- 1Department of Biology, Ecology and Earth Sciences, University of Calabria, Rende, Italy
- 2Biological and Environmental Sciences and Engineering Division (BESE), King Abdullah University of Science and Technology (KAUST), Thuwal, Saudi Arabia
- 3SDAIA-KAUST Center of Excellence in Data Science and Artificial Intelligence, Thuwal, Saudi Arabia
- 4Institute of Chemical Biology, Ilia State University, Tbilisi, Georgia
- 5Scientific Direction, IRCCS INRCA, Ancona, Italy
- 6Diabetology Unit, IRCCS INRCA, Ancona, Italy
- 7Unit of Geriatric Medicine, IRCCS INRCA, Cosenza, Italy
- 8Department of Pharmacy, Health and Nutritional Sciences, University of Calabria, Rende, Italy
- 9Department of Clinical and Molecular Sciences, Università Politecnica delle Marche, Ancona, Italy
- 10Laboratory Medicine Unit, Azienda Ospedaliero Universitaria delle Marche, Ancona, Italy
- 11Geriatria, Accettazione geriatrica e Centro di ricerca per l’invecchiamento, IRCCS INRCA, Ancona, Italy
- 12Department of Medical and Surgical Science, University of Bologna, Bologna, Italy
- 13General Direction, IRCCS INRCA, Ancona, Italy
- 14Clinic of Laboratory and Precision Medicine, IRCCS INRCA, Ancona, Italy
Background: Prognostic risk stratification in older adults with type 2 diabetes (T2D) is important for guiding decisions concerning advance care planning.
Materials and methods: A retrospective longitudinal study was conducted in a real-world sample of older diabetic patients afferent to the outpatient facilities of the Diabetology Unit of the IRCCS INRCA Hospital of Ancona (Italy). A total of 1,001 T2D patients aged more than 70 years were consecutively evaluated by a multidimensional geriatric assessment, including physical performance evaluated using the Short Physical Performance Battery (SPPB). The mortality was assessed during a 5-year follow-up. We used the automatic machine-learning (AutoML) JADBio platform to identify parsimonious mathematical models for risk stratification.
Results: Of 977 subjects included in the T2D cohort, the mean age was 76.5 (SD: 4.5) years and 454 (46.5%) were men. The mean follow-up time was 53.3 (SD:15.8) months, and 209 (21.4%) patients died by the end of the follow-up. The JADBio AutoML final model included age, sex, SPPB, chronic kidney disease, myocardial ischemia, peripheral artery disease, neuropathy, and myocardial infarction. The bootstrap-corrected concordance index (c-index) for the final model was 0.726 (95% CI: 0.687–0.763) with SPPB ranked as the most important predictor. Based on the penalized Cox regression model, the risk of death per unit of time for a subject with an SPPB score lower than five points was 3.35 times that for a subject with a score higher than eight points (P-value <0.001).
Conclusion: Assessment of physical performance needs to be implemented in clinical practice for risk stratification of T2D older patients.
1 Introduction
The incidence of type 2 diabetes (T2D) has reached epidemic proportions globally, and it is rapidly growing resulting in a great clinical and economic burden for the healthcare systems over the world (1, 2). T2D is managed through the implementation of complications prevention plans based on lifestyle and dietary modifications usually followed by metformin monotherapy and, when necessary, the further addition of an increasingly complex array of therapies, including oral and injectable medications (1, 2). These prevention plans are aimed at reducing the microvascular (e.g., retinopathy, neuropathy, nephropathy) and macrovascular (e.g., ischemic heart disease, peripheral vascular disease, and cerebrovascular disease) complications that exert a strong impact in terms of morbidity and mortality of T2D patients (3). In addition to microvascular and macrovascular complications, older adults with T2D are at higher risk of developing geriatric syndromes leading to important cognitive or functional impairments (4). Accordingly, while in older adults with intact cognitive and physical functions the implementation of prevention plans should pursue the same targets adopted for younger adults, in many other older diabetic patients, their implementation is complicated and strongly depends on the cognitive function, depression, physical disability, comorbidities, and degree of frailty (5–7). Therefore, several clinical parameters should be taken into account when planning therapeutic strategies for complications risk reduction in the older patients: (i) older T2D patients are at high risk of adverse drug reactions consequent to the kidney and liver function disorders; (ii) the impaired adrenergic alert symptoms makes the incidence of asymptomatic hypoglycemia more frequent in this population setting; (iii) the lower medication adherence of older patients with respect to younger people (8). For these reasons, the promotion of healthy ageing in T2D patients requires a comprehensive age‐related healthcare approach, as advocated by the latest ADA standard of care recommendations for this age group (2, 9). On the basis of these recommendations, it is a priority to establish methodologic approaches to individualize prevention plans in order to avoid overtreatment in frail patients and under treatment in other groups of patients (6), recommending different glycosylated hemoglobin targets and glucose-lowering therapies focused on patient preferences, needs, and risk profile. The ADA recommendations, however, are difficult to implement because modern T2D care systems require integrated care between general practitioners, diabetologists and geriatricians, and other members of the healthcare team. Often, older T2D patients are excluded from the personalized treatment targets.
Several studies have identified the risk factors associated with survival in T2D patients younger than 70 years (10–14). Among these, the duration of diabetes, hypertension, hypercholesterolemia, and poor glycemic control were the most relevant (10, 15, 16). However, there is no clear evidence that such factors might also influence the risk of mortality of older diabetic patients. To date, there are few comprehensive data on the risk factors related to survival at the oldest ages and their interaction with indicators of frailty in T2D patients (16–18).
The aim of the present study is to evaluate the link between chemical-clinical risk factors, geriatric parameters, and mortality in T2D patients aged ≥70 years.
2 Materials and methods
2.1 Study population
This retrospective longitudinal study was conducted in a real-world sample of older T2D patients attending outpatient facilities of the Diabetology Unit of the IRCCS INRCA Hospital of Ancona (Central Italy) between January 2014 and October 2015. The study was approved by the Ethical Committee of the IRCCS INRCA Hospital, Italy (reference number: CE-INRCA-18013), with a waiver of informed consent as retrospective deidentified data were used. As the study was retrospective, the objectives of glycemic control, the correction of risk factors, and treatments have been managed by diabetologists according to their usual clinical practices in agreement with the official Italian recommendations (Italian standards for the treatment of diabetes mellitus, Standard Italiani per la cura del diabete mellito, joint project of Italian Association of Diabetologists, Associazione Medici Diabetologi, AMD and Italian Diabetes Society, Società Italiana di Diabetologia, SID) (19). In accordance with the AMD-SID recommendations, to better personalize the treatment of older patients, a comprehensive geriatric assessment has also been routinely performed (7). Patients with type 1 diabetes, secondary diabetes, acute disease stage, or severe intercurrent illnesses were excluded from the present study.
2.2 Data collection
The following baseline data were recorded:
- general characteristics: demographic data, anthropometric data, medical history, clinical laboratory parameters;
- diabetes features: diabetes duration, glycated hemoglobin, fasting glycemia, microalbuminuria, diabetic complications, antidiabetic drugs;
- geriatric assessment:
- Short Physical Performance Battery (SPPB): SPPB is a standardized measure of global physical function that has been validated in frail elderly people and predicts a wide range of clinical outcomes. It has three components: a standing balance test, a walking speed test (walking 4 m), and a strength test (assessed by the time it takes to get up from a chair five times). Each component is scored from 0 to 4; the sum of the scores ranges from 0 to 12, with lower scores indicating more severe physical dysfunction (20, 21);
- Activities of daily living (ADL) define the level of dependence/independence in six daily personal care activities including bathing, toileting, feedings, dressings, urine, and bowel continents and transferring (in and out of bed). The summary score ranges from 0 (low function, dependent) to 6 (high function, independent) (22);
- Instrumental activities of daily living (IADL) assess instrumental daily activities such as telephone, shopping, food preparation, housekeeping, doing laundry, taking medicine correctly, and transfers. The summary score ranges from 0 (low function, dependent) to 8 (high function, independent) (23);
- Mini-Nutritional Assessment (MNA) is a tool to assess the nutritional status of elderly individuals. The collective score of MNA ranges from 0 to 30. The MNA scores ≥24 are considered normal nutrition (24);
- Short Portable Mental Status Questionnaire (SPMSQ) is used for the assessment of organic brain deficit in elderly patients. Scoring: 0–2 errors: normal mental functioning, 3–4 errors: mild cognitive impairment, 5–7 errors: moderate cognitive impairment, 8 or more errors: severe cognitive impairment (25);
- mini Geriatric Depression Scale-5-items [mini GDS-5 items] is a tool for the investigation of signs suggestive of depression. Scoring: 0–1 normal, ≥2 depression (26);
- EuroQuol-5 Dimensions Questionnaire (EQ-5D-5L) is a quality of life survey instrument. The EQ-5D-5L consists of two pages: the EQ-5D descriptive system (mobility, selfcare, usual activities, pain/discomfort, anxiety/depression) and the EQ visual analogue scale (EQ VAS) (27).
The primary outcome of the study was all-cause 5-year mortality. Information on vital status has been ascertained after a mean period of 53.3 ± 15.8 months from the baseline visit, after which 209 T2D patients died (20.9%) and 778 (77.7%) were still alive, whereas for 14 patients (1.4%) vital status was unknown. The T2D cohort consisted of 987 T2D outpatients. After excluding 10 individuals with missing follow-up information, we obtained a final cohort of 977 individuals.
2.3 Data analyses
T2D patient characteristics were summarized using means (± standard deviations [SD]) for continuous variables and counts and percentages (%) for categorical variables. SPPB score was considered as either continuous or categorized (SPPB score 0–4 or 5–8 vs. 9–12) variable (28). Independent sample t-test, chi-squared test, and ANOVA are used when appropriate.
We used the automatic machine-learning tool JADBio to identify parsimonious mathematical models able to correctly stratify the patients according to their mortality risk (29). The tool uses a multivariate analysis approach for (a) identifying the minimal set of features needed for predicting a given outcome, (b) deriving the best predictive model based on the selected features, and (c) assessing the performance of the derived model. It employs the Bootstrap Bias Corrected Cross-Validation (BBC-CV) to provide an unbiased estimation of performance that adjusts (controls) for trying multiple ML pipelines. The classification algorithms used are linear, ridge, and Lasso regression, decision trees, random forests (RF), and support vector machines (SVMs) with Gaussian and polynomial kernels. To create a parsimonious model that can be more efficiently used in daily clinical practice, we performed feature selection by using the Statistically Equivalent Signatures (SES) included in JADBIO (30). To derive the predictive models, JADBio machine learning algorithms including penalized Cox regression models, survival decision trees, and survival random forests were employed. All steps of the analysis are cross validated, in order to fairly compute the performance of all candidate models, with an additional bootstrapping step added for removing any overoptimistic bias caused by overfitting (31).
Model performance was assessed through the concordance index (c-index) (32). The c-index computes the percentage of patient pairs that are correctly ordered by the predictive algorithm according to their time to event. Censored cases are dealt with by removing the corresponding pair whenever a meaningful comparison in terms of time to event is not possible. A c-index of 1 indicates perfect ranking of their patients according to their relative risk, whereas 0.5 indicates random risk assessment and a value < 0.5 corresponds to a model performance worse than random guessing.
The importance of the factors included in the final model is estimated by assessing the decrease in performance when the predictor is dropped from the model.
The decisions tree approach (33), a multivariate technique for both data exploration and prediction, was used to develop a predictive model for the mortality risk, SPPB score categories, and geriatric parameters. The model was divided into two groups at five split layers to produce the most division into four subgroups as possible for the mortality risk.
The statistical analyses were performed by using R Statistical Software (version 4.0.5, R Software for Statistical Computing), as well as SAS software (JMP 17.2) and JADBIO software as service platforms (https://jadbio.com). P‐values were considered statistically significant if less than 0.05.
3 Results
3.1 Clinical characteristics and survival status
The T2D cohort consisted of 977 T2D outpatients aged 76.5 (SD: 4.5) years and with 46.5% of men; individuals had a mean diabetes duration of 16.4 (SD: 11.1) years and a mean HbA1c of 7.4 (SD:1.2) %. The clinical characteristics of the whole sample of diabetic patients and of subgroups of deceased and surviving patients are reported in Table 1. Subjects deceased at follow-up were older, predominantly men, with longer duration of diabetes, higher blood sugar levels, higher prevalence of nephropathy, chronic renal failure, neuropathy, myocardial ischemia, and myocardial infarction compared with surviving subjects. Deceased subjects also had a higher frequency of SPPB categories with lower scores with respect to survival patients.
Regarding diabetic medications, approximately one-third of the patients (35.2%) were taking sulphonylureas alone or together with other medications, 31.4% were taking insulin, almost 49.5% were using biguanides, and 11.4% were taking dipeptidyl peptidase-4 (DPP-4) inhibitors. We excluded antidiabetic drugs to the 19 variables chosen as the minimum set of predictors to estimate the risk of death of patients with T2D. The main reason for this choice is that drug therapy is periodically monitored by the medical team and adjusted according to blood glucose control and the clinical requirements of the patient. Therefore, we have considered that the inclusion of drug therapy at baseline could lead to additional biases, especially considering the 5-year follow-up window. The absence of a time-dependent drug variable did not allow us to explore the potential effects of drug treatment on the observed findings. After a mean follow-up time of 53.3 (SD: 15.8) months from the baseline visit, 209 (21.4%) patients of the first cohort died (Table 1).
3.2 Machine learning approach
The JADBio analysis identified eight features out of 19 variables as the minimal set of predictors needed for optimally estimating the risk of death for T2D patients. The eight features included age, sex, SPPB, CKD, myocardial ischemia, PAD, neuropathy, and myocardial infarction. A penalized Cox regression model based on these predictors achieves a 10-fold cross validated, bootstrap-corrected concordance index of 0.722 (95% confidence interval: 0.683–0.758). Figure 1 reports the importance of each of the eight predictors in terms of their contribution to predictive performance.
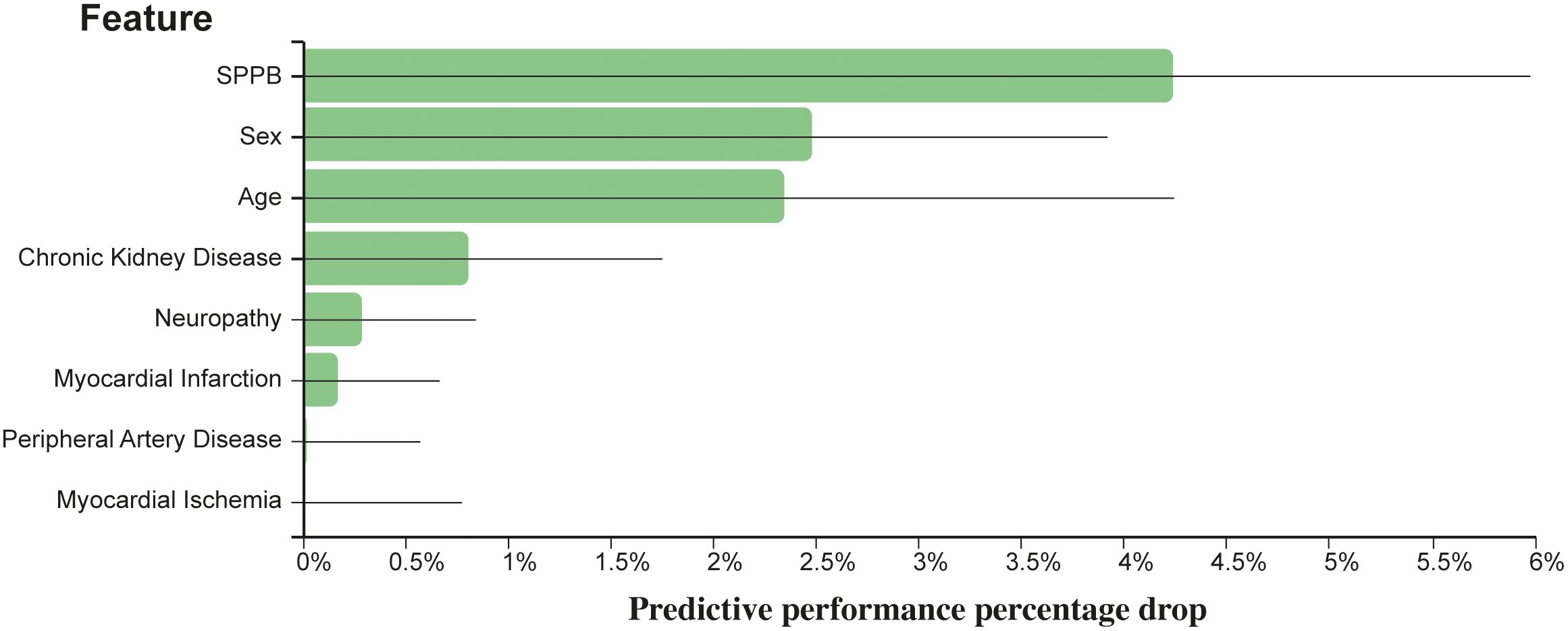
Figure 1 Relevance of each predictor with respect to predictive performance. The y-axis reports the reduction in concordance index that would be observed if the respective predictor is eliminated by the model.
The full JADBIO results are available at http://bit.ly/3ZWNFiJ. Using SPPB in its categorical form provides a model with the same features, and very similar predictive capabilities (concordance index: 0.726 [0.687–0.763]). These results are also available at http://bit.ly/3ZXJvrb.
Features are standardized before deriving the model; thus, the coefficients are directly comparable.
In summary, the SPPB score was ranked as the most important variable whose impact on mortality in terms of relative change of c-index was 4.23%. It was followed by male sex (2.47%), age (2.35%), CKD (0.81%), neuropathy (0.29%), myocardial infarction (0.17%), PAD (0.02%), and myocardial ischemia (<0.01%).
SPPB score (categorical version) was ranked as the most important variable whose impact on mortality in terms of relative change of c-index was 4.20%. It was followed by gender (2.84%), age (2.72%), CKD (0.61%), neuropathy (0.34%), PAD (0.07%), myocardial infarction (0.06%), and myocardial ischemia (<0.01%).
According to the Cox regression model trained on the variables selected by JADBio, the risk of death per unit of time for a subject with a SPPB score lower than five points is 3.35 times that for a subject with a score higher than eight points (P-value<0.001); the risk of death per unit of time for a subject with an intermediate SPPB score is 2.39 times that for a subject with a score higher than 8 points (P-value <0.001) (Table 2). In Figure 2, the survival functions for the three SPPB categories are reported.
3.3 Relationship between SPPB and geriatric baseline parameters
Parameters related to the multidimensional geriatric assessment across SPPB categories of T2D patients are reported in Table 3. T2D patients in the lowest SPPB score category were significantly older than those in the group with the highest scores and with a higher prevalence of women (P-value <0.001 in both cases).
T2D patients in the lowest SPPB score group had more limitations in the instrumental activity of daily living, higher prevalence of cognitive impairment and depression symptoms, and worse nutritional status and self-reported quality of life.
3.4 Decision tree for mortality
The decision tree with five layers identified as mortality risk factors is shown in Figure 3. Based on the results, in the subgroup with lower score SPPB categories (group 1 = 0–4 and group 2 = 5–8) and IADL <7, 39% of subjects were deceased (blue strip). Moreover, in the subgroup with IADL ≥7, MNA ≥22, and SPMSQ ≤7, the number of survival patients was about the same of those deceased. Finally, the subgroup with the SPPB category with the highest score (group 3 = 9–12) and GDS ≥2 and IADL = 8 included patients which are all survivors (Figure 3).
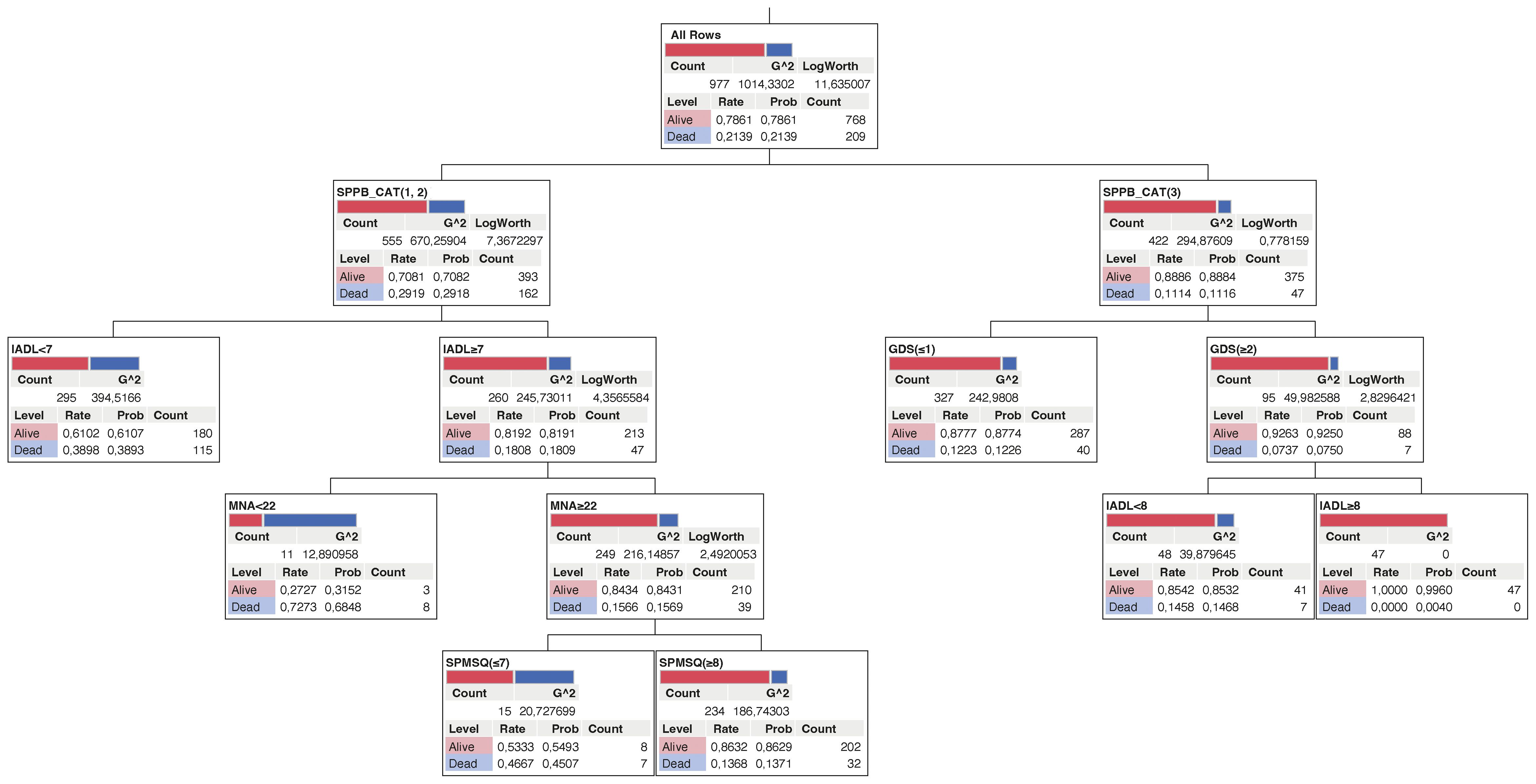
Figure 3 Decision tree for mortality in older diabetic subjects. The deceased and survival subjects are represented as blue and red respectively.
4 Discussion
T2D is a chronic disease, affecting an increasing number of older patient’s world wild. The associated micro- and macrovascular complications are drivers of morbidity and mortality. There is increasing interest in using stratification in type 2 diabetes to target resources, individualize care, and improve outcomes. The systematic reviews of studies applying population stratification in T2D identifies common themes in stratification and outcome variables, in particular a focus on HbA1c (34). The guidelines for managing diabetes in the T2D older patients recognize the need to consider frailty, comorbidities, and functionality when making decisions on patient management (35, 36). Frailty, considered as a generalized loss in physiological reserve capacity, is emerging as a high‐impact geriatric syndrome, and according to a number of studies, T2D adds a fivefold increase in the risk of frailty in middle‐aged and older people (37). Frailty is currently recognized as a complication of diabetes that may subsequently account for the unexplained disability excess seen in older diabetic populations (38).
However, in the clinical practice of diabetes care, especially in outpatient clinics, physical performance measures are not always performed routinely because of several practical issues such as limited consultation time, space, and manpower. Although the biological processes that underlie frailty are still unclear and likely to be complex and multifactorial, sarcopenia may play an important role in the accelerated decline in leg lean mass, muscle strength, and functional capacity seen in older people with diabetes compared with those without diabetes (39, 40).
The aim of the present study is to evaluate the interaction between diabetic features and parameters related to geriatric assessment, on the mortality risk prediction in a large sample of T2D outpatients aged ≥70 years. Since a prediction model derived from real-world data should be an important tool for managing the setting of older T2D patients, we applied an innovative analysis performed through the automatic machine-learning (ML) tool JADBIO, to identify the mathematical models able to correctly stratify the patients according to their mortality risk. ML has been slowly entering every aspect of our lives, and its positive impact on biomedical challenges is under investigation. JADBIO analysis identified eight features such as age, sex, SPPB, CKD, myocardial ischemia, PAD, neuropathy, and myocardial infarction as the minimal set of predictors needed for optimally estimating the risk of death for T2D patients. The SPPB is a well-established tool to assess lower-extremity physical performance status (39). In addition to the non-modifiable parameters such as age and sex, the SPPB test together with the evaluation of the complications of the disease are useful for predicting the risk of mortality and guiding the management of the older diabetic patient on an outpatient basis (41). Large literature data have confirmed that poor performance on the SPPB is associated with an increased risk of all-cause mortality, suggesting that the systematic implementation of the SPPB in clinical practice settings should be useful prognostic information (42–44).
Recently, it was demonstrated that the assessment of the SPPB scale before hospital discharge increased the ability to predict adverse events in older patients affected by acute coronary syndrome (45). We observed that the SPPB score was the best predictor of T2D mortality with respect to the other parameters. Patients with SPPB <5 experienced a 3.42-fold increased mortality risk compared with patients with SPPB >8. As previously reported, HbA1c was not associated with mortality risk (17). T2D patients in the lowest SPPB score group were significantly more disabled and had higher prevalence of cognitive impairment and depression symptoms and showed a better self-reported health and nutritional status.
Decision tree analysis highlighted the relationship among mortality, lower SPPB categories, loss of IADL, poor nutrition, and depression symptoms.
Group-based trajectories of SPPB scores identified distinct subgroups in LIFE Study participants, and using these group assignments in outcome models, a significant association with major mobility disability was observed (46). SPPB is a parameter of physical frailty, recently included as the primary outcome measure in the REHAB-HF clinical trial on older patients with heart failure (47). We did not include other parameters of sarcopenia and frailty, as we used SPPB as the index of physical frailty and sarcopenia (48). A multicomponent intervention seems to be effective in the reduction of the incidence of disability in older adults with physical frailty and sarcopenia, characterized by SPPB scores of 3–7. These data strongly suggest that physical frailty and sarcopenia may be targeted to preserve mobility in vulnerable older people (48).
In conclusion, stratification is an important tool to optimize effectiveness and efficiency in T2D management of older patients. Targeting interventions to the highest mortality risk T2D patients may allow resources to be better used and costs to be reduced.
Data availability statement
The raw data supporting the conclusions of this article will be made available by the authors, without undue reservation.
Ethics statement
The studies involving humans were approved by Ethical Committee of IRCCS INRCA, Ancona, Italy (reference number: CE-INRCA-18013). The studies were conducted in accordance with the local legislation and institutional requirements. The ethics committee/institutional review board waived the requirement of written informed consent for participation from the participants or the participants' legal guardians/next of kin because the study is retrospective. Considering that the data concerned routine visits for the management of diabetes in elderly subjects and that the observation period was rather long, the ethics committee approved the study protocol, waiving informed consent.
Author contributions
AM: Formal analysis, Writing – original draft. VL: Formal analysis, Writing – original draft. LS: Formal analysis, Writing – review & editing. ET: Writing – original draft, Data curation, Investigation. SR: Writing – original draft, Data curation, Investigation. AC: Supervision, Writing – review & editing. LS: Writing – original draft, Formal analysis. JS: Supervision, Writing – review & editing. AC: Funding acquisition, Writing – review & editing. MCo: Writing – review & editing. MCapr: Writing – review & editing. MCapa: Resources, Writing – review & editing. FL: Project administration, Writing – review & editing. FO: Writing – review & editing, Funding acquisition, Methodology. ARB: Conceptualization, Methodology, Supervision, Writing – original draft, Writing – review & editing, Data curation, Investigation.
Funding
The author(s) declare that financial support was received for the research, authorship, and/or publication of this article. This work was funded by the Italian Ministry of Health, Ricerca Corrente, to IRCCS INRCA and co-funding from Next Generation EU, in the context of the National Recovery and Resilience Plan, Investment PE8 – Project Age-It: “Ageing Well in an Ageing Society”. This resource was cofinanced by the Next Generation EU (DM 1557 11.10.2022).
Conflict of interest
The authors declare that the research was conducted in the absence of any commercial or financial relationships that could be construed as a potential conflict of interest.
The author(s) declared that they were an editorial board member of Frontiers, at the time of submission. This had no impact on the peer review process and the final decision.
Publisher’s note
All claims expressed in this article are solely those of the authors and do not necessarily represent those of their affiliated organizations, or those of the publisher, the editors and the reviewers. Any product that may be evaluated in this article, or claim that may be made by its manufacturer, is not guaranteed or endorsed by the publisher.
Author disclaimer
The views and opinions expressed are only those of the authors and do not necessarily reflect those of the European Union or the European Commission. Neither the European Union nor the European Commission can be held responsible for them.
References
1. Inzucchi SE, Bergenstal RM, Buse JB, Diamant M, Ferrannini E, Nauck M, et al. Management of hyperglycaemia in type 2 diabetes, 2015: a patient-centred approach. Update to a position statement of the American Diabetes Association and the European Association for the Study of Diabetes. Diabetologia. (2015) 58:429–42. doi: 10.1007/s00125-014-3460-0
2. Standards of medical care in diabetes-2016: summary of revisions. Diabetes Care. (2016) 39:S4–5. doi: 10.2337/dc16-S003
3. Fowler MJ. Microvascular and macrovascular complications of diabetes. Clin Diabetes. (2008) 26(2):77–82. doi: 10.2337/diaclin.26.2.77
4. American Diabetes Association. 12. Older adults: standards of medical care in diabetes-2019. Diab Care. (2019) 42:S139–47. doi: 10.2337/dc19-S012
5. American Diabetes Association. 11. Older adults: standards of medical care in diabetes-2018. Diabetes Care. (2018) 41:S119–25. doi: 10.2337/dc18-S011
6. Molist-Brunet N, Sevilla-Sánchez D, Puigoriol-Juvanteny E, González-Bueno J, Solà-Bonada N, Cruz-Grullón M, et al. Optimizing drug therapy in frail patients with type 2 diabetes mellitus. Aging Clin Exp Res. (2020) 32:1551–9. doi: 10.1007/s40520-019-01342-z
7. Sesti G, Antonelli Incalzi R, Bonora E, Consoli A, Giaccari A, Maggi S, et al. Management of diabetes in older adults. Nutr Metab Cardiovasc Dis. (2018) 28:206–18. doi: 10.1016/j.numecd.2017.11.007
8. Mooradian AD. Evidence-based management of diabetes in older adults. Drugs Aging. (2018) 35:1065–78. doi: 10.1007/s40266-018-0598-3
9. Professional practice committee for the standards of medical care in diabetes-2016. Diabetes Care. (2016) 39:S107–8. doi: 10.2337/dc16-S018
10. Intensive blood-glucose control with sulphonylureas or insulin compared with conventional treatment and risk of complications in patients with type 2 diabetes (UKPDS 33). UK Prospective Diabetes Study (UKPDS) Group. Lancet. (1998) 352:837–53. doi: 10.1016/S0140-6736(98)07019-6
11. Action to Control Cardiovascular Risk in Diabetes Study Group, Gerstein HC, Miller ME, Byington RP, Goff DC Jr, Bigger JT, et al. Effects of intensive glucose lowering in type 2 diabetes. N Engl J Med. (2008) 358:2545–59. doi: 10.1056/NEJMoa0802743
12. Holman RR, Paul SK, Bethel MA, Matthews DR, Neil HA. 10-year follow-up of intensive glucose control in type 2 diabetes. N Engl J Med. (2008) 359:1577–89. doi: 10.1056/NEJMoa0806470
13. ADVANCE Collaborative Group, Patel A, MacMahon S, Chalmers J, Neal B, Billot L, et al. Intensive blood glucose control and vascular outcomes in patients with type 2 diabetes. N Engl J Med. (2008) 358:2560–72. doi: 10.1056/NEJMoa0802987
14. Skyler JS, Bergenstal R, Bonow RO, Buse J, Deedwania P, Gale EA, et al. Intensive glycemic control and the prevention of cardiovascular events: implications of the ACCORD, ADVANCE, and VA diabetes trials: a position statement of the American Diabetes Association and a scientific statement of the American College of Cardiology Foundation and the American Heart Association. Diabetes Care. (2009) 32:187–92. doi: 10.2337/dc08-9026
15. American Diabetes Association. Executive summary: Standards of medical care in diabetes–2012. Diabetes Care. (2012) 35 Suppl 1:S4–S10. doi: 10.2337/dc12-s004
16. Bauduceau B, Doucet J, Le Floch JP, Verny C, SFD/SFGG Intergroup and the GERODIAB Group. Cardiovascular events and geriatric scale scores in elderly (70 years old and above) type 2 diabetic patients at inclusion in the GERODIAB cohort. Diabetes Care. (2014) 37:304–11. doi: 10.2337/dc13-1540
17. Doucet J, Verny C, Balkau B, Scheen AJ, Bauduceau B. Haemoglobin A1c and 5-year all-cause mortality in French type 2 diabetic patients aged 70 years and older: The GERODIAB observational cohort. Diabetes Metab. (2018) 44:465–72. doi: 10.1016/j.diabet.2018.05.003
18. Bauduceau B, Le Floch JP, Halimi S, Verny C, Doucet J, SFD/SFGG Intergroup. Cardiovascular complications over 5 years and their association with survival in the GERODIAB cohort of elderly french patients with type 2 diabetes. Diabetes Care. (2018) 41:156–62. doi: 10.2337/dc17-1437
19. Mannucci E, Candido R, Delle Monache L, Gallo M, Giaccari A, Masini ML, et al. Italian guidelines for the treatment of type 2 diabetes. Nutr Metab Cardiovasc Dis. (2022) 32:770–814. doi: 10.1016/j.numecd.2022.01.027
20. Guralnik JM, Simonsick EM, Ferrucci L, Glynn RJ, Berkman LF, Blazer DG, et al. A short physical performance battery assessing lower extremity function: association with self-reported disability and prediction of mortality and nursing home admission. J Gerontol. (1994) 49:M85–94. doi: 10.1093/geronj/49.2.m85
21. Volpato S, Cavalieri M, Guerra G, Sioulis F, Ranzini M, Maraldi C, et al. Performance-based functional assessment in older hospitalized patients: feasibility and clinical correlates. J Gerontol A Biol Sci Med Sci. (2008) 63:1393–8. doi: 10.1093/gerona/63.12.1393
22. Katz S, Downs TD, Cash HR, Grotz RC. Progress in development of the index of ADL. Gerontologist. (1970) 10:20–30. doi: 10.1093/geront/10.1_part_1.20
23. Lawton MP, Brody EM. Assessment of older people: self-maintaining and instrumental activities of daily living. Gerontologist. (1969) 9:179–86. doi: 10.1093/geront/9.3_Part_1.179
24. Taheri Tanjani P, Moradinazar M, Esmail Mottlagh M, Najafi F. The prevalence of diabetes mellitus (DM) type II among Iranian elderly population and its association with other age-related diseases, 2012. Arch Gerontol Geriatr. (2015) 60:373–9. doi: 10.1016/j.archger.2014.11.012
25. Ferrucci L, Guralnik JM, Salive ME, Pahor M, Corti MC, Baroni A, et al. Cognitive impairment and risk of stroke in the older population. J Am Geriatr Soc. (1996) 44:237–41. doi: 10.1111/j.1532-5415.1996.tb00908.x
26. Rinaldi P, Mecocci P, Benedetti C, Ercolani S, Bregnocchi M, Menculini G, et al. Validation of the five-item geriatric depression scale in elderly subjects in three different settings. J Am Geriatr Soc. (2003) 51:694–8. doi: 10.1034/j.1600-0579.2003.00216.x
27. Clarke PM, Hayes AJ, Glasziou PG, Scott R, Simes J, Keech AC. Using the EQ-5D index score as a predictor of outcomes in patients with type 2 diabetes. Med Care. (2009) 47:61–8. doi: 10.1097/MLR.0b013e3181844855
28. Corsonello A, Lattanzio F, Pedone C, Garasto S, Laino I, Bustacchini S, et al. Prognostic significance of the short physical performance battery in older patients discharged from acute care hospitals. Rejuvenation Res. (2012) 15:41–8. doi: 10.1089/rej.2011.1215
29. Tsamardinos I, Charonyktakis P, Papoutsoglou G, Borboudakis G, Lakiotaki K, Zenklusen JC, et al. Just Add Data: automated predictive modeling for knowledge discovery and feature selection. NPJ Precis Oncol. (2022) 6:38. doi: 10.1038/s41698-022-00274-8
30. Tsagris M, Tsamardinos I. Feature selection with the R package MXM. F1000Res. (2018) 20:7. doi: 10.12688/f1000research.16216.2
31. Tsamardinos I, Greasidou E, Borboudakis G. Bootstrapping the out-of-sample predictions for efficient and accurate cross-validation. Mach Learn. (2018) 107:1895–922. doi: 10.1007/s10994-018-5714-4
32. Harrell FE Jr, Califf RM, Pryor DB, Lee KL, Rosati RA. Evaluating the yield of medical tests. JAMA. (1982) 247:2543–6. doi: 10.1001/jama.247.18.2543
33. Aghasizadeh M, Samadi S, Sahebkar A, Miri-Moghaddam E, Esmaily H, Souktanloo M, et al. Serum HDL cholesterol uptake capacity in subjects from the MASHAD cohort study: Its value in determining the risk of cardiovascular endpoints. J Clin Lab Anal. (2021) 35:e23770. doi: 10.1002/jcla.23770
34. Hodgson S, Cheema S, Rani Z, Olaniyan D, O'Leary E, Price H, et al. Population stratification in type 2 diabetes mellitus: A systematic review. Diabetes Med. (2022) 39:e14688. doi: 10.1111/dme.14688
35. Kirkman MS, Briscoe VJ, Clark N, Florez H, Haas LB, Halter JB, et al. Diabetes in older adults. Diabetes Care. (2012) 35:2650–64. doi: 10.2337/dc12-1801
36. Sinclair A, Morley JE, Rodriguez-Mañas L, Paolisso G, Bayer T, Zeyfang A, et al. Diabetes mellitus in older people: position statement on behalf of the International Association of Gerontology and Geriatrics (IAGG), the European Diabetes Working Party for Older People (EDWPOP), and the International Task Force of Experts in Diabetes. J Am Med Dir Assoc. (2012) 13:497–502. doi: 10.1016/j.jamda.2012.04.012
37. Hanlon P, Nicholl BI, Jani BD, Lee D, McQueenie R, Mair FS. Frailty and pre-frailty in middle-aged and older adults and its association with multimorbidity and mortality: a prospective analysis of 493 737 UK Biobank participants. Lancet Public Health. (2018) 3:e323–32. doi: 10.1016/S2468-2667(18)30091-4
38. Yoon SJ, Kim KI. Frailty and disability in diabetes. Ann Geriatr Med Res. (2019) 23:165–9. doi: 10.4235/agmr.19.0036
39. Volpato S, Bianchi L, Lauretani F, Lauretani F, Bandinelli S, Guralnik JM, et al. Role of muscle mass and muscle quality in the association between diabetes and gait speed. Diabetes Care. (2012) 35:1672–9. doi: 10.2337/dc11-2202
40. Arévalo Lorido JC, Carretero Gomez J, Vazquez Rodriguez P, Gómez Huelgas R, Marín Silvente C, Sánchez Lora FJ, et al. Glycemic control and prescription profiles in internal medicine inpatients: The role of frailty. Eur J Intern Med. (2024) 121:103–8. doi: 10.1016/j.ejim.2023.10.022
41. Bandinelli S, Lauretani F, Boscherini V, Gandi F, Pozzi M, Corsi AM, et al. A randomized, controlled trial of disability prevention in frail older patients screened in primary care: the FRASI study. Design and baseline evaluation. Aging Clin Exp Res. (2006) 18:359–66. doi: 10.1007/BF03324831
42. Pavasini R, Guralnik J, Brown JC, di Bari M, Cesari M, Landi F, et al. Short Physical Performance Battery and all-cause mortality: systematic review and meta-analysis. BMC Med. (2016) 14:215. doi: 10.1186/s12916-016-0763-7
43. Mickute M, Henson J, Rowlands AV, Sargeant JA, Webb D, Hall AP, et al. Device-measured physical activity and its association with physical function in adults with type 2 diabetes mellitus. Diabetes Med. (2021) 38:e14393. doi: 10.1111/dme.14393
44. Rodriguez-Mañas L, Laosa O, Vellas B, Paolisso G, Topinkova E, Oliva-Moreno J, et al. Effectiveness of a multimodal intervention in functionally impaired older people with type 2 diabetes mellitus. J Cachexia Sarcopenia Muscle. (2019) 10:721–33. doi: 10.1002/jcsm.12432
45. Campo G, Maietti E, Tonet E, Biscaglia S, Ariza-Solè A, Pavasini R, et al. The assessment of scales of frailty and physical performance improves prediction of major adverse cardiac events in older adults with acute coronary syndrome. J Gerontol A Biol Sci Med Sci. (2020) 75:1113–9. doi: 10.1093/gerona/glz123
46. Brown JD, Lo-Ciganic WH, Shao H, Pahor M, Manini TM. Trajectories of short physical performance battery are strongly associated with future major mobility disability: results from the LIFE study. J Clin Med. (2020) 9:2332. doi: 10.3390/jcm9082332
47. Kitzman DW, Whellan DJ, Duncan P, Pastva AM, Mentz RJ, Reeves GR, et al. Physical rehabilitation for older patients hospitalized for heart failure. N Engl J Med. (2021) 385:203–16. doi: 10.1056/NEJMoa2026141
Keywords: type 2 diabetes, short physical performance battery, older, mortality, machine learning, decision tree analysis
Citation: Montesanto A, Lagani V, Spazzafumo L, Tortato E, Rosati S, Corsonello A, Soraci L, Sabbatinelli J, Cherubini A, Conte M, Capri M, Capalbo M, Lattanzio F, Olivieri F and Bonfigli AR (2024) Physical performance strongly predicts all-cause mortality risk in a real-world population of older diabetic patients: machine learning approach for mortality risk stratification. Front. Endocrinol. 15:1359482. doi: 10.3389/fendo.2024.1359482
Received: 21 December 2023; Accepted: 12 April 2024;
Published: 30 April 2024.
Edited by:
Fabio Monzani, University of Pisa, ItalyReviewed by:
Angela Abbatecola, Azienda Sanitaria Locale Frosinone, ItalyMikhail Ivanchenko, Lobachevsky State University of Nizhny Novgorod, Russia
Copyright © 2024 Montesanto, Lagani, Spazzafumo, Tortato, Rosati, Corsonello, Soraci, Sabbatinelli, Cherubini, Conte, Capri, Capalbo, Lattanzio, Olivieri and Bonfigli. This is an open-access article distributed under the terms of the Creative Commons Attribution License (CC BY). The use, distribution or reproduction in other forums is permitted, provided the original author(s) and the copyright owner(s) are credited and that the original publication in this journal is cited, in accordance with accepted academic practice. No use, distribution or reproduction is permitted which does not comply with these terms.
*Correspondence: Anna Rita Bonfigli, YS5ib25maWdsaUBpbnJjYS5pdA==
†These authors share last authorship