76). As a result, we recognize that Eubacterium coprostanoligenes group could possibly mediate the beneficial effects of DNP. MR analyses for diabetic microvascular complications on GM substantiated positive effects on the other GM. However, OR generally ranged from 0.9 to 1.1, demonstrating little causal relationship. These strains were still novel diagnostic biomarkers of diseases and therapeutic breakthrough.
Inevitably, it should be noted that our study has some limitations that could have affected the results. Firstly, the GWAS included only individuals of European descent; thus, the generation of our findings to other races is limited. Secondly, because the summary statistics instead of raw data were utilized in the analysis, microvascular complications caused by T1DM or T2DM were lack of specific information in the FinnGen database, which restricted the further subgroup analyses. Thirdly, a relatively lenient GWAS significance threshold (p < 1e−05) was set for more genetic variations in IVs in order to perform horizontal pleiotropy detection and sensitivity analysis. Thus, FDR correction was utilized to lower the probability of being false positive. Fourthly, GM together with its metabolites and by-products played important roles on the onset and progression of diabetic microvascular complications. However, our study only explored the causal relationship between microvascular complications and GM. Therefore, it would be helpful to perform causal association between GM and diabetic microvascular complications in diverse European and non-European populations for more generalizability. Multivariable MR analysis can also be considered for the corresponding metabolites in future endeavors. Lastly, although amplicon sequence variant-level analysis provided better resolution and more accurate results in the 16S rRNA gene-based microbiota studies in comparison with taxa-level analysis, we only obtained datasets of taxa.
Conclusion
The present study gave credence to the concept that GM may be a promising therapy in diabetic microvascular complications. Further experiments of GM therapy on diabetic microvascular complications are warranted to elucidate their effects and specific mechanisms.
Data availability statement
The original contributions presented in the study are included in the article/Supplementary Material. Further inquiries can be directed to the corresponding author.
Ethics statement
All the GWAS summary statistics of diabetic complications in this study were acquired from the FinnGen research project (https://r9.finngen.fi/). The studies were conducted in accordance with the local legislation and institutional requirements. Written informed consent for participation was not required from the participants or the participants’ legal guardians/next of kin. The summary-level data involved in this study are free of identified and publicly available for download. Respective institutions have approved the ethics statement of Each GWAS in this study. There was no individual-level data in this study, thus, new ethical review board approval was unnecessary.
Author contributions
PZ: Conceptualization, Methodology, Validation, Visualization, Writing – original draft, Writing – review & editing. ZH: Data curation, Software, Writing – original draft. WX: Methodology, Writing – original draft. YC: Formal analysis, Writing – review & editing. ZZ: Supervision, Visualization, Writing – review & editing. JY: Funding acquisition, Supervision, Validation, Writing – review & editing, Conceptualization.
Funding
The author(s) declare financial support was received for the research, authorship, and/or publication of this article. The present study was supported by the National Natural Science Foundation of China (grant nos. 82174293 and 82205025) and Jiangsu Province Postgraduate Research and Innovation Program (grant no. KYCX23_2173).
Conflict of interest
The authors declare that the research was conducted in the absence of any commercial or financial relationships that could be construed as a potential conflict of interest.
Publisher’s note
All claims expressed in this article are solely those of the authors and do not necessarily represent those of their affiliated organizations, or those of the publisher, the editors and the reviewers. Any product that may be evaluated in this article, or claim that may be made by its manufacturer, is not guaranteed or endorsed by the publisher.
Supplementary material
The Supplementary Material for this article can be found online at: https://www.frontiersin.org/articles/10.3389/fendo.2024.1364280/full#supplementary-material
Supplementary Figure 1 | Scatter plots for the causal association between (A) Bacteroidetes, (B) Bacteroidia, (C) Verrucomicrobiae, (D) Bacteroidaceae, (E) Victivallaceae, (F) Catenibacterium, (G) Coprococcus2, (H) Lachnoclostridium, (I) Lactococcus, and (J) Parasutterella and DKD. The slope of each line corresponds to the estimated MR effect in different models.
Supplementary Figure 2 | Scatter plots for the causal association between (A) Bacteroidia, (B) BacteroidalesS24_7group, (C) ClostridialesvadinBB60group, (D) Peptostreptococcaceae, (E) Eubacterium nodatum group, (F) Actinomyces, (G) Eisenbergiella, (H) Olsenella, (I) Parasutterella, (J) RuminococcaceaeUCG003, and (K) RuminococcaceaeUCG011 and DR. The slope of each line corresponds to the estimated MR effect in different models.
Supplementary Figure 3 | Scatter plots for the causal association between (A) Acidaminococcaceae, (B) Peptococcaceae, (C) Eubacterium coprostanoligenes group, (D) Alistipes, (E) ChristensenellaceaeR_7group, (F) Eggerthella, and (G) RuminococcaceaeUCG013 and DNP. The slope of each line corresponds to the estimated MR effect in different models.
Supplementary Figure 4 | MR leave-one-out sensitivity analysis for (A) Bacteroidetes, (B) Bacteroidia, (C) Verrucomicrobiae, (D) Bacteroidaceae, (E) Victivallaceae, (F) Catenibacterium, (G) Coprococcus2, (H) Lachnoclostridium, (I) Lactococcus, and (J) Parasutterella on DKD. Calculate the MR results of the remaining IVs after removing the IVs one by one.
Supplementary Figure 5 | MR leave-one-out sensitivity analysis for (A) Bacteroidia, (B) BacteroidalesS24_7group, (C) ClostridialesvadinBB60group, (D) Peptostreptococcaceae, (E) Eubacterium nodatum group, (F) Actinomyces, (G) Eisenbergiella, (H) Olsenella, (I) Parasutterella, (J) RuminococcaceaeUCG003, and (K) RuminococcaceaeUCG011 on DR. Calculate the MR results of the remaining IVs after removing the IVs one by one.
Supplementary Figure 6 | MR leave-one-out sensitivity analysis for (A) Acidaminococcaceae, (B) Peptococcaceae, (C) Eubacterium coprostanoligenes group, (D) Alistipes, (E) ChristensenellaceaeR_7group, (F) Eggerthella, and (G) RuminococcaceaeUCG013 on DNP. Calculate the MR results of the remaining IVs after removing the IVs one by one.
References
1. Mauricio D, Gratacos M, Franch-Nadal J. Diabetic microvascular disease in non-classical beds: the hidden impact beyond the retina, the kidney, and the peripheral nerves. Cardiovasc Diabetol. (2023) 22:314. doi: 10.1186/s12933-023-02056-3
2. Barrett EJ, Liu Z, Khamaisi M, King GL, Klein R, Klein BEK, et al. Diabetic microvascular disease: an endocrine society scientific statement. J Clin Endocrinol Metab. (2017) 102:4343–410. doi: 10.1210/jc.2017-01922
3. Lyssenko V, Vaag A. Genetics of diabetes-associated microvascular complications. Diabetologia. (2023) 66:1601–13. doi: 10.1007/s00125-023-05964-x
4. American Diabetes A. 11. Microvascular complications and foot care: standards of medical care in diabetes-2021. Diabetes Care. (2021) 44:S151–67. doi: 10.2337/dc21-S011
5. American Diabetes A. Economic costs of diabetes in the U.S. in 2017. Diabetes Care. (2018) 41:917–28. doi: 10.2337/dci18-0007
6. Cho NH, Shaw JE, Karuranga S, Huang Y, da Rocha Fernandes JD, Ohlrogge AW, et al. IDF Diabetes Atlas: Global estimates of diabetes prevalence for 2017 and projections for 2045. Diabetes Res Clin Pract. (2018) 138:271–81. doi: 10.1016/j.diabres.2018.02.023
7. Li Y, Teng D, Shi X, Qin G, Qin Y, Quan H, et al. Prevalence of diabetes recorded in mainland China using 2018 diagnostic criteria from the American Diabetes Association: national cross sectional study. BMJ. (2020) 369:m997. doi: 10.1136/bmj.m997
8. Komorowsky CV, Brosius FC 3rd, Pennathur S, Kretzler M. Perspectives on systems biology applications in diabetic kidney disease. J Cardiovasc Transl Res. (2012) 5:491–508. doi: 10.1007/s12265-012-9382-7
9. Sekirov I, Russell SL, Antunes LC, Finlay BB. Gut microbiota in health and disease. Physiol Rev. (2010) 90:859–904. doi: 10.1152/physrev.00045.2009
10. Sharma S, Tripathi P. Gut microbiome and type 2 diabetes: where we are and where to go? J Nutr Biochem. (2019) 63:101–8. doi: 10.1016/j.jnutbio.2018.10.003
11. Tanase DM, Gosav EM, Neculae E, Costea CF, Ciocoiu M, Hurjui LL, et al. Role of gut microbiota on onset and progression of microvascular complications of type 2 diabetes (T2DM). Nutrients. (2020) 12:3719. doi: 10.3390/nu12123719
12. David LA, Maurice CF, Carmody RN, Gootenberg DB, Button JE, Wolfe BE, et al. Diet rapidly and reproducibly alters the human gut microbiome. Nature. (2014) 505:559–63. doi: 10.1038/nature12820
13. Yatsunenko T, Rey FE, Manary MJ, Trehan I, Dominguez-Bello MG, Contreras M, et al. Human gut microbiome viewed across age and geography. Nature. (2012) 486:222–7. doi: 10.1038/nature11053
14. Wu H, Esteve E, Tremaroli V, Khan MT, Caesar R, Manneras-Holm L, et al. Metformin alters the gut microbiome of individuals with treatment-naive type 2 diabetes, contributing to the therapeutic effects of the drug. Nat Med. (2017) 23:850–8. doi: 10.1038/nm.4345
15. Zhao H, Yang CE, Liu T, Zhang MX, Niu Y, Wang M, et al. The roles of gut microbiota and its metabolites in diabetic nephropathy. Front Microbiol. (2023) 14:1207132. doi: 10.3389/fmicb.2023.1207132
16. Cai Y, Kang Y. Gut microbiota and metabolites in diabetic retinopathy: Insights into pathogenesis for novel therapeutic strategies. BioMed Pharmacother. (2023) 164:114994. doi: 10.1016/j.biopha.2023.114994
17. Brock C, Softeland E, Gunterberg V, Frokjaer JB, Lelic D, Brock B, et al. Diabetic autonomic neuropathy affects symptom generation and brain-gut axis. Diabetes Care. (2013) 36:3698–705. doi: 10.2337/dc13-0347
18. Mazala-de-Oliveira T, Jannini de Sa YAP, Carvalho VF. Impact of gut-peripheral nervous system axis on the development of diabetic neuropathy. Mem Inst Oswaldo Cruz. (2023) 118:e220197. doi: 10.1590/0074-02760220197
19. Emdin CA, Khera AV, Kathiresan S. Mendelian randomization. JAMA. (2017) 318:1925–6. doi: 10.1001/jama.2017.17219
20. Kurilshikov A, Medina-Gomez C, Bacigalupe R, Radjabzadeh D, Wang J, Demirkan A, et al. Large-scale association analyses identify host factors influencing human gut microbiome composition. Nat Genet. (2021) 53:156–65. doi: 10.1038/s41588-020-00763-1
21. Goodrich JK, Davenport ER, Beaumont M, Jackson MA, Knight R, Ober C, et al. Genetic determinants of the gut microbiome in UK twins. Cell Host Microbe. (2016) 19:731–43. doi: 10.1016/j.chom.2016.04.017
22. Luo M, Sun M, Wang T, Zhang S, Song X, Liu X, et al. Gut microbiota and type 1 diabetes: a two-sample bidirectional Mendelian randomization study. Front Cell Infect Microbiol. (2023) 13:1163898. doi: 10.3389/fcimb.2023.1163898
23. Li H, Li C. Causal relationship between gut microbiota and type 2 diabetes: a two-sample Mendelian randomization study. Front Microbiol. (2023) 14:1184734. doi: 10.3389/fmicb.2023.1184734
24. Sanderson E, Glymour MM, Holmes MV, Kang H, Morrison J, Munafo MR, et al. Mendelian randomization. Nat Rev Methods Primers. (2022) 2:6. doi: 10.1038/s43586-021-00092-5
25. Verbanck M, Chen CY, Neale B, Do R. Detection of widespread horizontal pleiotropy in causal relationships inferred from Mendelian randomization between complex traits and diseases. Nat Genet. (2018) 50:693–8. doi: 10.1038/s41588-018-0099-7
26. Papadimitriou N, Dimou N, Tsilidis KK, Banbury B, Martin RM, Lewis SJ, et al. Physical activity and risks of breast and colorectal cancer: a Mendelian randomisation analysis. Nat Commun. (2020) 11:597. doi: 10.1038/s41467-020-14389-8
27. He B, Lyu Q, Yin L, Zhang M, Quan Z, Ou Y. Depression and osteoporosis: A mendelian randomization study. Calcif Tissue Int. (2021) 109:675–84. doi: 10.1007/s00223-021-00886-5
28. Bowden J, Davey Smith G, Haycock PC, Burgess S. Consistent estimation in mendelian randomization with some invalid instruments using a weighted median estimator. Genet Epidemiol. (2016) 40:304–14. doi: 10.1002/gepi.21965
29. Burgess S, Butterworth A, Thompson SG. Mendelian randomization analysis with multiple genetic variants using summarized data. Genet Epidemiol. (2013) 37:658–65. doi: 10.1002/gepi.21758
30. Bowden J, Del Greco MF, Minelli C, Davey Smith G, Sheehan NA, Thompson JR. Assessing the suitability of summary data for two-sample Mendelian randomization analyses using MR-Egger regression: the role of the I2 statistic. Int J Epidemiol. (2016) 45:1961–74. doi: 10.1093/ije/dyw220
31. Bowden J, Davey Smith G, Burgess S. Mendelian randomization with invalid instruments: effect estimation and bias detection through Egger regression. Int J Epidemiol. (2015) 44:512–25. doi: 10.1093/ije/dyv080
32. Burgess S, Thompson SG. Interpreting findings from Mendelian randomization using the MR-Egger method. Eur J Epidemiol. (2017) 32:377–89. doi: 10.1007/s10654-017-0255-x
33. Hartwig FP, Davey Smith G, Bowden J. Robust inference in summary data Mendelian randomization via the zero modal pleiotropy assumption. Int J Epidemiol. (2017) 46:1985–98. doi: 10.1093/ije/dyx102
34. Walker VM, Davies NM, Hemani G, Zheng J, Haycock PC, Gaunt TR, et al. Using the MR-Base platform to investigate risk factors and drug targets for thousands of phenotypes. Wellcome Open Res. (2019) 4:113. doi: 10.12688/wellcomeopenres
35. Hemani G, Tilling K, Davey Smith G. Orienting the causal relationship between imprecisely measured traits using GWAS summary data. PloS Genet. (2017) 13:e1007081. doi: 10.1371/journal.pgen.1007081
36. Yu H, Wan X, Yang M, Xie J, Xu K, Wang J, et al. A large-scale causal analysis of gut microbiota and delirium: A Mendelian randomization study. J Affect Disord. (2023) 329:64–71. doi: 10.1016/j.jad.2023.02.078
37. Storey JD, Tibshirani R. Statistical significance for genomewide studies. Proc Natl Acad Sci USA. (2003) 100:9440–5. doi: 10.1073/pnas.1530509100
38. Skrivankova VW, Richmond RC, Woolf BAR, Yarmolinsky J, Davies NM, Swanson SA, et al. Strengthening the reporting of observational studies in epidemiology using mendelian randomization: the STROBE-MR statement. JAMA. (2021) 326:1614–21. doi: 10.1001/jama.2021.18236
39. Wang J, Teng M, Feng R, Su X, Xu K, Wang J, et al. Large-scale causal analysis of gut microbiota and six common complications of diabetes: a mendelian randomization study. Diabetol Metab Syndr. (2024) 16:66. doi: 10.1186/s13098-024-01298-9
40. Selby NM, Taal MW. An updated overview of diabetic nephropathy: Diagnosis, prognosis, treatment goals and latest guidelines. Diabetes Obes Metab. (2020) 22(Suppl 1):3–15. doi: 10.1111/dom.14007
41. Tao S, Li L, Li L, Liu Y, Ren Q, Shi M, et al. Understanding the gut-kidney axis among biopsy-proven diabetic nephropathy, type 2 diabetes mellitus and healthy controls: an analysis of the gut microbiota composition. Acta Diabetol. (2019) 56:581–92. doi: 10.1007/s00592-019-01316-7
42. Que Y, Cao M, He J, Zhang Q, Chen Q, Yan C, et al. Gut bacterial characteristics of patients with type 2 diabetes mellitus and the application potential. Front Immunol. (2021) 12:722206. doi: 10.3389/fimmu.2021.722206
43. Yang T, Santisteban MM, Rodriguez V, Li E, Ahmari N, Carvajal JM, et al. Gut dysbiosis is linked to hypertension. Hypertension. (2015) 65:1331–40. doi: 10.1161/HYPERTENSIONAHA.115.05315
44. Henneke L, Schlicht K, Andreani NA, Hollstein T, Demetrowitsch T, Knappe C, et al. A dietary carbohydrate - gut Parasutterella - human fatty acid biosynthesis metabolic axis in obesity and type 2 diabetes. Gut Microbes. (2022) 14:2057778. doi: 10.1080/19490976.2022.2057778
45. Lin JR, Wang ZT, Sun JJ, Yang YY, Li XX, Wang XR, et al. Gut microbiota and diabetic kidney diseases: Pathogenesis and therapeutic perspectives. World J Diabetes. (2022) 13:308–18. doi: 10.4239/wjd.v13.i4.308
46. Blander JM, Longman RS, Iliev ID, Sonnenberg GF, Artis D. Regulation of inflammation by microbiota interactions with the host. Nat Immunol. (2017) 18:851–60. doi: 10.1038/ni.3780
47. Chen PP, Zhang JX, Li XQ, Li L, Wu QY, Liu L, et al. Outer membrane vesicles derived from gut microbiota mediate tubulointerstitial inflammation: a potential new mechanism for diabetic kidney disease. Theranostics. (2023) 13:3988–4003. doi: 10.7150/thno.84650
48. Dai Y, Quan J, Xiong L, Luo Y, Yi B. Probiotics improve renal function, glucose, lipids, inflammation and oxidative stress in diabetic kidney disease: a systematic review and meta-analysis. Ren Fail. (2022) 44:862–80. doi: 10.1080/0886022X.2022.2079522
49. Stilling RM, van de Wouw M, Clarke G, Stanton C, Dinan TG, Cryan JF. The neuropharmacology of butyrate: The bread and butter of the microbiota-gut-brain axis? Neurochem Int. (2016) 99:110–32. doi: 10.1016/j.neuint.2016.06.011
50. Wang X, Cai Z, Wang Q, Wu C, Sun Y, Wang Z, et al. Bacteroides methylmalonyl-CoA mutase produces propionate that promotes intestinal goblet cell differentiation and homeostasis. Cell Host Microbe. (2024) 32:63–78 e7. doi: 10.1016/j.chom.2023.11.005
51. Zaky A, Glastras SJ, Wong MYW, Pollock A, Saad S. The role of the gut microbiome in diabetes and obesity-related kidney disease. Int J Mol Sci. (2021) 22:9641. doi: 10.3390/ijms22179641
52. Cheung N, Mitchell P, Wong TY. Diabetic retinopathy. Lancet. (2010) 376:124–36. doi: 10.1016/S0140-6736(09)62124-3
53. Cioana M, Deng J, Nadarajah A, Hou M, Qiu Y, Chen SSJ, et al. Global prevalence of diabetic retinopathy in pediatric type 2 diabetes: A systematic review and meta-analysis. JAMA Netw Open. (2023) 6:e231887. doi: 10.1001/jamanetworkopen.2023.1887
54. Ham B, Hwang HB, Jung SH, Chang S, Kang KD, Kwon MJ. Distribution and diversity of ocular microbial communities in diabetic patients compared with healthy subjects. Curr Eye Res. (2018) 43:314–24. doi: 10.1080/02713683.2017.1406528
55. St Leger AJ, Caspi RR. Visions of eye commensals: the known and the unknown about how the microbiome affects eye disease. Bioessays. (2018) 40:e1800046. doi: 10.1002/bies.201800046
56. Ozkan J, Willcox M, Wemheuer B, Wilcsek G, Coroneo M, Thomas T. Biogeography of the human ocular microbiota. Ocul Surf. (2019) 17:111–8. doi: 10.1016/j.jtos.2018.11.005
58. Verma A, Xu K, Du T, Zhu P, Liang Z, Liao S, et al. Expression of human ACE2 in lactobacillus and beneficial effects in diabetic retinopathy in mice. Mol Ther Methods Clin Dev. (2019) 14:161–70. doi: 10.1016/j.omtm.2019.06.007
59. Beli E, Yan Y, Moldovan L, Vieira CP, Gao R, Duan Y, et al. Restructuring of the gut microbiome by intermittent fasting prevents retinopathy and prolongs survival in db/db mice. Diabetes. (2018) 67:1867–79. doi: 10.2337/db18-0158
60. Liu K, Zou J, Fan H, Hu H, You Z. Causal effects of gut microbiota on diabetic retinopathy: A Mendelian randomization study. Front Immunol. (2022) 13:930318. doi: 10.3389/fimmu.2022.930318
61. Callaghan BC, Price RS, Chen KS, Feldman EL. The importance of rare subtypes in diagnosis and treatment of peripheral neuropathy: A review. JAMA Neurol. (2015) 72:1510–8. doi: 10.1001/jamaneurol.2015.2347
62. Feldman EL, Callaghan BC, Pop-Busui R, Zochodne DW, Wright DE, Bennett D, et al. Diabetic neuropathy. Nat Rev Dis Primers. (2019) 5:42. doi: 10.1038/s41572-019-0092-1
63. Ma P, Mo R, Liao H, Qiu C, Wu G, Yang C, et al. Gut microbiota depletion by antibiotics ameliorates somatic neuropathic pain induced by nerve injury, chemotherapy, and diabetes in mice. J Neuroinflamm. (2022) 19:169. doi: 10.1186/s12974-022-02523-w
64. Heiss CN, Olofsson LE. The role of the gut microbiota in development, function and disorders of the central nervous system and the enteric nervous system. J Neuroendocrinol. (2019) 31:e12684. doi: 10.1111/jne.12684
65. Nyavor Y, Brands CR, May G, Kuther S, Nicholson J, Tiger K, et al. High-fat diet-induced alterations to gut microbiota and gut-derived lipoteichoic acid contributes to the development of enteric neuropathy. Neurogastroenterol Motil. (2020) 32:e13838. doi: 10.1111/nmo.13838
66. Handzlik MK, Gengatharan JM, Frizzi KE, McGregor GH, Martino C, Rahman G, et al. Insulin-regulated serine and lipid metabolism drive peripheral neuropathy. Nature. (2023) 614:118–24. doi: 10.1038/s41586-022-05637-6
67. Papanas N, Ziegler D. Risk factors and comorbidities in diabetic neuropathy: an update 2015. Rev Diabetes Stud. (2015) 12:48–62. doi: 10.1900/RDS.2015.12.48
68. Yuan X, Chen R, Zhang Y, Lin X, Yang X, McCormick KL. Gut microbiota of chinese obese children and adolescents with and without insulin resistance. Front Endocrinol (Lausanne). (2021) 12:636272. doi: 10.3389/fendo.2021.636272
69. Gong S, Ye T, Wang M, Wang M, Li Y, Ma L, et al. Traditional chinese medicine formula kang shuai lao pian improves obesity, gut dysbiosis, and fecal metabolic disorders in high-fat diet-fed mice. Front Pharmacol. (2020) 11:297. doi: 10.3389/fphar.2020.00297
70. Huang W, Lin Z, Sun A, Deng J, Manyande A, Xiang H, et al. The role of gut microbiota in diabetic peripheral neuropathy rats with cognitive dysfunction. Front Microbiol. (2023) 14:1156591. doi: 10.3389/fmicb.2023.1156591
71. iMSMS Consortium. Gut microbiome of multiple sclerosis patients and paired household healthy controls reveal associations with disease risk and course. Cell. (2022) 185:3467–86.e16. doi: 10.1016/j.cell.2022.08.021
72. Yu D, Du J, Pu X, Zheng L, Chen S, Wang N, et al. The gut microbiome and metabolites are altered and interrelated in patients with rheumatoid arthritis. Front Cell Infect Microbiol. (2021) 11:763507. doi: 10.3389/fcimb.2021.763507
73. Zou R, Xu F, Wang Y, Duan M, Guo M, Zhang Q, et al. Changes in the gut microbiota of children with autism spectrum disorder. Autism Res. (2020) 13:1614–25. doi: 10.1002/aur.2358
74. Wei W, Jiang W, Tian Z, Wu H, Ning H, Yan G, et al. Fecal g. Streptococcus and g. Eubacterium_coprostanoligenes_group combined with sphingosine to modulate the serum dyslipidemia in high-fat diet mice. Clin Nutr. (2021) 40:4234–45. doi: 10.1016/j.clnu.2021.01.031
75. Li L, Bao J, Chang Y, Wang M, Chen B, Yan F. Gut microbiota may mediate the influence of periodontitis on prediabetes. J Dent Res. (2021) 100:1387–96. doi: 10.1177/00220345211009449
Keywords: diabetic kidney disease, diabetic microvascular complications, diabetic neuropathy, diabetic retinopathy, Mendelian randomization
Citation: Zhou P, Hao Z, Chen Y, Zhang Z, Xu W and Yu J (2024) Association between gut microbiota and diabetic microvascular complications: a two-sample Mendelian randomization study. Front. Endocrinol. 15:1364280. doi: 10.3389/fendo.2024.1364280
Received: 02 January 2024; Accepted: 27 June 2024;
Published: 02 August 2024.
Edited by:
Guojun Wu, Rutgers, The State University of New Jersey, United StatesReviewed by:
Chenglin Sun, Jilin University, ChinaGeorgia Damoraki, National and Kapodistrian University of Athens, Greece
Jonathan Samuel Chávez-Iñiguez, University of Guadalajara, Mexico
Copyright © 2024 Zhou, Hao, Chen, Zhang, Xu and Yu. This is an open-access article distributed under the terms of the Creative Commons Attribution License (CC BY). The use, distribution or reproduction in other forums is permitted, provided the original author(s) and the copyright owner(s) are credited and that the original publication in this journal is cited, in accordance with accepted academic practice. No use, distribution or reproduction is permitted which does not comply with these terms.
*Correspondence: Jiangyi Yu, eWp5MjAyMTA1QG5qdWNtLmVkdS5jbg==; Weilong Xu, MjAyMTUxMzBAbmp1Y20uZWR1LmNu