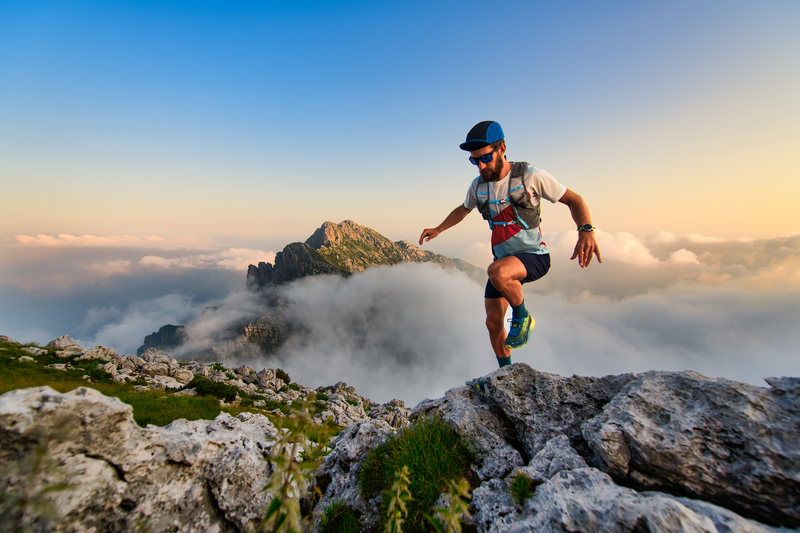
94% of researchers rate our articles as excellent or good
Learn more about the work of our research integrity team to safeguard the quality of each article we publish.
Find out more
SYSTEMATIC REVIEW article
Front. Endocrinol. , 29 May 2024
Sec. Cancer Endocrinology
Volume 15 - 2024 | https://doi.org/10.3389/fendo.2024.1351624
Introduction: Well-differentiated pancreatic neuroendocrine tumors (PNETs) can be non-functional or functional, e.g. insulinoma and glucagonoma. The majority of PNETs are sporadic, but PNETs also occur in hereditary syndromes, primarily multiple endocrine neoplasia type 1 (MEN1). The Knudson hypothesis stated a second, somatic hit in MEN1 as the cause of PNETs of MEN1 syndrome. In the recent years, reports on genetic somatic events in both sporadic and hereditary PNETs have emerged, providing a basis for a more detailed molecular understanding of the pathophysiology. In this systematic review and meta-analysis, we made a collation and statistical analysis of aggregated frequent genetic alterations and potential driver events in human grade G1/G2 PNETs.
Methods: A systematic search was performed in concordance with the Preferred Reporting Items for Systematic Review and Meta-Analyses (PRISMA) reporting guidelines of 2020. A search in Pubmed for published studies using whole exome, whole genome, or targeted gene panel (+400 genes) sequencing of human G1/G2 PNETs was conducted at the 25th of September 2023. Fourteen datasets from published studies were included with data on 221 patients and 225 G1/G2 PNETs, which were divided into sporadic tumors, and hereditary tumors with pre-disposing germline variants, and tumors with unknown germline status. Further, non-functioning and functioning PNETs were distinguished into two groups for pathway evaluation. The collated genetical analyses were conducted using the ‘maftools’ R-package.
Results: Sporadic PNETs accounted 72.0% (162/225), hereditary PNETs 13.3% (30/225), unknown germline status 14.7% (33/225). The most frequently altered gene was MEN1, with somatic variants and copy number variations in overall 42% (95/225); hereditary PNETs (germline variations in MEN1, VHL, CHEK2, BRCA2, PTEN, CDKN1B, and/or MUTYH) 57% (16/30); sporadic PNETs 36% (58/162); unknown germline status 64% (21/33). The MEN1 point mutations/indels were distributed throughout MEN1. Overall, DAXX (16%, 37/225) and ATRX-variants (12%, 27/225) were also abundant with missense mutations clustered in mutational hotspots associated with histone binding, and translocase activity, respectively. DAXX mutations occurred more frequently in PNETs with MEN1 mutations, p<0.05. While functioning PNETs shared few variated genes, non-functioning PNETs had more recurrent variations in genes associated with the Phosphoinositide 3-kinase, Wnt, NOTCH, and Receptor Tyrosine Kinase-Ras signaling onco-pathways.
Discussion: The somatic genetic alterations in G1/G2 PNETs are diverse, but with distinct differences between sporadic vs. hereditary, and functional vs. non-functional PNETs. Increased understanding of the genetic alterations may lead to identification of more drivers and driver hotspots in the tumorigenesis in well-differentiated PNETs, potentially giving a basis for the identification of new drug targets. (Funded by Novo Nordisk Foundation, grant number NNF19OC0057915).
Pancreatic neuroendocrine tumors (PNETs) represent a rare tumor type accounting for less than 3% of all pancreatic malignancies (1). Most PNETs develop sporadically. Here as only five to ten percent of PNETs occur due to hereditary syndromes including multiple endocrine neoplasia type 1 (MEN1), and more rarely von Hippel-Lindau disease, neurofibromatosis type 1, and tuberous sclerosis (2).
According to the Knudson’s two-hit hypothesis from 1993, PNETs occurring in patients with MEN1 syndrome are likely caused by a somatic second hit in MEN1 in the PNET in individuals carrying a germline MEN1 mutation (3, 4). The MEN1 gene encodes the putative tumor suppressor menin, which plays a role in biological processes such as histone and transcription regulation (5–9), DNA repair (10), and apoptosis (9).
Somatic variants in MEN1 gene are reported in 25–44% of all PNETs (11–14). Additionally, DAXX and ATRX, both encoding chromatin-remodelers, are frequently altered somatically in PNETs, as well as other genes in the mTOR and DNA repair pathways (12, 13). PNETs may be non-functioning, or functioning leading to a clinically measurable hormonal hypersecretion syndrome such as insulinoma and glucagonoma. A mutational hotspot in YY1, (p.Thr372Arg), has been identified in 8–30% of sporadic insulinomas (15–17). Along with YY1, other genes have been proposed to be drivers in insulinomas (18, 19), although their relative frequencies are rather low.
In the last decades, investigations with expanded genetic analyses have provided an explosion of our knowledge on somatic gene changes in tumors in general, which may contribute to clinical diagnosis, prognosis, treatment and categorization of patients. Little is known, however, about tumor genetics by expanded genetic analyses in well differentiated (wd)-PNETs.
Understanding the tumor genetics of PNETs may provide a basis for improved diagnosis and management for PNET patients and may contribute to the discovery of new drug targets as a supplement to present drugs, such as the mTOR-inhibitor everolimus and the kinase inhibitor sunitinib malate (20–22). Given these perspectives, we aimed to conduct a systematic review of published DNA sequencing data gained by expanded genetic analyses of wd-PNETs following the Preferred Reporting Items for Systematic Review and Meta-Analyses (PRISMA) reporting guidelines (23). Thereby we gathered information to shed light on the frequently altered genes in association to genetically predisposed patients and distinct functionality of PNETs.
Genetic datasets of PNETs using whole exome sequencing (WES), whole genome sequencing (WGS), and targeted gene-panels of more than 400 genes were searched for in Pubmed on 25th of September 2023 using the following search criteria: “Pancreatic neuroendocrine tumor” AND (“sequencing” OR “NGS”), “Pancreatic neuroendocrine neoplasm” AND (“sequencing” OR “NGS”),”Insulinoma” AND (“sequencing” OR “NGS”), “Glucagonoma” AND (“sequencing” OR “NGS”),”Somatostatinoma” AND (“sequencing” OR “NGS),”Gastrinoma” AND (“sequencing” OR “NGS”),”VIPoma” AND (“sequencing” OR “NGS”), “Serotonin-producing tumors” AND (“sequencing” OR “NGS”), “ACTH-producing tumors” AND (“sequencing” OR “NGS”), “Gastroenteropancreatic” AND (“sequencing” OR “NGS”), “GEP-NET” AND (“sequencing” OR “NGS”), “GEPNET” AND (“sequencing” OR “NGS”), “PNET” AND (“sequencing” OR “NGS”), “P-NET” AND (“sequencing” OR “NGS”), “PanNET” AND (“sequencing” OR “NGS”), “Pan-NET” AND (“sequencing” OR “NGS”), “PNEN” AND (“sequencing” OR “NGS”). The studies were screened by one reviewer and included datasets were also retrieved by the same reviewer.
All pancreatic functional tumor types in the search were retrieved from Guilmette et al (2019) (24) and WHO Classification of Tumors of Endocrine Organs (2017) (25). The search included words appearing in the title and abstract of each article. Selected articles were then screened individually for inclusion and exclusion criteria as stated below.
We included studies using WES, WGS and/or a gene panel of more than 400 genes to determine the somatic variants in PNETs. The cut-off of 400 genes was chosen based on a Japanese study with use of data from The Cancer Genome Atlas (26), showing that cancer-panel sizes at or above 400 genes had the best statistic performance power to identify tissue mutations in comparison to WES (26). In our dataset, we included human PNETs with tumor grade G1 or G2. Wd-G3 PNETs were not included in the present analyses given the interpretation difficulties and the fact that G3-NETs were not separated from G3 neuroendocrine carcinomas (NECs) in articles based on WHO classifications prior to 2017 (13). Both patients with and without tumor syndromes with both functioning and non-functioning PNETs were included. Both studies using formalin-fixed paraffin-embedded (FFPE) and fresh frozen samples were included.
Exclusion criteria included studies on cell-lines and human studies on extra-pancreatic tissues, carcinomas, non-islet PNETs, metastatic tissues, PNETs with tumor grade G3 only and NECs; Studies with data without proper sample characteristics were excluded. Studies without obtainable full text or main dataset were also excluded. Replicated data were only presented once from the original study. The literature search flow chart is presented in Figure 1. The full list of excluded and included papers is listed in Supplementary Tables 1, 2 and Table 1, respectively.
Figure 1 PRISMA diagram of the literature search. A total of 14/300 datasets were included. PNET, Pancreatic neuroendocrine tumor; G, histological grade. .
Table 1 The sequencing characteristics of all 225 pancreatic neuroendocrine tumors from each of the 14 included articles.
The meta-analysis of included data involved the following steps: 1) Select all datasets in each published database comprising samples belonging to the chosen conditions. 2) For each separate dataset, find and select PNETs with a tumor grade of G1 or G2. 3) Categorize the samples based on tumor type (functional vs non-functional) and sporadic vs hereditary when specified, retrieve DNA variations, including mutations, and copy number variations (CNVs; including amplifications (Amp), deletions (Del), and copy-neutral loss of heterozygosity (cnLOH) of gene region or whole chromosome), where applicable, 5) exclude synonymous variants, 6) exclude intronic variants, 7) exclude common variants identified in the general population (GnomAD, frequency < 1%), 8) list the mutations and CNVs in genes somatically altered in PNETs, 9) compose data to a single mutation annotation format MAF-like file for analysis as described below.
One dataset was aligned to the reference genome GRCh38 and was converted to GRCh37 using LiftOver (https://genome.ucsc.edu/cgi-bin/hgLiftOver). Twelve intronic variants in CUX could not be converted. Alterations on nucleotide level were predicted using the software Transvar (37) using the amino acid alteration and coding sequence (CDS) as input. The generated nucleotide alteration (reference and alternative allele) was compared to four of the included datasets (14, 15, 19, 32), n=71/225. By using the software, both strands could be considered altered, thus we accepted the generated nucleotide if it followed the base pairing rule in comparison to the datasets. Two studies lacked HGVS description of amino acid alterations (32, 33), and these were generated using the genomic coordinates of the CDS and the represented amino acids (reference and alternative amino acid) or nucleotide change, respectively. The study of Scarpa et al. (13) used a different annotation when describing indels, and the HGVS annotation was achieved using Transvar based on the genomic coordinates of the CDS and the chromosome number. A few splice-variants were excluded due to missing data.
Data were collected from each included article and the data were listed in a MAF-like structure. The collection of data was achieved in excel and the data-frame was compiled to a CSV-file before the generation of the MAF-file in R. The resultant MAF-like file was generated for all samples and consisted of the following information: Genetic data, including Human Genome Organization (HUGO) gene nomenclature symbol, variant classification, variant type, reference allele, tumor seq allele1, tumor seq allele2, and amino acid change along with the tumor sample barcode and the respective article. Clinical data about metastasis, tumor type, and tumor syndrome was also added to the MAF file for each tumor sample barcode. When applicable, CNV data were collected and added to an additional customized table in a second file. The file could be merged to the MAF-like file during the meta-analysis.
The generated MAF-like file including all retrieved sample information was used for further analysis. We categorized each PNET of a patient into sporadic vs. hereditary, including germline variations in MEN1, VHL, NF1, CDKN1B, BRCA2, MUTYH, CHEK2, PTEN, by use of the descriptions from the articles and corresponding datasets. Further, each PNET was categorized into non-functional (e.g. PPomas, and other PNETs without hypersecreting syndrome) vs. functional PNETs (e.g. insulinoma, glucagonoma, VIPoma, gastrinoma) according to the retrieved published data. Further, the presence of distant metastases was registered, if published.
The R-package Maftools (38) was used to produce a summary of the file, oncoplots and a plot of implicated oncogenic pathways, along with analyses of gene-specific mutations, co-occurrence of mutations, and variant enrichment.
The pathogenicity of missense mutations in DAXX and ATRX were assessed using Polyphen-2 (39). Protein domains in DAXX and ATRX were retrieved from Wang et al. (40).
P-values were determined using two-tailed Fishers-exact test, a Freeman-Halton extended Fishers exact test, or Mann-Whitney U test where appropriate. P-values less than 0.05 were considered significant. Data were analyzed using R (v. 4.2.3.).
Our study included 14 of 300 datasets available using our search criteria, Table 1. The 14 datasets comprised a total of 225 individual G1/G2 PNET samples from 221 patients. Of the 225 PNETs included, 162 (72%) were sporadic with no associated germline alteration and 30 (13%) were hereditary with germline predisposing alterations in MEN1 (14 PNETs in 11 patients), MUTYH, CHEK2, VHL, CDKN1B, BRCA2, and PTEN, Table 2 and Supplementary Table 3. Two patients harbored variants in two predisposing genes (MEN1/MUTYH and CHEK2/MUTYH). In 33 PNETs (15%), information on germline predisposition was missing.
Patients with germline predisposition were significantly younger than patients with sporadic PNETs, 46 vs. 56 years, p=0.005. The PNETs were functional in 45 (20%), of which insulinomas accounted for 37 (82%), non-functional in 156 (69%), and with unspecified functionality in 24 (11%). Our meta-analysis showed no difference in functional and non-functional PNETs in hereditary vs. sporadic PNETs, 5/22 (22.7%) vs. 40/146 (27.4%), p=0.80.
Of the 225 PNETs, 25 (11%) were accompanied with distant metastasis. No difference was seen between genetically predisposed and sporadic PNETs regarding the presence of metastasis.
The 225 PNETs had a total of 6,194 reported non-synonymous somatic variants. We identified a difference between computed nucleotide change by Transvar and the annotated change in 74 cases, accounting for 7.3% of tested nucleotide alterations. In addition, the Transvar software failed to identify nucleotide change for 420 of the reported changes. In 118 cases, the cause of failing was an invalid gene annotation, 294 lacked a valid transcript, 6 had an invalid mutation nomenclature and 2 were out of range. Due to the pronounced difference between the Transvar output and the published data, we decided only to use the annotations described by the articles regarding the nucleotides on each tumor allele. The overall distribution of transversions and transitions in the resultant dataset is shown in Supplementary Figure 1.
The most frequently somatically altered genes in all PNETs are highlighted in Figure 2. The top three variated genes were MEN1 (n=95; 42%), DAXX (n= 37, 17%) and ATRX (n=27; 12%). Somatic point mutations/indels in MEN1 were identified in 67 PNETs and CNVs in 41 PNETs, including 13 PNETs with both a point mutation/indel and CNV in the gene. The somatic MEN1 point mutations/indels in the coding region were distributed throughout the gene as shown in Figure 3 (lower row).
Figure 2 Genes frequently altered in pancreatic neuroendocrine tumors (PNETs). Oncoplot of genes altered in more than 2% of all samples are presented in the datasets (n=225). The sum of mutations annotated in each PNET is shown in the upper panel. Each colored bar indicates a somatic variant colored based on the mutation type and copy number variation as depicted. Colored filled boxes represents mutations, partly filled boxes represents copy number variations. The tumor types of each of the PNETs are shown in the lower bar. Panel on the right sums up the number of mutations (upper) and copy number variations (CNVs) (lower) identified in each specific gene. Further, the percentage of PNETs with mutations (- CNV) and mutations plus CNVs (+CNV) are presented at the right. TMB, tumor mutation burden; cnLOH, copy-neutral loss of heterozygosity; CNV, copy number variations.
Figure 3 MEN1 mutational distribution in MEN1 patients and sporadic PNETs, where MEN1 is altered in 30% on mutational level. (Upper panel) MEN1 mutations found in germline from 11 (73%) of MEN1 patients. One MEN1 patient harbored germline copy number variations in MEN1, and one MEN1 patients had a splice site variant (not shown). Lastly, one PNET had an unspecified MEN1-germline variant. (Lower panel) somatic MEN1 mutations from two patient with MEN1 (p.Leu175Pro and p.G469Afs*35) and 65 sporadic PNETs. Nine somatic variants were further identified in the MEN1 splice sites (not shown). Twelve (80%) MEN1 patients had somatic copy number variations in the MEN1 locus. Transcript: NM_130799 (isoform 2), protein identifier: NP_570711, menin length: 610 amino acids.
Variants in the coding regions of DAXX and ATRX are presented in Figure 4. The DAXX and ATRX mutations were unique except for one DAXX alteration, which was present in two PNETs. All of the missense mutations in DAXX (n=3) and ATRX (n=6) were predicted as probably damaging by PolyPhen2. Notably, the DAXX missense mutations were located in close proximity to each other (amino acid 328 to 331) in the histone-binding domain. The majority of missense variants in ATRX (5/6) were all located in the ATPase domain spanning residues 1,550–2,226.
Figure 4 DAXX and ATRX somatic alterations in pancreatic neuroendocrine tumors (PNETs). Somatic variations uncovered in the frequently altered genes (A) DAXX (15%) and (B) ATRX (8.5%). Each protein is highlighted in gray, using the transcripts NM_001141970 and NM_000489, respectively. Domains are represented as colored boxes. The pins correspond to single somatic mutations identified in PNETs of the cohort and the color of the pin indicates the mutation type, and the height depicts the number of the variant type in the locus. Annotated domains are adapted from Wang et al. (40). Domains in DAXX: SIM, Sumo-interaction motif; DHB, DAXX helical bundle; HBD, histone binding domain; A, Acidic segment rich in Glu/Asp residues; SPE, segment rich in Ser/Pro/Glu residues; SPT, segment rich in Ser/Pro/Thr residues. Domains in ATRX: ADD, ATRX-DNMT1-DNMT1L domain; HP1, HP1-binding motif; DBM, DAXX binding motif; ATPase, ATPase domain.
In analysis of co-occurrence of mutated genes, DAXX and MEN1 mutations were more frequently co-occurring compared to other pairwise gene co-occurrences, p<0.05, Figure 5. A trend toward mutually exclusiveness for DAXX and ATRX variants was observed (p<0.1), whereas MEN1 and YY1 variants were mutually exclusive, however insignificant due to low numbers.
Figure 5 Co-occurrence plot showing mutually exclusive (brown) or co-occurring (cyan) set of altered genes (mutations) identified in PNETs. The plot shows the top of mutated genes (altered in more than 2% of PNETs) and p-values are indicated as asterics (p-value < 0.05) or dots (p-value < 0.1) determined by pair-wise Fisher’s exact test. * P-value < 0.05 and the dot represents p-value < 0.1
MEN1 was the most frequently altered gene in both hereditary and sporadic PNETs (Figures 6A, B). Somatic MEN1 mutations were found in 5/30 (16.7%) of hereditary PNETs vs. 52/162 (32.1%) of the sporadic PNETs (p=0.13).
Figure 6 Frequently altered genes in hereditary and sporadic pancreatic neuroendocrine tumors (PNETs). The gene list is ordered after the frequency of somatic mutations in specific genes. (A) Data from PNETs from patients with germline mutation in MEN1, MUTYH, CHEK2, BRCA, CDKN1B, CHEK2/MUTYH, MEN1/MUTYH or VHL (n=30) and (B) PNETs from patients with sporadic PNETs (n=162).The tumor syndrome of each of the PNETs is shown in the lower bar. Each colored bar in the oncoplot indicates a somatic variant colored based on the mutation type or copy number variation as depicted. Filled colored boxes represent gene-specific somatic mutations, and partly colored boxes represent copy number variations in the specific gene region or chromosome. Panel on the right sums up the number of PNETs with mutations (upper), and mutations plus copy number variations (CNVs) (lower) identified in each specific gene. Likewise, the percentage is represented for PNETs with mutations (-CNV) and for PNETs with mutations and CNVs (+CNV). PNETs with more than one mutation in a gene were represented as a multi-hit (black), and genes with mutation and copy number variation were depicted as a complex event (grey). The sum of variations annotated in each PNET is shown in the upper panel. CNV, copy number variation; cnLOH, copy-neutral loss of heterozygosity; TMB, tumor mutation burden.
Of the 14 hereditary PNETs from patients with a germline MEN1 mutation, all 13 with available data had a second somatic hit in MEN1. CNVs in the MEN1 region were seen in 12 (11 deletions and 1 cnLOH; one with an additional somatic MEN1 point mutation); one had a somatic MEN1 point mutation only. Notably, MEN1-germline PNETs did not share other somatic gene mutations than MEN1.
The germline MEN1 mutations in the hereditary group are shown in upper row of the Figure 3, and constitute of 11 point mutations/indels in addition to 1 splice site variant and one 1 germline CNV in MEN1. One germline mutation (1/14) was unknown. As for the somatic MEN1 mutations, the germline mutations were distributed throughout the gene. CNVs in MEN1 were more frequently detected in hereditary compared to sporadic PNETs, 12/30 vs. 8/162, p<0.00001. CNVs were rarely reported in other genes in hereditary PNETs except for CRY2 CNVs, which accounted 8/10 (80%).
DAXX and ATRX point mutations and CNVs occurred equally frequent in hereditary vs. sporadic PNETs, Figures 4A, B. Somatic mutations in DAXX were found in four PNETs with hereditary CHEK2 and/or MUTYH. A trend toward more frequent PTEN mutations in hereditary vs. sporadic PNETs was observed (13% vs. 4% including CNVs, p=0.07).
The abundancy of other shared altered genes was low in both hereditary and sporadic PNETs. None of the other 17 abundant genes in hereditary PNETs were found among the 10 other genes in sporadic PNETs. Notably, three PNETs with germline MUTYH and one with a germline BRCA2 variant had the highest tumor mutational burden (TMB) compared to the other germline predisposed PNETs.
Non-functioning and functioning PNETs accounted for 201/225 (89.3%) of the included PNETs and 24 (10.7%) PNETs had non-specified functionality. Non-functioning PNETs accounted 156/201 (77.6%) and functioning PNETs with specified functionality 42/201 (21%). The functioning PNETs were insulinomas (n=37), glucagonomas (n=2), gastrinomas (n=2) and VIPoma (n=1).
Separate mutational profiles for non-functioning and functioning PNETs are seen in Figures 7A, B. As for hereditary and sporadic PNETs, somatic mutations in MEN1, DAXX and ATRX were most frequent in non-functioning PNETs.
Figure 7 Oncoplots of somatic mutations and copy number variations identified in pancreatic neuroendocrine tumors (PNETs) divided into tumor types. Frequently altered genes identified in (A) non-functioning PNETs and (B) functioning PNETs are presented (found in more than 3%). Variant type is depicted in the barplot and the sum of mutations (upper) and the sum of mutations plus copy number variations (lower) in each gene is represented in the right bar. The percentage represents the percentage of PNETs with mutations (-CNV) and mutations plus copy number variations (+CNV) in each gene. The upper bar shows the total number of mutations in each specific PNET sample. (C) Groupwise comparison of gene variants based on tumor type. Copy number variations are not included in the analysis. The corresponding bars are colored by tumor type and indicates genes significantly altered (p-value < 0.05) between tumor types. The y-axis represents the odds ratio. TMB, Tumor mutation burden; CNV, copy number variation; cnLOH, copy-neutral loss of heterozygosity.
Functioning PNETs only shared mutations in eight genes, of which YY1 (5/45, 11.1%) and MEN1 (4/45, 8.9%) were the most frequently mutated (Figure 7B). Additionally, CNVs in MEN1 were abundant (9/45, 20%) in functioning PNETs. However, when comparing the SNVs, MEN1 mutations were only enriched in non-functioning PNETs (Figure 7C). Apart from MEN1, non-functioning and functioning PNETs did not share other altered genes. DAXX mutations were only identified in 1/45 (2.2%) of the functioning vs. 32/156 (21%) of the non-functioning PNETs, p=0.0023, highlighting the enrichment of DAXX mutations in non-functioning PNETs (Figure 7C). The single DAXX mutation was detected in one of the two glucagonomas.
Variants in YY1, STAG2, ZCCHC6, and ZZEF1 were insulinoma-specific, when compared to other functioning PNETs. Enrichment of YY1-variants was significantly higher in insulinomas vs. other PNETs (Figure 7C). In line with this, the recurrent mutation in YY1 (p.Thr372Arg) was identified in five insulinoma samples (5/37 insulinomas, 14%), and a single non-functioning PNET (n=1/156, accounting for 0.6% of the non-functioning PNETs), p=0.0011. No other YY1-variants were identified.
The most enriched oncogenic pathway in non-functioning PNETs were phosphoinositide 3-kinase (PI3K) (Figure 8A), also known as PI3K/AKT/mTOR pathway. In this pathway, the most abundant altered genes were coding for PTEN (n=9), TSC2 (n=6), TSC1 (n=5) and MTOR (n=4). Other onco-pathways altered in more than 10% of non-functioning PNETs were Receptor Tyrosine Kinase-Ras (RTK-Ras) signaling pathway (n=20), NOTCH (n=16), and Wnt (n=16) pathways (Supplementary Figure 2). Few non-functioning PNETs had acquired ≥2 variants in the same pathway.
Figure 8 Top oncogenic pathway enriched in (A) non-functioning (n=30) and (B) functioning (n=5) pancreatic neuroendocrine tumors (PNETs). The oncogenic pathways uncovered in PNETs are based on somatic mutations. The oncogenic signaling pathways are based on pathways from The Cancer Genome Atlas (TCGA) cohorts. Genes highlighted in red are tumor suppressor genes, and genes highlighted in blue are proto-oncogenes. Each column indicates a PNET and a somatic mutation is represented by a red box in the row of the altered gene. PI3K, Phosphoinositide 3-kinase; RTK-RAS, Receptor Tyrosine Kinase-Ras.
Functioning PNETs did not share any variants in relations to annotated onco-pathways, however six genes were altered in five different PNETs in association to the RTK-Ras (Figure 8B). No other onco-pathway was enriched for more than 10% of functioning PNETs.
In this meta-analysis of published somatic mutations in G1/G2 PNETs, MEN1 was the most frequently altered gene across all PNETs no matter heredity and functionality. DAXX and ATRX were also abundant in hereditary, sporadic and non-functioning PNETs, but rare in functioning PNETs, in which the recurrent YY1 variant (p.Thr372Arg) was significantly enriched. Only non-functioning PNETs had enrichment of onco-pathways.
MEN1 point mutations was identified in 30% of the analyzed tumors and 42% when including CNVs. Consistently, previous smaller individual studies with or without CNV analyses reported a frequency of 25–44% (11–14). In PNETs from MEN1 patients, MEN1 was somatically altered as a second hit in all 13 with available data. Inactivation of a second MEN1 allele may happen due to LOH, point mutations, or epigenetic inactivation. In our dataset, 92% (12/13) of the MEN1 patients acquired LOH in the MEN1 locus as the somatic second hit (one patient had no MEN1 somatic data). Likewise, LOH has earlier been proposed to be the main mechanism of full MEN1 inactivation, accounting for 90% of PNETs in MEN1 patients (41). MEN1 patients did not share other somatic gene mutations, which may reflect the sovereignty of menin deficiency in their PNET development.
In sporadic PNETs, Jiao et al. identified point mutations/indels in MEN1 in 30/68 (44%) of the examined cohort. (12). In our meta-analyses, which did not include this study, 52/162 (32%) sporadic PNETs harbored somatic MEN1 point mutations/indels. Only six sporadic PNETs had a CNV of the MEN1 locus, and CNVs in MEN1 were significantly more frequent in hereditary PNETs compared to sporadic PNETs. This difference may, however, be assigned the low number of CNV data in sporadic PNETs. Somatic MEN1 alterations were equally distributed in non-functioning vs. functioning PNETs with a high frequency of CNVs in each group. This further highlights the need of CNV analyses in all subtypes of PNETs when studying their tumorigenesis.
DAXX and ATRX were the second and third most frequently altered genes in our meta-analysis. Two patients with hereditary MEN1 had a somatic alteration in both MEN1 and DAXX. The heterogeneity of ATRX and DAXX mutations has been explored earlier and it was also apparent in the cohort of PNETs. Only one variant in DAXX was altered in two PNETs, the remaining DAXX-mutations were exclusive and specific to each PNET.
A mutational hotspot in DAXX has earlier been proposed, resulting in alterations in the amino acid NM_001141970.1, Serine 102; p.(Ser102Leu) and p.(Ser102*) (42). In our dataset, which did not include the mentioned study, we did not identify variants altering Serine 102. Instead, we identified another hotspot for three non-synonymous missense variations in DAXX spanning from amino acid 328 to 331, located in a histone binding domain (amino acids 178–389) of the protein (40). Indeed, Jiao et al. (12)also identified a DAXX missense variant in this domain p.(Ala297Pro) and an in-frame deletion p.(Leu309GlnPhe), and Quevedo et al.(43) identified a p.(Ala313Val) variant in a PNET. Taken together, the DAXX histone-binding domain 178–389 represents a new mutational hotspot in PNETs, although DAXX mutations were widespread.
ATRX mutations were also unique in regard to the position in the gene and were only observed once and with no tendency toward specific mutation types. The six somatic missense mutations in ATRX were all predicted probably damaging. Five of the identified missense mutations, (p.(His1759Asp), p.(Met1800Ile), p.(Lys2036Glu), p.(Ser2116Phe), and p.(Tyr2176Cys), were clustered in the C-terminal ATPase domain (amino acid 1,550–2,226) of ATRX (40). This domain contains seven conserved motifs responsible for the ATP-hydrolysis and may further be responsible for DNA translocase activity (44, 45). Experimental evidence has shown that this domain is DNA-dependent and may be associated with chromatin remodeling or DNA replication (46). The variant p.(Lys2036Glu) is placed within the highly conserved helicase domain IV (47, 48). The five mutations could have a noticeable effect on the function of ATRX, and may hinder the ATPase activity of the protein. The mutational hotspot may indicate, that this function is pivotal for endocrine cells, as the interaction with DAXX at H3.3 still may be intact. Interestingly, other PNET study data from Jiao et al. and Quevedo et al. showed a total of four ATRX missense variants, all clustering in the ATPase domain (12, 43), supporting the importance of the ATRX ATPase activity in PNETs.
In the sporadic cohort, DAXX and ATRX were mutually exclusive except for one PNET which had a CNV in both genes. This further strengthens the potential for DAXX and ATRX alterations as independent tumor drivers in PNETs. Furthermore, DAXX was altered significantly more in non-functioning compared to functioning PNETs, indicating a frequent role of DAXX in the tumorigenesis of non-functioning PNETs. In functioning PNETs, only one DAXX and one ATRX alteration was seen in a glucagonoma, and an insulinoma, respectively. ATRX and DAXX mutations has previously been associated with a more alpha cell-like phenotype in a study not included in the present meta-analysis (49).
YY1 mutations was especially frequent in insulinomas, in which 11% harbored a point mutation or indel. Mutations in YY1 was less frequently seen in the meta-analysis compared to the included Asian study of Cao et al., where up to 30% of insulinomas had YY1 alterations (15). The recurrent YY1 mutation p.(Thr372Arg) occurred in 14% in our analysis, narrowing the frequency of 8–30% of reported in three individual studies, of which only the study by Cao et al. was included in our meta-analysis (15–17). Of note, Lichtenhauer et al. found a lower prevalence of YY1 alterations of 12% in insulinomas from their Caucasian cohort (16), compared to sporadic insulinomas from an Asian cohort (15).
Surprisingly, YY1 was not exclusively altered in insulinomas in our datasets. One non-functioning tumor also harbored the characteristic p.(Thr372Arg) mutation, and the YY1 region was amplified in nine, especially non-functioning, PNETs. The latter may indicate that YY1 could be a proto-oncogene just as observed for the gene amplifications and overexpression of MDM2 in cancer (50). YY1 codes for the potential proto-oncogenic ying yang 1 transcription factor (51), confirming the relevance of YY1 in tumorigenesis.
In hereditary PNETs from other than MEN1 patients, germline mutations were observed in MUTYH, CHEK2, BRCA2, VHL, PTEN, and CDKN1B, of which one had germline mutations in both CHEK2 and MUTYH. Furthermore, one MEN1 patient had germline mutations in both MEN1 and MUTYH. Moreover, DAXX variants occurred in four patients with CHEK2 and/or MUTYH germline presentation, highlighting the need of expanded genetics in PNET tumorigenesis research.
Of the six patients with germline MUTYH variants, three of the variants were predicted as pathogenic (13). Our datasets indicated a high level of tumor mutational burden in these individuals, even though the variant numbers between datasets should be compared with caution.
While the MUTYH protein is involved in base-excision-repair during DNA damage (52), BRCA2 and CHK2 are members of the homologous recombination pathway, repairing double-stranded DNA breaks (53). A BRCA germline variant was detected in one patient, and CHEK2 germline variants were identified in four patients. Genomic instability caused by defective DNA repair proteins is a well-described hallmark of cancer (54). Accordingly, patients with germline variants in MUTYH, BRCA2 or CHEK2 should be considered at risk of PNET development, as well as at risk for hereditary cancer (55). The presence of BRCA2 germline variants in pancreatic lesions, including PNETs, has been described earlier (13, 14, 56–58).
Germline mutations in VHL causing von-Hippel Lindau syndrome appeared in five patients from our datasets. Development of PNETs in this rare syndrome is seen in 17% (273/2,330) of all VHL patients according to The European-American-Asian-VHL-panNET-Registry (59, 60). Especially variants in exon 3 have been associated with malignancy (59, 61). Of the five patients with a VHL germline alteration, one had a missense variant in exon 3 and somatic LOH of the second allele and was metastasis-free (13). Somatically, we identified eight VHL variants in the whole dataset, of which one was a frameshift mutation and seven were missense variants distributed in all three exons. However, only a single of these PNETs had metastasis and this variant was in exon 2. Seven of the eight PNETs with VHL variants were non-functioning. This predominance of non-functioning PNETs is in line with other studies (61, 62).
Notably, one PNET-patient included in our datasets, had been diagnosed with Cowden syndrome, harboring a deleterious germline PTEN-variant (34). A case report of the patient has been published (63), highlighting the rarity of the association of PNET development and the syndrome. Lastly, one PNET harbored a germline variant in CDKN1B. Loss of germline CDKN1B is referred to as MEN4 syndrome and can cause a MEN1-like phenotype. Only very few PNETs have been described having germline variants in CDKN1B (64, 65). Notably, patients with hereditary PNETs were significantly younger than patients with sporadic PNETs, as described earlier (1, 66).
PNETs, which are mostly non-functioning, have been associated with variants in AKT/PI3K/mTOR pathway, i.e. PTEN, TSC1, TSC2, PIK3CA and DEPDC5, which regulates cell survival and proliferation (13, 67). It has been suggested that patients with mTOR pathway aberrations may benefit from therapy using mTOR inhibitors (12, 13). In our combined datasets, we also found variants in the PI3K/mTOR pathway, especially in the non-functioning tumors (30/156 PNETs). The most abundant altered genes were coding for PTEN (n=9), TSC2 (n=6), TSC1 (n=5) and MTOR (n=4). Only one tumor had mutations in more than one gene encoding a PI3K/mTOR pathway interactor. Interestingly, the use of everolimus in non-functioning NETs improved the progression-free survival compared to placebo (11 vs 3.9 months, RADIANT-4 trial, (68)), and although the documented use of everolimus in functioning NETs is sparse, mTOR-inhibitor treatment may also affect the clinical symptoms, independent of restrain of tumor growth (69, 70).
RTK-Ras (n=20), Wnt pathway (n=16) and NOTCH (n=16) pathways were also altered in more than 10% of non-functioning PNET. While most of the NOTCH associated proteins were tumor suppressors, the RTK-Ras pathway mostly consists of proto-oncogenes (Supplementary Figure 2), and a maximum of three non-functioning PNETs had mutations in the same gene (ERBB2, AXIN2, and NOTCH2), thereby indicating common pathway variations, but not necessarily gene specific alterations also among the non-functioning tumors. The identification of aberrant pathways may in turn give insight into potential targets for drug designs in the future. Drugs targeting the distinct protooncogenic pathways RTK-Ras, Wnt and NOTCH do already exist. These include for example the multi-kinase inhibitor sorafenib (71), which may impact the entire pathway. For the Wnt and NOTCH-pathways only very few FDA approved drugs are available, however a few new pathway-specific drugs have entered clinical trials (72, 73).
Strengths our study included the systematic search for eligible articles and, by nature of a meta-analysis, our large sample size from 14 datasets compared to individual datasets. Moreover, our inclusion criteria of reported G1/G2 PNETs ensured a focus on wd-PNETs, as G3 PNET is a newly defined entity, not incorporated in most previous studies.
In the WHO version of 2010, wd-NETs of low to intermediate grade was defined as G1-G2, whereas poorly differentiated, high-grade neoplasms were defined as G3 (74). In 2017, WHO altered the definition and introduced a G3 NET described as well-defined with a distinct morphology compared to G3 neuroendocrine carcinomas (NECs) (75). G3-NET shares a molecular mutation profile similar to G1 and G2 NET and are associated with better survival than the poorly differentiated G3 NEC (76), which also has a mutational profile more similar to pancreatic ductal adenocarcinomas (77). G3 NETs may be difficult to distinguish from (G3) NECs, as both entities share a high proliferation rate (Ki67 proliferation index > 20% and/or mitotic rate above 20 per high power field) (78). New studies on wd-PNETs should, however, include G3 PNETs according to the new WHO classification.
Limitations included the varying methods in the included articles, which may have affected the results. The variations include different tissue preparations (FFPE or frozen), different type of library preparations (with or without gene enrichment), different sequencing platform, and resultant different read depth. Six of the articles did not describe the average read depth, although for one article the quality of the sequencing was described using Q30 (27), which indicates the likelihood of incorrect base calling in 1 of 1000 times (79). The differences between the article’s methods may be reflected by the variant calling, as some articles presented with very few variants (28, 29, 34) compared to others (32, 33, 80). Of note, after retrieving the variants, we excluded synonymous and intronic variants, even though such variants can alter the splicing of genes (81).
Moreover, CNV information could only be gathered from 33% of the PNETs, of which we only included structural variations, i.e. amp, del, and cnLOH as structural variations, e.g. tandem duplications, inversions, and translocations, have unpredictable consequences and such variations were only described in one of the 14 included studies (n=33) (32). Underreporting of clinically important CNVs could, therefore, not be ruled out.
Lastly, patients without a known germline predisposition were termed ‘sporadic’, even though most articles did not specify other germline gene results but for MEN1. This may lead to underreporting of rarer germline gene variants in PNETs such as VHL, PTEN, CDKN1B, BRCA2, CHEK2, and MUTYH.
In 225 G1/G2 PNETs, MEN1 was most frequently somatically altered in all patient groups. DAXX and ATRX were abundant in hereditary, sporadic and non-functioning PNETs, but rare in functioning PNETs. Whereas MEN1 mutations were distributed throughout the gene, DAXX, and ATRX, missense variants were clustered in mutational hotspots associated with histone binding, and translocase activity, respectively. In functioning PNETs, the well-known YY1 variant (p.Thr372Arg) was significantly enriched while few other gene alterations were shared. Non-functioning PNETs had more recurrent variations in genes associated with the PI3K, Wnt, NOTCH, and RTK -Ras signaling onco-pathways.
Our review of PNET variations may contribute to the overall understanding of the genetic alterations in PNETs. Future studies on expanded genetics in PNETs should precisely describe the functional status and germline dispositions, including not only MEN1, but expanded germline gene analyses, and expanded CNV analyses of the PNETs. Genotype-phenotype correlations should be strengthened not only for PNETs with a single gene alteration, but also for PNETs with co-occurrence of more than one possibly oncogenic gene alteration. The meta-analysis could be helpful in the search for new targeted treatment approaches in PNETs. Future approaches to characterize PNETs could involve genomic, transcriptomic, proteomic and importantly epigenetic analyses to better understand the complexity of the tumorigenesis.
Publicly available datasets were analyzed in this study. This data can be found here: (13): PMID: 28199314 DOI: 10.1038/nature21063; (15): PMID: 24326773 DOI: 10.1038/ncomms3810; (27): PMID: 29435419 DOI: 10.1002/2211-5463.12366; (19): PMID: 28974674 DOI: 10.1038/s41467-017-00992-9; (28): PMID: 28503312 DOI: 10.1038/hgv.2017.13; (29): PMID: 33840689 DOI: 10.2220/biomedres.42.89; (30): PMID: 20857520 DOI: 10.3748/wjg.v16.i36.4515; (14): PMID: 33747156 DOI: 10.3892/etm.2021.9859; (31): PMID: 29725435 DOI: 10.3892/ol.2018.8184; (32): PMID: 34880079 DOI: 10.1158/2159-8290.CD-21-0669; (33): PMID: 35794609 DOI: 10.1186/s12967-022-03511-7; (34): PMID: 30865533 DOI: 10.4158/EP-2018-0603; (35): PMID: 34644566 DOI: 10.1016/j.celrep.2021.109817; (36): PMID: 37099786 DOI: 10.1097/MPA.0000000000002199.
KA: Conceptualization, Data curation, Formal analysis, Project administration, Software, Writing – original draft, Writing – review & editing. SD: Supervision, Writing – review & editing. KB: Data curation, Supervision, Writing – review & editing. HC: Supervision, Writing – original draft, Writing – review & editing.
The author(s) declare financial support was received for the research, authorship, and/or publication of this article. This work was supported by Novo Nordisk Foundation, grant number NNF19OC0057915. The funder was not involved in the study design, collection, analysis, interpretation of data, the writing of this article or the decision to submit it for publication.
The authors declare that the research was conducted in the absence of any commercial or financial relationships that could be construed as a potential conflict of interest.
All claims expressed in this article are solely those of the authors and do not necessarily represent those of their affiliated organizations, or those of the publisher, the editors and the reviewers. Any product that may be evaluated in this article, or claim that may be made by its manufacturer, is not guaranteed or endorsed by the publisher.
The Supplementary Material for this article can be found online at: https://www.frontiersin.org/articles/10.3389/fendo.2024.1351624/full#supplementary-material
1. Marini F, Giusti F, Tonelli F, Brandi ML. Pancreatic neuroendocrine neoplasms in multiple endocrine neoplasia type 1. Int J Mol Sci. (2021) 22. doi: 10.3390/ijms22084041
2. Ro C, Chai W, Yu VE, Yu R. Pancreatic neuroendocrine tumors: biology, diagnosis,and treatment. Chin J Cancer. (2013) 32:312–24. doi: 10.5732/cjc.012.10295
3. Knudson AG. Mutation and cancer: statistical study of retinoblastoma. Proc Natl Acad Sci. (1971) 68:820–3. doi: 10.1073/pnas.68.4.820
4. Knudson AG. Antioncogenes and human cancer. Proc Natl Acad Sci U.S.A. (1993) 90:10914–21. doi: 10.1073/pnas.90.23.10914
5. Yang YJ, Song TY, Park J, Lee J, Lim J, Jang H, et al. Menin mediates epigenetic regulation via histone H3 lysine 9 methylation. Cell Death Dis. (2013) 4:e583. doi: 10.1038/cddis.2013.98
6. Lin W, Watanabe H, Peng S, Francis JM, Kaplan N, Pedamallu CS, et al. Dynamic epigenetic regulation by menin during pancreatic islet tumor formation. Mol Cancer Res. (2015) 13:689–98. doi: 10.1158/1541-7786.MCR-14-0457
7. Feng Z, Ma J, Hua X. Epigenetic regulation by the menin pathway. Endocr Relat Cancer. (2017) 24:T147–t159. doi: 10.1530/ERC-17-0298
8. Wu G, Yuan M, Shen S, Ma X, Fang J, Zhu L, et al. Menin enhances c-Myc-mediated transcription to promote cancer progression. Nat Commun. (2017) 8:15278. doi: 10.1038/ncomms15278
9. Wagener N, Buchholz M, Bertolino P, Zhang CX, Di Fazio P. Exploring the MEN1 dependent modulation of caspase 8 and caspase 3 in human pancreatic and murine embryo fibroblast cells. Apoptosis. (2022) 27:70–9. doi: 10.1007/s10495-021-01700-1
10. Marek LR, Kottemann MC, Glazer PM, Bale AE. MEN1 and FANCD2 mediate distinct mechanisms of DNA crosslink repair. DNA Repair (Amst). (2008) 7:476–86. doi: 10.1016/j.dnarep.2007.12.009
11. Corbo V, Dalai I, Scardoni M, Barbi S, Beghelli S, Bersani S, et al. MEN1 in pancreatic endocrine tumors: analysis of gene and protein status in 169 sporadic neoplasms reveals alterations in the vast majority of cases. Endocr Relat Cancer. (2010) 17:771–83. doi: 10.1677/ERC-10-0028
12. Jiao Y, Shi C, Edil BH, de Wilde RF, Klimstra DS, Maitra A, et al. DAXX/ATRX, MEN1, and mTOR pathway genes are frequently altered in pancreatic neuroendocrine tumors. Science. (2011) 3316021:1199–203. doi: 10.1126/science.1200609
13. Scarpa A, Chang DK, Nones K, Corbo V, Patch A-M, Bailey P, et al. Whole-genome landscape of pancreatic neuroendocrine tumours. Nature. (2017) 543:65–71. doi: 10.1038/nature21063
14. Zheng K, Liu T, Zhao J, Meng P, Bian Y, Ni C, et al. Mutational landscape and potential therapeutic targets for sporadic pancreatic neuroendocrine tumors based on target next-generation sequencing. Exp Ther Med. (2021) 21:415. doi: 10.3892/etm
15. Cao Y, Gao Z, Li L, Jiang X, Shan A, Cai J, et al. Whole exome sequencing of insulinoma reveals recurrent T372R mutations in YY1. Nat Commun. (2013) 4:2810. doi: 10.1038/ncomms3810
16. Lichtenauer UD, Di Dalmazi G, Slater EP, Wieland T, Kuebart A, Schmittfull A, et al. Frequency and clinical correlates of somatic Ying Yang 1 mutations in sporadic insulinomas. J Clin Endocrinol Metab. (2015) 100:E776–782. doi: 10.1210/jc.2015-1100
17. Parekh VI, Modali SD, Welch J, Simonds WF, Weinstein LS, Kebebew E, et al. Frequency and consequence of the recurrent YY1 p.T372R mutation in sporadic insulinomas. Endocr Relat Cancer. (2018) 25:L31–l35. doi: 10.1530/ERC-17-0311
18. Challis BG, Powlson AS, Casey RT, Pearson C, Lam BY, Ma M, et al. Adult-onset hyperinsulinaemic hypoglycaemia in clinical practice: diagnosis, aetiology and management. Endocr Connect. (2017) 6:540–8. doi: 10.1530/EC-17-0076
19. Wang H, Bender A, Wang P, Karakose E, Inabnet WB, Libutti SK, et al. Insights into beta cell regeneration for diabetes via integration of molecular landscapes in human insulinomas. Nat Commun. (2017) 8:767. doi: 10.1038/s41467-017-00992-9
20. Raymond E, Dahan L, Raoul J-L, Bang Y-J, Borbath I, Lombard-Bohas C, et al. Sunitinib malate for the treatment of pancreatic neuroendocrine tumors. New Engl J Med. (2011) 364:501–13. doi: 10.1056/NEJMoa1003825
21. Yao JC, Shah MH, Ito T, Bohas CL, Wolin EM, Van Cutsem E, et al. Everolimus for advanced pancreatic neuroendocrine tumors. N Engl J Med. (2011) 364:514–23. doi: 10.1056/NEJMoa1009290
22. Vandamme T, Beyens M, de Beeck KO, Dogan F, van Koetsveld PM, Pauwels P, et al. Long-term acquired everolimus resistance in pancreatic neuroendocrine tumours can be overcome with novel PI3K-AKT-mTOR inhibitors. Br J Cancer. (2016) 114:650–8. doi: 10.1038/bjc.2016.25
23. Page MJ, McKenzie JE, Bossuyt PM, Boutron I, Hoffmann TC, Mulrow CD, et al. The PRISMA 2020 statement: an updated guideline for reporting systematic reviews. Bmj. (2021) 372:n71. doi: 10.1136/bmj.n71
24. Guilmette JM, Nosé V. Neoplasms of the neuroendocrine pancreas: an update in the classification, definition, and molecular genetic advances. Adv Anat Pathol. (2019) 26:13–30. doi: 10.1097/PAP.0000000000000201
25. Lloyd RV, Osamura RY, Klöppel G, Rosai J. WHO Classification of Tumours of Endocrine Organs. Lyon, France: International Agency for Research on Cancer (2017).
26. Nagahashi M, Wakai T, Shimada Y, Ichikawa H, Kameyama H, Kobayashi T, et al. Genomic landscape of colorectal cancer in Japan: clinical implications of comprehensive genomic sequencing for precision medicine. Genome Med. (2016) 8:136. doi: 10.1186/s13073-016-0387-8
27. Qi C, Duan J, Shi Q, Wang M, Yan C. Two nonsense somatic mutations in MEN1 identified in sporadic insulinomas. FEBS Open Bio. (2018) 8:295–301. doi: 10.1002/2211-5463.12366
28. Naruoka A, Ohnami S, Nagashima T, Serizawa M, Ohshima K, Ohnami S, et al. Germline and somatic genetic changes in multicentric tumors obtained from a patient with multiple endocrine neoplasia type 1. Hum Genome Var. (2017) 4:17013. doi: 10.1038/hgv.2017.13
29. Naruoka A, Ohnami S, Nagashima T, Serizawa M, Hatakeyama K, Ohshima K, et al. Genomic profiling of multiple tissues in two patients with multiple endocrine neoplasia type 1. BioMed Res. (2021) 42:89–94. doi: 10.2220/biomedres.42.89
30. Tamura A, Ogasawara T, Fujii Y, Kaneko H, Nakayama A, Higuchi S, et al. Glucagonoma with necrolytic migratory erythema: metabolic profile and detection of biallelic inactivation of DAXX gene. J Clin Endocrinol Metab. (2018) 103:2417–23. doi: 10.1210/jc.2017-02646
31. Wang Z, Liu L, Luo J, Guo J, Zhai M, Zhang W, et al. Uncovering the heterogeneous genetic variations in two insulin-expressing tumors in a patient with MEN1. Oncol Lett. (2018) 15:7123–31. doi: 10.3892/ol
32. Yachida S, Totoki Y, Noë M, Nakatani Y, Horie M, Kawasaki K, et al. Comprehensive genomic profiling of neuroendocrine carcinomas of the gastrointestinal system. Cancer Discovery. (2022) 12:692–711. doi: 10.1158/2159-8290.CD-21-0669
33. Melone V, Salvati A, Palumbo D, Giurato G, Nassa G, Rizzo F, et al. Identification of functional pathways and molecular signatures in neuroendocrine neoplasms by multi-omics analysis. J Transl Med. (2022) 20:306. doi: 10.1186/s12967-022-03511-7
34. Tirosh A, Killian JK, Zhu YJ, Petersen D, Walling J, Mor-Cohen R, et al. ONCOGENE PANEL SEQUENCING ANALYSIS IDENTIFIES CANDIDATE ACTIONABLE GENES IN ADVANCED WELL-DIFFERENTIATED GASTROENTEROPANCREATIC NEUROENDOCRINE TUMORS. Endocr Pract. (2019) 25:580–8. doi: 10.4158/EP-2018-0603
35. Yang KC, Kalloger SE, Aird JJ, Lee MKC, Rushton C, Mungall KL, et al. Proteotranscriptomic classification and characterization of pancreatic neuroendocrine neoplasms. Cell Rep. (2021) 37:109817. doi: 10.1016/j.celrep.2021.109817
36. Hu HF, Hu YH, Xu XW, Ye Z, Lou X, Zhang WH, et al. Role of somatostatin receptor 2 in nonfunctional pancreatic neuroendocrine tumors: clinicopathological analysis of 223 cases and whole exome sequencing of a multifocal case. Pancreas. (2022) 51:1404–10. doi: 10.1097/MPA.0000000000002199
37. Zhou W, Chen T, Chong Z, Rohrdanz MA, Melott JM, Wakefield C, et al. TransVar: a multilevel variant annotator for precision genomics. Nat Methods. (2015) 12:1002–3. doi: 10.1038/nmeth.3622
38. Mayakonda A, Lin D-C, Assenov Y, Plass C, Koeffler HP. Maftools: efficient and comprehensive analysis of somatic variants in cancer. Genome Res. (2018) 28:1747–56. doi: 10.1101/gr.239244.118
39. Adzhubei IA, Schmidt S, Peshkin L, Ramensky VE, Gerasimova A, Bork P, et al. A method and server for predicting damaging missense mutations. Nat Methods. (2010) 7:248–9. doi: 10.1038/nmeth0410-248
40. Wang X, Zhao Y, Zhang J, Chen Y. Structural basis for DAXX interaction with ATRX. Protein Cell. (2017) 8:767–71. doi: 10.1007/s13238-017-0462-y
41. Lemos MC, Thakker RV. Multiple endocrine neoplasia type 1 (MEN1): analysis of 1336 mutations reported in the first decade following identification of the gene. Hum Mutat. (2008) 29:22–32. doi: 10.1002/humu.v29:1
42. Vandamme T, Beyens M, Boons G, Schepers A, Kamp K, Biermann K, et al. Hotspot DAXX, PTCH2 and CYFIP2 mutations in pancreatic neuroendocrine neoplasms. Endocrine-Related Cancer. (2019) 26:1–12. doi: 10.1530/ERC-18-0120
43. Quevedo R, Spreafico A, Bruce J, Danesh A, El Ghamrasni S, Giesler A, et al. Centromeric cohesion failure invokes a conserved choreography of chromosomal mis-segregations in pancreatic neuroendocrine tumor. Genome Med. (2020) 12:38. doi: 10.1186/s13073-020-00730-9
44. Gibbons RJ, Bachoo S, Picketts DJ, Aftimos S, Asenbauer B, Bergoffen J, et al. Mutations in transcriptional regulator ATRX establish the functional significance of a PHD-like domain. Nat Genet. (1997) 17:146–8. doi: 10.1038/ng1097-146
45. Aguilera P, López-Contreras AJ. ATRX, a guardian of chromatin. Trends Genet. (2023) 39:505–19. doi: 10.1016/j.tig.2023.02.009
46. Xue Y, Gibbons R, Yan Z, Yang D, McDowell TL, Sechi S, et al. The ATRX syndrome protein forms a chromatin-remodeling complex with Daxx and localizes in promyelocytic leukemia nuclear bodies. Proc Natl Acad Sci U.S.A. (2003) 100:10635–40. doi: 10.1073/pnas.1937626100
47. Mitson M, Kelley LA, Sternberg MJ, Higgs DR, Gibbons RJ. Functional significance of mutations in the Snf2 domain of ATRX. Hum Mol Genet. (2011) 20:2603–10. doi: 10.1093/hmg/ddr163
48. Bhattacharyya B, Keck JL. Grip it and rip it: structural mechanisms of DNA helicase substrate binding and unwinding. Protein Sci. (2014) 23:1498–507. doi: 10.1002/pro.2533
49. Chan CS, Laddha SV, Lewis PW, Koletsky MS, Robzyk K, Da Silva E, et al. ATRX, DAXX or MEN1 mutant pancreatic neuroendocrine tumors are a distinct alpha-cell signature subgroup. Nat Commun. (2018) 9:4158. doi: 10.1038/s41467-018-06498-2
50. Hou H, Sun D, Zhang X. The role of MDM2 amplification and overexpression in therapeutic resistance of Malignant tumors. Cancer Cell Int. (2019) 19:216. doi: 10.1186/s12935-019-0937-4
51. Zhang Q, Stovall DB, Inoue K, Sui G. The oncogenic role of Yin Yang 1. Crit Rev Oncog. (2011) 16:163–97. doi: 10.1615/CritRevOncog.v16.i3-4
52. Markkanen E, Dorn J, Hübscher U. MUTYH DNA glycosylase: the rationale for removing undamaged bases from the DNA. Front Genet. (2013) 4. doi: 10.3389/fgene.2013.00018
53. Zhang J, Willers H, Feng Z, Ghosh JC, Kim S, Weaver DT, et al. Chk2 phosphorylation of BRCA1 regulates DNA double-strand break repair. Mol Cell Biol. (2004) 24:708–18. doi: 10.1128/MCB.24.2.708-718.2004
54. Negrini S, Gorgoulis VG, Halazonetis TD. Genomic instability–an evolving hallmark of cancer. Nat Rev Mol Cell Biol. (2010) 11:220–8. doi: 10.1038/nrm2858
55. Yamamoto H, Hirasawa A. Homologous recombination deficiencies and hereditary tumors. Int J Mol Sci. (2021) 23. doi: 10.3390/ijms23010348
56. Sharma MB, Carus A, Sunde L, Hamilton-Dutoit S, Ladekarl M. BRCA-associated pancreatico-biliary neoplasms: Four cases illustrating the emerging clinical impact of genotyping. Acta Oncol. (2016) 55:377–81. doi: 10.3109/0284186X.2015.1044023
57. Ji S, Yang W, Liu J, Zhao J, Chen L, Ni Q, et al. High throughput gene sequencing reveals altered landscape in DNA damage responses and chromatin remodeling in sporadic pancreatic neuroendocrine tumors. Pancreatology. (2018) 18:318–27. doi: 10.1016/j.pan.2018.01.006
58. Finnerty BM, Moore MD, Verma A, Aronova A, Huang S, Edwards DP, et al. UCHL1 loss alters the cell-cycle in metastatic pancreatic neuroendocrine tumors. Endocr Relat Cancer. (2019) 26:411–23. doi: 10.1530/ERC-18-0507
59. Krauss T, Ferrara AM, Links TP, Wellner U, Bancos I, Kvachenyuk A, et al. Preventive medicine of von Hippel-Lindau disease-associated pancreatic neuroendocrine tumors. Endocr Relat Cancer. (2018) 25:783–93. doi: 10.1530/ERC-18-0100
60. Coco D, Leanza S. Von hippel-lindau is associated to pancreatic neuroendocrine tumors: A comprehensive review. J Kidney Cancer VHL. (2023) 10:13–20. doi: 10.15586/jkcvhl.v10i2.272
61. Libutti SK, Choyke PL, Alexander HR, Glenn G, Bartlett DL, Zbar B, et al. Clinical and genetic analysis of patients with pancreatic neuroendocrine tumors associated with von Hippel-Lindau disease. Surgery. (2000) 128:1022–1027;discussion 1027–1028. doi: 10.1067/msy.2000.110239
62. Tamura K, Nishimori I, Ito T, Yamasaki I, Igarashi H, Shuin T. Diagnosis and management of pancreatic neuroendocrine tumor in von Hippel-Lindau disease. World J Gastroenterol. (2010) 16:4515–8. doi: 10.3748/wjg.v16.i36.4515
63. Neychev V, Sadowski SM, Zhu J, Allgaeuer M, Kilian K, Meltzer P, et al. Neuroendocrine tumor of the pancreas as a manifestation of cowden syndrome: A case report. J Clin Endocrinol Metab. (2016) 101:353–8. doi: 10.1210/jc.2015-3684
64. Alrezk R, Hannah-Shmouni F, Stratakis CA. MEN4 and CDKN1B mutations: the latest of the MEN syndromes. Endocr Relat Cancer. (2017) 24:T195–t208. doi: 10.1530/ERC-17-0243
65. Seabrook A, Wijewardene A, De Sousa S, Wong T, Sheriff N, Gill AJ, et al. MEN4, the MEN1 mimicker: A case series of three phenotypically heterogenous patients with unique CDKN1B mutations. J Clin Endocrinol Metab. (2022) 107:2339–49. doi: 10.1210/clinem/dgac162
66. Capelli P, Martignoni G, Pedica F, Falconi M, Antonello D, Malpeli G, et al. Endocrine neoplasms of the pancreas: pathologic and genetic features. Arch Pathol Lab Med. (2009) 133:350–64. doi: 10.5858/133.3.350
67. Roy S, LaFramboise WA, Liu TC, Cao D, Luvison A, Miller C, et al. Loss of chromatin-remodeling proteins and/or CDKN2A associates with metastasis of pancreatic neuroendocrine tumors and reduced patient survival times. Gastroenterology. (2018) 154:2060–2063.e2068. doi: 10.1053/j.gastro.2018.02.026
68. Yao JC, Fazio N, Singh S, Buzzoni R, Carnaghi C, Wolin E, et al. Everolimus for the treatment of advanced, non-functional neuroendocrine tumours of the lung or gastrointestinal tract (RADIANT-4): a randomised, placebo-controlled, phase 3 study. Lancet. (2016) 387:968–77. doi: 10.1016/S0140-6736(15)00817-X
69. Ito T, Lee L, Jensen RT. Treatment of symptomatic neuroendocrine tumor syndromes: recent advances and controversies. Expert Opin Pharmacother. (2016) 17:2191–205. doi: 10.1080/14656566.2016.1236916
70. Lee L, Ito T, Jensen RT. Everolimus in the treatment of neuroendocrine tumors: efficacy, side-effects, resistance, and factors affecting its place in the treatment sequence. Expert Opin Pharmacother. (2018) 19:909–28. doi: 10.1080/14656566.2018.1476492
71. Bahar ME, Kim HJ, Kim DR. Targeting the RAS/RAF/MAPK pathway for cancer therapy: from mechanism to clinical studies. Signal Transduction Targeted Ther. (2023) 8:455. doi: 10.1038/s41392-023-01705-z
72. Pathak Y, Camps I, Mishra A, Tripathi V. Targeting notch signaling pathway in breast cancer stem cells through drug repurposing approach. Mol Divers. (2023) 27:2431–40. doi: 10.1007/s11030-022-10561-y
73. Pećina-Šlaus N, Aničić S, Bukovac A, Kafka A. Wnt signaling inhibitors and their promising role in tumor treatment. Int J Mol Sci. (2023) 24:6733. doi: 10.3390/ijms24076733
74. Rindi G, Petrone G, Inzani F. The 2010 WHO Classification of Digestive Neuroendocrine Neoplasms: a Critical Appraisal four years after Its Introduction. Endocrine Pathol. (2014) 25:186–92. doi: 10.1007/s12022-014-9313-z
75. Inzani F, Petrone G, Rindi G. The new world health organization classification for pancreatic neuroendocrine neoplasia. Endocrinol Metab Clin North Am. (2018) 47:463–70. doi: 10.1016/j.ecl.2018.04.008
76. Rindi G, Mete O, Uccella S, Basturk O, La Rosa S, Brosens LAA, et al. Overview of the 2022 WHO classification of neuroendocrine neoplasms. Endocr Pathol. (2022) 33:115–54. doi: 10.1007/s12022-022-09708-2
77. Venizelos A, Elvebakken H, Perren A, Nikolaienko O, Deng W, Lothe IMB, et al. The molecular characteristics of high-grade gastroenteropancreatic neuroendocrine neoplasms. Endocr Relat Cancer. (2021) 29:1–14. doi: 10.1530/ERC-21-0152
78. Nagtegaal ID, Odze RD, Klimstra D, Paradis V, Rugge M, Schirmacher P, et al. The 2019 WHO classification of tumours of the digestive system. Histopathology. (2020) 76:182–8. doi: 10.1111/his.13975
79. Ewing B, Green P. Base-calling of automated sequencer traces using phred. II. Error probabilities. Genome Res. (1998) 8:186–94. doi: 10.1101/gr.8.3.186
80. Scarpa A. The landscape of molecular alterations in pancreatic and small intestinal neuroendocrine tumours. Ann Endocrinol (Paris). (2019) 80:153–8. doi: 10.1016/j.ando.2019.04.010
Keywords: pancreatic neuroendocrine tumors, systematic review, meta-analysis, genetics, somatic, germline, MEN1, Knudson’s two-hit hypothesis
Citation: Andersen KØ, Detlefsen S, Brusgaard K and Christesen HT (2024) Well-differentiated G1 and G2 pancreatic neuroendocrine tumors: a meta-analysis of published expanded DNA sequencing data. Front. Endocrinol. 15:1351624. doi: 10.3389/fendo.2024.1351624
Received: 06 December 2023; Accepted: 02 May 2024;
Published: 29 May 2024.
Edited by:
Leo T. O. Lee, University of Macau, ChinaReviewed by:
Giulia Puliani, IRCCS Regina Elena National Cancer Institute, ItalyCopyright © 2024 Andersen, Detlefsen, Brusgaard and Christesen. This is an open-access article distributed under the terms of the Creative Commons Attribution License (CC BY). The use, distribution or reproduction in other forums is permitted, provided the original author(s) and the copyright owner(s) are credited and that the original publication in this journal is cited, in accordance with accepted academic practice. No use, distribution or reproduction is permitted which does not comply with these terms.
*Correspondence: Henrik Thybo Christesen, SGVucmlrLkNocmlzdGVzZW5AcnN5ZC5kaw==
†These authors share last authorship
Disclaimer: All claims expressed in this article are solely those of the authors and do not necessarily represent those of their affiliated organizations, or those of the publisher, the editors and the reviewers. Any product that may be evaluated in this article or claim that may be made by its manufacturer is not guaranteed or endorsed by the publisher.
Research integrity at Frontiers
Learn more about the work of our research integrity team to safeguard the quality of each article we publish.