- 1Reproductive Medical Center, People’s Hospital of Zhengzhou University, Zhengzhou, China
- 2Reproductive Medical Center, Henan Provincial People’s Hospital, Zhengzhou, China
Background: Identifying poor ovarian response (POR) among patients with good ovarian reserve poses a significant challenge within reproductive medicine. Currently, there is a lack of published data on the potential risk factors that could predict the occurrence of unexpected POR. The objective of this study was to develop a predictive model to assess the individual probability of unexpected POR during in vitro fertilization/intracytoplasmic sperm injection (IVF/ICSI) treatments.
Methods: The development of the nomogram involved a cohort of 10,404 patients with normal ovarian reserve [age, ≤40 years; antral follicle count (AFC), ≥5; and anti-Müllerian hormone (AMH), ≥1.2 ng/ml] from January 2019 to December 2022. Univariate regression analyses and least absolute shrinkage and selection operator regression analysis were employed to ascertain the characteristics associated with POR. Subsequently, the selected variables were utilized to construct the nomogram.
Results: The predictors included in our model were body mass index, basal follicle-stimulating hormone, AMH, AFC, homeostasis model assessment of insulin resistance (HOMA-IR), protocol, and initial dose of gonadotropin. The area under the receiver operating characteristic curve (AUC) was 0.753 [95% confidence interval (CI) = 0.7257–0.7735]. The AUC, along with the Hosmer–Lemeshow test (p = 0.167), demonstrated a satisfactory level of congruence and discrimination ability of the developed model.
Conclusion: The nomogram can anticipate the probability of unexpected POR in IVF/ICSI treatment, thereby assisting professionals in making appropriate clinical judgments and in helping patients to effectively manage expectations.
1 Introduction
During treatment of infertile couples, controlled ovarian stimulation (COS) can facilitate the synchronous growth of numerous follicles and oocyte maturation, thereby enhancing the oocyte yield, which plays a pivotal role in achieving in vitro fertilization–embryo transfer (IVF-ET) success. However, despite appropriate ovarian stimulation, some patients may still experience suboptimal outcomes with lower than anticipated numbers of retrieved oocytes. This condition is referred to as “poor ovarian response” (POR) (1).
The occurrence of POR ranges from 9% and 24% in the COS procedure (2), resulting in a decrease in ovarian response, a lower number of retrieved oocytes, a reduced rate of pregnancy, and an elevated likelihood of cycle cancellation and miscarriage (3). How to identify and manage the POR population has been a key area in reproductive medicine. The Bologna criteria, established by the European Society of Human Reproduction and Embryology (ESHRE), are universally acknowledged standards for POR (4). Populations defined as POR with distinct clinical features and prognoses are categorized together. Later, the “Patient-Oriented Strategies Encompassing Individualized Oocyte Number” (POSEIDON) criteria were proposed as novel low-prognosis categories for individuals with POR in 2016 (5). The POSEIDON criteria divide poor responders into four groups based on ovarian reserve markers, i.e., age, anti-Müllerian hormone (AMH), and antral follicle count (AFC), to better assess patients. Poor responders are further distributed into the “expected POR group” and the “unexpected POR group” according to POR heterogeneity. Both of these key criteria defined that a retrieval oocyte count ≤3 is POR. In existing studies, the identification of the POR population mainly relies on ovarian reserve markers. However, these biomarkers are suboptimal for predicting patients who are hyporesponders (6). Despite a good ovarian reserve function, a subset of patients may still exhibit POR. In the clinical setting, there is a scarcity of precise methods for predicting unexpected POR. The factors influencing POR occurrence are intricate and diverse. Factors such as body mass index (BMI), gonadotropin (Gn) dose, COS protocols, and insulin metabolism could also have an influence on the ovarian response. Hence, the aim of this study was to identify the factors that can predict unforeseen POR and develop a personalized prediction model in order to identify and implement individualized strategies for patients with unexpected POR. The prediction model was demonstrated as a nomogram that can be applied during patient counseling and clinical decision-making.
2 Materials and methods
2.1 Subjects
A retrospective analysis was conducted in patients who underwent IVF or intracytoplasmic sperm injection (ICSI) treatment in Henan Provincial People’s Hospital between January 1, 2019, and December 31, 2022. The criteria for inclusion were as follows: 1) first cycle of IVF/ICSI treatment; 2) 20 years ≤ age ≤ 40 years; 3) normal ovarian reserve function: AFC ≥ 5 and AMH ≥ 1.2 ng/ml; 4) gonadotropin-releasing hormone (GnRH) agonist or GnRH antagonist protocols; and 5) complete relevant records in the patient electronic medical record system. The exclusion criteria were the following: 1) pre-implantation genetic testing (PGT) cycle; 2) cycles involving oocyte cryopreservation or donation; 3) female chromosomal abnormalities or chromosomal polymorphisms; 4) coexisting factors that may affect ovarian response, such as history of ovarian surgery or pathological ovarian cyst; 5) uterine abnormalities; and 6) the following metabolic disorders: diabetes, hypertension, hyperprolactinemia, hyperthyroidism, hypothyroidism, and autoimmune disorders. The process for the inclusion and exclusion of patients is presented in Figure 1.
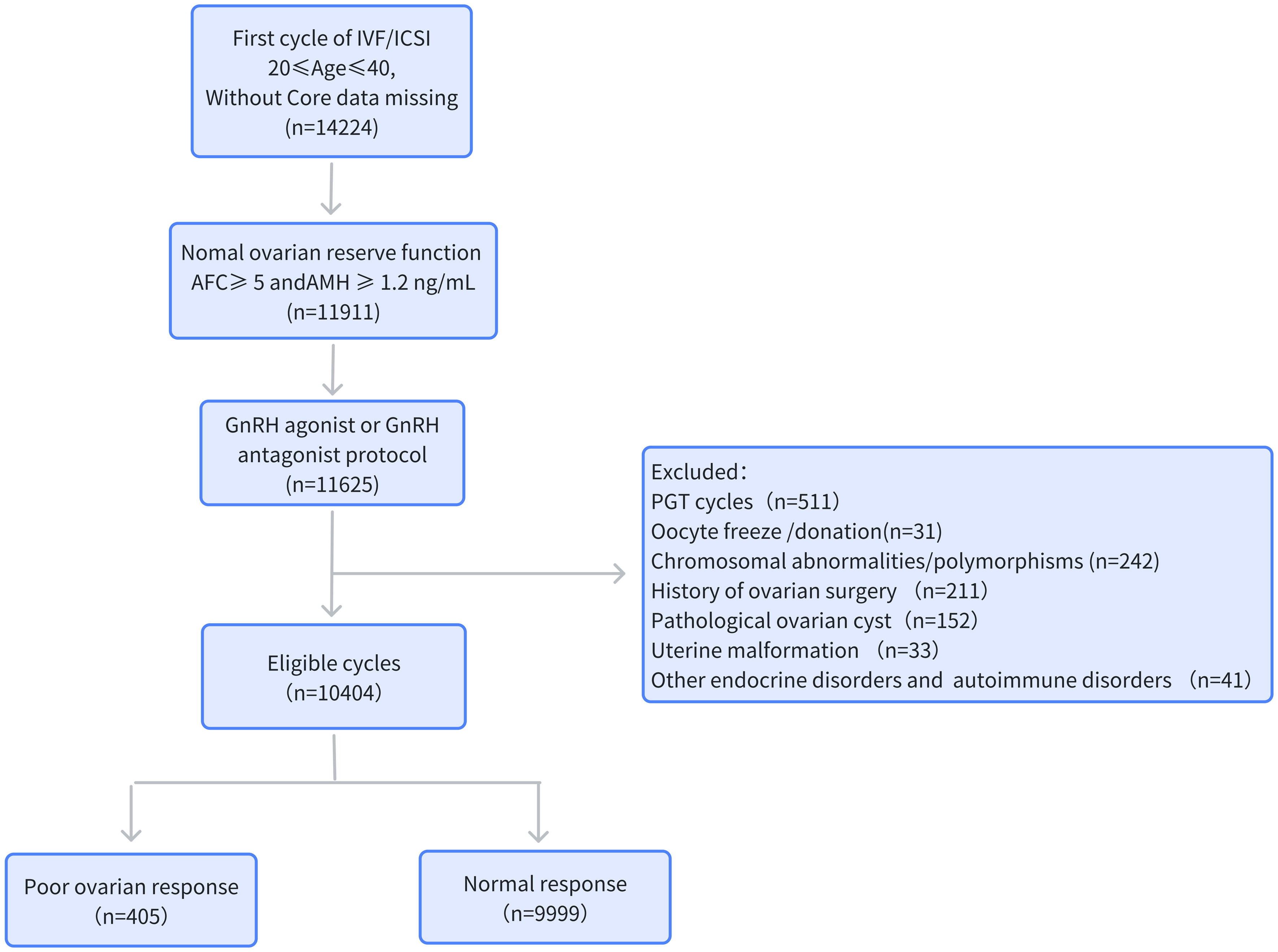
Figure 1 Flowchart of the data collection process. AMH, anti-Müllerian hormone; AFC, antral follicle count; BMI, body mass index; PGT, pre-implantation genetic testing.
According to the Bologna and the POSEIDON criteria, in this study, cases with no more than 3 were established as “POR.” All patients were categorized into two groups based on their ovarian response: the “unexpected poor ovarian response” group (POR group) and the “normal ovarian response” group (NOR group).
2.2 Data acquisition
The primary data for this study were derived from the electronic medical record system of our hospital, encompassing all assisted reproductive data. This analysis was authorized by the Henan Provincial People’s Hospital Ethics Committee (no. 2022139).
The basal sex hormone and AMH levels were measured on days 2–4 of the menstrual cycle in patients undergoing IVF/ICSI. The concentration of AMH was determined using the electrochemiluminescence immunoassay method [sensitivity = 0.1 ng/ml, coefficient of variation (CV) < 3.2%] on a fully automatic chemiluminescence immunoassay analyzer (Cobas8000 e602; Roche Diagnostics GmbH, Mannheim, Germany) in the laboratory of the Department of Reproductive Endocrinology at Henan Provincial People’s Hospital. Our laboratory undergoes annual qualification checks by the External Quality Assessment of Clinical Laboratory Center annually (Ministry of Health of the People’s Republic of China, Beijing, China). The assay characteristics were as follows: sensitivity = 0.16 ng/ml, intra-assay CV = 3.2%, and inter-assay CV = 7.0%. AFC was defined as the sum of the number of follicles 2–10 mm in diameter in both ovaries. Assessment was performed using transvaginal sonography by a relatively fixed group of 3 experienced reproductive physicians before COS. These doctors underwent standardized training and adhered to uniform standards. Transvaginal ultrasonography (Voluson E8 Expert; GE Healthcare, Chicago, IL, USA) was performed using a 4- to 9-MHz probe. Homeostasis model assessment of insulin resistance (HOMA-IR) was calculated using the following formula: HOMA-IR = fasting blood glucose (mmol/L) × fasting insulin (μU/ml)/22.5 (7). Pregnancy outcome data were collected until September 2023. Live birth rate referred to a live birth per embryo transfer (ET) cycle. Patients who were more than 3 months pregnant but had not yet delivered were not included in the calculation. The cumulative pregnancy rate was defined as the total number of pregnancies achieved across all ET cycles in one retrieval cycle.
2.3 Ovarian stimulation protocol, embryo transfer, and luteal support
The COS protocol and Gn dose were customized based on the patient’s age, weight, and ovarian reserve using a step-up regimen for the Gn dose. The protocols included the early follicular-phase long-acting GnRH agonist long protocol, the mid-luteal-phase short-acting GnRH agonist long protocol, and the antagonist flexible protocol. In the short-acting GnRH agonist protocol, the patients were injected 0.1 mg triptorelin from day 6 to day 8 after ovulation for 14–18 days until achieving pituitary downregulation. Thereafter, Gn and 0.05 mg/day triptorelin were administered together until human chorionic gonadotropin (hCG) triggering was scheduled. Triptorelin acetate (triptorelin, 3.75 mg) was given during the menstrual period in the long-acting GnRH agonist regimen. When satisfactory pituitary downregulation was achieved after approximately 30–35 days, Gn was injected every day. In the GnRH antagonist flexible protocol, Gn was administrated from day 2 to day 3 of this menstrual cycle and a GnRH antagonist (cetrotide, 0.25 mg) injected daily from day 6 to day 7 of stimulation when one dominant follicle was ≥12 mm. When one dominant follicle was ≥20 mm in diameter or three follicles were ≥17 mm in diameter or more than 2/3 follicles were ≥16 mm in diameter, recombinant hCG (250 μg) or urinary hCG (2,000 U) was injected. Transvaginal ultrasound-guided aspiration was conducted 35–38 h following hCG injection to pick up oocytes. The Istanbul Consensus scoring system was applied for embryo evaluation (8). A transferable embryo referred to an embryo with ≥ 4 cells, < 26% fragmentation, and either no asymmetry or moderate asymmetry, which could be transferred or cryopreserved. ET was conducted on day 3 or day 5 after oocyte retrieval. The endometrial preparation protocol was selected individually in frozen ET cycles. Approximately 1–2 cleavage embryos or blastocysts would be transferred. Combined vaginal and oral progesterone was administered for luteal-phase support until 8–10 weeks.
2.4 Statistical analyses
Data analysis was conducted using EmpowerStats statistical software (X&Y Solutions). Continuous variables were reported as the mean ± standard deviation (SD), while categorical variables were expressed as number and percentages. Univariable logistic regression analyses were performed to identify the relevant factors for unexpected POR. The least absolute shrinkage and selection operator (LASSO) binary logistic regression analyses were implemented with the “glmnet” package in R for variable selection. In addition, a nomogram was created using the “rms” package to offer graphical representations of the selected factors in the model and to facilitate users in calculating probabilities (9). For validation, bootstrap and the Hosmer–Lemeshow test were carried out to estimate the goodness-of-fit of the model. Receiver operating characteristic (ROC) curves and the areas under the curves (AUC) were calculated to compare the prediction model and single related factors. Optimal cutoff values were determined using the Youden index.
3 Results
3.1 Analysis of patients’ baseline characteristics
This study included a total of 10,404 patients, 405 cases (3.89%) in the unexpected POR group and 9,999 (96.11%) cases in the NOR group. A total of 13 cycles were canceled after oocyte retrieval and 5,850 cycles underwent fresh ET. Table 1 outlines the baseline characteristics. Significant differences were observed in age, weight, BMI, basal follicle-stimulating hormone (bFSH), basal progesterone, AMH, AFC, fasting blood glucose, fasting insulin, HOMA-IR, protocol composition, Gn initial dose, Gn duration, and total Gn usage between the two groups (p < 0.05). No statistical significance was observed in the infertility type, infertility duration, polycystic ovary syndrome (PCOS) composition, basal luteinizing hormone (LH) level, estrogen (E2), prolactin (PRL), testosterone (T), and progesterone (P) (p > 0.05).
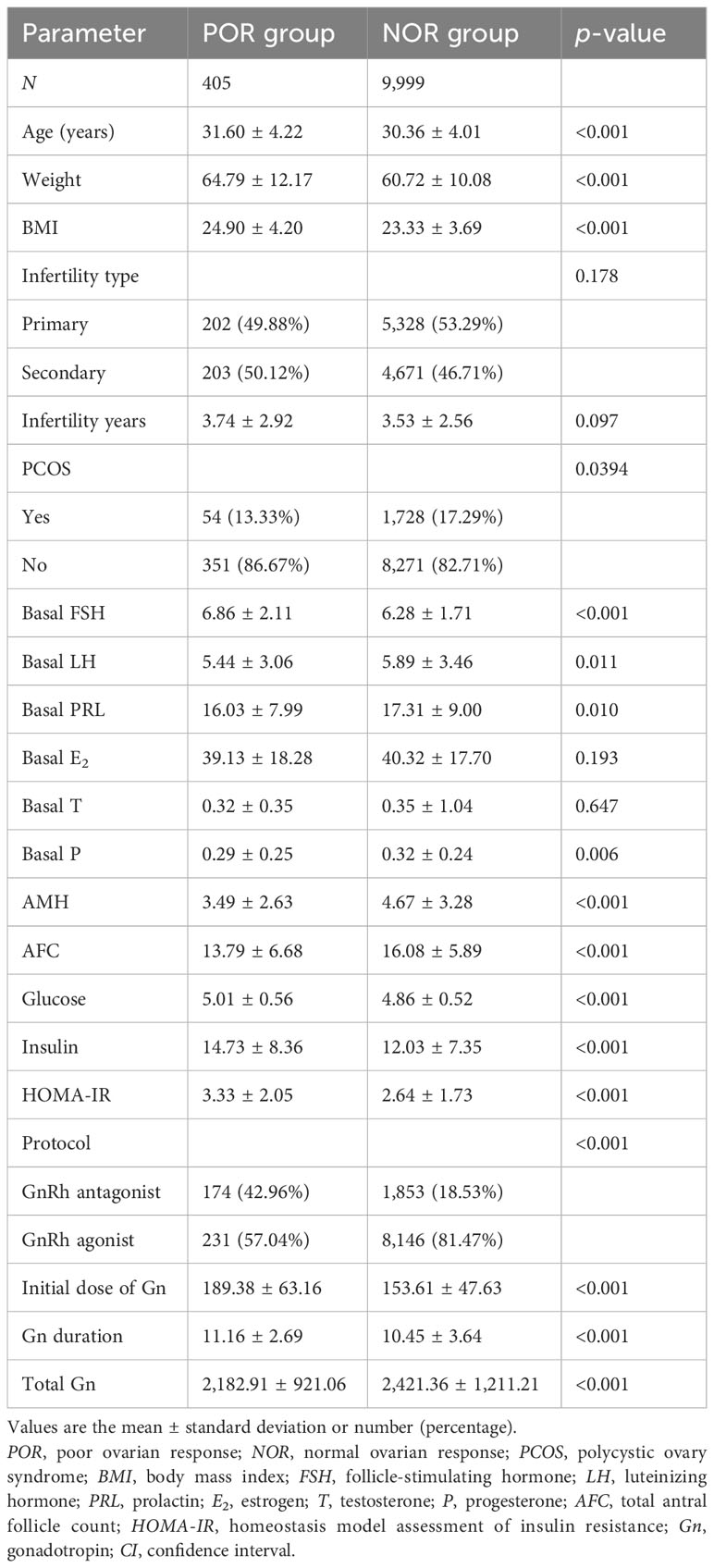
Table 1 Comparison of the basic parameters of the study population using univariable logistic regression analysis.
3.2 Analysis of patients’ laboratory index and clinical outcomes
The NOR group exhibited significantly higher numbers of dominant follicles on hCG trigger day, retrieved oocytes, MII oocytes, 2PN embryos, and transferable embryos and higher rates of fresh cycle pregnancy and cumulative pregnancy compared to the POR group (p < 0.05). There was no statistically significant difference in the ectopic rate, abortion rate, and live birth rate of fresh cycle between the two groups (p > 0.05) (Table 2).
3.3 Feature selection and parameter building
Aiming to screen associated factors for unexpected POR, we adopted a LASSO regression mechanism, which is an ideal method for performing interaction testing, variable selection, and parameter estimation without overfitting. A total of 21 potential related variables (listed in Table 1) were analyzed. Of these, seven variables were ultimately suggested as predictors (namely, BMI, bFSH, AMH, AFC, HOMA-IR, protocol, and initial dose of Gn) for unanticipated POR using the LASSO regression model, as shown in Figures 2A, B.
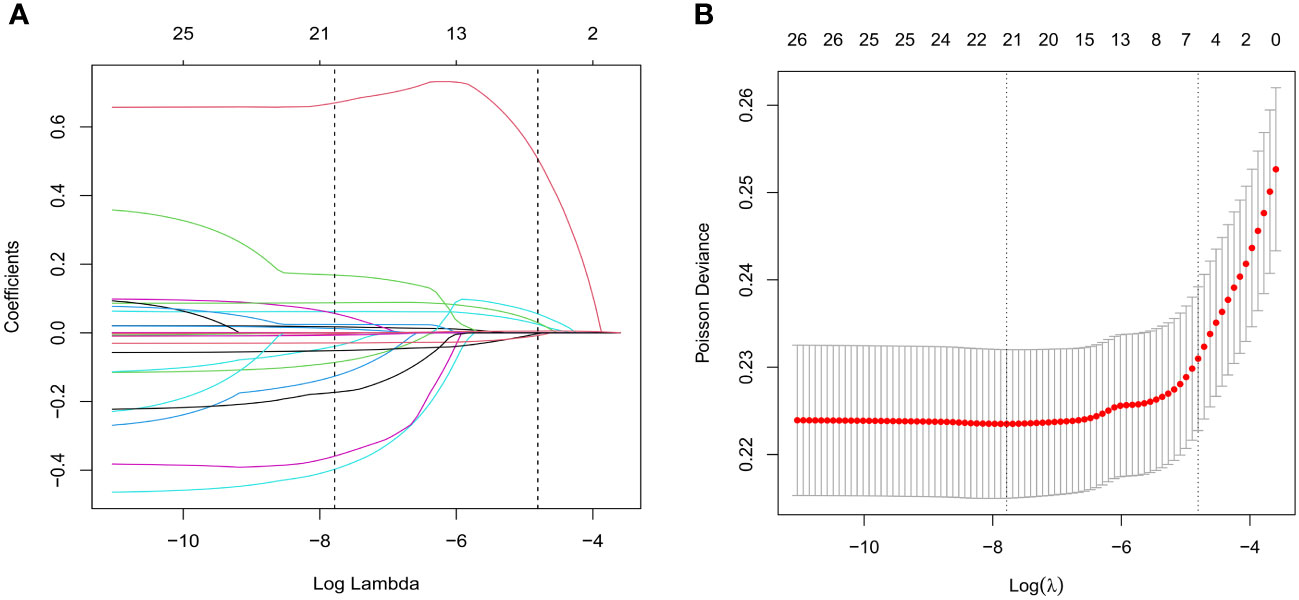
Figure 2 Variable selection using the least absolute shrinkage and selection operator (LASSO) regression algorithm. (A) Lasso regression path diagram. (B) LASSO coefficient profiles of the characteristics. Parameters were screened out by 10-fold cross-validation and using lambda.1se as the criteria.
3.4 Development of an individualized prediction model
The developed model for estimating the POR used the selected variables, i.e., BMI, bFSH, AMH, AFC, HOMA-IR, protocol, and initial dose of Gn, as indicators. The nomogram for prediction is depicted in Figure 3. Each parameter was assigned a vertical extension (shown in the top points bar) individually. The total score was determined by summing up the scales for each factor. The overall point projected on the bottom scale suggests the likelihood of poor response. The equation for the nomogram is as follows: logit(unexpected POR) = −4.54532 + 0.08169 × BMI + 0.11129 × bFSH − 0.06736 × AMH − 0.04022 × AFC + 0.13237 × HOMA-IR − 0.91707 × protocol + 0.00406 × initial dose of Gn.
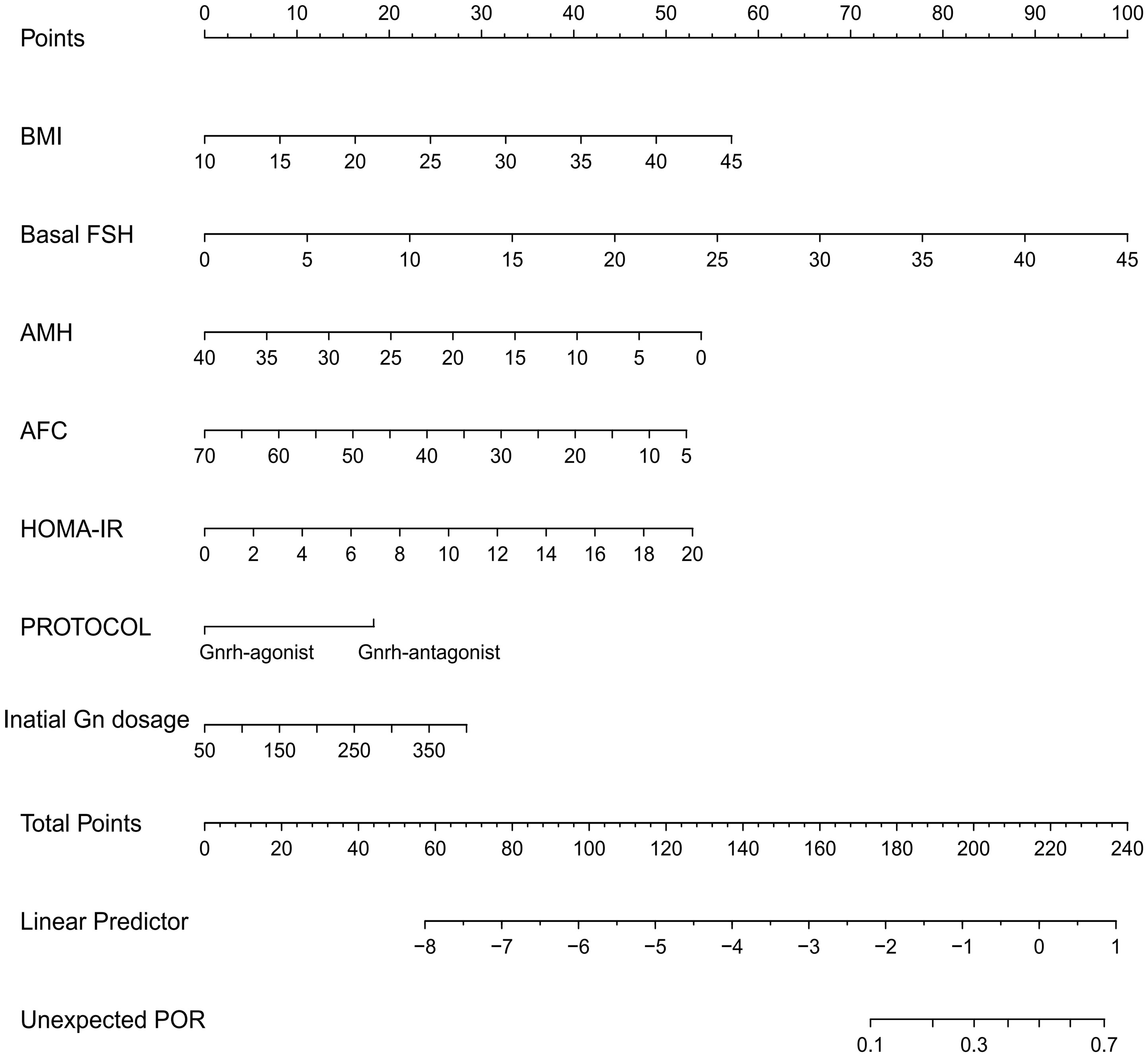
Figure 3 Nomogram for predicting unanticipated poor ovarian response (POR). The point of each variable was obtained at the intersection of the vertical line drawn from each variable to the point ruler. The total score of all factors was calculated and a descending line drawn from the “Total Points” until it intersected with the lower line to acquire the probability of POR.
3.5 Validation of the nomogram
Analyses of the ROC curves were performed to investigate the forecast value of the prediction model. The results of AUC analyses indicated that our model demonstrated good discriminative potential, with an AUC of 0.753 (95%CI = 0.730–0.77.7). The AUC confidence interval and significance test were obtained using the bootstrap method (bootstrap resampling times = 500) (Figures 4A, B). The Hosmer–Lemeshow test exhibited a non-significant difference (p = 0.167), demonstrating the satisfactory level of effectiveness and reliability of this model. Furthermore, the model was also validated in non-PCOS patients and exhibited fairly good discriminative ability, with an AUC of 0.758 (95%CI = 0.732–0.783). The Hosmer–Lemeshow test showed a non-significant difference (p = 0.112) (Figure 4C). In addition, we compared the predictive potential of the single variables and the predictive model using the ROC curves. The AUC of the nomogram was significantly higher than that of the single variables BMI, bFSH, AMH, AFC, HOMA-IR, and initial dose of Gn (Figure 4D, Table 3).
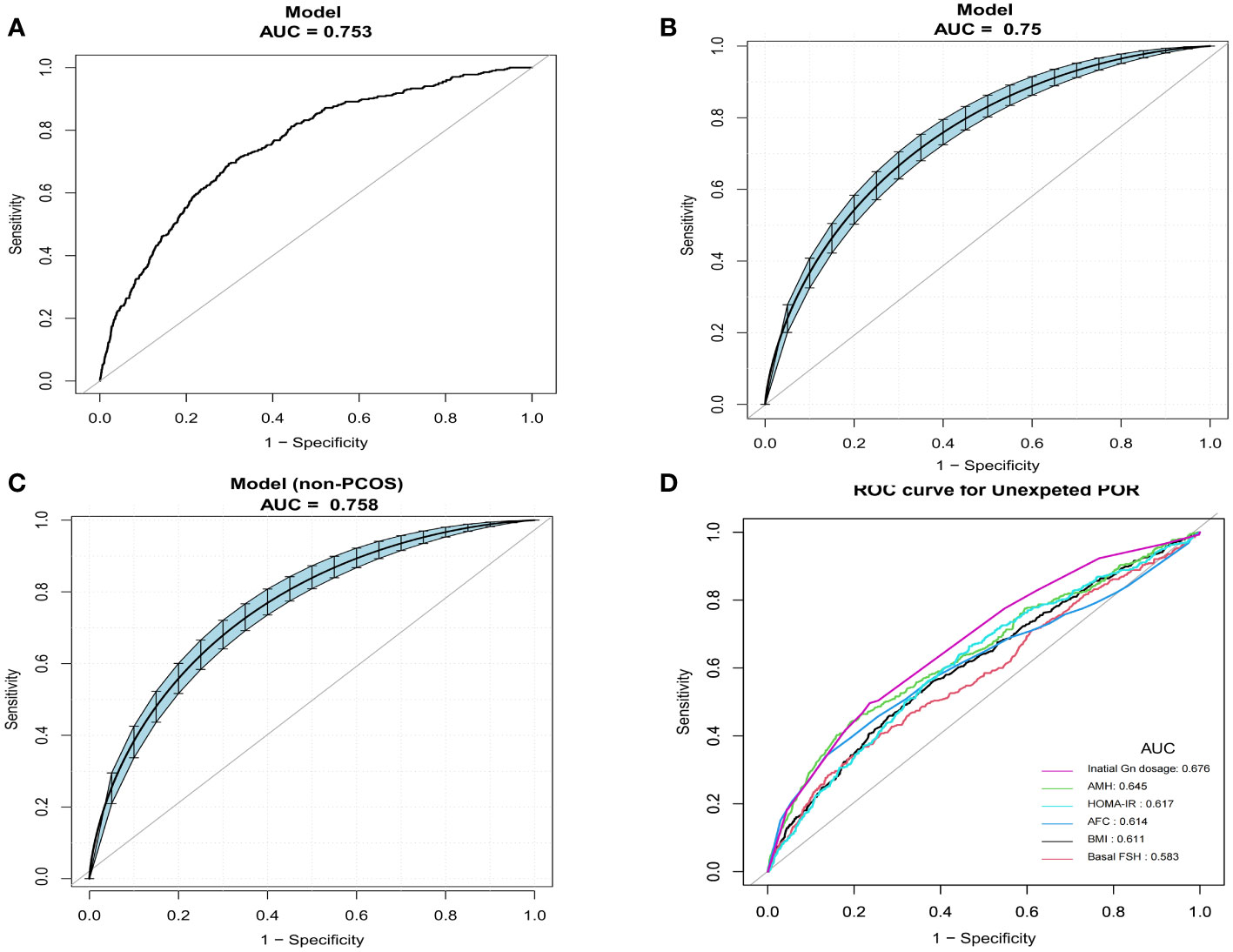
Figure 4 Comparison of the predictive potential of different variables for poor ovarian response (POR) using receiver operating characteristic (ROC) curves. (A) Predictive ability of the nomogram models for unexpected POR. (B) Confidence interval of the area under the curve (AUC) and significance tests using bootstrap resampling (bootstrap resampling times = 500). (C) Confidence interval of the AUCs in non-polycystic ovary syndrome (PCOS) patients (bootstrap resampling times = 500). (D) Predictive ability of individual variables.
4 Discussion
Precise prediction of ovarian response is essential for successful ovarian stimulation. The association of the ovarian reserve biomarkers including age, AFC, and AMH with ovarian response has been clarified (10). However, a subset of patients with a seemingly normal ovarian reserve may manifest as “unexpected poor responders” during COS. Currently, there is no consensus on how to identify these patients before oocyte retrieval and no acknowledged mathematical model for prediction. Early detection of infertile women with declined ovarian sensitivity is important as it could impact the treatment decision during ovulation induction. Therefore, our study developed and validated a predictive model for unexpected POR. To our knowledge, this is the first work on the prediction of POR in infertile patients with normal ovarian reserve.
Consistent with multiple studies, our research revealed that the ovarian reserve markers (i.e., bFSH, AMH, and AFC) can serve as predictive factors to identify individuals at risk of unanticipated POR. A large sample real-world analysis reported that AFC and AMH showed remarkable precision in predicting POR. Thresholds of AFC ≤ 5 and AMH ≤ 1.18 ng/ml were suggested. Age was also suggested for consideration (11). Xu et al. established a mathematical model with AMH, AFC, and bFSH as predictors to estimate the probability of POR. The AUCs in the inner/outer validation data were 0.861/0.850 (12). Despite AFC and AMH being ovarian reserve markers with high sensitivity, their reliability is not absolute as they could yield false-positive results at rates ranging from 10% to 20% (9). In this study, the AUC of the developed model was 0.7533, which is noticeably higher than those of the single predictors including the ovarian reserve marker, BMI, HOMA-IR, and initial dose of Gn. This finding indicates that the combination of different predictors can improve the predictive accuracy.
Our study also suggested that metabolic indicators are associated with POR. We therefore incorporated HOMA-IR and BMI into the predictive model. This is the primary innovation of our research and the main distinction from previous findings, given that patients with PCOS have a higher risk of insulin resistance and are overweight. The predictive model was also validated in non-PCOS patients and showed similar predictive performance. HOMA-IR, a commonly used metric to assess insulin sensitivity, exhibits high sensitivity and specificity in measuring insulin resistance (13). Several studies have reported that insulin resistance may directly impact oocyte maturation and ovulation in patients with PCOS (14, 15). However, only a few studies have focused on the relationship between HOMA-IR and POR in patients without PCOS. Li et al. found a negative relationship between HOMA-IR and ovarian response in infertile patients with good ovarian reserve (16). The mechanisms underlying the declining ovarian sensitivity during COS are not fully understood. Insulin might play a crucial role in ovarian function and could be involved in promoting follicle development (17). In addition, previous research revealed that being overweight may suppress the ovarian response (18) and decrease the number of retrieved oocytes (19, 20). Increased insulin levels in obese sufferers will promote the generation of androgen in the ovary, leading to increased estrogen and creating a negative impact on the hypothalamic–pituitary–ovarian axis. Consequently, this can inhibit follicle growth by suppressing the formation of FSH and prolonging the duration of ovarian stimulation (21, 22). Fortunately, insulin resistance and body weight are reversible parameters. Due to the higher risk of POR, insulin and weight management should be encouraged to potentially improve the outcomes of assisted reproductive techniques (ART).
An appropriate COS protocol and an adequate starting dose of Gn are important to avoid unforeseen POR (23). Consistent with other research, it was revealed that the GnRH antagonist protocol and the initial dose of Gn are critical factors in estimating the chances of unexpected POR. A randomized clinical trial (RCT) uncovered a decrease in the average quantity of retrieved oocytes in the GnRH antagonist protocol (24). Wang et al. reported that the initial dose of Gn, FSH, and the LH level on the initial day of Gn administration were variables independently contributing to fewer oocytes retrieved than expected (25). A cohort study revealed that the COS protocol, average Gn dose, previous instances of POR, and a history of ovarian surgery were independent factors influencing the occurrence of POR (26). Some scholars have suggested that insufficient Gn is the main factor for suboptimal ovarian response in a long-agonist regimen (27). However, definitive criteria for selecting the optimal Gn starting dose have yet to be completely identified (9). Some researchers are developing indices to calculate the suitable initial exogenous dose of Gn. For instance, a nomogram was created by La Marca et al. to indicate the ideal initial FSH dose according to the age, AMH, and FSH levels of patients (28).
This study has some limitations that need to be highlighted. First, the inherent biases associated with retrospective analysis may impact the results. To mitigate selection bias, we set relatively broad criteria for ovarian reserve, BMI, and the COS protocol. We also excluded individuals with factors including untreated metabolic or endocrine abnormalities, pathological ovarian cysts, or history of ovarian surgery that could potentially influence the ovarian response. These inclusion and exclusion criteria were employed to ensure population homogeneity and to enhance practical clinical applicability. Moreover, we conducted model validation in non-PCOS patients to address the potential confounding of PCOS. Furthermore, limited by the data collection, information regarding weight loss during ovulation induction, the metformin dose in patients with insulin resistance, and posttreatment HOMA-IR levels remains unclear. However, our center adopted a standardized treatment for patients with insulin resistance and obesity without any bias across different populations. Therefore, further exploration is warranted to investigate relevant indicators and prediction models.
5 Conclusion
In summary, in this study, a predictive model was developed incorporating BMI, bFSH, AMH, AFC, HOMA-IR, protocol, and the initial dose of Gn as indicators to estimate the probability of POR in patients with good ovarian reserve. We anticipate that our model would be significant in evaluating unexpected POR occurrences and in providing assistance to clinicians in making clinical decisions.
Data availability statement
The original contributions presented in the study are publicly available. Further inquiries can be directed to the corresponding author.
Ethics statement
The studies involving humans were approved by Henan Provincial People’s Hospital Ethics Committee. The studies were conducted in accordance with the local legislation and institutional requirements. The ethics committee/institutional review board waived the requirement of written informed consent for participation from the participants or the participants’ legal guardians/next of kin because the retrospective nature of the research.
Author contributions
XX: Writing – original draft, Conceptualization, Methodology, Writing – review & editing. XW: Conceptualization, Formal analysis, Methodology, Writing – original draft. YJ: Formal analysis, Software. HS: Formal analysis, Software. YC: Data curation. CZ: Funding acquisition, Project administration, Supervision, Writing – review & editing.
Funding
The author(s) declare financial support was received for the research, authorship, and/or publication of this article. This research was supported by National Natural Science Foundation of China-Henan Joint Fund (U2004130).
Acknowledgments
The authors express their sincere appreciation to the patients participated in this study and their colleagues for their kind assistance.
Conflict of interest
The authors declare that the research was conducted in the absence of any commercial or financial relationships that could be construed as a potential conflict of interest.
Publisher’s note
All claims expressed in this article are solely those of the authors and do not necessarily represent those of their affiliated organizations, or those of the publisher, the editors and the reviewers. Any product that may be evaluated in this article, or claim that may be made by its manufacturer, is not guaranteed or endorsed by the publisher.
References
1. Abu-Musa A, Haahr T, Humaidan P. Novel physiology and definition of poor ovarian response; clinical recommendations. Int J Mol Sci. (2020) 21:2110. doi: 10.3390/ijms21062110
2. Drakopoulos P, Bardhi E, Boudry L, Vaiarelli A, Blockeel C. Update on the management of poor ovarian response in IVF: the shift from Bologna criteria to the Poseidon concept. Ther Adv Reprod Health. (2020) 14:263349412094148. doi: 10.1177/2633494120941480
3. Marca AL, Grisendi V, Giulini S, Sighinolfi G, Tirelli A, Argento C, et al. Live birth rates in the different combinations of the Bologna criteria poor ovarian responders: a validation study. J Assisted Reproduction&Genetics. (2015) 32. doi: 10.1007/s10815-015-0476-4
4. Ferraretti AP, La Marca A, Fauser BC, Tarlatzis B, Nargund G, Gianaroli L. ESHRE consensus on the definition of ‘poor response’ to ovarian stimulation for in vitro fertilization: the Bologna criteria. Hum Reproduction. (2011) 26:1616–24. doi: 10.1093/humrep/der092
5. Alviggi C, Andersen CY, Buehler K, Conforti A, De Placido G, Esteves SC, et al. A new more detailed stratification of low responders to ovarian stimulation: from a poor ovarian response to a low prognosis concept. Fertility Sterility. (2016) 105(6):1452–3. doi: 10.1016/j.fertnstert.2016.02.005
6. Christensen MW, Kesmodel US, Christensen K, Kirkegaard K, Ingerslev HJ. Early ovarian ageing: is a low number of oocytes harvested in young women associated with an earlier and increased risk of age-related diseases? Hum Reprod. (2020) 35(10):2375–90. doi: 10.1093/humrep/deaa188
7. Matthews DR, Hosker JP, Rudenski AS, Naylor BA, Treacher DF, Turner RC. Homeostasis model assessment: insulin resistance and beta-cell function from fasting plasma glucose and insulin concentrations in man. Diabetologia. (1985) 28:412. doi: 10.1007/BF00280883
8. Alpha Scientists In Reproductive Medicine And Eshre Special Interest Group Embryology. The Istanbul consensus workshop on embryo assessment: proceedings of an expert meeting. Hum Reprod. (2011) 22:632–46. doi: 10.1016/j.rbmo.2011.02.001
9. Zlotnik A, Abraira V. A general-purpose nomogram generator for predictive logistic regression models. stata J. (2015) (2):537–46. doi: 10.1177/1536867X1501500212
10. Antonio LM, Kamal SS. Individualization of controlled ovarian stimulation in IVF using ovarian reserve markers: from theory to practice. Hum Reprod Update. (2013) 1(1):124–40. doi: 10.1093/humupd/dmt037
11. Wang X, Jin L, Mao YD, Shi JZ, Huang R, Jiang YN, et al. Evaluation of ovarian reserve tests and age in the prediction of poor ovarian response to controlled ovarian stimulation—A real-world data analysis of 89,002 patients. Front Endocrinology. (2021) 12:1044–. doi: 10.3389/fendo.2021.702061
12. Xu H, Wang H, Yang R, Song Y, Han Y. A novel mathematical model of true ovarian reserve assessment based on predicted probability of poor ovarian response: a retrospective cohort study. J Assisted Reprod Genet. (2020) 37:1–10. doi: 10.1007/s10815-020-01700-1
13. Keskin M, Kurtoglu S, Kendirci M, Atabek ME, Yazici C. Homeostasis model assessment is more reliable than the fasting glucose/insulin ratio and quantitative insulin sensitivity check index for assessing insulin resistance among obese children and adolescents. Pediatrics. (2005) 115:e500. doi: 10.1542/peds.2004-1921
14. Hassani F, Oryan S, Yazdi PE, Ghaheri A. Association between the number of retrieved mature oocytes and insulin resistance or sensitivity in infertile women with polycystic ovary syndrome. Int J fertility sterility. (2019) 12(4):310–5. doi: 10.22074/ijfs.2019.5422
15. Vandermolen DT, Ratts VS, Evans WS, Stovall DW, Kauma SW, Nestler JE. Metformin increases the ovulatory rate and pregnancy rate from clomiphene citrate in patients with polycystic ovary syndrome who are resistant to clomiphene citrate alone. Fertility Sterility. (2001) 75:310–5. doi: 10.1016/S0015-0282(00)01675-7
16. Li Y, Jiang Y, Zhang S, Liu H, Zhang C. Association of HOMA-IR with ovarian sensitivity index in women undergoing IVF/ICSI: A retrospective cohort study. Diabetes Metab Syndr Obes. (2023) 16:309–20. doi: 10.2147/DMSO.S399105
17. Ms C KP, Mh D, Ry Y, Pj M, Rj C. Enhanced granulosa cell responsiveness to follicle-stimulating hormone during insulin infusion in women with polycystic ovary syndrome treated with pioglitazone. J Clin Endocrinol Metab. (2003) 88(12):5624–31. doi: 10.1210/jc.2003-030745
18. Li Yu, Yang D, Zhang Q. Impact of overweight and underweight on IVF treatment in Chinese women. Gynecological Endocrinol. (2010) 26:416–22. doi: 10.3109/09513591003632118
19. Van Swieten ECAM, Loes LH, Badings EA, van der L Paul JQ. Obesity and Clomiphene Challenge Test as predictors of outcome of in vitro fertilization and intracytoplasmic sperm injection. Gynecologic Obstetric Invest. (2005) 59:220–4. doi: 10.1159/000084347
20. Spandorfer SD, Kump L, Goldschlag D, Brodkin T, Rosenwaks Z. Obesity and in vitro fertilization: Negative influences on outcome. J Reprod Med. (2005) 49:973–7. doi: 10.1111/j.1447-0756.2004.00230.x
21. Broughton DE, Moley KH. Obesity and female infertility: potential mediators of obesity’s impact. Fertil Steril. (2017) 107:840–7. doi: 10.1016/j.fertnstert.2017.01.017
22. Pasquali R, Pelusi C, Genghini S, Cacciari M, Gambineri A. Obesity and reproductive disorders in women. Hum Reprod Update. (2003) 9:359–72. doi: 10.1093/humupd/dmg024
23. van Tilborg TC, Oudshoorn SC, Eijkemans MJC, Mochtar MH, van Golde RJT, Hoek A, et al. Individualized FSH dosing based on ovarian reserve testing in women starting IVF/ICSI: a multicentre trial and cost-effectiveness analysis. Hum Reprod. (2017) 32:2485–95. doi: 10.1093/humrep/dex321
24. Tehraninejad E, Ghahghaei Nezamabadi A, Rashidi B, Sohrabi M, Bagheri M, Haghollahi F, et al. GnRH antagonist versus agonist in normoresponders undergoing ICSI: a randomized clinical trial in Iran. Iran J Reprod Med. (2011) 9:171–6.
25. Wang B, Liu W, Liu Y, Zhang W, Ren C, Guan Y. What does unexpected suboptimal response during ovarian stimulation suggest, an overlooked group? Front Endocrinol (Lausanne). (2021) 12:795254. doi: 10.3389/fendo.2021.795254
26. Wang X, Fan YY, Li L, Zhang SD, Zhang CL. [Analysis of related factors and prediction of poor ovarian response in patients with controlled ovarian stimulation]. Zhonghua Fu Chan Ke Za Zhi. (2022) 57:110–6. doi: 10.3760/cma.j.cn112141-20210930-00561
27. Panagiotis D, Samuel SR, Ernesto B, Juan GV, Christophe B, Alessia R, et al. The effect of dose adjustments in a subsequent cycle of women with suboptimal response following conventional ovarian stimulation. Front Endocrinology. (2018) 9:361–. doi: 10.3389/fendo.2018.00361
28. La Marca A, Papaleo E, Grisendi V, Argento C, Giulini S, Volpe A. Development of a nomogram based on markers of ovarian reserve for the individualisation of the follicle-stimulating hormone starting dose in in vitro fertilisation cycles. BJOG. (2012) 119:1171–9. doi: 10.1111/j.1471-0528.2012.03412.x
Keywords: predictive model, nomogram, poor ovarian response, ovarian reserve, IVF/ICSI
Citation: Xu X, Wang X, Jiang Y, Sun H, Chen Y and Zhang C (2024) Development and validation of a prediction model for unexpected poor ovarian response during IVF/ICSI. Front. Endocrinol. 15:1340329. doi: 10.3389/fendo.2024.1340329
Received: 17 November 2023; Accepted: 31 January 2024;
Published: 04 March 2024.
Edited by:
Tom Kelsey, University of St Andrews, United KingdomReviewed by:
Akmal El-Mazny, Cairo University, EgyptXiaoling Ma, First Hospital of Lanzhou University, China
Copyright © 2024 Xu, Wang, Jiang, Sun, Chen and Zhang. This is an open-access article distributed under the terms of the Creative Commons Attribution License (CC BY). The use, distribution or reproduction in other forums is permitted, provided the original author(s) and the copyright owner(s) are credited and that the original publication in this journal is cited, in accordance with accepted academic practice. No use, distribution or reproduction is permitted which does not comply with these terms.
*Correspondence: Cuilian Zhang, bHVja3l6Y2xAcXEuY29t