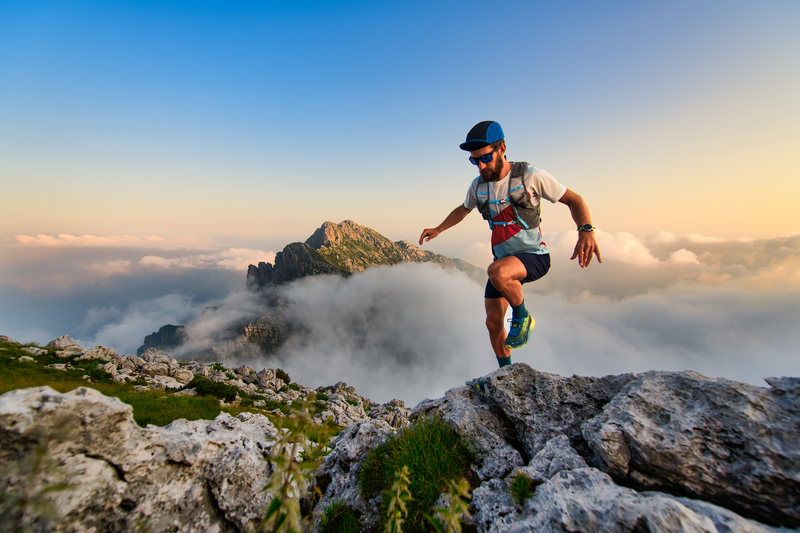
94% of researchers rate our articles as excellent or good
Learn more about the work of our research integrity team to safeguard the quality of each article we publish.
Find out more
ORIGINAL RESEARCH article
Front. Endocrinol. , 21 March 2024
Sec. Cardiovascular Endocrinology
Volume 15 - 2024 | https://doi.org/10.3389/fendo.2024.1302296
Background: Cardiometabolic multimorbidity (CMM) has emerged as a prominent public health concern. Hypertensive patients are prone to develop comorbidities. Moreover, the accumulation of visceral adipose tissue is the main cause for the development of cardiometabolic diseases. The cardiometabolic index (CMI), lipid accumulation product (LAP), visceral adiposity index (VAI), and Chinese visceral adiposity index (CVAI) not only assess adipose tissue mass but also reflect adipose tissue dysfunction. So far, no study has been reported to evaluate the association of CMI, LAP, VAI, and CVAI with CMM risk in hypertensive patients. Therefore, this study aimed to assess the association between these adiposity indicators and the risk of CMM among Chinese hypertensive patients.
Methods: In this cross-sectional study, a total of 229,287 hypertensive patients aged 35 years and older were included from the National Basic Public Health Service Project. All participants underwent a face-to-face questionnaire survey, physical examination, and the collection of fasting venous blood samples. Multivariable logistic regression models were performed to estimate odds ratios (ORs) and 95% confidence intervals (CIs). Receiver operating characteristic curve was utilized to evaluate the identification ability for CMM.
Results: After adjusting for confounders, each 1-standard deviation increase in CMI, LAP, VAI, and CVAI was associated with a 14%, 8%, 12%, and 54% increased risk of CMM, respectively. When comparing the highest quartile of these indicators with the lowest quartile, individuals in the highest quartile of CMM, LAP, VAI, and CVAI had a 1.39-fold (95% CI 1.30, 1.48), 1.28-fold (95% CI 1.19, 1.37), 1.37-fold (95% CI 1.29, 1.46), and 2.56-fold (95% CI 2.34, 2.79) increased risk of CMM after adjusting for potential confounders. Notably, a nonlinear association was observed for CMI, LAP, and VAI with the risk of CMM (all P nonlinearity < 0.001). CVAI exhibited the highest area under the receiver operating characteristic curve (AUC) among all the included adiposity indices in this analysis.
Conclusion: This study indicated the significant positive association of CMI, LAP, VAI, and CVAI with the risk of CMM in hypertensive patients. Among these indicators, CVAI demonstrated the most robust performance in predicting CMM risk and may serve as a valuable tool for identifying CMM risk in Chinese hypertensive patients.
Cardiometabolic multimorbidity (CMM), characterized by the simultaneous presence of at least two cardiometabolic conditions such as hypertension, diabetes, coronary heart disease, and stroke, represents a prevalent and severe form of multimorbidity (1, 2). The prevalence of CMM has been on a rapid rise, largely driven by the increasing proportion of aging individuals in the global population (3, 4). Studies conducted on a global scale, within the United Kingdom, and among Chinese populations have consistently demonstrated that CMM is associated with decreased life expectancy and an elevated risk of all-cause mortality (2, 5, 6). Given its high prevalence and adverse outcomes, CMM has emerged as a prominent concern in the field of public health. Furthermore, hypertension, which is the most prevalent chronic condition worldwide, is highly predisposed to the development of comorbidities (7). Approximately one quarter of hypertensive patients go on to develop CMM (8). Research has indicated that in hypertensive patients, the risk of all-cause mortality substantially rises, increasing from 7% to 30% when combined with diabetes and reaching 136% in the presence of cardiovascular disease (6). As a result, evaluating the risk of CMM in the hypertensive population holds paramount importance.
Mounting evidence suggests the accumulation of visceral adipose tissue as the primary cause for the development of cardiometabolic disorders (9). Nowadays, imaging techniques like magnetic resonance imaging and computed tomography offer quantitative assessments of visceral adipose tissue and fat distribution (10). However, these techniques are inappropriate for routine medical screenings due to their time-consuming nature, high costs, and potential radiation risks. Therefore, there is a demand for a simple and cost-effective predictor to identify hypertensive patients at high risk of CMM.
In recent years, there has been the development of several novel adiposity indices, namely the cardiometabolic index (CMI), lipid accumulation product (LAP), visceral adiposity index (VAI), and Chinese visceral adiposity index (CVAI). These indices not only measure adipose tissue mass but also provide insights into adipose tissue dysfunction (11–13). In 2015, Wakabayashi et al. proposed the concept of CMI, which incorporates waist-to-height ratio (WHtR), triglyceride (TG), and high-density lipoprotein cholesterol ratio (HDL-C) as a novel index to evaluate both the distribution and dysfunction of visceral adipose tissue (11, 14). LAP, based on a combination of waist circumference (WC) and TG levels, was proposed as a more accurate continuous index for describing lipid overaccumulation associated with central obesity and metabolic risk (13, 15). VAI, comprising WC, body mass index (BMI), TG, and HDL-C, allows for the measurement of abdominal fat distribution and has shown significant associations with cardiovascular and cerebrovascular events, as well as all factors of metabolic syndrome (12, 16). CVAI, a simple clinical index consisting of age, BMI, WC, TG, and HDL-C, has been considered as a surrogate biomarker for assessing visceral fat accumulation (17).
Previous studies have examined the association of CMI, LAP, VAI, and CVAI with the risk of individual cardiovascular disease in the general population, including conditions like hypertension, diabetes, and stroke (18–22). To the best of our knowledge, there has been no prior study that has specifically evaluated the association of CMI, LAP, VAI, and CVAI with CMM, particularly among individuals with hypertension. To address this research gap, the primary objective of this study was to evaluate how CMI, LAP, VAI and CVAI are associated with the risk of CMM in hypertensive populations. We hypothesize that higher levels of CMI, LAP, VAI, and CVAI are associated with a higher risk of CMM. The findings from our study are expected to provide valuable insights into the roles of CMI, LAP, VAI, and CVAI in CMM risk, potentially informing targeted strategies for the prevention of cardiometabolic diseases.
This study is based on the National Basic Public Health Service Project (NBPHS), which represents a core healthcare service provided by the government, free of charge, to all residents. Its primary objective is to cater to the primary health requirements of both urban and rural residents, with a specific focus on children, pregnant women, the elderly, and individuals with chronic health conditions (23).
A total of 289,015 individuals who were recipients of the NBPHS in Guangzhou in the year 2020 were enrolled in this study. Inclusion criteria were as follows: individuals aged 35 years or older, Guangdong natives or residents with a minimum of six months’ residency in Guangzhou, individuals diagnosed with primary hypertension, and those who had undergone a physical examination. Hypertension was defined as having a resting blood pressure of ≥ 140/90 mmHg, current use of antihypertensive drugs, or self-reported hypertension (24). To assess this, participants received three separate blood pressure measurements on different days. A diagnosis of hypertension was confirmed if all three readings showed a blood pressure of ≥140/90 mmHg. Exclusions were made for participants who lacked information on ethnicity or education (n=2335); those without measurements of height, weight, WC, or blood pressure (n=430); individuals with missing data related to lifestyle and lipids levels (n=12,713); and those with outliers in blood pressure, height, weight, WC, and lipid measurements (n=44,250). Consequently, a total of 229,287 participants were included in the final analysis (Figure 1).
This study received approval from the Ethical Review Committee for School of Public Health, Sun Yat-sen University (approval number: 2023-007). The study was performed in accordance with the principles outlined in the Declaration of Helsinki.
All participants underwent face-to-face interviews conducted by trained healthcare staff at community-level healthcare facilities using a standardized questionnaire. The collected information included socio-demographic characteristics (age, sex, ethnicity, educational level, and marital status) as well as lifestyle factors (dietary habits, physical activity, smoking habits, and alcohol drinking). Dietary habits were categorized as either healthy or unhealthy. Healthy dietary habits were characterized by a balanced intake of meat and vegetable, moderate consumption of salt, oil, and added sugar, while unhealthy dietary habits were identified as a preference for meat or a vegetarian diet, along with high consumption of salt, oil, or added sugar (25). Active physical activity was defined as engaging in at least 150 minutes per week of moderate activity or at least 75 minutes per week of vigorous activity (or a comparable combination of both) (26). Smoking status was divided into two groups: never smokers and smokers (comprising both former and current smokers). Similarly, drinking status was classified into never drinkers and drinkers (including both ever and current drinkers). Individuals were classified as drinkers if they consumed 10 grams or more of alcohol per day (27).
Standardized methods were used to measure the height, weight, WC, and blood pressure of the participants. BMI was calculated by dividing body weight in kilograms by the square of height in meters. WHtR was calculated as the ratio of WC to height in meters. systolic blood pressure (SBP) and diastolic blood pressure (DBP) were measured while participants were in a seated position, with readings taken from both arms. The mean value of the two measurements obtained from the left and right arms was considered as the individual’s blood pressure readings.
Fasting venous blood sample was collected, and the serum concentrations of total cholesterol (TC), TG, HDL-C, and low-density lipoprotein cholesterol (LDL-C) were measured using an automatic biochemical analyzer.
In our study, we evaluated three adiposity variables, namely CMI, LAP, VAI, and CVAI, through indirect calculations using relevant anthropometric parameters. TG/HDL-C was computed as the ratio of TG to HDL-C. CMI was derived as TG/HDL-C multiplied by WHtR (11). LAP was a sex-specific index calculated as follows: for males, LAP = [WC (cm)-65] × TG (mmol/L), and for females, LAP = [WC (cm)-58] × TG (mmol/L) (13). The formulas for VAI calculation were as follows: for males, VAI=WC (cm)/[39.68 + 1.88 × BMI (kg/m2)] × TG (mmol/L)/1.03 × 1.31/HDL-C (mmol/L); for females, VAI=WC (cm)/[(36.58 + 1.89 × BMI (kg/m2)] × TG (mmol/L)/0.81 × 1.52/HDL-C (mmol/L) (12). CVAI was computed using sex-specific formulas as follows: for males, CVAI= −267.93 + 0.68 × age (years) +0.03 × BMI (kg/m2) +4.00 × WC (cm) + 22.00 × log10 [TG (mmol/L)] −16.32 × HDL-C (mmol/L); and for females, CVAI= −187.32 + 1.71 × age (years) + 4.32 × BMI (kg/m2) + 1.12 × WC (cm) + 39.76 × log10 [TG (mmol/L)] − 11.66 × HDL-C (mmol/L) (22).
We collected participants’ medical histories using a questionnaire, and these histories were confirmed based on self-reports of physician diagnoses. CMM is commonly defined as the presence of two or more concurrent cardiometabolic diseases. For this study, we defined CMM as the occurrence of at least one of the following cardiometabolic diseases in conjunction with hypertension: diabetes, coronary heart disease, or stroke (1, 28).
Participants were categorized according to their CMM status. The normality of continuous variables was assessed using the Kolmogorov–Smirnov test. Normally distributed continuous variables were presented as mean and standard deviation (SD), while skewed continuous variables were described using the median and interquartile range (IQR). Categorical variables were presented as frequencies and percentages. Differences across the various CMM status groups were assessed using the chi-square test for categorical variables and either t-test or Wilcoxon rank-sum test for continuous variables. Outliers were excluded if they fell below the first quartile (Q1-1.5) or above the third quartile (Q3 + 1.5) (29, 30). Supplementary Table S1 provides a comparison of the characteristics between the excluded participants and those retained in the study.
We explored the association of CMI, LAP, VAI, and CVAI as continuous variables and categorized into quartiles with the CMM. Multivariable logistic regression models were applied to evaluate the impact of CMI, LAP, VAI, and CVAI on CMM risk, and the associations were expressed as odds ratios (ORs) and 95% confidence intervals (CIs). Potential confounders were selected based on a comparison of factors between the non-CMM and CMM groups, taking into consideration previous relevant studies. The following potential confounding variables were included in the multivariable model: age, sex, ethnicity, education level, marital status, BMI, dietary habits, physical activity, smoking, alcohol consumption, SBP, and DBP. Additionally, we utilized restricted cubic spline (RCS) regression based on multivariable adjusted logistics regression to examine the potential nonlinear relationship. Receiver operating characteristic (ROC) curves were used to calculate the area under the ROC curve (AUC) to assess and compare the discriminatory performance of each adiposity indicators, including CMI, LAP, VAI, and CVAI, in identifying the risk of CMM.
Stratified analysis was conducted by age group (<65 or ≥65 years), sex (males or females), BMI (<24 or ≥24 kg/m2), dietary habits (unhealthy or healthy), physical activity (non-active or active), smoking (yes or no), alcohol consumption (yes or no), SBP (<140 or ≥140 mmHg), and DBP (<90 or ≥90 mmHg). To assess potential interactive effects, interaction terms were included in the multiple regression model. Sensitivity analyses were conducted to assess robustness of our findings. We examined the association between adiposity indicators and the risk of CMM without excluding outliers. Furthermore, to account for potential residual confounding factors, we additionally adjusted for TC and LDL-C in the analytical models.
All analyses were performed using R software (4.3.0). A two-sided P-value less than 0.05 was considered significant.
A total of 229,287 participants with hypertension were included in the present study, of which 220,020 (95.96%) participants were classified into the non-CMM group and 9267 (4.04%) into the CMM group. The distribution of CMM is shown in Supplementary Table S2. Among all participants, the median (IQR) age was 69.41 (10.65) years, with 92,126 (40.18%) being male and 137,161 (59.82%) being female. Comparing the non-CMM group to the CMM group, it was observed that more participants in the former were younger, female, had a higher level of education, were married, exhibited healthy dietary habits, engaged in less physical activity, were non-smokers but drinkers, had higher SBP, DBP, TC, LDL-C, and HDL-C levels, and had lower BMI, CMI, LAP, VAI, and CVAI levels. There were no significant differences in terms of ethnicity, and TG level (P > 0.05) (Table 1).
The risk of CMM exhibited a positive association with increasing levels of CMI, LAP, VAI, and CVAI (Table 2). After considering possible confounders, each 1- standard deviation increase in CMI, LAP, VAI, and CVAI was associated with a 14% (OR 1.14, 95% CI 1.12, 1.17), 8% (OR 1.08, 95% CI 1.06, 1.11), 12% (OR 1.12, 95% CI 1.10, 1.15), and 54% (OR 1.54, 95% CI 1.49, 1.59) increased risk of CMM, respectively. When comparing individuals in the highest quartile of CMI, LAP, VAI, and CVAI with those in the lowest quartile, the former group had a 1.39-fold (95% CI 1.30, 1.48, P trend < 0.001), 1.28-fold (95% CI 1.19, 1.37, P trend < 0.001), 1.37-fold (95% CI 1.29, 1.46, P trend < 0.001), and 2.56-fold (95% CI 2.34, 2.79, P trend < 0.001) increased risk of CMM after adjusting for potential confounders. Figure 2 illustrates the associations of CMI, LAP, and VAI with CMM risk using RCS curves with four knots, revealing significant nonlinear associations (all P nonlinearity < 0.001). Nevertheless, no nonlinear association was observed between CVAI and CMM (P nonlinearity = 0.176). CMM risk exhibited a rapid increase with rising levels of CMI, LAP, and VAI until approximately 1, 40, and 5, respectively, after which the increment slowed down.
Figure 2 Restricted cubic spline of the relationship of cardiometabolic multimorbidity with CMI (A), LAP (B), VAI (C), and CVAI (D). Potential nonlinear relationships were examined using restricted cubic splines, with odd ratios (ORs) based on logistic regression models. The ORs was adjusted for age, sex, ethnicity, education level, marital status, body mass index, dietary habits, physical activity, smoking, alcohol drinking, systolic blood pressure, and diastolic blood pressure. CMI, cardiometabolic index; LAP, lipid accumulation product; VAI, visceral adiposity index; CVAI, Chinese visceral adiposity index.
The ROC curves and corresponding AUCs for CMI, LAP, VAI, and CVAI in identifying CMM are shown in Table 3 and Figure 3. The adjusted AUCs (95% CIs) were 0.612 (0.607, 0.618), 0.608 (0.602, 0.613), 0.612 (0.607, 0.618), and 0.634 (0.628, 0.639), respectively. Notably, CVAI exhibited the highest AUC among all the adiposity indices included in this analysis.
Table 3 Predictive performance of adiposity indicators for cardiometabolic multimorbidity in hypertension patients.
Figure 3 Receiver operating characteristic curves for adiposity indicators for predicting cardiometabolic multimorbidity among hypertension participants. CMI, cardiometabolic index; LAP, lipid accumulation product; VAI, visceral adiposity index; CVAI, Chinese visceral adiposity index.
In the stratified analysis, the positive associations of CMI, LAP, VAI, and CVAI with CMM risk remained statistically significant in all subgroups, except for the dietary habits and alcohol drinking subgroup (Table 4). Notably, the association between CMI and CMM risk appeared to be more pronounced in individuals with a BMI < 24 kg/m2 (P interaction < 0.05). Similarly, the association between LAP and CMM risk was stronger in subjects with BMI < 24 kg/m2 and SBP ≥ 140mmHg (both P interaction < 0.05). Additionally, the association between VAI and CMM risk exhibited greater significance in individuals aged ≥ 65 years and those with a BMI < 24 kg/m2 (all P interaction < 0.05). The association between CVAI and CMM risk appeared to be more pronounced in females, individuals with unhealthy dietary habits, and those were non-active physical activity (all P interaction < 0.05). No significant interactions were found between other factors and CMI, LAP, VAI, or CVAI.
Table 4 Stratified analyses for the association between adiposity indicators and cardiometabolic multimorbidity stratified by different factors.
In the sensitivity analysis, we observed consistent findings regarding the association between adiposity indicators and the risk of CMM when outliers were not excluded (Supplementary Table S3). Furthermore, additional adjustment for TC and LDL-C had minimal impact on the results (Supplementary Table S4).
This is the first study to investigate the association of CMI, LAP, VAI, and CVAI with the risk of CMM in Chinese hypertensive patients. Our findings demonstrated that CMI, LAP, VAI, and CVAI were positively and independently associated with CMM among individuals with hypertension, even after adjusting for other confounding factors. Additionally, it was observed that the levels of CMI, LAP, and VAI displayed a nonlinear relationship with CMM. Furthermore, CVAI demonstrated the highest predictive for CMM compared to CMI, LAP, and VAI.
CMI offers the advantage of applicability in assessing various conditions such as type 2 diabetes mellitus, stroke, and atherosclerotic progression (11, 31, 32). It has been found to be associated with an increased risk of diabetes in both Chinese and Japanese adult populations (11, 33). A large cross-sectional study conducted in rural China showed that for each 1 standard deviation increment in CMI, the risk of ischemic stroke increased by 18% for females and 14% for males (31). Moreover, Wakabayashi et al. reported a correlation between CMI and the severity of atherosclerosis in individuals with peripheral arterial disease (32). However, no prior studies have explored the impact of CMI on CMM risk. The present study was initiated to examine the utility of CMI in identifying individuals with CMM among hypertensive patients. Our findings strongly suggest that higher CMI values are closely associated with CMM.
LAP has emerged as a promising anthropometric index for assessing cardiometabolic risk (31, 34). Evidence showed that LAP serves as a predictor of diabetes risk in both Japanese and Chinese populations, outperforming traditional obesity indicators (35, 36). A population-based prospective cohort study conducted in China revealed that individuals in the high LAP group had a 1.67 times higher risk of stroke compared to those in the low LAP group (37). Furthermore, LAP has been reported to exhibit a positive association with the incidence of cardiovascular events and all-cause mortality (38, 39). In line with these findings, the present study identified a positive association between LAP and the risk of CMM in hypertensive patients.
VAI has been linked to the development of various cardiometabolic diseases, atherosclerosis, and stroke (12, 16, 40). A meta-analysis, including 9 cohort studies, found that for each 1-unit increase in VAI, there was a 42% higher risk of type 2 diabetes mellitus (41). The National Health and Nutrition Examination Survey, including 29,337 adults, showed that VAI was associated with a 12% increase in the prevalence of stroke (16). Han et al. revealed a strong association between VAI and the severity of coronary heart disease in Chinese adults (42). Furthermore, findings from the UK Biobank cohort study showed a positive relationship between VAI and the increased risk of all-cause mortality as well as cause-specific mortalities (43). In alignment with these findings, our study revealed that each 1- standard deviation increase in VAI was associated with a 12% increased risk of CMM.
CVAI is considered to be a reliable indicator of visceral adipose scores specific to the Chinese population, and it has been used to assess various diseases, including diabetes, stroke, and cardiovascular events (22, 44, 45). Studies have shown that CVAI is associated with an increased risk of diabetes in both Chinese and Japanese adult populations (22, 46). The China Health and Retirement Longitudinal Study, including 7242 middle-aged and elderly residents, showed that CVAI was associated with a 17% increased risk of incident stroke (44). Two cohort studies conducted in Xinjiang and Tangshan showed that CVAI was associated with an elevated risk of cardiovascular events in patients with hypertension and type 2 diabetes (45, 47). In line with these findings, the present study identified a positive association between CVAI and the risk of CMM in hypertensive patients. Furthermore, we discovered that CVAI exhibited the highest predictive capacity for CMM compared to CMI, LAP, and VAI. In line with our results, the Rural Chinese Cohort Study found that CVAI was the most accurate predictor of stroke (20). Additionally, a cross-sectional study including 2514 Chinese women aged between 45 and 55 years showed that CVAI outperformed five other obesity indicators as a predictor of metabolic dysfunction-associated fatty liver disease (48). Moreover, data from the China Nutrition and Health Surveillance showed that CVAI was the most robust predictor of hypertension in both men and women, surpassing other obesity indices (49). These findings suggest that CVAI may serve as a valuable and easily measurable indicator for identifying individuals at high risk of CMM.
The RCS analysis suggested nonlinear associations of CMI, LAP, and VAI with the risk of CMM. At the initial stage of increasing CMI, LAP, and VAI, the risk of CMM increased rapidly, but the rate of increase subsequently slowed down. A cross-sectional study conducted in Japan demonstrated a nonlinear dose-response association between CMI and the risk of non-alcoholic fatty liver disease (50). Furthermore, RCS results from a community-based prospective cohort in Lishui, China observed that elevated LAP level was associated with a higher atherosclerotic burden in coronary arteries (51). Similarly, the National Health and Nutrition Examination Survey identified a significant nonlinear association between VAI and all-cause mortality (43). However, our finding did not observe a nonlinear association between CVAI and CMM. Similarly, a cohort study found no nonlinear relationship between CVAI and the risk of diabetes mellitus in non-obese Japanese adults (52). Additionally, the China Health and Retirement Longitudinal Study found no evidence of a nonlinear link between CVAI and the incidence of stroke (44). Overall, the findings from various dose-response analyses across different studies have consistently indicated that higher levels of CMI, LAP, and VAI are linked to an increased diseases burden and mortality, including hypertension. We believe that further investigation into the mechanisms underlying this nonlinear dose-response relationship between visceral obesity and CMM is warranted in future research.
Although the exact mechanisms underlying the association between these adiposity indicators and CMM among hypertensive patients are unclear, several hypotheses have been proposed. First, obesity may lead to elevated levels of inflammatory cytokines such as tumor necrosis factor-α, interleukin-6, and C-reactive protein (53). These inflammatory cytokines play a crucial role in the development and progression of metabolic regulation disorders and atherosclerotic plaques on blood vessel walls, which could ultimately contribute to CMM (54, 55). Second, previous evidence has demonstrated that obesity can lead to endothelial dysfunction, further contributing to insulin resistance (56, 57). Insulin resistance results in increased basal lipolysis in adipose tissue, the releasing free fatty acids into circulation (58). Excess free fatty acids are generated outside of fat storage tissue and transported to ectopic locations such as the viscera organs, the heart, and vasculature, eventually contributing to the development of CMM (59). Third, abdominal fat has been associated with elevated cortisol levels due to the possible hyperactivity of the hypothalamic-pituitary-adrenocortical axis and local adipocyte cortisol production (60). Long-term exposure to high level of cortisol may disrupt glucose homeostasis, induce insulin resistance, elevate blood pressure, and increase triglyceride levels, ultimately leading to the development of CMM (61, 62). Finally, individuals with obesity often adopt unhealthy lifestyles, including physical inactivity, excessive calorie intake, smoking, and excessive alcohol consumption, all of which are recognized risk factors for CMM (63, 64). Our study demonstrated a positive association of CMI, LAP, VAI, and CVAI with CMM, suggesting that these adiposity indicators could potentially serve as simple and effective markers for assessing CMM risk. However, further mechanistic studies are needed to elucidate the specific roles of these indicators in the development of CMM among hypertensive patients.
This study possesses several strengths that deserve recognition. Firstly, it is the first investigation to examine the associations of adiposity indicators, including CMI, LAP, VAI, and CVAI, with the risk of CMM, especially among a substantial large group of hypertensive individuals. Secondly, utilizing a large sample of Chinese hypertensive adults, uncommon in similar investigations, our study enhances the statistical power and validity. Additionally, the analytical approach, treating CMI, LAP, VAI, and CVAI as both continuous and categorical variables, alongside conducting sensitivity analysis and trend tests, enhances the robustness and reliability of our results. Furthermore, the consistency of our primary findings across subgroups underscores the stability of our results. However, there are also several limitations to consider in this study. Firstly, a large proportion of participants were excluded due to outliers, potentially impacting the generalizability of our findings. Nevertheless, when including outliers in the analysis, we obtained results consistent with the main findings, reinforcing the robustness of our conclusions. Secondly, the identification of CMM relied on self-reported information obtained through interviews, a common procedure in substantial epidemiological investigations (65, 66). This method may introduce recall bias, potentially leading to an underestimation of CMM prevalence. Nevertheless, the significant associations found between the CMI, LAP, VAI, CVAI, and CMM emphasize the importance of our observations, despite the acknowledged limitations. Thirdly, the definition of a healthy dietary habits relied solely on self-reported data. Therefore, our definition of a healthy dietary habits was limited to our study population and there may be restrictions to extrapolation of results. Fourthly, the cross-sectional nature of this study limits our ability to establish causal relationships between abdominal obesity indices and CMM in hypertensive individuals. However, it serves as a preliminary step towards further longitudinal studies. Consequently, there is a pressing need for future longitudinal studies to further investigate the role of CMI, LAP, VAI, and CVAI in the development of CMM. Fifthly, despite our efforts to adjust for potential risk factors in multivariable analyses, there remains the possibility of residual or unassessed confounding variables influencing our results. Lastly, acknowledging the diverse factors, including economic, cultural, dietary differences and genetic diversity, it needs for caution when extrapolating our data and results to other populations.
This study is the first to investigate the association of CMI, LAP, VAI, and CVAI with the risk of CMM among Chinese hypertensive patients. This cross-sectional study has revealed significant association of CMI, LAP, VAI, and CVAI with CMM in Chinese adults diagnosed with hypertension. Notably, among these obesity indicators, CVAI demonstrated superior performance in predicting CMM risk. The findings from this study suggest that CVAI might serve as a valuable tool for identifying the risk of CMM in Chinese hypertensive patients. The global prevalence of CMM remains high, underlining the importance of bolstering disease management for hypertensive patients through lifestyle interventions and effective treatment aimed at preventing visceral obesity and, consequently, reducing the incidence of CMM. Additionally, the utility and validity of CMI, LAP, VAI, and CVAI as reliable indicators for the prevention of new-onset CMM warrant further investigation and exploration.
The raw data supporting the conclusions of this article will be made available by the authors, without undue reservation.
The studies involving humans were approved by the ethical committee of the School of Public Health of Sun Yat-Sen University. The studies were conducted in accordance with the local legislation and institutional requirements. The ethics committee/institutional review board waived the requirement of written informed consent for participation from the participants or the participants’ legal guardians/next of kin because Informed consent did not apply due to the use of secondary data.
TD: Conceptualization, Formal analysis, Writing – original draft. WL: Conceptualization, Data curation, Formal analysis, Funding acquisition, Writing – original draft. QZ: Data curation, Funding acquisition, Writing – original draft. YY: Data curation, Writing – original draft. XL: Data curation, Writing – original draft. JC: Data curation, Writing – original draft. HL: Conceptualization, Data curation, Funding acquisition, Writing – review & editing. CZ: Conceptualization, Formal analysis, Writing – review & editing.
The author(s) declare financial support was received for the research, authorship, and/or publication of this article. This study was supported by the National Natural Science Foundation of China (No.72104061); Science and Technology Plan Project of Guangzhou (No.202201010022); The Key Project of Medicine Discipline of Guangzhou (No.2021-2023-12); Basic Research Project of Key Laboratory of Guangzhou (No.202102100001), Basic and Applied Research Project of Guangzhou (No.SL2022A03J01446).
We gratefully acknowledge the participation and contribution of the participants; without them, this study would not have been possible.
The authors declare that the research was conducted in the absence of any commercial or financial relationships that could be construed as a potential conflict of interest.
All claims expressed in this article are solely those of the authors and do not necessarily represent those of their affiliated organizations, or those of the publisher, the editors and the reviewers. Any product that may be evaluated in this article, or claim that may be made by its manufacturer, is not guaranteed or endorsed by the publisher.
The Supplementary Material for this article can be found online at: https://www.frontiersin.org/articles/10.3389/fendo.2024.1302296/full#supplementary-material
1. Xie H, Li J, Zhu X, Li J, Yin J, Ma T, et al. Association between healthy lifestyle and the occurrence of cardiometabolic multimorbidity in hypertensive patients: a prospective cohort study of UK Biobank. Cardiovasc Diabetol. (2022) 21:199. doi: 10.1186/s12933-022-01632-3
2. Di Angelantonio E, Kaptoge S, Wormser D, Willeit P, Butterworth AS, Bansal N, et al. Association of cardiometabolic multimorbidity with mortality. JAMA. (2015) 314:52–60. doi: 10.1001/jama.2015.7008
3. Fan J, Sun Z, Yu C, Guo Y, Pei P, Yang L, et al. Multimorbidity patterns and association with mortality in 0.5 million Chinese adults. Chin Med J (Engl). (2022) 135:648–57. doi: 10.1097/cm9.0000000000001985
4. Smith SM, Soubhi H, Fortin M, Hudon C, O'Dowd T. Managing patients with multimorbidity: systematic review of interventions in primary care and community settings. BMJ. (2012) 345:e5205. doi: 10.1136/bmj.e5205
5. Canoy D, Tran J, Zottoli M, Ramakrishnan R, Hassaine A, Rao S, et al. Association between cardiometabolic disease multimorbidity and all-cause mortality in 2 million women and men registered in UK general practices. BMC Med. (2021) 19:258. doi: 10.1186/s12916-021-02126-x
6. Zhang D, Tang X, Shen P, Si Y, Liu X, Xu Z, et al. Multimorbidity of cardiometabolic diseases: prevalence and risk for mortality from one million Chinese adults in a longitudinal cohort study. BMJ Open. (2019) 9:e024476. doi: 10.1136/bmjopen-2018-024476
7. Lawes CM, Vander Hoorn S, Law MR, Elliott P, MacMahon S, Rodgers A. Blood pressure and the global burden of disease 2000. Part II: estimates of attributable burden. J Hypertens. (2006) 24:423–30. doi: 10.1097/01.hjh.0000209973.67746.f0
8. Feng L, Jehan I, de Silva HA, Naheed A, Farazdaq H, Hirani S, et al. Prevalence and correlates of cardiometabolic multimorbidity among hypertensive individuals: a cross-sectional study in rural South Asia-Bangladesh, Pakistan and Sri Lanka. BMJ Open. (2019) 9:e030584. doi: 10.1136/bmjopen-2019-030584
9. Neeland IJ, Ross R, Després JP, Matsuzawa Y, Yamashita S, Shai I, et al. Visceral and ectopic fat, atherosclerosis, and cardiometabolic disease: a position statement. Lancet Diabetes Endocrinol. (2019) 7:715–25. doi: 10.1016/s2213-8587(19)30084-1
10. Cheung AS, de Rooy C, Hoermann R, Gianatti EJ, Hamilton EJ, Roff G, et al. Correlation of visceral adipose tissue measured by Lunar Prodigy dual X-ray absorptiometry with MRI and CT in older men. Int J Obes. (2016) 40:1325–8. doi: 10.1038/ijo.2016.50
11. Wakabayashi I, Daimon T. The "cardiometabolic index" as a new marker determined by adiposity and blood lipids for discrimination of diabetes mellitus. Clin Chim Acta. (2015) 438:274–8. doi: 10.1016/j.cca.2014.08.042
12. Amato MC, Giordano C, Galia M, Criscimanna A, Vitabile S, Midiri M, et al. Visceral Adiposity Index: a reliable indicator of visceral fat function associated with cardiometabolic risk. Diabetes Care. (2010) 33:920–2. doi: 10.2337/dc09-1825
13. Kahn HS. The "lipid accumulation product" performs better than the body mass index for recognizing cardiovascular risk: a population-based comparison. BMC Cardiovasc Disord. (2005) 5:26. doi: 10.1186/1471-2261-5-26
14. Liu X, Wu Q, Yan G, Duan J, Chen Z, Yang P, et al. Cardiometabolic index: a new tool for screening the metabolically obese normal weight phenotype. J Endocrinol Invest. (2021) 44:1253–61. doi: 10.1007/s40618-020-01417-z
15. Kahn HS, Valdez R. Metabolic risks identified by the combination of enlarged waist and elevated triacylglycerol concentration. Am J Clin Nutr. (2003) 78:928–34. doi: 10.1093/ajcn/78.5.928
16. Chen Q, Zhang Z, Luo N, Qi Y. Elevated visceral adiposity index is associated with increased stroke prevalence and earlier age at first stroke onset: Based on a national cross-sectional study. Front Endocrinol (Lausanne). (2022) 13:1086936. doi: 10.3389/fendo.2022.1086936
17. Xia MF, Chen Y, Lin HD, Ma H, Li XM, Aleteng Q, et al. A indicator of visceral adipose dysfunction to evaluate metabolic health in adult Chinese. Sci Rep. (2016) 6:38214. doi: 10.1038/srep38214
18. Shi WR, Wang HY, Chen S, Guo XF, Li Z, Sun YX. Estimate of prevalent diabetes from cardiometabolic index in general Chinese population: a community-based study. Lipids Health Dis. (2018) 17:236. doi: 10.1186/s12944-018-0886-2
19. Li FE, Luo Y, Zhang FL, Zhang P, Liu D, Ta S, et al. Association between cardiometabolic index and stroke: A population- based cross-sectional study. Curr Neurovasc Res. (2021) 18:324–32. doi: 10.2174/1567202618666211013123557
20. Zhao Y, Zhang J, Chen C, Qin P, Zhang M, Shi X, et al. Comparison of six surrogate insulin resistance indexes for predicting the risk of incident stroke: The Rural Chinese Cohort Study. Diabetes Metab Res Rev. (2022) 38:e3567. doi: 10.1002/dmrr.3567
21. Lee WC, Wu PY, Huang JC, Tsai YC, Chiu YW, Chen SC, et al. Sex difference in the associations among obesity-related indices with incident hypertension in a large Taiwanese population follow-up study. J Pers Med. (2022) 12:972. doi: 10.3390/jpm12060972
22. Xia MF, Lin HD, Chen LY, Wu L, Ma H, Li Q, et al. Association of visceral adiposity and its longitudinal increase with the risk of diabetes in Chinese adults: A prospective cohort study. Diabetes Metab Res Rev. (2018) 34:e3048. doi: 10.1002/dmrr.3048
23. Wang Z, Ao Q, Luo Y, Wang Q, Lu Z, Liu JA. Estimating the costs of the national basic public health services in Zhuhai, China, through activity-based costing: a cross-sectional study. BMJ Open. (2019) 9:e024831. doi: 10.1136/bmjopen-2018-024831
24. Cao Y, Sathish T, Haregu T, Wen Y, de Mello GT, Kapoor N, et al. Factors associated with hypertension awareness, treatment, and control among adults in kerala, India. Front Public Health. (2021) 9:753070. doi: 10.3389/fpubh.2021.753070
25. Che M, Zhou Q, Lin W, Yang Y, Sun M, Liu X, et al. Healthy lifestyle score and glycemic control in type 2 diabetes mellitus patients: A city-wide survey in China. Healthcare (Basel). (2023) 11:2037. doi: 10.3390/healthcare11142037
26. Xiong S, Hou N, Tang F, Li J, Deng H. Association of cardiometabolic multimorbidity and adherence to a healthy lifestyle with incident dementia: a large prospective cohort study. Diabetol Metab Syndr. (2023) 15:208. doi: 10.1186/s13098-023-01186-8
27. Chen Q, Peng L, Xiong G, Peng Y, Luo D, Zou L, et al. Recurrence is a noticeable cause of rifampicin-resistant mycobacterium tuberculosis in the elderly population in jiangxi, China. Front Public Health. (2019) 7:182. doi: 10.3389/fpubh.2019.00182
28. Yang L, Luo Y, He L, Yin J, Li T, Liu S, et al. Shift work and the risk of cardiometabolic multimorbidity among patients with hypertension: A prospective cohort study of UK biobank. J Am Heart Assoc. (2022) 11:e025936. doi: 10.1161/jaha.122.025936
29. Haileslasie H, Tsegaye A, Teklehaymanot G, Belay G, Gebremariam G, Gebremichail G, et al. Community based hematological reference intervals among apparently healthy adolescents aged 12-17 years in Mekelle city, Tigrai, northern Ethiopia: A cross sectional study. PloS One. (2020) 15:e0234106. doi: 10.1371/journal.pone.0234106
30. Reddy IA, Smith NK, Erreger K, Ghose D, Saunders C, Foster DJ, et al. Bile diversion, a bariatric surgery, and bile acid signaling reduce central cocaine reward. PloS Biol. (2018) 16:e2006682. doi: 10.1371/journal.pbio.2006682
31. Wang H, Chen Y, Guo X, Chang Y, Sun Y. Usefulness of cardiometabolic index for the estimation of ischemic stroke risk among general population in rural China. Postgrad Med. (2017) 129:834–41. doi: 10.1080/00325481.2017.1375714
32. Wakabayashi I, Sotoda Y, Hirooka S, Orita H. Association between cardiometabolic index and atherosclerotic progression in patients with peripheral arterial disease. Clin Chim Acta. (2015) 446:231–6. doi: 10.1016/j.cca.2015.04.020
33. Wang Z, He S, Chen X. Capacity of different anthropometric measures to predict diabetes in a Chinese population in southwest China: a 15-year prospective study. Diabetes Med. (2019) 36:1261–7. doi: 10.1111/dme.14055
34. Khanmohammadi S, Tavolinejad H, Aminorroaya A, Rezaie Y, Ashraf H, Vasheghani-Farahani A. Association of lipid accumulation product with type 2 diabetes mellitus, hypertension, and mortality: a systematic review and meta-analysis. J Diabetes Metab Disord. (2022) 21:1943–73. doi: 10.1007/s40200-022-01114-z
35. Liu T, Lu W, Zhao X, Yao T, Song B, Fan H, et al. Relationship between lipid accumulation product and new-onset diabetes in the Japanese population: a retrospective cohort study. Front Endocrinol (Lausanne). (2023) 14:1181941. doi: 10.3389/fendo.2023.1181941
36. Lin CY, Li JB, Wu F, Wang JJ, An HH, Qiu HN, et al. Comparison of lipid accumulation product and visceral adiposity index with traditional obesity indices in early-onset type 2 diabetes prediction: a cross-sectional study. Diabetol Metab Syndr. (2023) 15:111. doi: 10.1186/s13098-023-01056-3
37. Zhong C, Xia W, Zhong X, Xu T, Li H, Zhang M, et al. Lipid accumulation product and hypertension related to stroke: a 9.2-year prospective study among Mongolians in China. J Atheroscler Thromb. (2016) 23:830–8. doi: 10.5551/jat.33514
38. Wehr E, Pilz S, Boehm BO, März W, Obermayer-Pietsch B. The lipid accumulation product is associated with increased mortality in normal weight postmenopausal women. Obes (Silver Spring). (2011) 19:1873–80. doi: 10.1038/oby.2011.42
39. Ioachimescu AG, Brennan DM, Hoar BM, Hoogwerf BJ. The lipid accumulation product and all-cause mortality in patients at high cardiovascular risk: a PreCIS database study. Obes (Silver Spring). (2010) 18:1836–44. doi: 10.1038/oby.2009.453
40. Qian X, Keerman M, Zhang X, Guo H, He J, Maimaitijiang R, et al. Study on the prediction model of atherosclerotic cardiovascular disease in the rural Xinjiang population based on survival analysis. BMC Public Health. (2023) 23:1041. doi: 10.1186/s12889-023-15630-x
41. Jayedi A, Soltani S, Motlagh SZ, Emadi A, Shahinfar H, Moosavi H, et al. Anthropometric and adiposity indicators and risk of type 2 diabetes: systematic review and dose-response meta-analysis of cohort studies. BMJ. (2022) 376:e067516. doi: 10.1136/bmj-2021-067516
42. Han L, Fu KL, Zhao J, Wang ZH, Tang MX, Wang J, et al. Visceral adiposity index score indicated the severity of coronary heart disease in Chinese adults. Diabetol Metab Syndr. (2014) 6:143. doi: 10.1186/1758-5996-6-143
43. He Q, Liu S, Feng Z, Li T, Chu J, Hu W, et al. Association between the visceral adiposity index and risks of all-cause and cause-specific mortalities in a large cohort: Findings from the UK biobank. Nutr Metab Cardiovasc Dis. (2022) 32:2204–15. doi: 10.1016/j.numecd.2022.05.020
44. Zhang Z, Zhao L, Lu Y, Meng X, Zhou X. Association between Chinese visceral adiposity index and risk of stroke incidence in middle-aged and elderly Chinese population: evidence from a large national cohort study. J Transl Med. (2023) 21:518. doi: 10.1186/s12967-023-04309-x
45. Qiao T, Luo T, Pei H, Yimingniyazi B, Aili D, Aimudula A, et al. Association between abdominal obesity indices and risk of cardiovascular events in Chinese populations with type 2 diabetes: a prospective cohort study. Cardiovasc Diabetol. (2022) 21:225. doi: 10.1186/s12933-022-01670-x
46. Shang L, Li R, Zhao Y, Sun H, Tang B, Hou Y. Association between chinese visceral adiposity index and incident type 2 diabetes mellitus in Japanese adults. Diabetes Metab Syndr Obes. (2021) 14:3743–51. doi: 10.2147/dmso.S322935
47. Wu Y, Xu W, Guo L, Li W, Zhang L, Gao L, et al. Association of the time course of Chinese visceral adiposity index accumulation with cardiovascular events in patients with hypertension. Lipids Health Dis. (2023) 22:90. doi: 10.1186/s12944-023-01852-w
48. Lu Y, Ge L, Yang H, He Y, Wang Y. Chinese visceral adipose index shows superior diagnostic performance in predicting the risk of metabolic dysfunction associated fatty liver disease in early postmenopausal Chinese women. Diabetes Metab Syndr Obes. (2023) 16:607–17. doi: 10.2147/dmso.S402814
49. Li Y, Yu D, Yang Y, Cheng X, Piao W, Guo Q, et al. Comparison of several adiposity indexes in predicting hypertension among chinese adults: data from China nutrition and health surveillance (2015-2017). Nutrients. (2023) 15:2146. doi: 10.3390/nu15092146
50. Zou J, Xiong H, Zhang H, Hu C, Lu S, Zou Y. Association between the cardiometabolic index and non-alcoholic fatty liver disease: insights from a general population. BMC Gastroenterol. (2022) 22:20. doi: 10.1186/s12876-022-02099-y
51. Sun J, Meng X, Huang H, Jing J, Pan Y, Mei L, et al. Higher visceral adiposity index and lipid accumulation product in relation to increased risk of atherosclerotic burden in community-dwelling older adults. Exp Gerontol. (2023) 174:112115. doi: 10.1016/j.exger.2023.112115
52. Lin M, Xie Y, Hong L, Wu S, Deng X, Chen Y, et al. Abnormal adiposity indices are associated with an increased risk of diabetes in a non-obese Asian population. Public Health. (2024) 226:144–51. doi: 10.1016/j.puhe.2023.11.012
53. Park HS, Park JY, Yu R. Relationship of obesity and visceral adiposity with serum concentrations of CRP, TNF-alpha and IL-6. Diabetes Res Clin Pract. (2005) 69:29–35. doi: 10.1016/j.diabres.2004.11.007
54. Van Gaal LF, Mertens IL, De Block CE. Mechanisms linking obesity with cardiovascular disease. Nature. (2006) 444:875–80. doi: 10.1038/nature05487
55. Friedman E, Shorey C. Inflammation in multimorbidity and disability: An integrative review. Health Psychol. (2019) 38:791–801. doi: 10.1037/hea0000749
56. Pinkney JH, Stehouwer CD, Coppack SW, Yudkin JS. Endothelial dysfunction: cause of the insulin resistance syndrome. Diabetes. (1997) 46 Suppl 2:S9–13. doi: 10.2337/diab.46.2.S9
57. de Jongh RT, Serné EH, IJzerman RG, de Vries G, Stehouwer CD. Impaired microvascular function in obesity: implications for obesity-associated microangiopathy, hypertension, and insulin resistance. Circulation. (2004) 109:2529–35. doi: 10.1161/01.CIR.0000129772.26647.6F
58. Arner P, Langin D. Lipolysis in lipid turnover, cancer cachexia, and obesity-induced insulin resistance. Trends Endocrinol Metab. (2014) 25:255–62. doi: 10.1016/j.tem.2014.03.002
59. Britton KA, Fox CS. Ectopic fat depots and cardiovascular disease. Circulation. (2011) 124:e837–41. doi: 10.1161/circulationaha.111.077602
60. Incollingo Rodriguez AC, Epel ES, White ML, Standen EC, Seckl JR, Tomiyama AJ. Hypothalamic-pituitary-adrenal axis dysregulation and cortisol activity in obesity: A systematic review. Psychoneuroendocrinology. (2015) 62:301–18. doi: 10.1016/j.psyneuen.2015.08.014
61. Kyrou I, Chrousos GP, Tsigos C. Stress, visceral obesity, and metabolic complications. Ann N Y Acad Sci. (2006) 1083:77–110. doi: 10.1196/annals.1367.008
62. Rosmond R, Björntorp P. The hypothalamic-pituitary-adrenal axis activity as a predictor of cardiovascular disease, type 2 diabetes and stroke. J Intern Med. (2000) 247:188–97. doi: 10.1046/j.1365-2796.2000.00603.x
63. Chudasama YV, Khunti K, Gillies CL, Dhalwani NN, Davies MJ, Yates T, et al. Healthy lifestyle and life expectancy in people with multimorbidity in the UK Biobank: A longitudinal cohort study. PloS Med. (2020) 17:e1003332. doi: 10.1371/journal.pmed.1003332
64. Zheng Y, Zhou Z, Wu T, Zhong K, Hu H, Zhang H, et al. Association between composite lifestyle factors and cardiometabolic multimorbidity in Chongqing, China: A cross-sectional exploratory study in people over 45 years and older. Front Public Health. (2023) 11:1118628. doi: 10.3389/fpubh.2023.1118628
65. Al-Hazzaa HM, Alhussain MH, Alhowikan AM, Obeid OA. Insufficient sleep duration and its association with breakfast intake, overweight/obesity, socio-demographics and selected lifestyle behaviors among Saudi school children. Nat Sci Sleep. (2019) 11:253–63. doi: 10.2147/nss.S225883
Keywords: cardiometabolic multimorbidity, cardiometabolic index, lipid accumulation product, visceral adiposity index, Chinese visceral adiposity index, hypertension
Citation: Dong T, Lin W, Zhou Q, Yang Y, Liu X, Chen J, Liu H and Zhang C (2024) Association of adiposity indicators with cardiometabolic multimorbidity risk in hypertensive patients: a large cross-sectional study. Front. Endocrinol. 15:1302296. doi: 10.3389/fendo.2024.1302296
Received: 26 September 2023; Accepted: 08 March 2024;
Published: 21 March 2024.
Edited by:
Basil Nwaneri Okeahialam, University of Jos, NigeriaReviewed by:
Aleksandra Zdrojowy-Wełna, Wroclaw Medical University, PolandCopyright © 2024 Dong, Lin, Zhou, Yang, Liu, Chen, Liu and Zhang. This is an open-access article distributed under the terms of the Creative Commons Attribution License (CC BY). The use, distribution or reproduction in other forums is permitted, provided the original author(s) and the copyright owner(s) are credited and that the original publication in this journal is cited, in accordance with accepted academic practice. No use, distribution or reproduction is permitted which does not comply with these terms.
*Correspondence: Hui Liu, Z3pjZGNfbGl1aEBnei5nb3YuY24=; Caixia Zhang, emhhbmdjeDNAbWFpbC5zeXN1LmVkdS5jbg==
†These authors have contributed equally to this work
Disclaimer: All claims expressed in this article are solely those of the authors and do not necessarily represent those of their affiliated organizations, or those of the publisher, the editors and the reviewers. Any product that may be evaluated in this article or claim that may be made by its manufacturer is not guaranteed or endorsed by the publisher.
Research integrity at Frontiers
Learn more about the work of our research integrity team to safeguard the quality of each article we publish.