- 1Department of Gynecology, Longhua Hospital, Shanghai University of Traditional Chinese Medicine, Shanghai, China
- 2Central Laboratory for Research, Longhua Hospital, Shanghai University of Traditional Chinese Medicine, Shanghai, China
Background: To the best of our knowledge, numerous observational studies have linked pregnancy complications to increased risks of diabetes and cardiovascular disease (CVD), causal evidence remains lacking. Our aim was to estimate the association of adverse pregnancy outcomes with diabetes and cardiovascular diseases.
Methods: A two-sample Mendelian randomization (MR) analysis was employed, which is not subject to potential reverse causality. Data for pregnancy complications were obtained from the FinnGen consortium. For primary analysis, outcome data on diabetes, related traits, stroke, and coronary heart disease (CHD) were extracted from the GWAS Catalog, MAGIC, MEGASTROKE, and CARDIoGRAMplusC4D consortium. The MAGIC and UKB consortium datasets were used for replication and meta-analysis. Causal effects were appraised using inverse variance weighted (IVW), weighted median (WM), and MR-Egger. Sensitivity analyses were implemented with Cochran’s Q test, MR-Egger intercept test, MR-PRESSO, leave-one-out (LOO) analysis and the funnel plot.
Results: Genetically predicted gestational diabetes mellitus (GDM) was causally associated with an increased diabetes risk (OR=1.01, 95% CI=1–1.01, P<0.0001), yet correlated with lower 2-hour post-challenge glucose levels (OR=0.89, 95% CI=0.82–0.97, P=0.006). Genetic liability for pregnancy with abortive outcomes indicated decreased fasting insulin levels (OR=0.97, 95% CI=0.95–0.99, P=0.02), but potentially elevated glycated hemoglobin levels (OR=1.02, 95% CI=1.01–1.04, P=0.01). Additionally, hypertensive disorders in pregnancy was tentatively linked to increased risks of stroke (OR=1.11, 95% CI=1.04–1.18, P=0.002) and CHD (OR=1.3, 95% CI=1.2–1.4, P=3.11E-11). Gestational hypertension might have a potential causal association with CHD (OR=1.11, 95% CI=1.01–1.22, P=0.04). No causal associations were observed between preterm birth and diabetes, stroke, or CHD.
Conclusion: The findings of this study provide genetic evidence that gestational diabetes, pregnancy with abortive outcomes, and hypertensive disorders in pregnancy may serve as early indicators for metabolic and cardiovascular risks. These insights are pivotal for the development of targeted screening and preventive strategies.
Introduction
Gestational diabetes mellitus (GDM), hypertensive disorders in pregnancy (HDPs), miscarriage, and preterm birth are prevalent complications during pregnancy. GDM affects approximately 16.7% of live births (1), and HDPs occurs in 2–8% of pregnancies worldwide, posing a significant risk for maternal and perinatal mortality (2). Additionally, the prevalence of miscarriage and preterm labor is 10.8% and 5–18%, respectively (3, 4). Consequently, pregnancy complications exert a considerable burden on global maternal and neonatal health.
While existing research has primarily focused on the short-term consequences of pregnancy complications, including etiology, mechanisms, treatments, and the health outcomes for offspring (5), emerging evidence suggests that pregnancy complications may also have long-term effects on women’s health. Specifically, numerous studies have linked pregnancy complications to a higher risk of developing diabetes (6–8), cardiovascular disease (CVD) (9, 10), and even premature death (11, 12). Diabetes and CVD account for over 70% of global deaths (13). Furthermore, CVD is a leading cause of mortality among women. In 2019, ischemic heart disease and stroke were identified as the major contributors to CVD-related deaths in women (14). Recent findings from the Nurses’ Health Study II have shown that women with a history of spontaneous abortion face a significantly higher risk of premature death, particularly from cardiovascular causes (12). This finding is corroborated by several other studies (15–18).
Despite these associations, there is still a paucity of comprehensive and systematic research appraising the causal relationship between pregnancy complications (abortion, preterm delivery, GDM, HDPs), and the subsequent onset of diabetes and cardiovascular disease. Observational studies are inherent with defects unable to exclude, including potential reverse causality and confounding factors, leading to biased results (19). Furthermore, conducting randomized controlled trials (RCTs) on this subject is both unethical and impractical due to the required resource commitments and long follow-up periods. As such, mendelian randomization (MR) offers a viable alternative for investigating such causal relationships (20). This method utilizes exposure-related genetic variants as instrumental variables (IVs) to explore associations between exposures (e.g., pregnancy complications) and outcomes (e.g., diabetes and CVD) (21). The random assortment of genetic variants at conception prior to disease onset could efficiently minimize the influence of confounding factors, thus providing a more reliable causal inference (22).
Given the above considerations, this study employed genome-wide association study (GWAS) statistics in a two-sample MR framework to rigorously assess the potential causal associations between pregnancy complications and both glycometabolic and cardiovascular disease. Publicly available data from six databases and five sets of diabetes-related traits instruments were used in this study. Additionally, spontaneous delivery cohort served as negative control, replication analysis, and meta-analysis were used to enhance the robustness of MR estimates. Our aim is to provide not only evidence for identification of women at higher risk of diabetes and cardiovascular disease, but also actionable insights for postpartum monitoring and clinical intervention.
Methods
This Mendelian Randomization (MR) analysis utilized published GWAS summary statistics. The study adheres to the Strengthening the Reporting of Observational Studies in Epidemiology Using Mendelian Randomization (STROBE-MR) guidelines (23).
Study design
We employed a two-sample MR approach to systematically investigate the causal relationship between pregnancy complications and the risk of diabetes and cardiovascular diseases. A convincing MR design complies with three fundamental assumptions (1): genetic instruments are strongly correlated with exposures (2); these instruments are not associated with confounders (3); the instruments affect the outcome only through the exposure (24). The latter two assumptions are known collectively as independence from horizontal pleiotropy and can be tested through various statistical methods (25). Genetic data for diabetes and cardiovascular diseases were obtained from five independent GWAS consortia for both primary and replication analyses, and then meta-analysis was performed. The study overview is presented in Figure 1. All statistical analyses were preformed using the TwoSampleMR package (Version 0.5.7), MRPRESSO (Version 1.0) in R (Version 4.2.2), and Reviewer Manager software (Version 5.3.3).
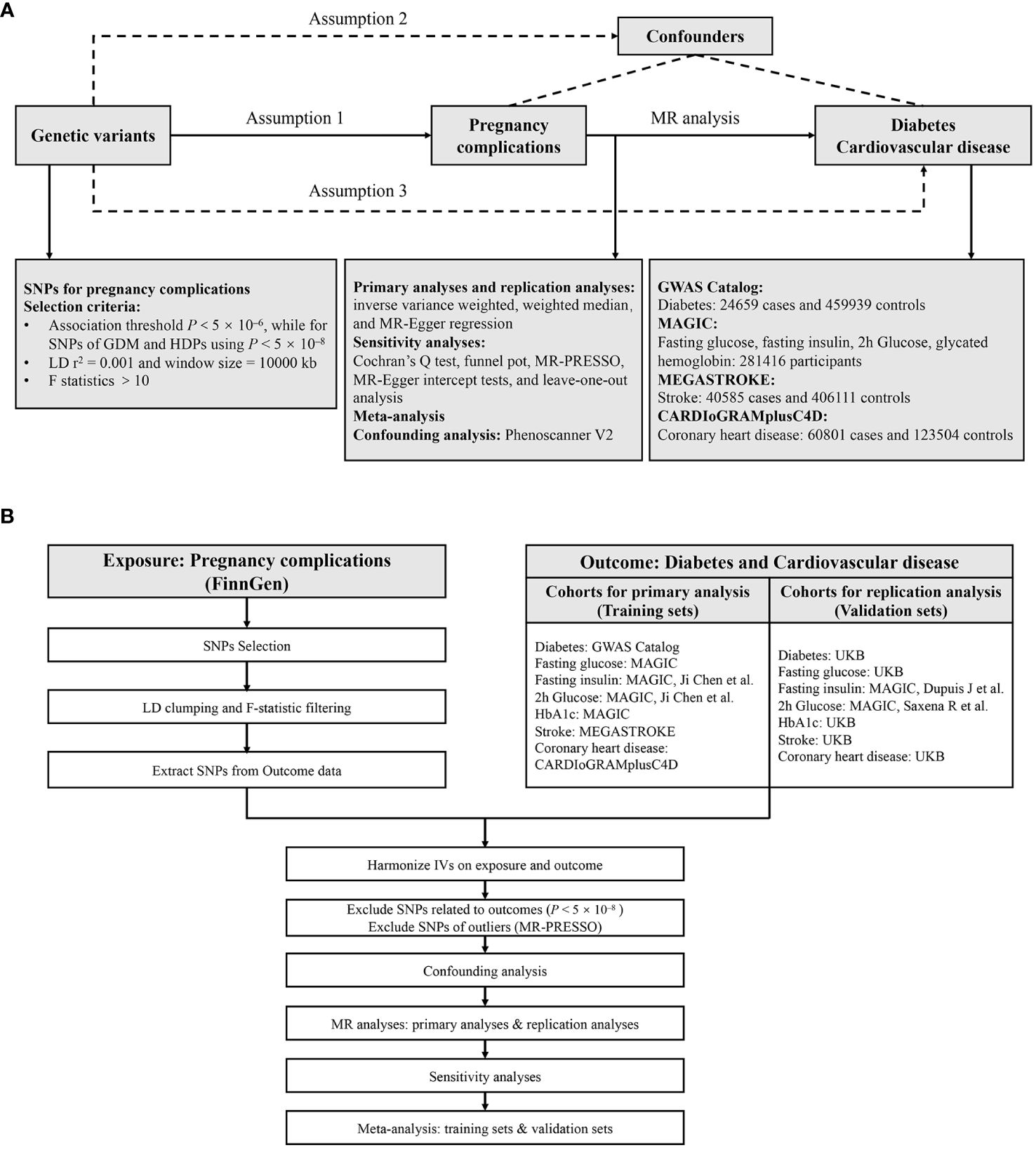
Figure 1 Study design (A) and workflow (B) of the current MR study. MR, Mendelian randomization; GDM, gestational diabetes mellitus; HDPs, hypertensive disorders in pregnancy; SNPs, single nucleotide polymorphisms; LD, linkage disequilibrium; HbA1c, glycated hemoglobin; UKB, UK Biobank; IVs, instrumental variables; MR-PRESSO, Mendelian randomization pleiotropy residual sum and outlier.
GWAS data for pregnancy complications
Six common pregnancy complications, namely gestational diabetes mellitus (GDM), hypertensive disorders in pregnancy (HDPs), gestational hypertension (GH), spontaneous abortion, pregnancy with abortive outcome, and preterm birth were included as exposure in this study. Additionally, spontaneous delivery was included as a negative control cohort to mitigate false positives. Summary-level statistics for these conditions were retrieved from the FinnGen GWAS R9 release (26). The Coordinating Ethics Committee of the Helsinki and Uusimaa Hospital District has approved the FinnGen consortium (Nr HUS/990/2017). Definitions of the above pregnancy outcomes can be found in Supplementary Table 1.
GWAS data for diabetes and cardiovascular diseases
We utilized five sets of instruments indicating different aspects of glucose metabolism and pathophysiology: diabetes, fasting glucose, fasting insulin, 2-hour post-challenge glucose (2hGlu), and glycated hemoglobin (HbA1c). Diabetes is diagnosed as a chronic illness related to dysglycemia and pancreatic impairment. Fasting glucose, 2hGlu, and HbA1c serve as diagnostic indicators for this disease. Moreover, HbA1c is frequently employed as a key biomarker for ongoing glucose control in diabetic patients. Fasting insulin, on the other hand, encapsulates both insulin secretion and insulin resistance, which are integral components in the pathophysiology of diabetes and in insulin clearance (27). Taken together, these four glycemic traits are indispensable for a comprehensive understanding of both diabetes pathophysiology (28–30) and associated cardiometabolic outcomes (31).
The GWAS statistics for diabetes (24659 cases and 459939 controls) in primary analysis were retrieved from GWAS Catalog consortium (32), and SNPs for other markers were obtained from the MAGIC consortium (33). A total of 281416 individuals (~70% European ancestry) without diabetes were included in this study. Data for stroke (40585 cases and 406111 controls) and coronary heart disease (60801 cases and 123504 controls) were obtained from MEGASTROKE and CARDIoGRAMplusC4D consortia, respectively (34). Details of data source are listed in Table 1.
To corroborate our findings, we conducted replication and meta-analyses using data from the UK Biobank for diabetes, fasting glucose, HbA1c, stroke and CHD, which is publicly available at the website: http://www.nealelab.is/uk-biobank/, and two independent GWAS studies in MAGIC consortium for fasting insulin and 2hGlu (35, 36). More details about the definitions, corresponding ethic committees and approval IDs can be found in Supplementary Table 2. GWAS data used in primary analysis is regarded as training sets, while data in replication analysis is validation sets.
Instruments selection
A multi-step protocol to identify eligible SNPs associated with pregnancy complications were performed. First, given the limited number of SNPs reaching genome-wide significance for gestational hypertension, spontaneous abortion, pregnancy with abortive outcome, preterm birth, and spontaneous delivery, we relaxed the association threshold using P < 5 × 10–6, while for SNPs of gestational diabetes mellitus and hypertensive disorders in pregnancy using P < 5 × 10–8. Second, the SNP selection was further refined through linkage disequilibrium (LD) clumping (r2 = 0.001 and window size = 10000 kb) and F-statistic filtering to ensure robust instruments (37). SNPs with F < 10 were recognized as weak instruments and were discarded to ensure all the SNPs conferred sufficient variance for corresponding pregnancy complications (38). Third, we extracted the exposure SNPs from the outcome data and excluded those associated with the outcome (P < 5 × 10–8). Finally, harmonization was conducted to align the alleles of exposure- and outcome-SNPs, and discard palindromic SNPs with intermediate effect allele frequencies (EAF > 0.42) or SNPs with incompatible alleles (e.g. A/G vs. A/C).
Primary analyses
The random-effect inverse variance weighted (IVW) method was conducted as the primary analysis to identify significant causal effect with P < 0.05. Furthermore, weighted median (WM) and MR-Egger regression were served as supplement to IVW. The weighted median method consistently estimates effects when at least half of the weighted variance remains unaffected by horizontal pleiotropy (39). MR-Egger regression could offer a test for unbalanced pleiotropy and considerable heterogeneity, although it demands a larger sample size for the same underexposure variation (40). Specifically, IVW estimates would be biased if horizontal pleiotropy existed. In this scenario, the MR-Egger estimates should be referenced because this method modifies the IVW analysis to accommodate the potential imbalances or directionalities in horizontal pleiotropic effects across all SNPs (41).
Sensitivity analyses
Horizontal pleiotropy occurs when genetic variants associated with the exposure of interest (pregnancy complications) directly affect the outcome (diabetes and cardiovascular diseases) through multiple pathways other than the proposed exposure. Therefore, we further conducted Cochran’s Q statistic, funnel plot, Mendelian Randomization Pleiotropy Residual Sum and Outlier (MR-PRESSO), MR-Egger intercept tests and leave-one-out (LOO) analysis to detect the presence of pleiotropy and validate the robustness of the results. Specifically, heterogeneity was detected if the P value of the Cochran Q test was less than 0.05. Funnel plots aided in evaluating the probable directional pleiotropy. SNPs with potential pleiotropy were removed after MR-PRESSO, then MR analyses were reperformed to assess robustness. We also appraised horizontal pleiotropy using MR-Egger intercept. To determine whether the causal estimate was driven by any single SNP, we performed LOO analysis, which entails cyclically discarding each exposure-associated SNP to repeat the IVW analysis.
Replication and meta−analysis
To validate the robustness of results, we replicated mendelian randomization analysis using another independent GWAS data from different consortia mentioned above. This was followed by a meta-analysis to consolidate the results.
Confounding analysis
Although multiple statistical methods were employed in sensitivity analysis to inspect potential violation of the MR assumptions, we further scrutinized the Phenoscanner V2 website (http://www.phenoscanner.medschl.cam.ac.uk/) to explore whether the exposure-associated SNPs were meanwhile associated with several common risk factors that might bias the MR estimates, including smoking, obesity (42), hypertension (43), and coronary heart disease (44). SNPs associated with these potential confounders at the threshold of P < 1×10–5 were removed.
Results
Following the instrument selection steps, F statistics for SNPs after clumping were all over 10, suggesting no weak instruments were employed (Supplementary Table 3). The harmonized data of each outcome were presented in Supplementary Table 4.
MR estimates
In the tested gestational diabetes mellitus (GDM) phenotype, IVW analysis indicated that genetically predicted GDM increased the risk of diabetes (OR = 1.01, 95% CI 1–1.01; P = 0.01), and coronary heart disease (CHD) (OR = 1.08, 95% CI 1.04–1.13; P = 0.0004) (Figure 2). The results from other MR methods showed a consistent direction (Figure 3). Genetically proxied hypertensive disorders in pregnancy (HDPs) was significantly associated with an increased risk of stroke (OR = 1.11, 95% CI 1.04–1.18; P = 0.002), and CHD (OR = 1.3, 95% CI 1.2–1.4; P = 3.11E-11) in the IVW analysis (Figure 2). Similar causal estimates were obtained from WM model, as in stroke (OR = 1.11, 95% CI 1.02–1.22; P = 0.02), and CHD (OR = 1.25, 95% CI 1.13–1.38, P = 1.11E-05). Moreover, a positive causality between genetic predisposition toward PA and HbA1c was detected using IVW analysis (OR = 1.02, 95% CI 1.01–1.04; P = 0.01) and WM analysis (OR = 1.03, 95% CI 1.01–1.06; P = 0.01). A potential causal association of gestational hypertension (GH) with CHD was also observed in the IVW analysis (OR = 1.11, 95% CI 1.02–1.22; P = 0.04) (Figure 3). However, we did not observe evidence of causal association between preterm birth and diabetes and cardiovascular disease (Supplementary Table 6).
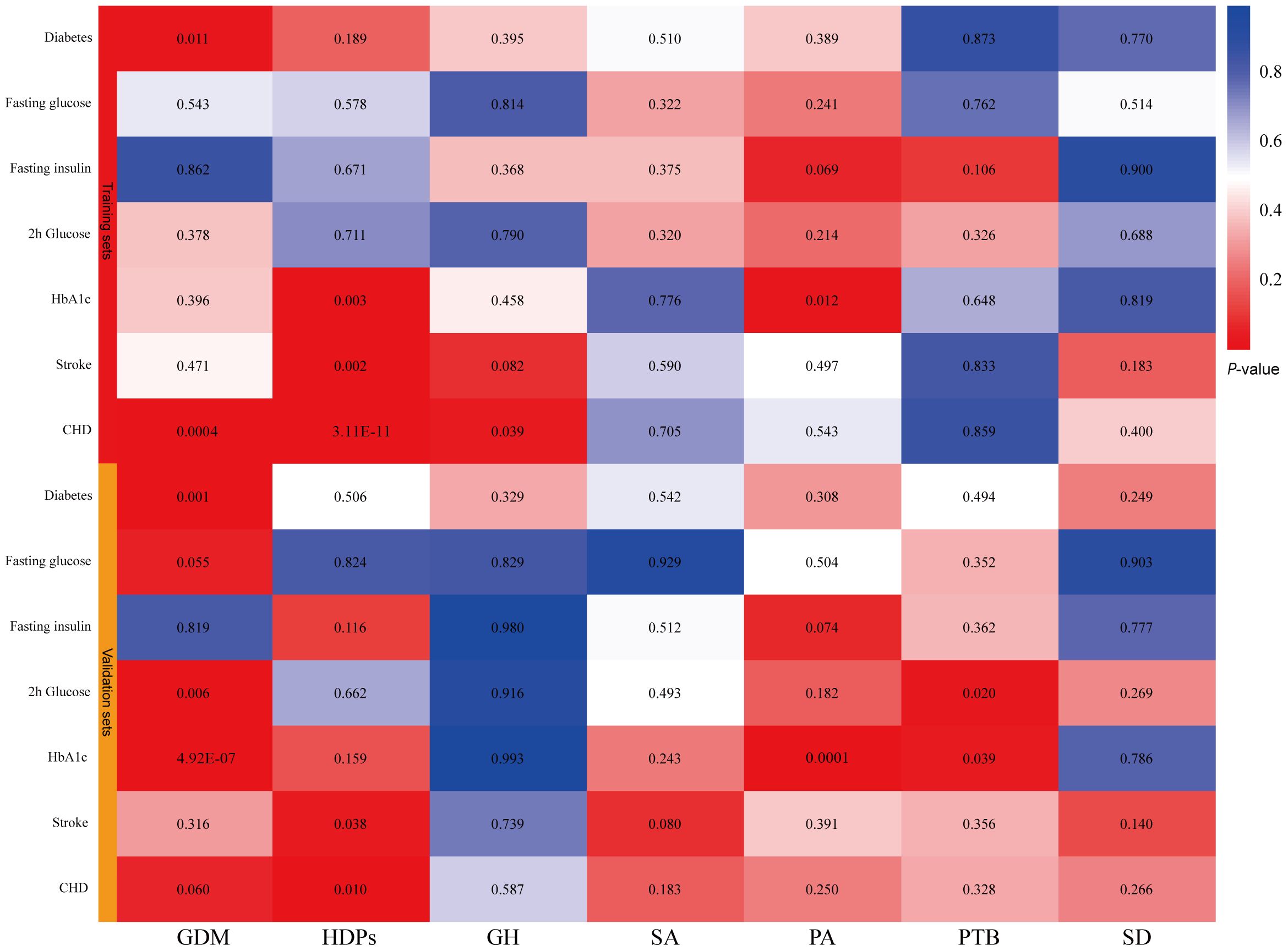
Figure 2 IVW estimates from pregnancy complications on diabetes and cardiovascular disease. The color of each block represents the IVW-derived P-values of every MR analysis. P-values of < 0.05 were shown in red and P-values of > 0.05 were shown in blue. GDM, gestational diabetes mellitus; HDPs, hypertensive disorders in pregnancy; GH, gestational hypertension; SA, spontaneous abortion; PA, pregnancy with abortive outcomes; PTB, preterm birth; SD, spontaneous delivery; HbA1c, glycated hemoglobin; CHD, coronary heart disease.
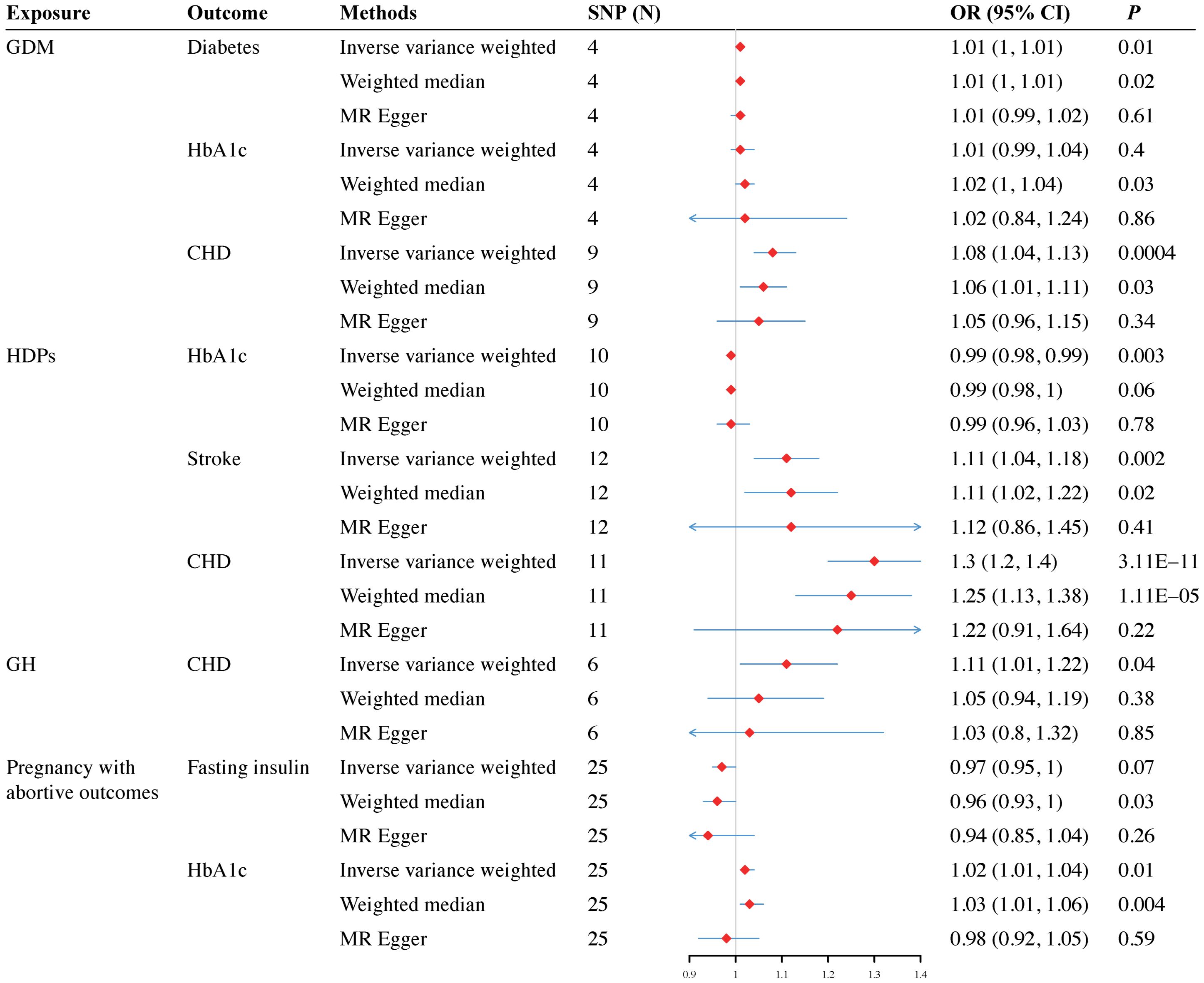
Figure 3 Forest plots for the causal effect of pregnancy complications on the risk of diabetes and cardiovascular disease derived from inverse variance weighted (IVW), weighted median (WM) and MR-Egger analysis. OR, odds ratio; CI, confidence interval; SNPs, single nucleotide polymorphisms; GDM, gestational diabetes mellitus; HDPs, hypertensive disorders in pregnancy; GH, gestational hypertension; HbA1c, glycated hemoglobin; CHD, coronary heart disease.
Sensitivity analyses
To assess the robustness of the above results, a series of sensitivity analyses, including Cochran’s Q test, MR-Egger intercept, and MR-PRESSO global test, were conducted (Supplementary Table 6). Results of MR-Egger intercept indicated that no pleiotropy existed. However, heterogeneity was observed in the Q test analysis of GDM and diabetes (Q = 10.71, P = 0.03), HDPs and CHD (Q = 38.57, P = 0.0001). Though heterogeneity was detected, it did not invalidate the MR estimates as random-effect IVW in the current study might balance the pooled heterogeneity. In addition, Egger intercepts did not identify any pleiotropy, suggesting that no pleiotropic bias was introduced to MR estimates in the context of heterogeneity (Supplementary Figures 1-6). Moreover, LOO analysis revealed that no SNP drove the results, and funnel plots were symmetrical (Supplementary Figures 7-12), indicating that none of the estimates were violated. Finally, after dropping the outliers detected by MR-PRESSO analysis, causal inference remained in the reperformed MR estimates.
Replication and meta−analysis
To further verify our results, replication analysis was conducted using independent GWAS data from two different consortia. As expected, similar trends were observed in GDM and diabetes, HDPs and cardiovascular disease (CVD), as well as pregnancy with abortive outcomes and HbA1c (Supplementary Table 7). Combined analysis of both datasets identified that genetic liability for GDM predicted a higher risk of diabetes (OR=1.01, 95% CI=1–1.01, P<0.0001), but a lower level of 2hGlu (OR=0.89, 95% CI=0.82–0.97, P=0.006). Moreover, genetically proxied pregnancy with abortive outcomes predicted a lower level of fasting insulin (OR=0.97, 95% CI=0.95–0.99, P=0.02) (Figure 4). However, null estimates of the meta-analysis were observed in HDPs and stroke or CHD, as well as pregnancy with abortive outcomes and HbA1c, which attributed to heterogeneity using the validation sets (Supplementary Figure 19).
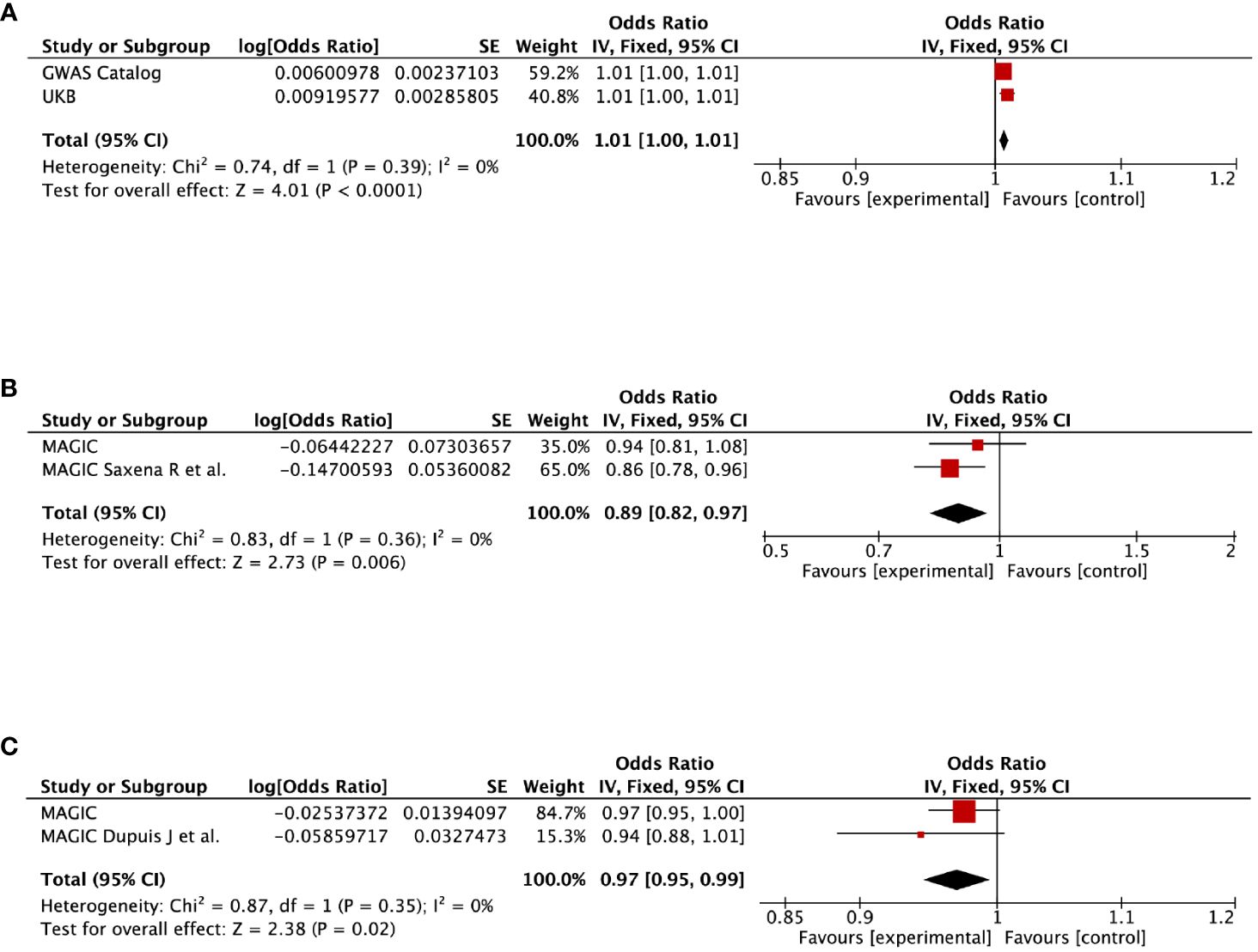
Figure 4 Meta-analysis of the causal impact of (A) gestational diabetes mellitus on diabetes, (B) gestational diabetes mellitus on 2-hour post-challenge glucose levels, and (C) pregnancy with abortive outcomes on fasting insulin levels. CI, confidence interval.
Confounding analysis
Although bias invalidating the MR estimates had been treated by multiple sensitivity analyses, we further manually investigated the risk factors (smoking, obesity, self-reported hypertension, and coronary artery disease) of the harmonized SNPs. Looking over the Phenoscanner, we found that SNPs associated with spontaneous abortion, GDM and GH were not associated with any of the confounders. For pregnancy with abortive outcomes, two SNPs (rs1898357 and rs2284174) were associated with obesity-related traits. Similar for preterm birth (rs2946160 and rs7591150), and spontaneous delivery (rs7209460 and rs6011779), we identified four SNPs associated with the risk factors mentioned above. The estimates remained after discarding these SNPs.
Discussion
The present study indicated that genetic predisposition to gestational diabetes mellitus (GDM) was causally associated with an increased risk of diabetes. Furthermore, genetic liability to GDM and pregnancy with abortive outcomes correlated with reduced levels of 2hGlu and fasting insulin, respectively. Additionally, genetic proxied pregnancy with abortive outcomes might lead to a higher level of HbA1c in later life. Genetic liability for hypertensive disorders in pregnancy (HDPs) appeared to be causally related to stroke and coronary heart disease (CHD). Consistent results were obtained in both of the independent datasets, although the meta-analysis results were inconclusive.
Genetic proxied gestational hypertension might have a potential causal impact on risk of CHD. No associations were observed between preterm birth and outcomes of diabetes, stroke, or CHD. To the best of our knowledge, this is the first Mendelian randomization (MR) study using both replication and meta-analysis to systematically evaluate the causal impact of pregnancy complications on diabetes and cardiovascular disease (CVD).
Given the high prevalence and mortality of diabetes and cardiovascular disease, early screening and prevention are of great importance. Previous studies have identified several pregnancy complications as risk factors for diabetes, cardiovascular disease, and even premature mortality. For instance, Wang et al. reported that spontaneous abortion was associated with an increased risk of premature mortality, especially death from cardiovascular disease (12). Moreover, the American Heart Association has classified women with a history of preeclampsia, gestational diabetes, or pregnancy-induced hypertension as being at risk of cardiovascular disease since 2011 (45). However, the existing literature has yet to definitively establish the causal role of pregnancy complications in the onset of diabetes and CVD. Inspired by You et al. (6) and McNestry et al. (46), we designed this multi-exposure MR study to systematically evaluate the causality between pregnancy complications and diabetes, as well as cardiovascular disease (stroke and CHD), aiming to provide robust evidence for risk stratification and targeted screening for women with a history of pregnancy complications.
Our results align with existing literature, suggesting that a genetic predisposition for GDM has detrimental long-term effects, particularly in increasing diabetes risk. In a retrospective study of 321 women with GDM followed up for 1–6 years postpartum, Miao et al. found that insulin resistance and insufficient compensatory insulin secretion remain long-lasting in the postpartum phase, and thus the risk of diabetes is higher (17). During a healthy pregnancy, maternal metabolism undergoes notable shifts, driven primarily by placental hormones. These hormonal changes induce a state of progressive insulin resistance, ensuring that the fetus receives adequate nutrition for optimal growth and development. To compensate for increased insulin resistance, the mother’s β-cells upregulate insulin secretion. However, when this compensatory mechanism is overwhelmed, insulin resistance escalates to prediabetes and eventually leads to a reduction in insulin secretion, culminating in overt diabetes (17). Though specific pathways underlying these metabolic adaptations remain elusive, the parallels between GDM and diabetes are striking. Both conditions are characterized by failed β-cell compensation and insulin insufficiency, suggesting shared pathophysiological mechanisms (47). Recent studies have postulated that pregnancy serves as a metabolic “stress test” for the mother, unmasking latent predispositions to chronic conditions such as diabetes (48). This concept aligns with research suggesting that GDM may be a manifestation of “recessive” diabetes, in which genetic susceptibilities become clinically apparent only under the physiological stress of pregnancy (45). In this way, pregnancy could offer a unique window for early identification and intervention in women at risk for future metabolic and cardiovascular diseases.
Notably, some results deviated from initial expectations. While a higher genetic liability for gestational diabetes mellitus typically suggests impaired glycemic control, our study paradoxically found an association with lower 2hGlu levels. Herein, the MR estimates were consistent across independent datasets and showed no signs of pleiotropy, which also consist with those of previous reports. Riviello et al. demonstrated that postpartum women with overt diabetes, particularly type 1, often require less insulin during lactation and are more prone to hypoglycemia (49). One plausible hypothesis is that lactation enhances pancreatic β-cell adaptability to insulin resistance, thereby stabilizing long-term glycemic control (50). However, this area remains underexplored, underscoring the need for future research. Similarly for pregnancy with abortive outcome, scant literature exists on its relationship with fasting insulin. Existing evidence indicated that threatened miscarriage was inversely correlated with glucose intolerance severity, possibly due to reduced insulin resistance influenced by a lower level of progesterone (51). This was also confirmed in animal experiments (52). Lower fasting insulin levels could also signal compromised β-cell function (53). Further elucidative studies are therefore warranted.
In this study, we performed replication analyses across two independent datasets. Both datasets corroborated that genetic predisposition to pregnancy with abortive outcome was linked with higher HbA1c levels, while genetic liability for hypertensive disorders in pregnancy increased the risk of stroke and coronary heart disease. However, meta-analytic outcomes varied, possibly due to the application of a random-effects model accounting for gender-based heterogeneity. In fact, we could get a positive result if a fixed-effects model was utilized. Though heterogeneity is an unavoidable limitation due to the scarcity of publicly available sex-stratified data, the consistency in our replicated analysis lends credence to our conclusions. Yet, these findings would benefit from further validation through future sex-specific GWAS data. We analyzed gestational hypertension (GH) as a subgroup of hypertension disorders in pregnancy (HDPs). In our study, a potential causal association between genetically predicted GH and coronary heart disease (CHD) was observed only in the training set, with the association being negative in the validation set and meta-analysis. Considering the strong statistical power of the IVW method and the absence of heterogeneity and pleiotropy in sensitivity analyses, we contend that this result should not be ignored. This discrepancy may be due to the relatively small sample size of GH (8502 cases) and CHD (2930 cases) in the validation set. Therefore, our findings warrant validation in future studies with larger sample sizes.
We found no significant associations between preterm birth and diabetes, stroke or coronary heart disease. In contrast, some observational studies suggest such associations (54–56). The discrepancy might arise from residual confounding inherent to observational setting. This is particularly true for cardiovascular disease, which is known to have multifactorial influences (43, 44).
The current study has several strengths. First, a notable strength is the comprehensive selection of exposure and outcome. We incorporated double cohorts (spontaneous abortion and pregnancy with abortive outcome) related to abortion and a negative cohort (spontaneous delivery) for MR analysis. By utilizing five sets of instruments, we provided an in-depth view of glycemia metabolic function, making our study a systematic exploration of pregnancy complications’ contribution to diabetes and cardiovascular disease. Second, the MR design mitigates concerns about reverse causation and residual confounding. We employed multiple MR and sensitivity analyses to confirm adherence to MR assumptions, enhancing the validity of our estimates. Third, application of replication and meta-analyses further strengthen the study. The consistent findings across datasets validate the robustness of our results.
Several limitations should be noted in our study. First, given the limited SNPs for spontaneous abortion, pregnancy with abortive outcome, preterm birth, and spontaneous delivery reaching genome-wide significance, we relaxed the P threshold, which is a widely accepted approach (57). However, the F statistic for these SNPs exceeded 10, suggesting no weak instruments were included. Second, the study predominantly features European participants. While this minimizes population heterogeneity, it necessitates further validation in diverse ancestries to ensure broad applicability. Third, although we examined multiple exposures, we did not adjust MR estimations for multiple testing. Instead, we opted for replication analysis with independent datasets, enhancing the credibility. We contend that an overly conservative multiple testing threshold might overshadow individually significant associations. Furthermore, due to the lack of GWAS data that exclusively includes patients with hypertensive disorders in pregnancy (HDPs), the interpretation of the results considering HDPs in our study needs to be cautious. Finally, although we used sex-specific data for exposure, outcome data were largely pooled from GWAS statistics for both genders due to the scarcity of gender-stratified data in public repositories, which is inevitable in similar studies (58).
Conclusions
In conclusion, gestational diabetes was causally associated with an increased risk of diabetes but a lower level of 2hGlu. Pregnancy with abortive outcomes predicted a lower level of fasting insulin but probably a higher level of HbA1c in later life. In addition, hypertensive disorders in pregnancy were probably causally associated with a higher risk of stroke and coronary heart disease (CHD). However, no association were noted between preterm birth and the outcome of diabetes, stroke or CHD. Our findings underscore the clinical importance of pregnancy as a critical period for identifying women at higher risk for diabetes and cardiovascular disease, thereby informing targeted risk stratification and early interventions.
Data availability statement
The datasets presented in this study can be found in online repositories. The names of the repository/repositories and accession number(s) can be found in the article/Supplementary Material.
Ethics statement
The studies involving humans were approved by corresponding ethics committee. The studies were conducted in accordance with the local legislation and institutional requirements. Written informed consent for participation was not required from the participants or the participants’ legal guardians/next of kin in accordance with the national legislation and institutional requirements.
Author contributions
YX: Conceptualization, Data curation, Investigation, Methodology, Writing – original draft, Writing – review & editing. JZ: Conceptualization, Formal Analysis, Visualization, Writing – review & editing, Funding acquisition. SN: Conceptualization, Visualization, Writing – review & editing. JL: Conceptualization, Funding acquisition, Supervision, Writing – review & editing.
Funding
The author(s) declare financial support was received for the research, authorship, and/or publication of this article. This work was supported by grant from the National Natural Science Foundation of China (82174428), and Shanghai Municipal Health Commission project (SHGZS-202209).
Acknowledgments
We thank all the participants and investigators of the FinnGen, GWAS Catalog, MAGIC, MEGASTROKE, CARDIoGRAMplusC4D, and UK Biobank consortium.
Conflict of interest
The authors declare that the research was conducted in the absence of any commercial or financial relationships that could be construed as a potential conflict of interest.
Publisher’s note
All claims expressed in this article are solely those of the authors and do not necessarily represent those of their affiliated organizations, or those of the publisher, the editors and the reviewers. Any product that may be evaluated in this article, or claim that may be made by its manufacturer, is not guaranteed or endorsed by the publisher.
Supplementary material
The Supplementary Material for this article can be found online at: https://www.frontiersin.org/articles/10.3389/fendo.2024.1293292/full#supplementary-material
Abbreviations
2hGlu, 2-hour post-challenge glucose; HbA1c, glycated hemoglobin; GDM, gestational diabetes mellitus; GH, gestational hypertension; CVD, cardiovascular disease; CHD, coronary heart disease; IVW, inverse variance weighted; WM, weighted median.
References
1. Saravanan P. Diabetes in pregnancy working G, maternal medicine clinical study G, royal college of O, gynaecologists UK. Gestational diabetes: opportunities for improving maternal and child health. Lancet Diabetes Endocrinol. (2020) 8:793–800. doi: 10.1016/S2213–8587(20)30161–3
2. Gestational hypertension and preeclampsia: acog practice bulletin, number 222. Obstet Gynecol. (2020) 135:e237–e60. doi: 10.1097/AOG.0000000000003891
3. Quenby. S, Gallos. ID, Dhillon-Smith. RK, Podesek. M, Stephenson. MD, Fisher. J, et al. Miscarriage matters: the epidemiological, physical, psychological and economic burden of early pregnancy loss. Lancet. (2021) 397:1658–67. doi: 10.1016/S0140–6736(21)00682–6
4. Blencowe H, Cousens S, Oestergaard MZ, Chou D, Moller AB, Narwal R, et al. National, regional, and worldwide estimates of preterm birth rates in the year 2010 with time trends since 1990 for selected countries: A systematic analysis and implications. Lancet. (2012) 379:2162–72. doi: 10.1016/S0140–6736(12)60820–4
5. Ye W, Luo C, Huang J, Li C, Liu Z, Liu F. Gestational diabetes mellitus and adverse pregnancy outcomes: systematic review and meta-analysis. BMJ. (2022) 377:e067946. doi: 10.1136/bmj-2021–067946
6. You Q, Jiang Q, Shani I, Lou Y, Huang S, Wang S, et al. Miscarriage, stillbirth and the risk of diabetes in women: A systematic review and meta-analysis. Diabetes Res Clin Pract. (2023) 195:110224. doi: 10.1016/j.diabres.2022.110224
7. Liu B, Song L, Li H, Zheng X, Yuan J, Liang Y, et al. History of spontaneous miscarriage and the risk of diabetes mellitus among middle-aged and older chinese women. Acta Diabetol. (2018) 55:579–84. doi: 10.1007/s00592-018-1125-z
8. Alejandro EU, Mamerto TP, Chung G, Villavieja A, Gaus NL, Morgan E, et al. Gestational diabetes mellitus: A harbinger of the vicious cycle of diabetes. Int J Mol Sci. (2020) 21. doi: 10.3390/ijms21145003
9. Bortoletto P, Lucas ES, Melo P, Gallos ID, Devall AJ, Bourne T, et al. Miscarriage syndrome: linking early pregnancy loss to obstetric and age-related disorders. EBioMedicine. (2022) 81:104134. doi: 10.1016/j.ebiom.2022.104134
10. Okoth K, Chandan JS, Marshall T, Thangaratinam S, Thomas GN, Nirantharakumar K, et al. Association between the reproductive health of young women and cardiovascular disease in later life: umbrella review. BMJ. (2020) 371:m3502. doi: 10.1136/bmj.m3502
11. Hinkle SN, Schisterman EF, Liu D, Pollack AZ, Yeung EH, Mumford SL, et al. Pregnancy complications and long-term mortality in a diverse cohort. Circulation. (2023) 147:1014–25. doi: 10.1161/CIRCULATIONAHA.122.062177
12. Wang YX, Minguez-Alarcon L, Gaskins AJ, Missmer SA, Rich-Edwards JW, Manson JE, et al. Association of spontaneous abortion with all cause and cause specific premature mortality: prospective cohort study. BMJ. (2021) 372:n530. doi: 10.1136/bmj.n530
13. Chan JCN, Lim LL, Wareham NJ, Shaw JE, Orchard TJ, Zhang P, et al. The lancet commission on diabetes: using data to transform diabetes care and patient lives. Lancet. (2021) 396:2019–82. doi: 10.1016/S0140–6736(20)32374–6
14. Vogel B, Acevedo M, Appelman Y, Bairey Merz CN, Chieffo A, Figtree GA, et al. The lancet women and cardiovascular disease commission: reducing the global burden by 2030. Lancet. (2021) 397:2385–438. doi: 10.1016/S0140-6736(21)00684-X
15. Wagner MM, Bhattacharya S, Visser J, Hannaford PC, Bloemenkamp KW. Association between miscarriage and cardiovascular disease in a scottish cohort. Heart. (2015) 101:1954–60. doi: 10.1136/heartjnl-2015–307563
16. Lao TT. The mother–the long-term implications on metabolic and cardiovascular complications. Best Pract Res Clin Obstet Gynaecol. (2015) 29:244–55. doi: 10.1016/j.bpobgyn.2014.06.010
17. Miao Z, Wu H, Ren L, Bu N, Jiang L, Yang H, et al. Long-term postpartum outcomes of insulin resistance and beta-cell function in women with previous gestational diabetes mellitus. Int J Endocrinol. (2020) 2020:7417356. doi: 10.1155/2020/7417356
18. Parikh NI, Gonzalez JM, Anderson CAM, Judd SE, Rexrode KM, Hlatky MA, et al. Adverse pregnancy outcomes and cardiovascular disease risk: unique opportunities for cardiovascular disease prevention in women: A scientific statement from the american heart association. Circulation. (2021) 143:e902–e16. doi: 10.1161/CIR.0000000000000961
19. Sekula P, Del Greco MF, Pattaro C, Kottgen A. Mendelian randomization as an approach to assess causality using observational data. J Am Soc Nephrol. (2016) 27:3253–65. doi: 10.1681/ASN.2016010098
20. Richmond RC, Davey Smith G. Mendelian randomization: concepts and scope. Cold Spring Harb Perspect Med. (2022) 12. doi: 10.1101/cshperspect.a040501
21. Burgess S, Thompson SG. Multivariable mendelian randomization: the use of pleiotropic genetic variants to estimate causal effects. Am J Epidemiol. (2015) 181:251–60. doi: 10.1093/aje/kwu283
22. Davey Smith G, Hemani G. Mendelian randomization: genetic anchors for causal inference in epidemiological studies. Hum Mol Genet. (2014) 23:R89–98. doi: 10.1093/hmg/ddu328
23. Skrivankova VW, Richmond RC, Woolf BAR, Yarmolinsky J, Davies NM, Swanson SA, et al. Strengthening the reporting of observational studies in epidemiology using mendelian randomization: the strobe-mr statement. JAMA. (2021) 326:1614–21. doi: 10.1001/jama.2021.18236
24. Boef AG, Dekkers OM, le Cessie S. Mendelian randomization studies: A review of the approaches used and the quality of reporting. Int J Epidemiol. (2015) 44:496–511. doi: 10.1093/ije/dyv071
25. Emdin. CA, Khera. AV, Kathiresan. S. Mendelian randomization. JAMA. (2017) 318:1925–6. doi: 10.1001/jama.2017.17219
26. Kurki MI, Karjalainen J, Palta P, Sipila TP, Kristiansson K, Donner KM, et al. Finngen provides genetic insights from a well-phenotyped isolated population. Nature. (2023) 613:508–18. doi: 10.1038/s41586–022-05473–8
27. Goodarzi MO, Cui J, Chen YD, Hsueh WA, Guo X, Rotter JI. Fasting insulin reflects heterogeneous physiological processes: role of insulin clearance. Am J Physiol Endocrinol Metab. (2011) 301:E402–8. doi: 10.1152/ajpendo.00013.2011
28. Udler MS, McCarthy MI, Florez JC, Mahajan A. Genetic risk scores for diabetes diagnosis and precision medicine. Endocr Rev. (2019) 40:1500–20. doi: 10.1210/er.2019–00088
29. Dimas AS, Lagou V, Barker A, Knowles JW, Magi R, Hivert MF, et al. Impact of type 2 diabetes susceptibility variants on quantitative glycemic traits reveals mechanistic heterogeneity. Diabetes. (2014) 63:2158–71. doi: 10.2337/db13–0949
30. Udler MS, Kim J, von Grotthuss M, Bonas-Guarch S, Cole JB, Chiou J, et al. Type 2 diabetes genetic loci informed by multi-trait associations point to disease mechanisms and subtypes: A soft clustering analysis. PloS Med. (2018) 15:e1002654. doi: 10.1371/journal.pmed.1002654
31. Emerging Risk Factors Collaboration NS, Gao P, Kondapally Seshasai SR, Gobin R, Kaptoge S, Di Angelantonio E, et al. Diabetes mellitus, fasting blood glucose concentration, and risk of vascular disease: A collaborative meta-analysis of 102 prospective studies. Lancet. (2010) 375:2215–22. doi: 10.1016/S0140-6736(10)60484-9
32. Donertas HM, Fabian DK, Valenzuela MF, Partridge L, Thornton JM. Common genetic associations between age-related diseases. Nat Aging. (2021) 1:400–12. doi: 10.1038/s43587–021-00051–5
33. Chen. J, Spracklen. CN, Marenne. G, Varshney. A, Corbin. LJ, Luan JA. The trans-ancestral genomic architecture of glycemic traits. Nat Genet. (2021) 53:840–60.
34. Nikpay M, Goel A, Won HH, Hall LM, Willenborg C, Kanoni S, et al. A comprehensive 1,000 genomes-based genome-wide association meta-analysis of coronary artery disease. Nat Genet. (2015) 47:1121–30. doi: 10.1038/ng.3396
35. Saxena R, Hivert MF, Langenberg C, Tanaka T, Pankow JS, Vollenweider P, et al. Genetic variation in gipr influences the glucose and insulin responses to an oral glucose challenge. Nat Genet. (2010) 42:142–8. doi: 10.1038/ng.521
36. Dupuis J, Langenberg C, Prokopenko I, Saxena R, Soranzo N, Jackson AU, et al. New genetic loci implicated in fasting glucose homeostasis and their impact on type 2 diabetes risk. Nat Genet. (2010) 42:105–16. doi: 10.1038/ng.520
37. Cai J, He L, Wang H, Rong X, Chen M, Shen Q, et al. Genetic liability for prescription opioid use and risk of cardiovascular diseases: A multivariable mendelian randomization study. Addiction. (2022) 117:1382–91. doi: 10.1111/add.15767
38. Burgess S, Butterworth A, Thompson SG. Mendelian randomization analysis with multiple genetic variants using summarized data. Genet Epidemiol. (2013) 37:658–65. doi: 10.1002/gepi.21758
39. Bowden J, Davey Smith G, Haycock PC, Burgess S. Consistent estimation in mendelian randomization with some invalid instruments using a weighted median estimator. Genet Epidemiol. (2016) 40:304–14. doi: 10.1002/gepi.21965
40. Bowden J, Davey Smith G, Burgess S. Mendelian randomization with invalid instruments: effect estimation and bias detection through egger regression. Int J Epidemiol. (2015) 44:512–25. doi: 10.1093/ije/dyv080
41. Bowden J, Del Greco MF, Minelli C, Davey Smith G, Sheehan NA, Thompson JR. Assessing the suitability of summary data for two-sample mendelian randomization analyses using mr-egger regression: the role of the I2 statistic. Int J Epidemiol. (2016) 45:1961–74. doi: 10.1093/ije/dyw220
42. Nathan DM. Diabetes: advances in diagnosis and treatment. JAMA. (2015) 314:1052–62. doi: 10.1001/jama.2015.9536
43. Dalen JE, Alpert JS, Goldberg RJ, Weinstein RS. The epidemic of the 20(Th) century: coronary heart disease. Am J Med. (2014) 127:807–12. doi: 10.1016/j.amjmed.2014.04.015
44. Haast RA, Gustafson DR, Kiliaan AJ. Sex differences in stroke. J Cereb Blood Flow Metab. (2012) 32:2100–7. doi: 10.1038/jcbfm.2012.141
45. Mosca L, Benjamin EJ, Berra K, Bezanson JL, Dolor RJ, Lloyd-Jones DM, et al. Effectiveness-based guidelines for the prevention of cardiovascular disease in women–2011 update: A guideline from the american heart association. Circulation. (2011) 123:1243–62. doi: 10.1161/CIR.0b013e31820faaf8
46. McNestry C, Killeen SL, Crowley RK, McAuliffe FM. Pregnancy complications and later life women’s health. Acta Obstet Gynecol Scand. (2023) 102:523–31. doi: 10.1111/aogs.14523
47. Williams D. Pregnancy: A stress test for life. Curr Opin Obstet Gynecol. (2003) 15:465–71. doi: 10.1097/01.gco.0000103846.69273.ba
48. Sanghavi M, Rutherford JD. Cardiovascular physiology of pregnancy. Circulation. (2014) 130:1003–8. doi: 10.1161/CIRCULATIONAHA.114.009029
49. Riviello. C, Mello. G, Jovanovic. LG. Breastfeeding and the basal insulin requirement in type 1 diabetic women. Endocr Pract. (2009) 15:187–93. doi: 10.4158/EP.15.3.187
50. McManus RM, Cunningham I, Watson A, Harker L, Finegood DT. Beta-cell function and visceral fat in lactating women with a history of gestational diabetes. Metabolism. (2001) 50:715–9. doi: 10.1053/meta.2001.23304
51. Lee HJ, Norwitz E, Lee B. Relationship between threatened miscarriage and gestational diabetes mellitus. BMC Pregnancy Childbirth. (2018) 18:318. doi: 10.1186/s12884–018-1955–2
52. Picard F, Wanatabe M, Schoonjans K, Lydon J, O’Malley BW, Auwerx J. Progesterone receptor knockout mice have an improved glucose homeostasis secondary to beta -cell proliferation. Proc Natl Acad Sci U.S.A. (2002) 99:15644–8. doi: 10.1073/pnas.202612199
53. Angueira AR, Ludvik AE, Reddy TE, Wicksteed B, Lowe WL Jr., Layden BT. New insights into gestational glucose metabolism: lessons learned from 21st century approaches. Diabetes. (2015) 64:327–34. doi: 10.2337/db14–0877
54. Wu P, Gulati M, Kwok CS, Wong CW, Narain A, O’Brien S, et al. Preterm delivery and future risk of maternal cardiovascular disease: A systematic review and meta-analysis. J Am Heart Assoc. (2018) 7. doi: 10.1161/JAHA.117.007809
55. Crump C, Sundquist J, Sundquist K. Preterm delivery and long-term risk of stroke in women: A national cohort and cosibling study. Circulation. (2021) 143:2032–44. doi: 10.1161/CIRCULATIONAHA.120.052268
56. Lykke JA, Paidas MJ, Damm P, Triche EW, Kuczynski E, Langhoff-Roos J. Preterm delivery and risk of subsequent cardiovascular morbidity and type-ii diabetes in the mother. BJOG. (2010) 117:274–81. doi: 10.1111/j.1471-0528.2009.02448.x
57. Cai J, Li X, Wu S, Tian Y, Zhang Y, Wei Z, et al. Assessing the causal association between human blood metabolites and the risk of epilepsy. J Transl Med. (2022) 20:437. doi: 10.1186/s12967–022-03648–5
Keywords: gestational diabetes, gestational hypertension, miscarriage, diabetes, cardiovascular disease, Mendelian randomization, causality
Citation: Xie Y, Zhang J, Ni S and Li J (2024) Assessing the causal association of pregnancy complications with diabetes and cardiovascular disease. Front. Endocrinol. 15:1293292. doi: 10.3389/fendo.2024.1293292
Received: 12 September 2023; Accepted: 21 May 2024;
Published: 05 June 2024.
Edited by:
Wei Wu, Nanjing Medical University, ChinaReviewed by:
Anamaria Savu, University of Alberta, CanadaLiv Cecilie Vestrheim Thomsen, University of Bergen, Norway
Copyright © 2024 Xie, Zhang, Ni and Li. This is an open-access article distributed under the terms of the Creative Commons Attribution License (CC BY). The use, distribution or reproduction in other forums is permitted, provided the original author(s) and the copyright owner(s) are credited and that the original publication in this journal is cited, in accordance with accepted academic practice. No use, distribution or reproduction is permitted which does not comply with these terms.
*Correspondence: Ji Li, ZHJsaWppQDE2My5jb20=
†These authors have contributed equally to this work