- 1Laboratory of Molecular Cardiology, The First Affiliated Hospital of Shantou University Medical College, Shantou, Guangdong, China
- 2Laboratory of Medical Molecular Imaging, The First Affiliated Hospital of Shantou University Medical College, Shantou, Guangdong, China
- 3Shantou University Medical College, Shantou, Guangdong, China
- 4Tianjin Hospital, Tianjin, China
- 5Department of Cardiology, Binzhou Medical University Hospital, Binzhou, Shandong, China
- 6International Medical Service Center, The First Affiliated Hospital of Shantou University Medical College, Shantou, Guangdong, China
- 7Engineering Research Center of Key Technique for Biotherapy of Guangdong, Shantou, Guangdong, China
Background: The causal association between gut microbiota (GM) and the development of diabetic nephropathy (DN) remains uncertain. We sought to explore this potential association using two-sample Mendelian randomization (MR) analysis.
Methods: Genome-wide association study (GWAS) data for GM were obtained from the MiBioGen consortium. GWAS data for DN and related phenotypes were collected from the FinngenR9 and CKDGen databases. The inverse variance weighted (IVW) model was used as the primary analysis model, supplemented by various sensitivity analyses. Heterogeneity was assessed using Cochran’s Q test, while horizontal pleiotropy was evaluated through MR-Egger regression and the MR-PRESSO global test. Reverse MR analysis was conducted to identify any reverse causal effects.
Results: Our analysis identified twenty-five bacterial taxa that have a causal association with DN and its related phenotypes (p < 0.05). Among them, only the g_Eubacterium_coprostanoligenes_group showed a significant causal association with type 1 DN (p < Bonferroni-adjusted p-value). Our findings remained consistent regardless of the analytical approach used, with all methods indicating the same direction of effect. No evidence of heterogeneity or horizontal pleiotropy was observed. Reverse MR analysis did not reveal any causal associations.
Conclusions: This study established a causal association between specific GM and DN. Our findings contribute to current understanding of the role of GM in the development of DN, offering potential insights for the prevention and treatment strategies for this condition.
1 Introduction
Diabetes mellitus (DM) presents a substantial socioeconomic and medical challenge due to its high prevalence and associated morbidity and mortality rates. In, 2019, the estimated global prevalence of DM was 9.3%, affecting 463 million individuals, with projections indicating a rise to 579 million by, 2030 and 700 million by, 2045 (1). Among the various complications of DM, diabetic nephropathy (DN) is one of the most prevalent, afflicting approximately 40% of DM patients (2). DN is now understood to be a debilitating and potentially fatal condition that significantly impacts patient quality of life and life expectancy. While hyperglycemia is recognized as the primary culprit, the underlying mechanisms behind DN development remain elusive. Furthermore, the pathophysiological changes associated with DN encompass hemodynamic abnormalities, inflammatory responses, and oxidative stress (3). Genetic factors, dietary choices, and lifestyle also play pivotal roles in the progression of DN. Recent research has shed light on the relationship between dysregulated gut microbiota (GM) and the onset and progression of DN (4, 5).
GM has emerged as a research hotspot in recent years. The concept of the gut-kidney axis, elucidating the intricate interplay between kidney disease and GM, was first introduced by Meijers BK et al. in, 2011 (6). There is a growing consensus suggesting a close relationship between intestinal dysbiosis and the occurrence of DM and its associated complications, including DN (7, 8). It was reported that over the last seven years, 124 articles focusing on GM and DN were published, and these continue to grow (9). The exploration of the underlying pathogenic mechanisms around obesity, inflammation, oxidative stress, metabolite imbalance, and the efficacy of Chinese herbal medicine is a hotspot for related research (9). The pathogenic role of GM and its metabolites in the development of DN is complex (3, 10). On the one hand, intestinal dysbiosis can induce persistent intestinal inflammation and hyperpermeability due to abnormal continuous stimulation (11). This, coupled with the depletion of beneficial metabolites and the accumulation of harmful ones resulting from intestinal dysbiosis, may contribute to kidney damage. Conversely, impaired renal function diminishes the excretion of harmful substances, potentially affecting the integrity of the intestinal barrier (12). Nitrogenous substances permeate the intestinal barrier due to increased permeability, consequently influencing the composition and function of GM. Moreover, GM plays an integral role in diabetes development by modulating various pathophysiological processes, including insulin secretion, insulin sensitivity, immune system regulation, and glycemic and lipid regulation (13, 14). Short chain fatty acids (SCFAs) deficiency is another important factor that may contribute to the progression of DN induced by intestinal dysbiosis (15). Cai KD et al. found a decrease of SCFAs concentration and SCFAs-related GM were found in patients with DN (16). Except for SCFAs, other metabolites of GM, like free fat acid, bile acid and Trimethylamine-N-oxide (TMAO), also play crucial role in the development of DN (15). These findings have stimulated research into the potential causal relationship between the intestinal dysbiosis and the development of DN.
To date, several observational studies have investigated the association between intestinal dysbiosis and DN (17–22). However, these studies are prone to inherent biases and reverse causation due to the inability to fully account for confounding factors. Consequently, establishing a causal link between GM and DN has remained a formidable challenge. The rapid advancement of Mendelian randomization (MR) analysis has provided a robust analytical tool for investigating causal relationships between GM and DN (23). As alleles are randomly inherited from parents to offspring during conception, a process akin to the random assignment of individuals to treatment and control groups in experiments, common confounding factors yield little effect over genetic variants. Consequently, by employing genetic variants as instrumental variables (IVs) to stand in for the exposure variable, MR analysis effectively nullifies the adverse effects of confounders commonly encountered in observational studies (24). This approach enhances the comprehensibility of findings and bolsters their credibility. In recent years, MR analysis has gained widespread recognition and application as a potent tool for exploring potential causal links between exposures and outcomes (23). Herein, we harnessed the capabilities of two-sample MR analysis to delve into the plausible causal connection between GM and the development of DN. Our research endeavors open up promising avenues for gaining fresh perspectives on early DN diagnosis and treatment centered around GM’s intricate role in the process.
2 Materials and methods
2.1 Ethical statement
Our current MR analysis used publicly available genome-wide association study (GWAS) datasets. Informed consent and ethical approval were obtained during the original GWAS study. As no new data were added, the need for ethical approval was waived for the present MR analysis.
2.2 Assumptions underlying MR
The design of the present study was based on the three assumptions of MR as follows (25): (1) a strong and reliable association exists between the genetic variants and the risk factors (the gut bacteria); (2) there is no association between the genetic variants and confounding factors; (3) there are no alternative causal pathways linking the genetic variants to the outcome (the development of DN) other than through the risk factors under investigation. Figure 1 shows the flow chart of the present MR analysis.
2.3 Exposure data of MR analysis
The GWAS summary statistics data used to identify potential proxy single nucleotide polymorphisms (SNPs) were sourced from the MiBioGen database, which originated from an extensive GWAS meta-analysis comprising 18,340 participants with 16S rRNA gene sequencing data (26). This dataset encompassed information collected from 24 population-based cohorts representing diverse ethnic groups, with participants of European descent (n=13,266), from the Middle East (n=481), Latin America (n=1,097), East Asia (n=811), the United States (n=114), and of multiple ancestral backgrounds (n=2,571). Following the exclusion of 15 taxa with unidentified classification (12 at the genus level and 3 at the family level), a total of 196 taxa were included in the final analysis, comprising 119 genera, 32 families, 20 orders, 16 classes, and 9 phyla.
2.4 Outcome data of MR analysis
Three GWAS summary statistics data for DN were obtained from the FinngenR9 database (https://r9.finngen.fi/) (27), including diabetic nephropathy more control exclusions (DM_NEPHROPATHY_EXMORE), type 1 diabetes with renal complications (T1DN, E4_DM1REN) and type 2 diabetes with renal complications (T2DN, E4_DM2REN). In addition, three DN-related phenotypes, including microalbuminuria (MA) (28), urine albumin creatine ratio (UACR) (28), and glomerular filtration rate (GFR) (29) in diabetic cohorts, were obtained from the CKDGEN database.
2.5 Selection of optimal IVs for exposure
Firstly, we included SNPs related to gut bacterial taxa in the MiBioGen database with a genome-wide significance threshold of p < 1×10-05, as reported in a previous study (30). Next, to minimize the effect of linkage disequilibrium (LD) on the results, the LD threshold and the window size for clumping were set to r2 < 0.001 and, 10000kb, respectively, based on the European-based 1,000 Genome Projects. To mitigate bias from weak IVs, SNPs with an F-statistics value less than 10 were excluded (F-statistics value = (β/SE)2) (31). Potential IVs closely related to the outcome phenotype were removed (p<5×10-08).
2.6 MR statistical analyses
Several outcome indicators, including the inverse variance weighted (IVW) method under random effects (32), weighted median (WM) (33), MR-Egger regression (34), and MR-PRESSO methods (35), were used to improve the robustness of our findings. Among these, the IVW method was used as the primary analysis, while the other three methods were used as adjuncts to IVW to verify the robustness of IVW results. During IVW analysis, which yields unbiased estimates, all SNPs considered in the study are deemed valid instrumental variables. During WM analysis, consistent effect estimates can be achieved even when over half of the weight assigned to the instruments contributing to the analysis is not considered valid. To evaluate potential directional pleiotropy, the MR-Egger regression and MR-Egger intercept analyses were employed (a p-value of less than 0.05 in the intercept analysis suggests horizontal pleiotropy). Heterogeneity was assessed using Cochran’s Q test with a significance threshold of p < 0.05. The criteria for detecting significant results were defined as follows (36): (1) the p-value for the IVW analysis was less than 0.05; (2) the direction of the effect obtained in the IVW, WM, MR-Egger regression, and MR-PRESSO methods should be consistent; (3) no significant horizontal pleiotropy was found in the MR-Egger intercept and MR-PRESSO analyses (p > 0.05); (4) the gut bacteria have similar clinical value in different significant phenotypes of DN. In the present study, we determined the statistical significance and potential association between gut bacteria and the risk of developing DN. A P value less than the Bonferroni adjusted IVW p (pFDR) cut-off was considered as statistically significant, while pFDR < p < 0.05 was interpreted as indicative of a potential link between gut bacteria and the susceptibility to developing DN. The pFDR value was 4.20×10-04(0.05/119) for the genus level, 1.56×10-03(0.05/32) for the family level, 2.50×10-03(0.05/20) for the order level, 3.13×10-03(0.05/16) for the class level and 5.56×10-03(0.05/9) for the phylum level. All results presented in our MR analysis were generated by the “TwoSampleMR” and “MRPRESSO” packages in R language software (version 4.3.1).
2.7 Reverse MR analysis
The reverse MR analysis was performed to detect the potential causal inference of DN development on the identified gut bacteria. In this part, DN and its phenotypes were considered as exposures, while the identified gut bacteria were considered as outcomes. SNPs extracted from the GWAS data related to DN and its phenotypes were identified as IVs. The criteria for identifying significant outcomes were similar to those used in the MR analysis.
3 Results
3.1 Selection of optimal SNPs
A total of 13,671 SNPs (p < 1×10-05) were obtained from the MiBioGen database (shown in Supplementary Table S1). After excluding ineligible SNPs, the most suitable SNPs were included in the final analysis. Comprehensive information regarding proxy SNPs can be found in Supplementary Tables S2-S7.
3.2 MR analyses
The MR analyses (as outlined in Table 1) revealed that g_Eubacterium_coprostanoligenes_group exhibited a significantly negative association with the development of T1DN using the IVW method (OR = 0.475, 95% CI 0.314-0.717, p = 3.93×10-04). Moreover, another twenty-four GMs showed potential associations with the risk of developing DN in the IVW method (pFDR < p < 0.05). All these findings were consistent across WM, MR-Egger regression, and MR-PRESSO methods. Table 2 summarizes the roles of the identified GMs in the development of DN across different phenotypes. The identified GMs mainly belong to the phyla Bacteroidetes, Firmicutes, Proteobacteria, and Verrucomicrobia. For a detailed breakdown of the MR analyses, please refer to Supplementary Tables S8-S13. Several GMs were demonstrated to be causally associated with different DN-related phenotypes, and we presented these results by venn diagrams (Figure 2). Scatter plots, leave-one-out sensitivity analysis plots, forest plots, and funnel plots illustrating the causal relationship between the identified GMs and DN development are presented in Figure 3 (for g_Eubacterium_coprostanoligenes_group) and Supplementary File S1 (for all identified GMs).
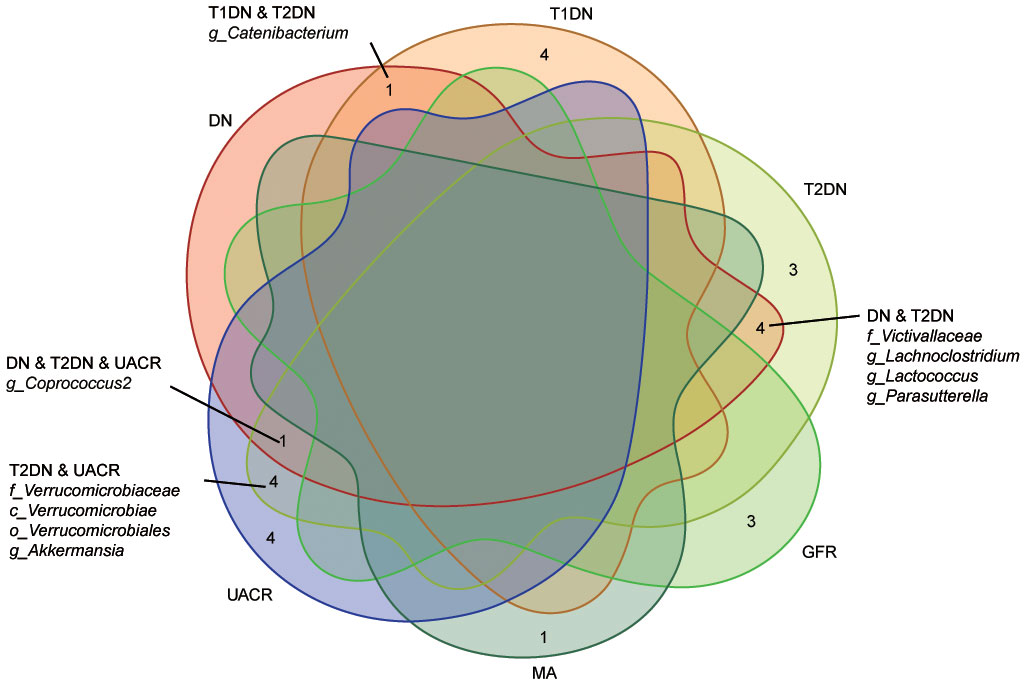
Figure 2 Venn diagram describes the significant findings obtained from different outcome GWAS data. Ten identified GMs were casually associated with the different kinds of phenotypes. DN, diabetic nephropathy; T1DN, type 1 diabetic nephropathy; T2DN, type 2 diabetic nephropathy; eGFR, estimated glomerular filtration rate; MA, microalbuminuria; UACR, urea albumin creatinine ratio.
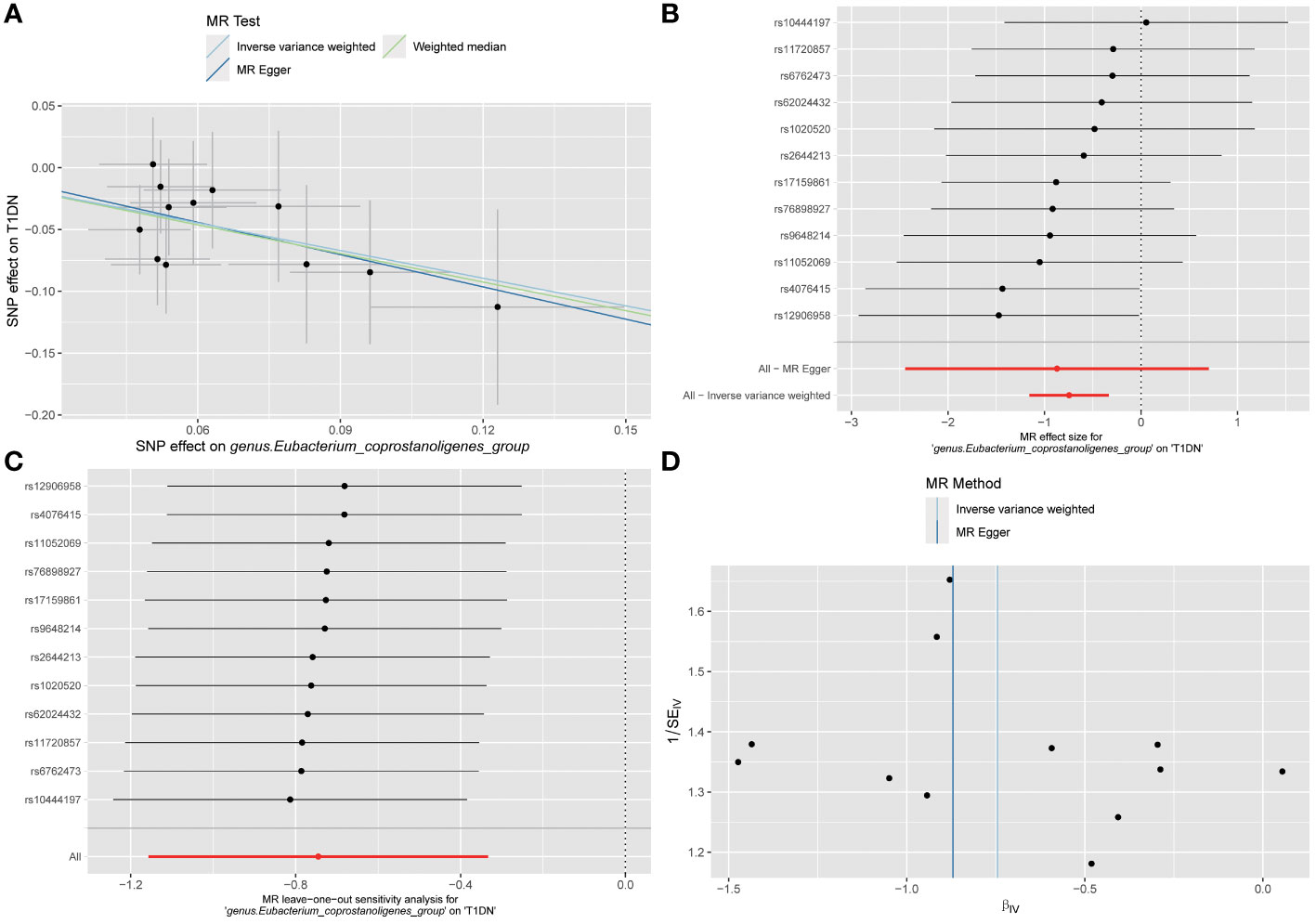
Figure 3 Visualization results for describing the causal association between g_Eubacterium_coprostanoligenes_group and the risk of developing T1DN. (A) scatter plot; (B) forest plot; (C) leave-one-out sensitivity analysis plot; (D) funnel plot. No significant outliers or publication bias found according to the visualization results.
3.3 Directional horizontal pleiotropy and heterogeneity analyses
Analysis of MR-Egger regression intercepts indicated the absence of significant directional horizontal pleiotropy (as depicted in Supplementary Table S14, all p > 0.05). While some potential outliers among IVs for GMs were identified in leave-one-out plots (refer to Supplementary File S1), no significant outliers were detected according to the results of MR-PRESSO global test analyses (outlined in Supplementary Table S15, all p > 0.05). Furthermore, in both IVW and WM analyses, Cochran’s Q test did not reveal any significant heterogeneity among the IVs (detailed in Supplementary Table S16, all p > 0.05). In summary, our findings suggest the absence of significant directional horizontal pleiotropy and heterogeneity in the current MR analysis.
3.4 Reverse MR analysis
In the reverse MR analysis, it was observed that the development of T2DN exhibited a positive causal association with the abundance of g_Lactococcus in the IVW analysis (OR 1.094, 95% CI 1.009-1.185, p = 0.028). However, this causal effect was not consistently robust. The MR-Egger method yielded a negative association, although it was not statistically significant (OR 0.997, 95% CI 0.744-1.337, p = 0.989). Due to the limited number of valid SNPs associated with UACR (n = 2), a systematic analysis of the causal effect of UACR on the identified GMs could not be carried out. Overall, there is no substantial evidence that the development of DN causally affects the abundance of all identified GMs (see Table 3).
4 Discussion
In the present study, the causal association between GM and DN was investigated by two-sample MR analysis. Leveraging a substantial amount of GWAS data, we preliminary explored GM’s potential causal influence at the genetic level on the onset and progression of DN. We found that the g_Eubacterium_coprostanoligenes_group exhibited a significant negative causal relationship with the development of T1DN. Furthermore, we identified an additional twenty-four GMs of interest with potential causal links to DN. Among these, eleven were identified as protective factors, while thirteen were associated with increased risk. Our study harnessed high-quality GWAS summary data sourced from the MiBioGen consortium, the largest microbiome GWAS consortium, in conjunction with DN GWAS data from the FinnGen and CKDGen consortia. This robust foundation enabled us to derive accurate and reliable results. Considering the substantial global burden of DN and the emerging significance of GM in health and disease, our findings suggest gaining more profound insights into the complex origins of DN and its interaction with GM could be pivotal in making progress toward early diagnosis and preventative strategies for this patient population (Figure 4 Graphic abstract).
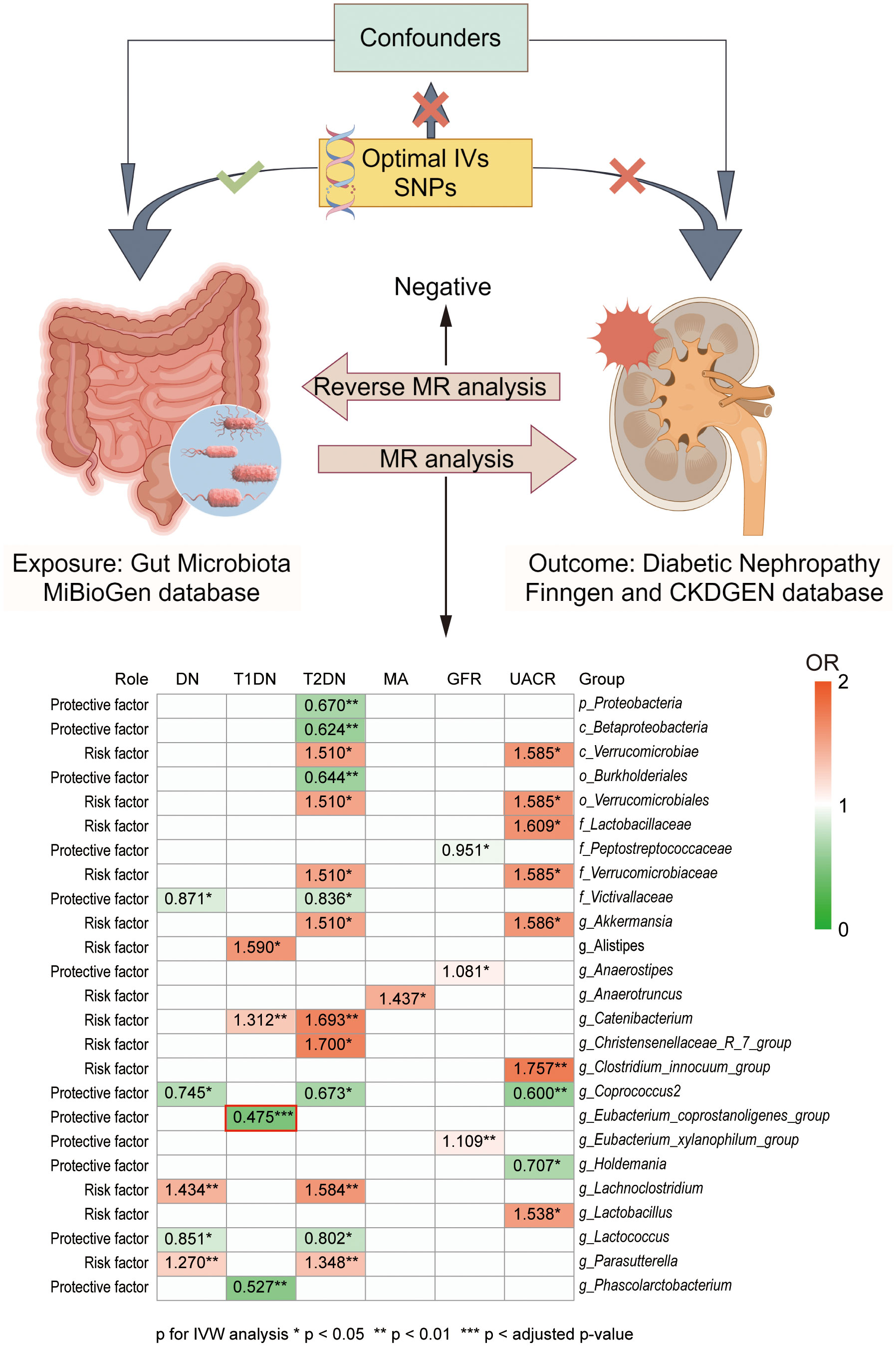
Figure 4 Graphic abstract of present study. Our present study investigated the casual association between GM and DN development at the genetic level. GWAS data of GMs was obtained from the MiBioGen database, while GWAS data of DN were obtained from the FinnGen and CKDGen database. Heat map graph showed the significant causal association between different GM and different outcomes. Red represents an odds ratio (OR) >1, while green represents an OR <1. *p < 0.05, **p < 0.01, ***p < adjusted p-value in inverse variance weighted (IVW) analysis. No significant difference was found in the reverse MR analysis.
In the present study, most of the identified GM causally associated with DN can be categorized into the taxonomic phyla p_Bacteroidetes, p_Firmicutes, p_Proteobacteria and p_Verrucomicrobia. The phyla Bacteroidetes and Firmicutes are the two largest bacterial phyla, accounting for more than 90% of human GM. Shang J et al. investigated the difference in fecal microbial community between patients with (n=180) and without (n=348) DN in China and reported a higher relative abundance of p_Verrucomicrobia but a lower abundance of p_Firmicutes in patients with DN, with no change in p_Bacteroidetes and p_Proteobacteria (20). However, in another study of T2DM patients with chronic kidney disease (CKD), only p_Verrucomicrobia was significantly increased compared to healthy controls, and no significant difference was found in the other three identified bacterial phyla (19). To date, no consensus has been reached regarding how GM changes in DN populations (17, 21). Alterations in metabolic regulation, including short-chain fatty acids (SCFAs), bile acids, TMAO, uremic toxins, lipopolysaccharide (LPS), and hydrogen sulfide, have been considered as the primary mechanisms by which the GM contributes to the progression of DN (3). For example, SCFAs are the special nutrients and energy components of the intestinal epithelium. They have been reported to play important roles in maintaining the integrity of the intestinal mucosa, inhibiting local intestinal inflammation, and improving gastrointestinal peristalsis (37). Current evidence suggests that SCFAs are mainly produced by p_Bacteroidetes and p_Firmicutes, with acetate and propionate mainly produced by p_Bacteroidetes and butyrate mainly produced by p_Firmicutes (38). TMAO and LPS have been recognized as risk factors for kidney disease and DM (39, 40).
The g_Eubacterium_coprostanoligenes_group, which belongs to the p_Firmicutes, plays a crucial role in regulating cholesterol metabolism. To our knowledge, no studies have hitherto investigated the association between the g_Eubacterium_coprostanoligenes_group and DN development. In this respect, it has been reported that the relative abundance of g_Eubacterium_coprostanoligenes_group was significantly decreased in patients with IgA-related nephropathy (41). In rats with kidney yang deficiency syndrome, g_Eubacterium_coprostanoligenes_group showed lower abundance than healthy controls (42). Besides, Banjong D et al. showed a higher relative abundance of g_Eubacterium_coprostanoligenes_group in patients with early-stage CKD of unknown etiology than those without CKD and in those with early-stage CKD with underlying disease (43). Research on diabetes-associated cognitive dysfunction (DACD) has found that the abundance of g_Eubacterium_coprostanoligenes_group was significantly increased in individuals with DACD in the T2DN cohort compared to those without DACD (44). Indeed, evidence on gut dysbiosis obtained from observational studies is not sufficient to clarify the role of g_Eubacterium_coprostanoligenes_group in the development of DN. Our results from MR analysis revealed that g_Eubacterium_coprostanoligenes_group can protect against T1DN from a genetic standpoint, although further research is warranted to better understand the underlying mechanisms.
Given the important role of g_Eubacterium_coprostanoligenes_group in regulating lipid metabolism, we sought to explain its potential mechanisms in relation to g_Eubacterium_coprostanoligenes_group, lipid metabolism, and DN interaction. It has been established that g_Eubacterium_coprostanoligenes_group lowers blood lipid levels by converting cholesterol to coprostanol, a substance that cannot be absorbed by the intestine. This intricate process may involve steps, including oxidation to 5-cholesten-3-one, followed by alkene isomerization to 4-cholesten-3-one, conjugate reduction, and ketone reduction (45). Although the potential of g_Eubacterium_coprostanoligenes_group to reduce blood lipid levels has been observed, their relationship with blood lipid levels remains inconclusive. As Deng M and Wei W showed that g_Eubacterium_coprostanoligenes_group was negatively associated with triglyceride, total cholesterol and low-density lipoprotein cholesterol (LDL-C) (46, 47). However, a positive relationship between the abundance level of g_Eubacterium_coprostanoligenes_group and the levels of triglyceride, total cholesterol and LDL-C was reported by Lei S and Xie B (48, 49). We speculate that elevated blood lipids might induce the enrichment of g_Eubacterium_coprostanoligenes_group. It induces the reduction of cholesterol absorption in the gut induced by g_Eubacterium_coprostanoligenes_group may helps to lower blood lipid levels. Therefore, the relationship between lipid levels and the abundance of g_Eubacterium_coprostanoligenes_group may exhibit heterogeneity at different stages of the disease. Obesity and hyperlipidemia have been implicated as risk factors for DN development (50, 51). The accumulation and metabolic disorders of lipids is widely thought to cause oxidative stress, inflammatory reactions, and mitochondrial dysfunction. Consequently, this leads to the destruction of podocytes and glomerular interstitial fibrosis during the development of DN (52–54). A meta-analysis reported that statins (commonly used drugs to control cholesterol during clinical practice) contribute to the prevention and treatment of DN by mitigating albuminuria and urinary albumin excretion rates (55). Based on these findings, we speculate that the renoprotective effect of the g_Eubacterium_coprostanoligenes_group may be related to its regulatory effect on lipid metabolism.
Besides the lipid-lowering effects mentioned above, the role of g_Eubacterium_coprostanoligenes_group in improving intestinal barrier function and regulating butyric acid should not be overlooked. Bai D et al. reported that the colonization of Eubacterium_coprostanoligenes maintained the integrity of colonic tissue structure and a healthy mucus layer, and alleviated inflammation and clinical symptoms in fast food diet-fed mouse models (56). A breakdown of the intestinal barrier can lead to microbial translocation, which may further result in the development of enterogenic infection, systemic inflammatory reaction, and even multiple organ dysfunction syndromes. Diabetes is a risk factor for infections, increasing the risk of urinary tract infections by 3.2 to 4.9 folds (57). In addition to the immunosuppressive effects of high glucose, insufficient blood supply and chronic neurological-vascular injury, increased intestinal permeability and intestinal dysbiosis have been considered as very important factors (58). When comorbid with chronic kidney disease, patients may have a higher risk of urinary tract infections, which are associated with immunocompromise, advanced age, and urinary tract obstruction (59). As common pathogenic bacteria of urinary tract infections, Gram-negative bacteria, including Escherichia coli, Citrobacter spp. and Enterobacter aerogenes, should be given more attention (60). Pyuria is an independent risk factor for progression of kidney disease and may accelerate progression to ESRD (59, 61). A well-functioning intestinal barrier is crucial for preventing DN by inhibiting microbial translocation and the onset of disease. Butyrate, one of the key molecules of SCFAs, is mainly produced by p_Firmicutes, including g_Eubacterium_coprostanoligenes_group (38). Although the protective mechanisms of butyrate in DN remain largely unclear, most studies suggest that butyrate can prevent DN progression via inhibition of inflammation, oxidative stress, fibrosis and apoptosis (62, 63). Consistently, it was found that supplementation of exogenous butyrate could restore the composition of GM in db/db mice (64). In addition, supplementation of probiotics, Bifidobacterium pseudolongum and Pediococcus acidilactici FZU106, alleviated intestinal barrier function and reduced excessive lipid accumulation in the liver by increasing the abundance of the g_Eubacterium_coprostanoligenes_group (65, 66). Decreasing the abundance of g_Clostridium_innocuum_group, one of the pathogenic GM identified in present study, is another mechanism underlying the therapeutic effect of Pediococcus acidilactici FZU106 (66). Indeed, the g_Eubacterium_coprostanoligenes_group plays a positive role in maintaining human health from multiple perspectives. Overall, more emphasis should be placed on the renoprotective effects of g_Eubacterium_coprostanoligenes_group in future studies.
The identified GM species belong to p_Firmicutes, most of which are producers of SCFAs, exhibited an inconsistent effect on the development of DN. G_Lactococcus, g_Phascolarctobacterium, g_Anaerostipes and g_Coprococcus were identified as protective factors in the present study. Lactococcus is one of the most commonly used probiotic bacteria (67). It has been reported that g_Lactococcus could ferment carbohydrates to produce lactic acid, which could be further converted to butyrate by other GM (68, 69). Lactococcus has long been considered as a favorable vehicle for drug delivery systems. Oral delivery of staphylococcal nuclease by Lactococcus lactis showed a beneficial protective effect on T1DM by inhibiting inflammation and regulating the immune system (70). Lactococcus lactis modified to express human proinsulin and human IL-10 showed an active role in the prevention and treatment of T1DM and has a favorable safety profile (71, 72). Previous studies have shown that g_Phascolarctobacterium and g_Coprococcus are less abundant in DN patients and animal models (73, 74), while g_Anaerostipes exhibited higher abundance in DN patients (18). A previous MR analysis reported the causal association between g_Anaerostipes and decreased risk of developing T2DM (75). G_Coprococcus has a beneficial effect on the prevention of diabetes, which was positively associated with insulin sensitivity but negatively associated with the presence of dysglycaemia (75). It is widely thought that their protective effect on DN may be closely related to the production of SCFAs (76). Paradoxically, g_Catenibacterium, g_Anaerotruncus, g_Christensenellaceae_R_7_group, g_Clostridium_innocuum_group, and g_Lachnoclostridium have been documented as the risk factors for DN, although they also contribute to the production of SCFAs (77, 78). A higher abundance of g_Catenibacterium and g_Anaerotruncus was also found in patients with CKD and end-stage renal disease (ESRD) compared to healthy people (79, 80). In an African American male cohort, higher abundance of g_Catenibacterium was observed in patients with higher glycosylated hemoglobin(A1c) (81). This result suggests that g_Catenibacterium may influence the serum glucose levels. The abundance of g_Anaerotruncus was positively associated with the levels of IL-17, indicating that g_Anaerotruncus may promote inflammation (82). Xh Su et al. found a positive correlation between the abundance of g_Christensenellaceae_R_7_group and the activation of oxidative stress in rats with DN (83). The adverse effects of g_Lachnoclostridium in the development of DN could be explained by dysregulated lipid metabolism. In DN cohorts, g_Lachnoclostridium exhibited a negative association with HbA1c levels, and a positive correlation with total cholesterol (TC) and triglyceride (TG) levels (84). In short, the evidence obtained from simple correlational analyses does not provide a complete and precise depiction of the involvement of the mentioned microbiota in DN. Further study is needed for better understanding.
It has been reported that g_Alistipe belonging to p_Bacteroidetes is associated with an increased risk of DN development. As reported by Chen WH et al., g_Alistipes demonstrated a positive association with 24-hour urine output and a negative correlation with serum albumin in individuals with DN (84). As a detrimental factor for DN, g_Alistipes contributes to the formation of inflammation and epithelial barrier injury (85), which could lead to the development of DN. Some findings of the present study are inconsistent with the prevailing opinion on GM. For instance, while g_Akkermansia has been associated with various beneficial effects in previous studies (86, 87), our research identified it as a risk factor. Conversely, p_Proteobacteria exhibited the opposite relationship in our study compared to prior findings (88). This discrepancy might attribute to the small sample size of our GWAS data, emphasizing the need for more research.
Our study boasts several strengths in its design. Indeed, using MR analysis to investigate the potential causal association between GM and DN can minimize the impact of confounders. The utilization of GWAS data sourced from well-established databases such as MiBioGen, FinnGen R9, and CKDGen enhances the robustness of our MR analysis. In addition, directionally consistent results were required to obtain robust results, and Bonferroni adjusted p-value was used. Although MR analysis helps to eliminate the influence of confounding factors, the results may also be influenced by pleiotropy and heterogeneity. To address this concern, we conducted several sensitivity analyses, encompassing MR-Egger regression, MR-PRESSO, and Cochran’s Q tests, in an effort to mitigate potential pleiotropic and heterogeneity effects.
Nonetheless, some inevitable limitations should be considered in interpreting our findings. Firstly, non-linear correlations cannot be analyzed because we used aggregated GWAS data from public databases rather than raw data. Secondly, our findings were based on data from individuals of European ancestry and cannot be generalized to other ethnicities, such as African and Asian populations, due to the inherent genetic differences between different ethnicities. Thirdly, further longitudinal studies are needed to confirm the causal association though convincing causal associations were found in our present studies. Fourth, we provided a novel idea of the causal associations between GM and DN development but were not able to differentiate the degree of association based on the different stages of DN. The relationship between disease severity and GM is crucial. It is highly conceivable that different degrees of DN may have different effects on GM, and the abundance of GM may also be influenced by different treatments given to patients with different degrees of DN (18). Therefore, the generalizability of the results may be limited. Fifthly, although we used by far the largest GWAS data of GM, consisting with 14,306 samples, the sample size was small. The sample size of the outcome GWAS data from the CKDGen database was not big enough. Future research would benefit from higher-quality GWAS datasets with larger sample sizes to enhance predictive power and reduce potential errors.
Our study carries important implications for further research and clinical practice in the management of DN. Further basic research should focus on unraveling the mechanisms responsible for the regulatory effects of the identified GM on DN development, especially the g_Eubacterium_coprostanoligenes_group. In addition, high-quality longitudinal clinical studies are needed to provide more conclusive causal evidence. In clinical practice, our research underscores the pivotal role of GM as part of a comprehensive approach to early DN diagnosis and treatment. So far, dietary interventions and exposure to probiotics and prebiotics have been proven effective in modulating GM distribution and function. Fecal microbiota transplantation (FMT) stands as an emerging therapeutic method for adjusting GM distribution and improving the intestinal environment, with its beneficial effects and safety well-documented in preclinical studies for diabetes and DN (89, 90). With further clinical research, FMT is poised to become a crucial tool in DN treatment.
5 Conclusion
Our present study provides novel insights into the association between GM and DN. The identified GM may be potential diagnostic biomarkers and therapeutic targets for DN. However, these causal associations and the potential mechanism warrant further investigation in the future. Our efforts may contribute to the development of innovative strategies for the early diagnosis and treatment of DN with emphasis on GM.
Data availability statement
The original contributions presented in the study are included in the article/Supplementary Material. Further inquiries can be directed to the corresponding author.
Author contributions
YF: Writing – original draft, Software, Project administration, Methodology, Formal Analysis, Data curation, Conceptualization. YZ: Writing – original draft, Visualization, Software, Methodology, Formal Analysis, Data curation. QL: Writing – review & editing, Supervision, Funding acquisition. ZZ: Writing – review & editing. CR: Writing – review & editing. XZ: Writing – review & editing, Supervision, Project administration, Funding acquisition, Conceptualization.
Funding
The author(s) declare financial support was received for the research, authorship, and/or publication of this article. Our present work was supported by National Nature Science Foundation of China, grant number, 31371509, 82100244; 2020 Li Ka Shing Foundation Cross-Disciplinary Research Grant, grant number, 2020LKSFG20B; 2022 Characteristics and Innovation Grant for college of Guangdong Province, grant number, 2022KTSCX040.
Acknowledgments
The authors are grateful to the scientists for the provision of high quality GWAS data. We thank home-for-researchers (https://www.home-for-researchers.com/) and Figdraw (https://www.figdraw.com/) platforms for polishing up the manuscript and drawing Graphic abstracts.
Conflict of interest
The authors declare that the research was conducted in the absence of any commercial or financial relationships that could be construed as a potential conflict of interest.
Publisher’s note
All claims expressed in this article are solely those of the authors and do not necessarily represent those of their affiliated organizations, or those of the publisher, the editors and the reviewers. Any product that may be evaluated in this article, or claim that may be made by its manufacturer, is not guaranteed or endorsed by the publisher.
Supplementary material
The Supplementary Material for this article can be found online at: https://www.frontiersin.org/articles/10.3389/fendo.2024.1329954/full#supplementary-material
Abbreviations
CKD, chronic kidney disease; DACD, diabetes-associated cognitive dysfunction; DM, diabetes mellitus; DN, diabetic nephropathy; ESRD, end-stage renal disease; FMT, fecal microbiota transplantation; GFR, glomerular filtration rate; GM, gut microbiota; GWAS, Genome-wide association study; IV, instrumental variable; IVW, inverse variance weighted; LD, linkage disequilibrium; LDL-C, low density lipoprotein cholesterol; LPS, lipopolysaccharide; MA, microalbuminuria; MR, mendelian randomization; SCFAs, short chain fatty acids; SNP, single nucleotide polymorphism; T1DN, type 1 diabetes with diabetic nephropathy; T2DN, type 2 diabetes with diabetic nephropathy; TMAO, trimethylamine-N-oxide; UACR, urine albumin creatine ratio; WM, weighted median method.
References
1. Saeedi P, Petersohn I, Salpea P, Malanda B, Karuranga S, Unwin N, et al. Global and regional diabetes prevalence estimates for 2019 and projections for 2030 and 2045: Results from the International Diabetes Federation Diabetes Atlas, 9th edition. Diabetes Res Clin Pract. (2019) 157:107843. doi: 10.1016/j.diabres.2019.107843
2. Yang D, Livingston MJ, Liu Z, Dong G, Zhang M, Chen JK, et al. Autophagy in diabetic kidney disease: regulation, pathological role and therapeutic potential. Cell Mol Life Sci. (2018) 75:669–88. doi: 10.1007/s00018-017-2639-1
3. Zhao H, Yang CE, Liu T, Zhang MX, Niu Y, Wang M, et al. The roles of gut microbiota and its metabolites in diabetic nephropathy. Front Microbiol. (2023) 14:1207132. doi: 10.3389/fmicb.2023.1207132
4. Sabatino A, Regolisti G, Cosola C, Gesualdo L, Fiaccadori E. Intestinal microbiota in type 2 diabetes and chronic kidney disease. Curr Diabetes Rep. (2017) 17:16. doi: 10.1007/s11892-017-0841-z
5. Zhang X, Shen D, Fang Z, Jie Z, Qiu X, Zhang C, et al. Human gut microbiota changes reveal the progression of glucose intolerance. PLoS One. (2013) 8:e71108. doi: 10.1371/journal.pone.0071108
6. Meijers BK, Evenepoel P. The gut-kidney axis: Indoxyl sulfate, p-cresyl sulfate and CKD progression. Nephrol Dial Transplant. (2011) 26:759–61. doi: 10.1093/ndt/gfq818
7. Lv Q, Li Z, Sui A, Yang X, Han Y, Yao R. The role and mechanisms of gut microbiota in diabetic nephropathy, diabetic retinopathy and cardiovascular diseases. Front Microbiol. (2022) 13:977187. doi: 10.3389/fmicb.2022.977187
8. Sechovcová H, Mahayri TM, Mrázek J, Jarošíková R, Husáková J, Wosková V, et al. Gut microbiota in relationship to diabetes mellitus and its late complications with a focus on diabetic foot syndrome: A review. Folia Microbiol (Praha). (2023). doi: 10.1007/s12223-023-01119-y
9. Gao LW, Yang XY, Yu YF, Yin S, Tong KK, Hu G, et al. Bibliometric analysis of intestinal microbiota in diabetic nephropathy. Eur Rev Med Pharmacol Sci. (2023) 27:8812–28. doi: 10.26355/eurrev_202309_33802
10. Tian E, Wang F, Zhao L, Sun Y, Yang J. The pathogenic role of intestinal flora metabolites in diabetic nephropathy. Front Physiol. (2023) 14:1231621. doi: 10.3389/fphys.2023.1231621
11. Stavropoulou E, Kantartzi K, Tsigalou C, Konstantinidis T, Romanidou G, Voidarou C, et al. Focus on the gut-kidney axis in health and disease. Front Med (Lausanne). (2020) 7:620102. doi: 10.3389/fmed.2020.620102
12. Su X, Yu W, Liu A, Wang C, Li X, Gao J, et al. San-huang-yi-shen capsule ameliorates diabetic nephropathy in rats through modulating the gut microbiota and overall metabolism. Front Pharmacol. (2021) 12:808867. doi: 10.3389/fphar.2021.808867
13. Letchumanan G, Abdullah N, Marlini M, Baharom N, Lawley B, Omar MR, et al. Gut microbiota composition in prediabetes and newly diagnosed type 2 diabetes: A systematic review of observational studies. Front Cell Infect Microbiol. (2022) 12:943427. doi: 10.3389/fcimb.2022.943427
14. Li WZ, Stirling K, Yang JJ, Zhang L. Gut microbiota and diabetes: From correlation to causality and mechanism. World J Diabetes. (2020) 11:293–308. doi: 10.4239/wjd.v11.i7.293
15. Guo Z, Pan J, Zhu H, Chen ZY. Metabolites of gut microbiota and possible implication in development of diabetes mellitus. J Agric Food Chem. (2022) 70:5945–60. doi: 10.1021/acs.jafc.1c07851
16. Cai K, Ma Y, Cai F, Huang X, Xiao L, Zhong C, et al. Changes of gut microbiota in diabetic nephropathy and its effect on the progression of kidney injury. Endocrine. (2022) 76:294–303. doi: 10.1007/s12020-022-03002-1
17. Al-Obaide M, Singh R, Datta P, Rewers-Felkins KA, Salguero MV, Al-Obaidi I, et al. Gut microbiota-dependent trimethylamine-N-oxide and serum biomarkers in patients with T2DM and advanced CKD. J Clin Med. (2017) 6:86. doi: 10.3390/jcm6090086
18. Du X, Liu J, Xue Y, Kong X, Lv C, Li Z, et al. Alteration of gut microbial profile in patients with diabetic nephropathy. Endocrine. (2021) 73:71–84. doi: 10.1007/s12020-021-02721-1
19. Salguero MV, Al-Obaide M, Singh R, Siepmann T, Vasylyeva TL. Dysbiosis of Gram-negative gut microbiota and the associated serum lipopolysaccharide exacerbates inflammation in type 2 diabetic patients with chronic kidney disease. Exp Ther Med. (2019) 18:3461–9. doi: 10.3892/etm.2019.7943
20. Shang J, Cui W, Guo R, Zhang Y, Wang P, Yu W, et al. The harmful intestinal microbial community accumulates during DKD exacerbation and microbiome-metabolome combined validation in a mouse model. Front Endocrinol (Lausanne). (2022) 13:964389. doi: 10.3389/fendo.2022.964389
21. Tao S, Li L, Li L, Liu Y, Ren Q, Shi M, et al. Understanding the gut-kidney axis among biopsy-proven diabetic nephropathy, type 2 diabetes mellitus and healthy controls: an analysis of the gut microbiota composition. Acta Diabetol. (2019) 56:581–92. doi: 10.1007/s00592-019-01316-7
22. Zhang L, Wang Z, Zhang X, Zhao L, Chu J, Li H, et al. Alterations of the gut microbiota in patients with diabetic nephropathy. Microbiol Spectr. (2022) 10:e0032422. doi: 10.1128/spectrum.00324-22
23. Davey Smith G, Hemani G. Mendelian randomization: genetic anchors for causal inference in epidemiological studies. Hum Mol Genet. (2014) 23:R89–98. doi: 10.1093/hmg/ddu328
24. Ji C, Deng Y, Yang A, Lu Z, Chen Y, Liu X, et al. Rhubarb enema improved colon mucosal barrier injury in 5/6 nephrectomy rats may associate with gut microbiota modification. Front Pharmacol. (2020) 11:1092. doi: 10.3389/fphar.2020.01092
25. Didelez V, Sheehan N. Mendelian randomization as an instrumental variable approach to causal inference. Stat Methods Med Res. (2007) 16:309–30. doi: 10.1177/0962280206077743
26. Kurilshikov A, Medina-Gomez C, Bacigalupe R, Radjabzadeh D, Wang J, Demirkan A, et al. Large-scale association analyses identify host factors influencing human gut microbiome composition. Nat Genet. (2021) 53:156–65. doi: 10.1038/s41588-020-00763-1
27. Kurki MI, Karjalainen J, Palta P, Sipilä TP, Kristiansson K, Donner KM, et al. FinnGen provides genetic insights from a well-phenotyped isolated population. Nature. (2023) 613:508–18. doi: 10.1038/s41586-022-05473-8
28. Teumer A, Tin A, Sorice R, Gorski M, Yeo NC, Chu AY, et al. Genome-wide association studies identify genetic loci associated with albuminuria in diabetes. Diabetes. (2016) 65:803–17. doi: 10.2337/db15-1313
29. Pattaro C, Teumer A, Gorski M, Chu AY, Li M, Mijatovic V, et al. Genetic associations at 53 loci highlight cell types and biological pathways relevant for kidney function. Nat Commun. (2016) 7:10023. doi: 10.1038/ncomms10023
30. Sanna S, van Zuydam NR, Mahajan A, Kurilshikov A, Vich Vila A, Võsa U, et al. Causal relationships among the gut microbiome, short-chain fatty acids and metabolic diseases. Nat Genet. (2019) 51:600–5. doi: 10.1038/s41588-019-0350-x
31. Burgess S, Thompson SG, CRP CHD genetics collaboration. Avoiding bias from weak instruments in Mendelian randomization studies. Int J Epidemiol. (2011) 40:755–64. doi: 10.1093/ije/dyr036
32. Burgess S, Butterworth A, Thompson SG. Mendelian randomization analysis with multiple genetic variants using summarized data. Genet Epidemiol. (2013) 37:658–65. doi: 10.1002/gepi.21758
33. Bowden J, Davey Smith G, Haycock PC, Burgess S. Consistent estimation in mendelian randomization with some invalid instruments using a weighted median estimator. Genet Epidemiol. (2016) 40:304–14. doi: 10.1002/gepi.21965
34. Bowden J, Davey Smith G, Burgess S. Mendelian randomization with invalid instruments: effect estimation and bias detection through Egger regression. Int J Epidemiol. (2015) 44:512–25. doi: 10.1093/ije/dyv080
35. Verbanck M, Chen CY, Neale B, Do R. Detection of widespread horizontal pleiotropy in causal relationships inferred from Mendelian randomization between complex traits and diseases. Nat Genet. (2018) 50:693–8. doi: 10.1038/s41588-018-0099-7
36. Zhang L, Zi L, Kuang T, Wang K, Qiu Z, Wu Z, et al. Investigating causal associations among gut microbiota, metabolites, and liver diseases: a Mendelian randomization study. Front Endocrinol (Lausanne). (2023) 14:1159148. doi: 10.3389/fendo.2023.1159148
37. Larsen N, Vogensen FK, van den Berg FW, Nielsen DS, Andreasen AS, Pedersen BK, et al. Gut microbiota in human adults with type 2 diabetes differs from non-diabetic adults. PLoS One. (2010) 5:e9085. doi: 10.1371/journal.pone.0009085
38. Levy M, Thaiss CA, Elinav E. Metabolites: messengers between the microbiota and the immune system. Genes Dev. (2016) 30:1589–97. doi: 10.1101/gad.284091.116
39. Chang YC, Chu YH, Wang CC, Wang CH, Tain YL, Yang HW. Rapid detection of gut microbial metabolite trimethylamine N-oxide for chronic kidney disease prevention. Biosensors (Basel). (2021) 11:339. doi: 10.3390/bios11090339
40. Li SY, Chen S, Lu XT, Fang AP, Chen YM, Huang RZ, et al. Serum trimethylamine-N-oxide is associated with incident type 2 diabetes in middle-aged and older adults: a prospective cohort study. J Transl Med. (2022) 20:374. doi: 10.1186/s12967-022-03581-7
41. Chai L, Luo Q, Cai K, Wang K, Xu B. Reduced fecal short-chain fatty acids levels and the relationship with gut microbiota in IgA nephropathy. BMC Nephrol. (2021) 22:209. doi: 10.1186/s12882-021-02414-x
42. Chen R, Wang J, Zhan R, Zhang L, Wang X. Fecal metabonomics combined with 16S rRNA gene sequencing to analyze the changes of gut microbiota in rats with kidney-yang deficiency syndrome and the intervention effect of You-gui pill. J Ethnopharmacol. (2019) 244:112139. doi: 10.1016/j.jep.2019.112139
43. Banjong D, Pongking T, Tran N, Pinlaor S, Dangtakot R, Intuyod K, et al. Slight changes in the gut microbiome in early-stage chronic kidney disease of unknown etiology. Microbes Environ. (2023) 38:ME22097. doi: 10.1264/jsme2.ME22097
44. Huang H, Zhao T, Li J, Shen J, Xiao R, Ma W. Gut microbiota regulation of inflammatory cytokines and microRNAs in diabetes-associated cognitive dysfunction. Appl Microbiol Biotechnol. (2023) 107:7251–67. doi: 10.1007/s00253-023-12754-3
45. Koppel N, Maini Rekdal V, Balskus EP. Chemical transformation of xenobiotics by the human gut microbiota. Science. (2017) 356:eaag2770. doi: 10.1126/science.aag2770
46. Deng M, Zhang S, Dong L, Huang F, Jia X, Su D, et al. Shatianyu (Citrus grandis L. Osbeck) flavonoids and dietary fiber in combination are more effective than individually in alleviating high-fat-diet-induced hyperlipidemia in mice by altering gut microbiota. J Agric Food Chem. (2022) 70:14654–64. doi: 10.1021/acs.jafc.2c03797
47. Wei W, Jiang W, Tian Z, Wu H, Ning H, Yan G, et al. Fecal g. Streptococcus and g. Eubacterium_coprostanoligenes_group combined with sphingosine to modulate the serum dyslipidemia in high-fat diet mice. Clin Nutr. (2021) 40:4234–45. doi: 10.1016/j.clnu.2021.01.031
48. Lei S, He S, Li X, Zheng B, Zhang Y, Zeng H. Effect of lotus seed resistant starch on small intestinal flora and bile acids in hyperlipidemic rats. Food Chem. (2023) 404:134599. doi: 10.1016/j.foodchem.2022.134599
49. Xie B, Zu X, Wang Z, Xu X, Liu G, Liu R. Ginsenoside Rc ameliorated atherosclerosis via regulating gut microbiota and fecal metabolites. Front Pharmacol. (2022) 13:990476. doi: 10.3389/fphar.2022.990476
50. Dejenie TA, Abebe EC, Mengstie MA, Seid MA, Gebeyehu NA, Adella GA, et al. Dyslipidemia and serum cystatin C levels as biomarker of diabetic nephropathy in patients with type 2 diabetes mellitus. Front Endocrinol (Lausanne). (2023) 14:1124367. doi: 10.3389/fendo.2023.1124367
51. Ou YL, Lee MY, Lin IT, Wen WL, Hsu WH, Chen SC. Obesity-related indices are associated with albuminuria and advanced kidney disease in type 2 diabetes mellitus. Ren Fail. (2021) 43:1250–8. doi: 10.1080/0886022X.2021.1969247
52. Abrass CK. Cellular lipid metabolism and the role of lipids in progressive renal disease. Am J Nephrol. (2004) 24:46–53. doi: 10.1159/000075925
53. Ducasa GM, Mitrofanova A, Fornoni A. Crosstalk between lipids and mitochondria in diabetic kidney disease. Curr Diabetes Rep. (2019) 19:144. doi: 10.1007/s11892-019-1263-x
54. Forbes JM, Cooper ME. Mechanisms of diabetic complications. Physiol Rev. (2013) 93:137–88. doi: 10.1152/physrev.00045.2011
55. Shen X, Zhang Z, Zhang X, Zhao J, Zhou X, Xu Q, et al. Efficacy of statins in patients with diabetic nephropathy: a meta-analysis of randomized controlled trials. Lipids Health Dis. (2016) 15:179. doi: 10.1186/s12944-016-0350-0
56. Bai D, Sun T, Zhao J, Du J, Bu X, Cao W, et al. Oroxylin A maintains the colonic mucus barrier to reduce disease susceptibility by reconstituting a dietary fiber-deprived gut microbiota. Cancer Lett. (2021) 515:73–85. doi: 10.1016/j.canlet.2021.05.018
57. Luk A, Wu H, Lau E, Yang A, So WY, Chow E, et al. Temporal trends in rates of infection-related hospitalisations in Hong Kong people with and without diabetes, 2001-2016: a retrospective study. Diabetologia. (2021) 64:109–18. doi: 10.1007/s00125-020-05286-2
58. Holt R, Cockram CS, Ma R, Luk A. Diabetes and infection: review of the epidemiology, mechanisms and principles of treatment. Diabetologia. (2024). doi: 10.1007/s00125-024-06102-x
59. Kuo IC, Lee JJ, Hwang DY, Lim LM, Lin HY, Hwang SJ, et al. Pyuria, urinary tract infection and renal outcome in patients with chronic kidney disease stage 3-5. Sci Rep. (2020) 10:19460. doi: 10.1038/s41598-020-76520-5
60. Micle O, Antal L, Naghi P, Tica O, Zaha DC, Zdrinca MM, et al. The prevalence of urinary tract infections in pregnancy and implications on foetal development. FARMACIA. (2020) 68:463–9. doi: 10.31925/farmacia.2020.3.11
61. Almaiman L, Allemailem KS, El-Kady AM, Alrasheed M, Almatroudi A, Alekezem FS, et al. Prevalence and significance of pyuria in chronic kidney disease patients in Saudi Arabia. J Pers Med. (2021) 11:831. doi: 10.3390/jpm11090831
62. Dong W, Jia Y, Liu X, Zhang H, Li T, Huang W, et al. Sodium butyrate activates NRF2 to ameliorate diabetic nephropathy possibly via inhibition of HDAC. J Endocrinol. (2017) 232:71–83. doi: 10.1530/JOE-16-0322
63. Du Y, Yang YT, Tang G, Jia JS, Zhu N, Yuan WJ. Butyrate alleviates diabetic kidney disease by mediating the miR-7a-5p/P311/TGF-β1 pathway. FASEB J. (2020) 34:10462–75. doi: 10.1096/fj.202000431R
64. Xu YH, Gao CL, Guo HL, Zhang WQ, Huang W, Tang SS, et al. Sodium butyrate supplementation ameliorates diabetic inflammation in db/db mice. J Endocrinol. (2018) 238:231–44. doi: 10.1530/JOE-18-0137
65. Guo W, Mao B, Cui S, Tang X, Zhang Q, Zhao J, et al. Protective effects of a novel probiotic bifidobacterium pseudolongum on the intestinal barrier of colitis mice via modulating the Pparγ/STAT3 pathway and intestinal microbiota. Foods. (2022) 11:1551. doi: 10.3390/foods11111551
66. Zhang Q, Guo WL, Chen GM, Qian M, Han JZ, Lv XC, et al. Pediococcus acidilactici FZU106 alleviates high-fat diet-induced lipid metabolism disorder in association with the modulation of intestinal microbiota in hyperlipidemic rats. Curr Res Food Sci. (2022) 5:775–88. doi: 10.1016/j.crfs.2022.04.009
67. Li O, Zhang H, Wang W, Liang Y, Chen W, Din AU, et al. Complete genome sequence and probiotic properties of Lactococcus petauri LZys1 isolated from healthy human gut. J Med Microbiol. (2021) 70. doi: 10.1099/jmm.0.001397
68. Burakova I, Smirnova Y, Gryaznova M, Syromyatnikov M, Chizhkov P, Popov E, et al. The effect of short-term consumption of lactic acid bacteria on the gut microbiota in obese people. Nutrients. (2022) 14:3384. doi: 10.3390/nu14163384
69. Duncan SH, Louis P, Flint HJ. Lactate-utilizing bacteria, isolated from human feces, that produce butyrate as a major fermentation product. Appl Environ Microbiol. (2004) 70:5810–7. doi: 10.1128/AEM.70.10.5810-5817.2004
70. Lang J, Wang X, Liu K, He D, Niu P, Cao R, et al. Oral delivery of staphylococcal nuclease by Lactococcus lactis prevents type 1 diabetes mellitus in NOD mice. Appl Microbiol Biotechnol. (2017) 101:7653–62. doi: 10.1007/s00253-017-8480-5
71. Mathieu C, Wiedeman A, Cerosaletti K, Long SA, Serti E, Cooney L, et al. A first-in-human, open-label Phase 1b and a randomised, double-blind Phase 2a clinical trial in recent-onset type 1 diabetes with AG019 as monotherapy and in combination with teplizumab. Diabetologia. (2023) 67:27–41. doi: 10.1007/s00125-023-06014-2
72. Preisser TM, da Cunha VP, Santana MP, Pereira VB, Cara DC, Souza BM, et al. Recombinant Lactococcus lactis Carrying IL-4 and IL-10 Coding Vectors Protects against Type 1 Diabetes in NOD Mice and Attenuates Insulitis in the STZ-Induced Model. J Diabetes Res. (2021) 2021:6697319. doi: 10.1155/2021/6697319
73. Jiang C, Pan X, Luo J, Liu X, Zhang L, Liu Y, et al. Alterations in microbiota and metabolites related to spontaneous diabetes and pre-diabetes in rhesus macaques. Genes (Basel). (2022) 13:1513. doi: 10.3390/genes13091513
74. Sheng Y, Zheng SJ, Zhang CH, Zhao CH, He XY, Xu WT, et al. Mulberry leaf tea alleviates diabetic nephropathy by inhibiting PKC signaling and modulating intestinal flora. J Funct Foods. (2018) 46:118. doi: 10.1016/j.jff.2018.04.040
75. Yang Q, Lin SL, Kwok MK, Leung GM, Schooling CM. The roles of 27 genera of human gut microbiota in ischemic heart disease, type 2 diabetes mellitus, and their risk factors: A mendelian randomization study. Am J Epidemiol. (2018) 187:1916–22. doi: 10.1093/aje/kwy096
76. Koh A, De Vadder F, Kovatcheva-Datchary P, Bäckhed F. From dietary fiber to host physiology: Short-chain fatty acids as key bacterial metabolites. Cell. (2016) 165:1332–45. doi: 10.1016/j.cell.2016.05.041
77. Kageyama A, Benno Y. Catenibacterium mitsuokai gen. nov., sp. nov., a gram-positive anaerobic bacterium isolated from human faeces. Int J Syst Evol Microbiol. (2000) 50 Pt 4:1595–9. doi: 10.1099/00207713-50-4-1595
78. Lawson PA, Song Y, Liu C, Molitoris DR, Vaisanen ML, Collins MD, et al. Anaerotruncus colihominis gen. nov., sp. nov., from human faeces. Int J Syst Evol Microbiol. (2004) 54:413–7. doi: 10.1099/ijs.0.02653-0
79. Margiotta E, Miragoli F, Callegari ML, Vettoretti S, Caldiroli L, Meneghini M, et al. Gut microbiota composition and frailty in elderly patients with Chronic Kidney Disease. PLoS One. (2020) 15:e0228530. doi: 10.1371/journal.pone.0228530
80. Vaziri ND, Wong J, Pahl M, Piceno YM, Yuan J, DeSantis TZ, et al. Chronic kidney disease alters intestinal microbial flora. Kidney Int. (2013) 83:308–15. doi: 10.1038/ki.2012.345
81. Ciubotaru I, Green SJ, Kukreja S, Barengolts E. Significant differences in fecal microbiota are associated with various stages of glucose tolerance in African American male veterans. Transl Res. (2015) 166:401–11. doi: 10.1016/j.trsl.2015.06.015
82. Schirmer M, Smeekens SP, Vlamakis H, Jaeger M, Oosting M, Franzosa EA, et al. Linking the human gut microbiome to inflammatory cytokine production capacity. Cell. (2016) 167:1125–36.e8. doi: 10.1016/j.cell.2016.10.020
83. Wang P, Guo R, Bai X, Cui W, Zhang Y, Li H, et al. Sacubitril/Valsartan contributes to improving the diabetic kidney disease and regulating the gut microbiota in mice. Front Endocrinol (Lausanne). (2022) 13:1034818. doi: 10.3389/fendo.2022.1034818
84. Chen W, Zhang M, Guo Y, Wang Z, Liu Q, Yan R, et al. The profile and function of gut microbiota in diabetic nephropathy. Diabetes Metab Syndr Obes. (2021) 14:4283–96. doi: 10.2147/DMSO.S320169
85. Parker BJ, Wearsch PA, Veloo A, Rodriguez-Palacios A. The genus alistipes: Gut bacteria with emerging implications to inflammation, cancer, and mental health. Front Immunol. (2020) 11:906. doi: 10.3389/fimmu.2020.00906
86. Luo L, Luo J, Cai Y, Fu M, Li W, Shi L, et al. Inulin-type fructans change the gut microbiota and prevent the development of diabetic nephropathy. Pharmacol Res. (2022) 183:106367. doi: 10.1016/j.phrs.2022.106367
87. Shin NR, Lee JC, Lee HY, Kim MS, Whon TW, Lee MS, et al. An increase in the Akkermansia spp. population induced by metformin treatment improves glucose homeostasis in diet-induced obese mice. Gut. (2014) 63:727–35. doi: 10.1136/gutjnl-2012-303839
88. Shah NB, Allegretti AS, Nigwekar SU, Kalim S, Zhao S, Lelouvier B, et al. Blood microbiome profile in CKD : A pilot study. Clin J Am Soc Nephrol. (2019) 14:692–701. doi: 10.2215/CJN.12161018
89. Bastos R, Simplício-Filho A, Sávio-Silva C, Oliveira L, Cruz G, Sousa EH, et al. Fecal microbiota transplant in a pre-clinical model of type 2 diabetes mellitus, obesity and diabetic kidney disease. Int J Mol Sci. (2022) 23:3842. doi: 10.3390/ijms23073842
Keywords: causal inference, intestinal flora, diabetic kidney disease, Mendelian randomization, MiBioGen, eubacterium
Citation: Fang Y, Zhang Y, Liu Q, Zheng Z, Ren C and Zhang X (2024) Assessing the causal relationship between gut microbiota and diabetic nephropathy: insights from two-sample Mendelian randomization. Front. Endocrinol. 15:1329954. doi: 10.3389/fendo.2024.1329954
Received: 31 October 2023; Accepted: 04 March 2024;
Published: 18 March 2024.
Edited by:
George Grant, University of Aberdeen, United KingdomReviewed by:
Jonathan Samuel Chávez-Iñiguez, University of Guadalajara, MexicoCosmin Mihai Vesa, University of Oradea, Romania
Copyright © 2024 Fang, Zhang, Liu, Zheng, Ren and Zhang. This is an open-access article distributed under the terms of the Creative Commons Attribution License (CC BY). The use, distribution or reproduction in other forums is permitted, provided the original author(s) and the copyright owner(s) are credited and that the original publication in this journal is cited, in accordance with accepted academic practice. No use, distribution or reproduction is permitted which does not comply with these terms.
*Correspondence: Xin Zhang, walterzhangx@139.com