- 1Unit of Pediatric Endocrinology and Diabetology, Pediatric Service, Woman-Mother-Child Department, Lausanne University Hospital, Lausanne, Switzerland
- 2Obstetric Service, Woman-Mother-Child Department, Lausanne University Hospital, Lausanne, Switzerland
- 3Nepean Clinical School, Faculty of Medicine and Health, The University of Sydney, Penrith, NSW, Australia
- 4Institute of Higher Education and Research in Healthcare (IUFRS), University of Lausanne, Lausanne, Switzerland
- 5Neonatology Service, Woman-Mother-Child Department, Lausanne University Hospital, Lausanne, Switzerland
Background: Gestational Diabetes Mellitus (GDM) is frequently associated with chronic, low-grade inflammation. Whether this environment affects offspring anthropometry during early childhood remains to be elucidated. The aim of this study was to investigate the associations between maternal and fetal (cord blood-umbilical artery) inflammatory biomarkers and offspring weight and BMI up to 1 year in pregnancies with GDM.
Methods: In this prospective secondary analysis of the MySweetheart study, we included 193 women with GDM and their offspring. Maternal and fetal (N=39) predictors included serum levels of inflammatory biomarkers including CRP, IL-6, and TNF-α at 24-32 weeks of gestational age (GA) and in the cord blood. Offspring outcomes were small and large for gestational age (SGA, LGA), sex- and age-adjusted weight, and BMI at birth and at 1 year. Univariate and multivariate regression models were performed. Associations were adjusted for maternal pre-pregnancy BMI, age, and ethnicity.
Results: Mean maternal age was 33.6 ± 4.8 years, and pre-pregnancy BMI 25.9 ± 5.6 kg/m2. Their mean gestational age at the 1st GDM visit was 29 ± 2.4 weeks. Gestational age at delivery was 39.7 ± 1.1 weeks, with a mean birthweight of 3.4 ± 0.46 kg; 11.8% of offspring were LGA and 10.8% were SGA. At 1 year of age, mean offspring weight was 9.8 ± 1.2 kg and BMI z-score 0.23 ± 1.1 kg/m2. In the models including only maternal predictors, TNF-α at 24-32 weeks of GA was positively associated with SGA and inversely with offspring weight and BMI at birth and at 1 year (p ≤0.034). In the models including only fetal predictors and the combined model, CRP was inversely associated with BMI at 1 year (p ≤0.020).
Conclusions: In women with GDM, maternal and fetal inflammatory biomarkers distinctively influenced offspring anthropometry during the first year of life, independent of maternal age, prepregnancy BMI and ethnicity. These results suggest that low-grade inflammation during pregnancy may affect the developing offspring by leading to a decrease in weight and BMI and may have implications for future personalized follow-up of women with GDM and their offspring.
1 Introduction
Inflammation is associated with an increased risk of insulin resistance, hyperglycemia, metabolic syndrome, and cardiovascular disease (1–3). Increased inflammation during pregnancy caries an increased risk for short-term complications in the mother and the offspring, including miscarriage, gestational diabetes mellitus (GDM), preeclampsia, preterm birth, intrauterine growth restriction, and birth defects (4–8). Long-term complications in the offspring such as specific behavioral complications, and psychiatric disorders have also been recently evoked (9). Maternal GDM may be associated with a state of chronic, low-grade inflammation, which often precedes its diagnosis (10). The complex relationship between gestational diabetes and inflammation is underscored by evidence of increased plasma levels of pro-inflammatory cytokines, including plasma C-reactive protein (CRP), interleukin-6 (IL-6), tumor necrosis factor alpha (TNF-α) and/or IL-1a, in women with GDM and their fetuses (umbilical cord) in some, but not all studies (11–16).
To the best of our knowledge, the association between maternal inflammatory biomarkers during pregnancy and offspring weight or BMI during the 1st year of life in pregnancies with GDM has not been previously investigated. Most of the studies in the general population as well as a study including a large population with a wide spectrum of glucose tolerance (Hyperglycemia and Adverse Pregnancy Outcome (HAPO) study), found an inverse association between pro-inflammatory biomarkers during pregnancy and weight and adiposity at birth (17–21). In the general pregnant population, this association was not significant (22). Beyond birth, 2nd trimester plasma CRP in healthy pregnancies has been associated with a higher childhood fat mass index (FMI) and trunk FMI in preschoolers (23). Regarding fetal predictors, existing studies in different populations (the general pregnant population, and populations with high prevalence of GDM), found no association between cord blood inflammatory biomarkers, such as CRP, IL-6 and TNF-α, and offspring weight and adiposity at birth (18, 24–26). However, the association between both maternal or fetal (cord blood) inflammatory biomarkers, including CRP, IL-6, and TNF-α has not been studied in populations with GDM (18, 21). There is also a lack of data on the impact of inflammatory biomarkers during the first year of life beyond birth in this population. Obesity and aging are associated with low-grade inflammation (27–29). Inflammatory biomarkers vary across different ethnic groups,underscoring the intricate interplay of genetic, environmental and social factors in shaping health disparities (30) A recent study in the general population found that maternal obesity-related inflammation during pregnancy increased the risk of childhood obesity in an ethnic-specific manner (31). However, it is unclear if this low-grade inflammation environment in pregnancies with GDM influences offspring anthropometry and contributes to the development of metabolic health disorders in offspring during early childhood as well as later in life.
The aims of this study were: 1) to investigate the associations between maternal and fetal inflammatory biomarkers on offspring anthropometric parameters at birth and at 1 year in a population of women with GDM; 2) to determine if the proposed associations are independent of maternal pre-pregnancy BMI, age, and ethnicity.
2 Materials and methods
2.1 Study design and informed consent
The present study is a secondary data analysis of the MySweetheart trial, a randomized-controlled intervention trial of 211 women ≥18 years with GDM and their offspring (Clinicaltrials.gov NCT02890693) (32), followed during pregnancy until one year postpartum in the Diabetes and Pregnancy Unit in the Lausanne University Hospital, Switzerland. GDM was diagnosed between 24 and 32 weeks of gestational age (GA), according to the International Association of Diabetes and Pregnancy Study Group (IADPSG Criteria) (33). Details of the study protocol have been previously described (32). Women on strict bed rest, with a severe mental health disorder, pre-existing diabetes, and women who did not understand English or French were excluded from the study. Signed informed consent was obtained from all participating women. The study was conducted in accordance with the guidelines of the declaration of Helsinki and good clinical practice. The Human Research Ethics Committee of the Canton de Vaud approved the study protocol (study number 2016-00745). Included women were randomized to the usual care or intervention group after the baseline visit and signing of an informed consent.
2.2 Allocation groups
2.2.1 Usual care group
Women randomized to usual care received treatment based on the American Diabetes Association and on the Endocrine Society guidelines for the management of GDM (34, 35). They had regular appointments every 1-3 weeks with a physician or a diabetes-specialist nurse and a dietician after the GDM diagnosis, and were encouraged to increase physical activity (34). During the 1st visit at 24-32 weeks of GA, they were taught how to perform self-monitoring of blood glucose (4 times during the day-fasting and 2 hours post-prandial). If glucose values remained above targets two or more times during a 1 to 2-week period (fasting glucose >5.3 mmol/l, 1-h postprandial glucose >8 mmol/l and/or 2-h postprandial glucose >7 mmol/l) despite lifestyle changes, insulin treatment or very rarely metformin was introduced depending on patient’s glucose values and preferences. After delivery, glucose controls and glucose-lowering treatments were stopped. Women saw a physician and a dietician at the 6-8 week postpartum visit after an oGTT test to discuss further management.
2.2.2 Intervention group
Women randomized to the intervention group received a multidimensional, interdisciplinary lifestyle and psychosocial intervention on top of usual care, centered on eating behavior, a balanced food intake, as well as physical activity and breastfeeding. The intervention also included a psychosocial component, including the assessment and treatment of depression during and after pregnancy. During pregnancy and up to 1 year postpartum, patients were supported by a lifestyle coach [see (32] for more details).
2.3 Follow-up
For the current analysis, we used maternal data from their 1st GDM visit at 24-32 weeks of GA, and offspring data at birth, and at 1 year. At the 1st GDM visit, information on maternal socio-demographic characteristics were collected and maternal anthropometric parameters and inflammatory biomarkers were measured in the serum. Immediately after birth, blood was drawn from the umbilical cord to measure inflammatory biomarkers. Newborn anthropometric parameters were obtained from the hospital birth record. At 1 year postpartum, the offspring’s anthropometric measures including weight and length were collected.
2.4 Maternal, fetal and offspring parameters
2.4.1 Maternal descriptive and confounder variables
Maternal socio-demographic parameters, including age, ethnicity, and parity were collected during the 1st GDM visit. Ethnicity was classified into Low (Europe, North America) and High Metabolic Risk (Asia, Central and South America, Africa, Oceania) ethnic groups (35). Pre-pregnancy BMI was calculated using weight information from medical charts and height measured during the 1st visit at the GDM clinic. In rare circumstances when pre-pregnancy weight was not mentioned in the chart, it was self-reported during the 1st GDM visit. Height was measured at the 1st GDM visit to the nearest 0.1 cm with a regularly calibrated Seca® height scale. GWG was determined as the difference between the weight at the end of pregnancy and pre-pregnancy weight. Glucose-lowering maternal medical treatment for GDM was classified into two categories (no treatment, treatment with insulin and/or very rarely metformin). HbA1c using a chemical photometric method (conjugation with boronate; Afinion®).
2.4.2 Maternal and fetal (cord blood) inflammatory predictor variables
At the 24-32 weeks of GA visit, maternal inflammatory parameters, including CRP, IL-6, and TNF-α were measured in maternal serum. At birth, CRP, IL-6, and TNF-α were again measured in the cord blood (umbilical artery) (36). CRP was analyzed at the Lausanne University Hospital in serum aliquots using a latex-enhanced immunoturbidimetric assay on a Cobas 8000 autoanalyzer (Roche Diagnostics, Mannheim, Germany) with assay characteristics as reported by the manufacturer. We also measured IL-6 (U-PLEX Human IL-6 Antibody Set) and TNF-α (U-PLEX Human TNF-α Antibody Set) using ELISA according to the manufacturer’s instructions.
2.4.3 Offspring descriptive and outcome variables
At birth, weight (g) and length (cm) were documented; percentiles and z-scores for these parameters were calculated using the Intergrowth 21st newborn size application tool (37). BMI was also calculated. LGA was defined as birth weight >90th percentile and SGA as birth weight <10th percentile for sex and gestational age. Gestational age was calculated according to the date of the last menstruation, or as assessed by the fetal ultrasound in the cases where gestational age was adapted during the early in-utero ultrasound evaluation. Neonatal antropometric parameters were obtained from patient medical charts. If the birth took place in another hospital or clinic, they were provided by the respective hospital.
At the 1 year visit, offspring weight (kg) and length (cm) were measured, using standardized methods (38). BMI was calculated. Z-scores for weight, length and BMI were calculated using the WHO Anthro Survey Analyser tool -Offline version (39).
2.4.4 Predictors and outcomes
Predictors comprised maternal (1st GDM visit) and fetal (cord blood), including CRP, IL-6, and TNF-α. Outcomes included offspring anthropometric parameters at birth and 1 year. More precisely, birth outcomes included weight, BMI, LGA, and SGA, and outcomes at 1 year, weight, and BMI.
2.5 Statistical analysis
Data analysis was performed using Stata/SE 16.0 (StataCorp LLC, TX, USA). The normality of continuous variables were assessed using histograms and Q-Q plots. Outcomes variables were normally distributed. Continuous variables were described as means and standard deviations and binary outcomes as N (percentages) (Table 1). Comparisons between the intervention and control group were conducted using the unpaired t-test for normally distributed continuous variables, the Mann-Whitney test for continuous variables with non-normal distribution and the Fisher’s exact test for binary variables. In all analyses, predictors and outcomes did not differ in the respective allocation groups (intervention vs usual care) and the effect sizes were similar. Thus, women from both groups were pooled together and we adjusted for group allocation in all analyses. Where appropriate, all analyses were also adjusted for infant age and sex.
We performed a Spearman’s rank correlation coefficient test to investigate the correlation between maternal and fetal cord inflammatory parameters and the presence of collinearity (Supplementary Table 1). No collinearity was found between predictors (rs < 0.6). We then conducted univariate linear and logistic regression analyses using offspring outcomes as the dependent variables (Supplementary Tables 2, 3). Maternal and fetal predictors with a p-value < 0.05 in univariate analysis were included in stepwise multiple regression analyses models. We performed different multivariate models. In terms of predictors, three multivariate models were used, a first model including only maternal predictors, a second model including only fetal predictors and a third model including both maternal and fetal predictors. Fetal predictors were available for N = 39 participants. In terms of adjustments, the above models were adjusted for maternal age, pre-pregnancy BMI, and ethnicity in addition to the already mentioned adjustments (group allocation, offspring age and sex). The analyses and adjustments were performed in order to identify the most significant maternal and fetal predictors of infant anthropometric parameters at birth and 1 year, and to determine the extent of the impact of these predictors independent of maternal confounder variables, i.e., ethnicity, age, and obesity; known to be associated with low-grade inflammation (27–29) (Table 2). For all analyses, β-coefficients (for continuous outcomes) and adjusted odds ratios (aORs-for binary outcomes) are reported along with their 95% confidence intervals (CIs), and statistical significance was set at p<0.05.
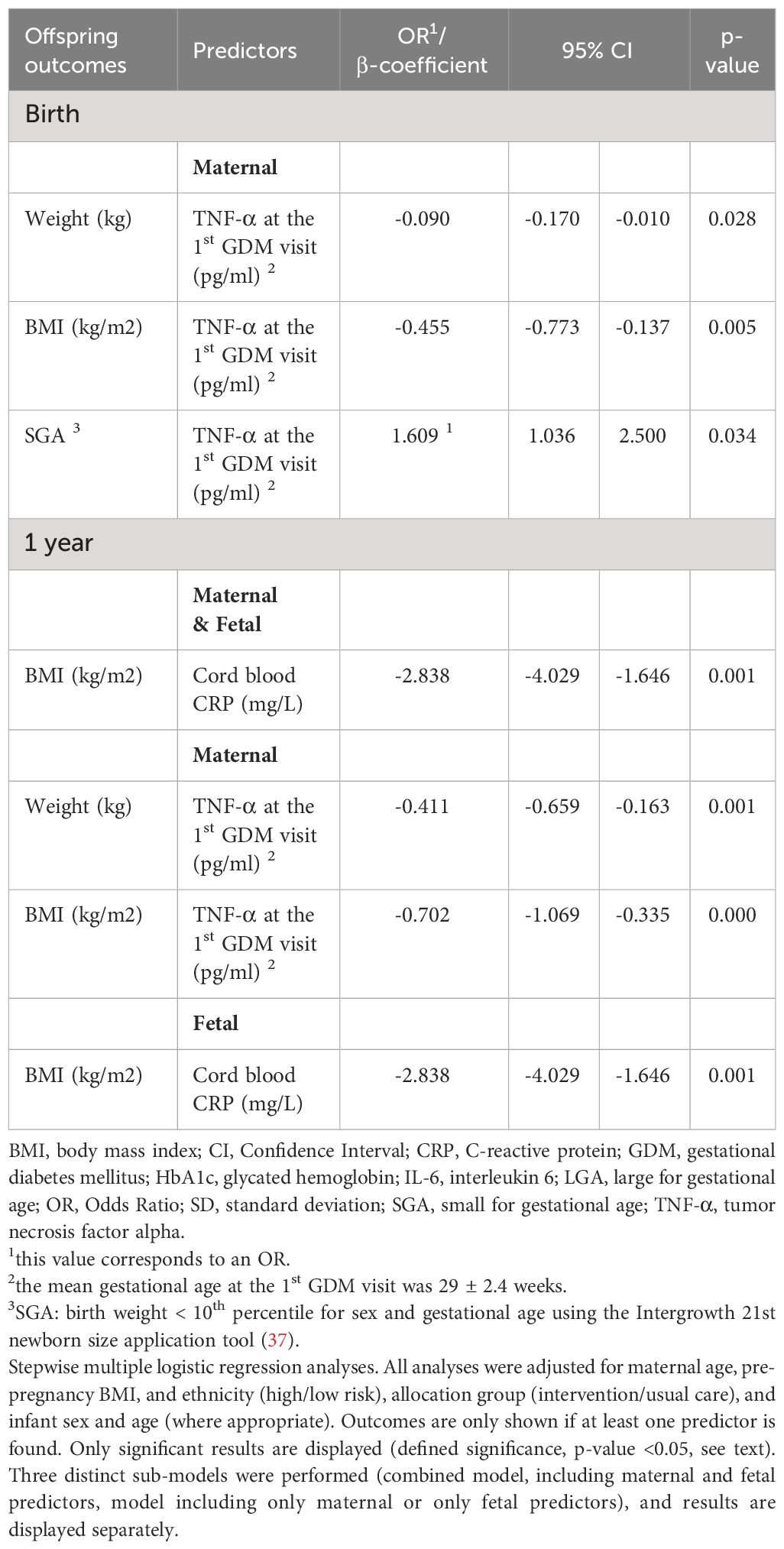
Table 2 Maternal serum and fetal cord blood predictors of offspring anthropometric outcomes in multivariate regression analyses.
3 Results
The initial population included 211 women with GDM. As previously described (38), one woman was excluded as the diagnosis of GDM was done too early (< 13 weeks of gestation), and 17 were excluded due to multiple gestation, and/or because their offspring were premature (gestational age (GA) < 37 weeks. Thus, 193 women and their offspring were included in the analyses.
3.1 Maternal, fetal and infant characteristics
Table 1 describes the maternal characteristics, fetal inflammatory parameters, and offspring anthropometry at birth and 1 year. In summary, mean maternal age was 33.6 ± 4.8 years, and pre-pregnancy BMI was 25.9 ± 5.6 kg/m2. Their mean gestational age at the 1st GDM visit at 24 to 32 weeks of GA was 29 ± 2.4 weeks. Gestational age at delivery was 39.7 ± 1.1 weeks, with a mean birthweight of 3.4 ± 0.46 kg; 11.8% of offspring were LGA and 10.8% were SGA. At 1 year of age, mean offspring weight was 9.8 ± 1.2 kg and BMI z-score 0.23 ± 1.1 kg/m2. Maternal and fetal (cord blood) inflammatory biomarkers, including CRP, IL-6, and TNF-α were not significantly correlated (Supplementary Table 1).
3.2 Associations between maternal and fetal predictors and offspring anthropometry at birth and 1 year in univariate analyses
3.2.1 Birth
TNF-α at the 1st GDM visit was inversely associated with offspring weight [β-coefficient= -0.107 (CI: -0.189; -0.026), p=0.010] and BMI at birth [β-coefficient= -0.501 (CI: -0.816; -0.186), p=0.002] and positively with SGA [OR= 0.492 (CI: 0.056; 0.927), p=0.027], and cord blood CRP was inversely associated with offspring weight [β-coefficient= -0.370 (CI: -0.659; -0.081), p=0.015] and BMI at birth [β-coefficient= -1.052 (CI: -1.900; -0.205), p=0.017]. No association was found between maternal and fetal inflammatory biomarkers and LGA (all p ≥0.199, Supplementary Table 2).
3.2.2 One year
TNF-α at the 1st GDM visit was inversely associated with offspring weight [β-coefficient= -0.376 (CI: -0.625; -0.128), p=0.003] and BMI at 1 year pβ-coefficient= -0.641 (CI: -1.003; -0.279), p=0.001], and cord blood CRP and TNF-α were inversely associated with offspring BMI at 1 year [β-coefficient= -2.566 (CI: -3.666; -1.465), p=0.000] and [β-coefficient= -2.177 (CI: -3.671; -0.684), p=0.006], respectively, Supplementary Table 3).
3.3 Associations between maternal and fetal predictors and offspring anthropometry at birth and 1 year in multivariate analyses
The significant results of all multivariate analyses are shown in Table 2.
3.3.1 Birth
In the models including only maternal predictors, TNF-α at the 1st GDM visit was inversely associated with offspring weight [β-coefficient= -0.090 (CI: -0.170; -0.010), p=0.028] and BMI [β-coefficient= -0.455 (CI: -0.773; -0.137), p=0.005], and positively with SGA [OR= 1.609 (CI: 1.036; 2.500), p=0.034]. No significant associations were found between maternal and fetal inflammatory biomarkers and offspring anthropometry in models including only fetal predictors or in the combined model (p ≥ 0.05).
3.3.2 One year
In models including maternal predictors, TNF-α at the 1st GDM visit was inversely associated with offspring weight [β-coefficient= -0.411 (CI: -0.659; -0.163), p=0.001] and BMI at 1 year [β-coefficient= -0.702 (CI: -1.069; -0.335), p=0.000]. In models including fetal predictors as well as in the combined model, cord blood CRP showed an inverse association with BMI at 1 year [β-coefficient= -2.838 (CI: -4.029; -1.646), p=0.001].
4 Discussion
This prospective, secondary analysis of the MySweethheart study, of women with GDM and their offspring showed that maternal and fetal inflammatory biomarkers predicted offspring weight and BMI during the 1st year of life, independent of maternal age, pre-pregnancy BMI, and ethnicity. In the adjusted analyses, maternal TNF-α at 24-32 weeks GA was positively associated with SGA and inversely with offspring weight and BMI at birth and at 1 year, whereas. CRP was inversely associated with offspring BMI at 1 year independent of maternal predictors. Thus, while maternal cytokines predicted lower weight and BMI, both at birth and up to 1 year, the impact of fetal CRP was observed at 1 year.
4.1 Impact of maternal and fetal inflammatory parameters on offspring anthropometry at birth
In our study, maternal serum concentrations of TNF-α at the 1st GDM visit at 24-32 weeks of GA were negatively associated with offspring weight and BMI at birth and positively with SGA. This is consistent with a recent study in a healthy population that found a negative correlation between inflammatory biomarkers such as CRP, and IL-6, and birthweight (17). Other studies have evaluated the impact of maternal inflammatory parameters and offspring birth outcomes in healthy pregnant women, as well as in populations with some degree of glucose intolerance (19, 20, 22, 40, 41). Thus, maternal CRP levels in early pregnancy were associated with higher rates of SGA in the general population (40), and those during the 3rd trimester were inversely associated with offspring weight and sum of skinfolds at birth in the general population and in a subpopulation of the HAPO Study (19, 20). No correlations between maternal 3rd trimester TNF-α, and IL-6 values and fetal adiposity or birthweight were found in a healthy pregnant population of women without GDM (22), whereas maternal 2nd and 3rd trimester IL-6 values were inversely associated with weight and sum of skinfolds at birth in a study including women with GDM and without GDM (41). A study performed in women with a previous history of having a macrosomic infant, but without GDM, found no association between maternal IL-6 and TNF-α and birthweight and adiposity measures in the total or male cohort; however, an inverse association was found between maternal 3rd trimester IL-6 levels and the sum of skinfolds at birth in the female cohort (18). Thus, most of the previous studies have shown an inverse association between maternal pro-inflammatory markers, including CRP, IL-6 and/or TNF-α, and offspring anthropometry at birth. For the first time, our data extends this now to a metabolically high-risk population of women with GDM. Evidence indicates a U-shaped curve relating the size at birth with long-term cardiometabolic disease, highlighting the significance of optimal fetal growth for long-term health outcomes (42, 43). The impact of maternal pro-inflammatory factors on fetal growth may be mediated by their direct and indirect effects on the placental development, function and immunomodulatory activity, as well as on the hormone synthesis and action in the materno-fetal unit (41, 44, 45). The absence of association between IL-6 levels and BMI or weight in our study may be possibly explained by the fact that IL-6 correlates more closely with visceral adiposity (46), which was not specifically evaluated.
We found no associations between cord blood inflammatory biomarkers and offspring anthropometry at birth. Similarly, studies in the general population as well as in populations with high prevalence of GDM, and/or pre-existing diabetes, found no association between cord blood IL-6, CRP or increased CRP [CRP >0.3 mg/l (2.9 nmol/l)] levels and one or more anthropometric/adiposity measures at birth (including weight, weight z-score, LGA, fat mass, % body fat, sum of skinfold thickness) (21, 24–26).During the process of birth, there is a physiological increase in the cord blood pro-inflammatory marker concentration and this increase may be further influenced by other parameters, including the duration of labor and the mode of delivery (47, 48).Therefore, cord blood inflammatory biomarkers may be a complex reflexion of both the prenatal and the perinatal fetal inflammatory milieu, explaining the correlation between inflammatory biomarkers in the cord blood and offspring anthropometry later in life (at 1 year) but not at birth. Yeung et al, found an association between cord blood CRP levels and DNA methylation in the cord blood, particularly in gene regions associated with angiogenic and inflammatory pathways, which in turn could have an impact on future cardio-metabolic risk (49). Recent research suggests that methylation in specific inflammation-related genes in the cord blood is associated with adiposity measures later in the development, such as in early childhood (50). Additional investigation is warranted to elucidate the complex mechanisms that underlie the impact of pro-inflammatory cytokines in the cord blood on offspring body composition and metabolic profile during childhood.
4.2 Impact of maternal and fetal metabolism on infant weight and BMI during childhood
In our population, maternal serum concentrations of TNF-α during the 3rd trimester were inversely associated with offspring weight and BMI at 1 year. Our results are in agreement with a study in a population of women with a relatively low GDM prevalence (4.5%), where higher 2nd trimester CRP levels were associated with a higher childhood fat mass index (FMI), and trunk FMI, but this later in the development, i.e. in early (3-5 years) and mid childhood (7-10 years) (23). Our results are, however, contrary to a study performed in the general population where 1st trimester IL-6 and TNF-α were not associated with offspring adiposity measures, including weight, BMI z-scores at 2-6 years (31). In another study, 1st trimester IL-6 and TNF-α were not associated with weight and adiposity measures at 6 months of age in the total and male cohort, but only with adiposity measures in the female cohort (18). In a large, Danish general population, CRP, TNF-α, IL-6, and IL-1b measures in the 3rd trimester was not associated with offspring BMI, waist circumference, blood pressure, glucose metabolism measures, or lipid profile at the age of 20 years (51).
Data on the association between fetal (cord blood) inflammatory parameters and offspring growth during the first years of life are scarce. The above mentioned study of women with a history of macrosomia in the absence of GDM, found no association between cord blood IL-6, and TNF-α and offspring weight or adiposity measures at 6 months in their cohort (18). Regarding fetal predictors, in a cohort of children born extremely premature (< 28 weeks gestational age), showed that elevated IL-6 on day 1 in the newborn was associated with an increased risk for obesity at 2 years in multivariate models (52).
4.3 Speculations on the mechanisms of impact of maternal and fetal metabolism on offspring anthropometry during the first years of life in women with GDM
In pregnancies with GDM, the impact on offspring anthropometry is multifaceted, involving intricate mechanisms that span maternal, placental, and fetal domains (38, 53, 54). These include various factors such as maternal insulin resistance, elevated cytokines, and subsequent effects on placental function (41, 44). Inflammation and hyperglycemia lead to disruptions in insulin-like growth factors and adiponectin influencing fetal growth patterns and adipose tissue development (55, 56). Exposure to GDM may induce epigenetic modifications, affecting gene expression linked to fetal development and could be a mechanism leading to metabolic dysfunction later in life (57). Additionally, maternal obesity and excessive gestational weight gain, prevalent in GDM, independently contribute to adverse offspring outcomes (58, 59). This collective interplay underscores the complexity of factors influencing offspring anthropometry in the context of GDM, emphasizing the need for a comprehensive understanding of both inflammatory and metabolic pathways in order to identify potential therapeutic targets.
4.4 Strengths and limitations
To our knowledge, this is the first study assessing the impact of maternal and fetal inflammatory biomarkers on offspring anthropometric parameters at birth and up to 1 year of life in a metabolically high-risk population of women with GDM and their offspring. Another strength of our study is the prospective design. Nevertheless, some limitations ought to be mentioned. Maternal inflammatory biomarkers were measured only once during gestation. Moreover, offspring predictors did not include height and head circumference as we opted to focus on metabolic health-related anthropometric parameters. As the focus of this study was to investigate the relationship between biomarkers and offspring metabolic health-related offspring in a metabolically high-risk population, we lack a proper control group of healthy participants. Cord blood (umbilical artery) parameters were only available for 39 patients; which could influence our results, especially the correlations. Additionally, due to the small sample size of cord blood parameters, separate analyses for the intervention and usual care group, as well as infant sex could not be performed. However, maternal and fetal predictors and infant anthropometry did not differ between both groups and we always adjusted for group allocation.
4.5 Conclusions
Maternal and fetal inflammatory biomarkers including TNF-α at 24-32 weeks of GA and cord blood CRP distinctively influenced offspring weight and BMI during the first year of life, and this independent of maternal age, ethnicity, and pre-pregnancy BMI. The impact of fetal inflammatory biomarkers was not apparent at birth but was observed at 1 year. Further research is warranted to elucidate how the inflammatory milieu before and during birth might impact the developing offspring, and may in turn have implications for designing intervention strategies based on maternal and cord blood inflammatory biomarkers in order to decrease the risk of offspring metabolic dysfunction in the medium and long-term.
MySweetHeart Research Group
AA, Pascal Bovet, Arnaud Chiolero, Stefano Di Bernardo, Adina Mihaela Epure, Sandrine Estoppey Younes, LG, Justine Gross, AH, Stefano Lanzi, Seyda Mayerat, Yvan Mivelaz, JP, DQ, Jean-Benoit Rossel, Nicole Sekarski, Umberto Simeoni, BS, Yvan Vial.
Data availability statement
The datasets presented in this article are not readily available because many of them are still being worked on but are available from the corresponding author on reasonable request. Requests to access the datasets should be directed toamFyZGVuYS5wdWRlckBjaHV2LmNo.
Ethics statement
The study was approved by the Human Research Ethics Committee of the Canton de Vaud (study number 2016-00745). The study was conducted in accordance with the local legislation and institutional requirements. Written informed consent for participation in this study was obtained from all participating women (for themselves and their offspring).
Author contributions
M-CA: Formal analysis, Writing – original draft. DYQ: Investigation, Methodology, Writing – review & editing. LG: Investigation, Methodology, Writing – review & editing. AA: Investigation, Methodology, Writing – review & editing. SS: Methodology, Writing – review & editing. AL: Methodology, Writing – review & editing. BS: Methodology, Writing – review & editing. AH: Conceptualization, Funding acquisition, Writing – review & editing. JJP: Conceptualization, Funding acquisition, Project administration, Supervision, Writing – review & editing.
Funding
The author(s) declare financial support was received for the research, authorship, and/or publication of this article. This study is funded by a project grant from the Swiss National Science Foundation (SNF 32003B_176119) and by an unrestricted educational grant from Novo Nordisk and The Gottfried und Julia Bangerter-Rhyner-Stiftung Foundation. M-CA’s research is also supported by an unrestricted grant by the Sophie Afenduli foundation and the Anna and André Livio-Glauser Foundation. The funding bodies did not take part in the design of the study, the collection, analysis, interpretation of data or in the writing of the manuscript.
Acknowledgments
We are very grateful to our study participants and their children and partners for their time and participation. We thank Deborah Degen, Dominique Stulz and Isabelle Cohen-Salmon who helped with data collection.
Conflict of interest
The authors declare that the research was conducted in the absence of any commercial or financial relationships that could be construed as a potential conflict of interest.
This study is funded by a project grant from the Swiss National Science Foundation (SNF 32003B_176119) and by an unrestricted educational grant from Novo Nordisk and The Gottfried und Julia Bangerter-Rhyner-Stiftung Foundation. The funders were not involved in the study design, collection, analysis, interpretation of data, the writing of this article or the decision to submit it for publication.
Publisher’s note
All claims expressed in this article are solely those of the authors and do not necessarily represent those of their affiliated organizations, or those of the publisher, the editors and the reviewers. Any product that may be evaluated in this article, or claim that may be made by its manufacturer, is not guaranteed or endorsed by the publisher.
Supplementary material
The Supplementary Material for this article can be found online at: https://www.frontiersin.org/articles/10.3389/fendo.2024.1333755/full#supplementary-material
Abbreviations
AGA, Appropriate for Gestational Age; BMI, body mass index; CI, confidence interval; CRP, C-reactive protein; DS, standard deviation, FMI, fat mass index; GA, Gestational age; GDM, gestational diabetes mellitus; GWG, gestational weight gain; HAPO, Hyperglycemia and Adverse Pregnancy Outcome; HbA1c, glycated hemoglobin; IADPSG, International Association of Diabetes and Pregnancy Study Group; IL, interleukin; IOM, institute of medicine; LGA, Large for gestational age; N, Number; oGTT, oral glucose tolerance test; OR, odds ratio; SGA, Small for gestational age; TNF-α, tumor necrosis factor alpha.
References
1. Cozlea DL, Farcas DM, Nagy A, Keresztesi AA, Tifrea R, Cozlea L, et al. The impact of C reactive protein on global cardiovascular risk on patients with coronary artery disease. Curr Health Sci J. (2013) 39:225–31.
2. Barzilay JI, Abraham L, Heckbert SR, Cushman M, Kuller LH, Resnick HE, et al. The relation of markers of inflammation to the development of glucose disorders in the elderly: the Cardiovascular Health Study. Diabetes. (2001) 50:2384–9. doi: 10.2337/diabetes.50.10.2384
3. Esposito K, Giugliano D. The metabolic syndrome and inflammation: association or causation? Nutr Metab Cardiovasc Dis. (2004) 14:228–32. doi: 10.1016/S0939-4753(04)80048-6
4. Gomez-Lopez N, Galaz J, Miller D, Farias-Jofre M, Liu Z, Arenas-Hernandez M, et al. The immunobiology of preterm labor and birth: intra-amniotic inflammation or breakdown of maternal-fetal homeostasis. Reproduction. (2022) 164:R11–r45. doi: 10.1530/REP-22-0046
5. Challis JR, Lockwood CJ, Myatt L, Norman JE, Strauss JF, Petraglia F. Inflammation and pregnancy. Reprod Sci. (2009) 16:206–15. doi: 10.1177/1933719108329095
6. Wolff Mv, Thaler CJ, Strowitzki T, Broome J, Stolz W, Tabibzadeh S. Regulated expression of cytokines in human endometrium throughout the menstrual cycle: dysregulation in habitual abortion. Mol Hum Reprod. (2000) 6:627–34. doi: 10.1093/molehr/6.7.627
7. Yockey LJ, Iwasaki A. Interferons and proinflammatory cytokines in pregnancy and fetal development. Immunity. (2018) 49:397–412. doi: 10.1016/j.immuni.2018.07.017
8. Alijotas-Reig J, Esteve-Valverde E, Ferrer-Oliveras R, Llurba E, Gris JM. Tumor necrosis factor-alpha and pregnancy: Focus on biologics. An updated and comprehensive review. Clin Rev Allergy Immunol. (2017) 53:40–53. doi: 10.1007/s12016-016-8596-x
9. Graham AM, Rasmussen JM, Rudolph MD, Heim CM, Gilmore JH, Styner M, et al. Maternal systemic interleukin-6 during pregnancy is associated with newborn amygdala phenotypes and subsequent behavior at 2 years of age. Biol Psychiatry. (2018) 83:109–19. doi: 10.1016/j.biopsych.2017.05.027
10. Pinto Y, Frishman S, Turjeman S, Eshel A, Nuriel-Ohayon M, Shrossel O, et al. Gestational diabetes is driven by microbiota-induced inflammation months before diagnosis. Gut. (2023) 72:918–28. doi: 10.1136/gutjnl-2022-328406
11. Li YX, Long DL, Liu J, Qiu D, Wang J, Cheng X, et al. Gestational diabetes mellitus in women increased the risk of neonatal infection via inflammation and autophagy in the placenta. Med (Baltimore). (2020) 99:e22152. doi: 10.1097/MD.0000000000022152
12. McLachlan KA, O'Neal D, Jenkins A, Alford FP. Do adiponectin, TNFalpha, leptin and CRP relate to insulin resistance in pregnancy? Studies in women with and without gestational diabetes, during and after pregnancy. Diabetes Metab Res Rev. (2006) 22:131–8. doi: 10.1002/dmrr.591
13. Can B, Tutuncu Y, Can B, Keskin H, Bekpinar S, Dinccag N. Inflammatory markers are associated with the progression of gestational diabetes to metabolic syndrome. J Obstet Gynaecol. (2022) 42:1857–61. doi: 10.1080/01443615.2022.2048363
14. Atègbo JM, Grissa O, Yessoufou A, Hichami A, Dramane KL, Moutairou K, et al. Modulation of adipokines and cytokines in gestational diabetes and macrosomia. J Clin Endocrinol Metab. (2006) 91:4137–43. doi: 10.1210/jc.2006-0980
15. Carpenter MW. Gestational diabetes, pregnancy hypertension, and late vascular disease. Diabetes Care. (2007) 30:S246–S50. doi: 10.2337/dc07-s224
16. Quansah DY, Horsch A, Gilbert L, Donath MY, Puder JJ. C-reactive protein during pregnancy and in the early postpartum predicts adverse metabolic health outcomes at 1 year postpartum in women with gestational diabetes. Cardiovasc Diabetol. (2023) 22:291. doi: 10.1186/s12933-023-02034-9
17. Kırıcı P, Çağıran FT, Kalı Z, Tanrıverdi ES, Mavral N, Ecin SM. Determination of maternal serum pro-inflammatory cytokine changes in intrauterine growth restriction. Eur Rev Med Pharmacol Sci. (2023) 27(5):1996–2001. doi: 10.26355/eurrev_202303_31565
18. Donnelly JM, Lindsay K, Walsh JM, Horan MK, O’Shea D, Molloy EJ, et al. Perinatal inflammation and childhood adiposity – a gender effect? J Maternal-Fetal Neonatal Med. (2020) 33:1203–10. doi: 10.1080/14767058.2018.1517315
19. Kuzawa CW, Fried RL, Borja JB, McDade TW. Maternal pregnancy C-reactive protein predicts offspring birth size and body composition in metropolitan Cebu, Philippines. J Dev Orig Health Dis. (2017) 8:674–81. doi: 10.1017/S2040174417000502
20. Lowe LP, Metzger BE, Lowe WL Jr., Dyer AR, McDade TW, McIntyre HD. Inflammatory mediators and glucose in pregnancy: Results from a subset of the hyperglycemia and adverse pregnancy outcome (HAPO) study. J Clin Endocrinol Metab. (2010) 95:5427–34. doi: 10.1210/jc.2010-1662
21. Radaelli T, Uvena-Celebrezze J, Minium J, Huston-Presley L, Catalano P, Hauguel-de Mouzon S. Maternal interleukin-6: marker of fetal growth and adiposity. J Soc Gynecol Investig. (2006) 13:53–7. doi: 10.1016/j.jsgi.2005.10.003
22. Farah N, Hogan AE, O'Connor N, Kennelly MM, O'Shea D, Turner MJ. Correlation between maternal inflammatory markers and fetomaternal adiposity. Cytokine. (2012) 60:96–9. doi: 10.1016/j.cyto.2012.05.024
23. Gaillard R, Rifas-Shiman SL, Perng W, Oken E, Gillman MW. Maternal inflammation during pregnancy and childhood adiposity. Obes (Silver Spring). (2016) 24:1320–7. doi: 10.1002/oby.21484
24. Lee IL, Barr ELM, Longmore D, Barzi F, Brown ADH, Connors C, et al. Cord blood metabolic markers are strong mediators of the effect of maternal adiposity on fetal growth in pregnancies across the glucose tolerance spectrum: the PANDORA study. Diabetologia. (2020) 63:497–507. doi: 10.1007/s00125-019-05079-2
25. Vasilakos LK, Steinbrekera B, Santillan DA, Santillan MK, Brandt DS, Dagle D, et al. Umbilical cord blood leptin and IL-6 in the presence of maternal diabetes or chorioamnionitis. Front Endocrinol (Lausanne). (2022) 13:836541. doi: 10.3389/fendo.2022.836541
26. Aramesh MR, Dehdashtian M, Malekian A, ShahAli S, Shojaei K. Relation between fetal anthropometric parameters and cord blood adiponectin and high-sensitivity C-reactive protein in gestational diabetes mellitus. Arch Endocrinol Metab. (2017) 61:228–32. doi: 10.1590/2359-3997000000235
27. Rea IM, Gibson DS, McGilligan V, McNerlan SE, Alexander HD, Ross OA. Age and age-related diseases: Role of inflammation triggers and cytokines. Front Immunol. (2018) 9. doi: 10.3389/fimmu.2018.00586
28. Cox AJ, West NP, Cripps AW. Obesity, inflammation, and the gut microbiota. Lancet Diabetes Endocrinol. (2015) 3:207–15. doi: 10.1016/S2213-8587(14)70134-2
29. Fernández-Sánchez A, Madrigal-Santillán E, Bautista M, Esquivel-Soto J, Morales-González A, Esquivel-Chirino C, et al. Inflammation, oxidative stress, and obesity. Int J Mol Sci. (2011) 12:3117–32. doi: 10.3390/ijms12053117
30. Yu MG, King GL. Inflammation and incident diabetes: The role of race and ethnicity. J Clin Endocrinol Metab. (2022) 107:e3082–e3. doi: 10.1210/clinem/dgac132
31. Maguire RL, House JS, Lloyd DT, Skinner HG, Allen TK, Raffi AM, et al. Associations between maternal obesity, gestational cytokine levels and child obesity in the NEST cohort. Pediatr Obes. (2021) 16:e12763. doi: 10.1111/ijpo.12763
32. Horsch A, Gilbert L, Lanzi S, Gross J, Kayser B, Vial Y, et al. Improving cardiometabolic and mental health in women with gestational diabetes mellitus and their offspring: study protocol for MySweetHeart Trial, a randomised controlled trial. BMJ Open. (2018) 8:e020462. doi: 10.1136/bmjopen-2017-020462
33. International Association of Diabetes and Pregnancy Study Groups. Recommendations on the diagnosis and classification of hyperglycemia in pregnancy. Diabetes Care. (2010) 33:676–82. doi: 10.2337/dc09-1848
34. Blumer I, Hadar E, Hadden DR, Jovanovič L, Mestman JH, Murad MH, et al. Diabetes and pregnancy: An endocrine society clinical practice guideline. J Clin Endocrinol Metab. (2013) 98:4227–49. doi: 10.1210/jc.2013-2465
35. ElSayed NA, Aleppo G, Aroda VR, Bannuru RR, Brown FM, Bruemmer D, et al. 15. Management of diabetes in pregnancy: Standards of care in diabetes-2023. Diabetes Care. (2023) 46:S254–s66. doi: 10.2337/dc23-S015
36. Saneh H, Mendez MD, Srinivasan VN. Cord Blood Gas. Treasure Island, Florida, USA: StatPearls Publishing (2023).
37. Villar J, Cheikh Ismail L, Victora CG, Ohuma EO, Bertino E, Altman DG, et al. International standards for newborn weight, length, and head circumference by gestational age and sex: the Newborn Cross-Sectional Study of the INTERGROWTH-21st Project. Lancet. (2014) 384:857–68. doi: 10.1016/S0140-6736(14)60932-6
38. Antoniou MC, Quansah DY, Mühlberg S, Gilbert L, Arhab A, Schenk S, et al. Maternal and fetal predictors of anthropometry in the first year of life in offspring of women with GDM. Front Endocrinol (Lausanne). (2023) 14:1144195. doi: 10.3389/fendo.2023.1144195
39. WHO. Child Growth Standards based on length/height, weight and age. Acta Paediatr Suppl. (2006) 450:76–85. doi: 10.1111/j.1651-2227.2006.tb02378.x
40. Nikbakht R, Moghadam EK, Nasirkhani Z. Maternal serum levels of C-reactive protein at early pregnancy to predict fetal growth restriction and preterm delivery: A prospective cohort study. Int J Reprod Biomed. (2020) 18:157–64. doi: 10.18502/ijrm.v18i3.6710
41. Francis EC, Li M, Hinkle SN, Chen J, Wu J, Zhu Y, et al. Maternal proinflammatory adipokines throughout pregnancy and neonatal size and body composition: A prospective study. Curr Dev Nutr. (2021) 5:nzab113. doi: 10.1093/cdn/nzab113
42. Huang RC, Burke V, Newnham JP, Stanley FJ, Kendall GE, Landau LI, et al. Perinatal and childhood origins of cardiovascular disease. Int J Obes (Lond). (2007) 31:236–44. doi: 10.1038/sj.ijo.0803394
43. Hokken-Koelega ACS, van der Steen M, Boguszewski MCS, Cianfarani S, Dahlgren J, Horikawa R, et al. International consensus guideline on small for gestational age: Etiology and management from infancy to early adulthood. Endocr Rev. (2023) 44:539–65. doi: 10.1210/endrev/bnad002
44. Romanowska-Próchnicka K, Felis-Giemza A, Olesińska M, Wojdasiewicz P, Paradowska-Gorycka A, Szukiewicz D. The role of TNF-α and anti-TNF-α Agents during preconception, pregnancy, and breastfeeding. Int J Mol Sci. (2021) 22(6):2922. doi: 10.3390/ijms22062922
45. Prins JR, Gomez-Lopez N, Robertson SA. Interleukin-6 in pregnancy and gestational disorders. J Reprod Immunol. (2012) 95:1–14. doi: 10.1016/j.jri.2012.05.004
46. Park HS, Park JY, Yu R. Relationship of obesity and visceral adiposity with serum concentrations of CRP, TNF-alpha and IL-6. Diabetes Res Clin Pract. (2005) 69:29–35. doi: 10.1016/j.diabres.2004.11.007
47. Nandanan B, Chua MC, Chiang WC, Goh A, Kumar D, Knippels L, et al. Influence of mode of delivery on cytokine expression in cord blood. Hum Immunol. (2019) 80:533–6. doi: 10.1016/j.humimm.2019.03.018
48. Logan CA, Thiel L, Bornemann R, Koenig W, Reister F, Brenner H, et al. Delivery mode, duration of labor, and cord blood adiponectin, leptin, and C-reactive protein: Results of the population-based ulm birth cohort studies. PLoS One. (2016) 11:e0149918. doi: 10.1371/journal.pone.0149918
49. Yeung EH, Guan W, Zeng X, Salas LA, Mumford SL, de Prado Bert P, et al. Cord blood DNA methylation reflects cord blood C-reactive protein levels but not maternal levels: a longitudinal study and meta-analysis. Clin Epigenetics. (2020) 12:60. doi: 10.1186/s13148-020-00852-2
50. Wu S, Gennings C, Wright RJ, Wilson A, Burris HH, Just AC, et al. Prenatal stress, methylation in inflammation-related genes, and adiposity measures in early childhood: the programming research in obesity, growth environment and social stress cohort study. Psychosom Med. (2018) 80:34–41. doi: 10.1097/PSY.0000000000000517
51. Danielsen I, Granström C, Rytter D, Halldorsson TI, Bech BH, Henriksen TB, et al. Subclinical inflammation during third trimester of pregnancy was not associated with markers of the metabolic syndrome in young adult offspring. Obes (Silver Spring). (2014) 22:1351–8. doi: 10.1002/oby.20650
52. Perrin EM, O'Shea TM, Skinner AC, Bose C, Allred EN, Fichorova RN, et al. Elevations of inflammatory proteins in neonatal blood are associated with obesity and overweight among 2-year-old children born extremely premature. Pediatr Res. (2018) 83:1110–9. doi: 10.1038/pr.2017.313
53. Lowe WL Jr., Lowe LP, Kuang A, Catalano PM, Nodzenski M, Talbot O, et al. Maternal glucose levels during pregnancy and childhood adiposity in the Hyperglycemia and Adverse Pregnancy Outcome Follow-up Study. Diabetologia. (2019) 62:598–610. doi: 10.1007/s00125-018-4809-6
54. Dabelea D, Hanson RL, Lindsay RS, Pettitt DJ, Imperatore G, Gabir MM, et al. Intrauterine exposure to diabetes conveys risks for type 2 diabetes and obesity: a study of discordant sibships. Diabetes. (2000) 49:2208–11. doi: 10.2337/diabetes.49.12.2208
55. Moyce Gruber BL, Dolinsky VW. The role of adiponectin during pregnancy and gestational diabetes. Life. (2023) 13:301. doi: 10.3390/life13020301
56. Martín-Estal I, Castorena-Torres F. Gestational diabetes mellitus and energy-dense diet: What is the role of the insulin/IGF axis? Front Endocrinol (Lausanne). (2022) 13:916042. doi: 10.3389/fendo.2022.916042
57. Dłuski DF, Wolińska E, Skrzypczak M. Epigenetic changes in gestational diabetes mellitus. Int J Mol Sci. (2021) 22(14):7649. doi: 10.3390/ijms22147649
58. Antoniou MC, Gilbert L, Gross J, Rossel JB, Fischer Fumeaux CJ, Vial Y, et al. Potentially modifiable predictors of adverse neonatal and maternal outcomes in pregnancies with gestational diabetes mellitus: can they help for future risk stratification and risk-adapted patient care? BMC Pregnancy Childbirth. (2019) 19:469. doi: 10.1186/s12884-019-2610-2
Keywords: gestational diabetes, offspring anthropometry, perinatal inflammation, cord blood CRP, maternal pro-inflammatory cytokines
Citation: Antoniou M-C, Quansah DY, Gilbert L, Arhab A, Schenk S, Lacroix A, Stuijfzand B, Horsch A and Puder JJ (2024) Association between maternal and fetal inflammatory biomarkers and offspring weight and BMI during the first year of life in pregnancies with GDM: MySweetheart study. Front. Endocrinol. 15:1333755. doi: 10.3389/fendo.2024.1333755
Received: 05 November 2023; Accepted: 22 April 2024;
Published: 10 May 2024.
Edited by:
Enrique Podaza, NewYork-Presbyterian, United StatesReviewed by:
Vipin Alukkal Vidyadharan, Baylor College of Medicine, United StatesSandeep Moothedath Subrahmanian, The Pennsylvania State University, United States
Copyright © 2024 Antoniou, Quansah, Gilbert, Arhab, Schenk, Lacroix, Stuijfzand, Horsch and Puder. This is an open-access article distributed under the terms of the Creative Commons Attribution License (CC BY). The use, distribution or reproduction in other forums is permitted, provided the original author(s) and the copyright owner(s) are credited and that the original publication in this journal is cited, in accordance with accepted academic practice. No use, distribution or reproduction is permitted which does not comply with these terms.
*Correspondence: Jardena Jacqueline Puder, amFyZGVuYS5wdWRlckBjaHV2LmNo
†These authors have contributed equally to this work and share first authorship