- Department of Reproductive Medicine, The Fourth Hospital of Hebei Medical University, Shijiazhuang, China
Purpose: This study aims to create and validate a clinical model that predict the probability of fertilization failure in routine in-vitro fertilization (IVF) cycles.
Methods: This study employed a retrospective methodology, gathering data from 1770 couples that used reproductive center’s of the Fourth Hospital of Hebei Medical University standard IVF fertilization between June 2015 and June 2023. 1062 were in the training set and 708 were in the validation set when it was randomly split into the training set and validation set in a 6:4 ratio. The study employed both univariate and multivariate logistic regression analysis to determine the factors those influence the failure of traditional in vitro fertilization. Based on the multiple regression model, a predictive model of traditional IVF fertilization failure was created. The calibration and decision curves were used to assess the effectiveness and therapeutic usefulness of this model.
Results: The following factors independently predicted the probability of an unsuccessful fertilization: infertility years, basal oestrogen, the rate of mature oocytes, oligoasthenozoospermia, sperm concentration, sperm vitality, percentage of abnormal morphological sperm, and percentage of progressive motility (PR%).The receiver operating characteristic curve’s area under the curve (AUC) in the training set is 0.776 (95% CI: 0.740,0.812), while the validation set’s AUC is 0.756 (95% CI: 0.708,0.805), indicating a rather high clinical prediction capacity.
Conclusion: Our generated nomogram has the ability to forecast the probability of fertilization failure in couples undergoing IVF, hence can assist clinical staff in making informed decisions.
1 Introduction
Fertilization failure, including total fertilization failure (TFF), is defined as a normal fertilization rate of less than 30% during in vitro fertilization-embryo transfer (IVF-ET) aided pregnancy (1). Failure to fertilize is inevitable and typically occurs in the range of 5% to 15%. The inability of couples with infertility issues to conceive is a contributing factor to fertilization failure, along with factors related to sperm and egg quality, the fertilization process, and the hormonal environment (2). Currently, it is suggested that oocyte activation failure (OAF) seems to be the primary cause of TFF (3). Sperm plays a vital role in fertilization, oocyte division, and paternal inheritance, making it a significant factor in fertilization failures (4). A significant number of fertilization failures are closely related to semen quality and low sperm function, including patients with severe, weak, and dysmospermia. Additionally, female factors such as oocytes maturity and oocytes quality are also associated with fertilization failure.
Rescue intracytoplasmic sperm injection (R-ICSI), also known as rescue ICSI, is widely used in reproductive centers to address IVF fertilization failure (5). Its goal is to improve the utilization rate of oocytes and reduce the psychological and economic burden on infertile couples caused by inadequate fertilization of precious oocytes. However, some studies suggested that rescue ICSI may result in lower later fertilization rates and clinical pregnancy rates due to the prolonged culture time of oocytes and slow embryo growth, which can leads to a mismatch with the endometrium (6). While ICSI can effectively address most cases of fertilization failure by directly injecting sperm into the oocyte plasma using micromanipulation, its use is limited to severe sperm factors and clear fertilization disorders. Moreover, the high cost and time-consuming nature of ICSI make it an unfavorable alternative to conventional IVF for pregnancy assistance (7). Therefor, accurately, predicting the probability of fertilization failure in advance is crucial to guide clinical workers in selecting the appropriate insemination methods and minimizing oocyte wastage. The objective of this paper is to develop a nomogram for predicting IVF fertilization failure.
2 Materials and methods
2.1 Patients’ selection
A retrospective study was conducted on couples undergoing IVF and Rescue ICSI cycles at the Fourth Hospital of Hebei Medical University from June 2015 to June 2023. According to the World Health Organization’s definition, infertility refers to couples who have been unable to conceive after at least one year unprotected intercourse (8). All participants in this study were infertile couples treated at our reproductive center. Infertility is categorized into primary infertility and secondary infertility. Primary infertility refers to the inability to conceive for more than one year, while secondary infertility refers to inability to conceive again for more than one year after a previous pregnancy (9). The inclusion criteria for the study were couples with a potential for fertilization failure. The following were the exclusion criteria: 1. Females with abnormal zona pellucida, such as indented zona pellucida; 2. Couples requiring sperm assistance due to the husband’s azoospermia; 3. Couples with chromosomal abnormalities or cancer; 4. Couples who did not undergo insemination or have oocytes retireved during the cycle. Informed consent was obtained from all participants. All infertile couples included in the study signed informed consent prior to undergoing IVF. A total of 1770 couples were included in the study, with 264 of them using rescue-ICSI due to fertilization failure.
2.2 The procedure of IVF
2.2.1 Controlled ovulation stimulation protocol
The individualized ovarian stimulation program is designed based on the female’s age, basic hormone levels, and ovarian reserve function. This program involves monitoring follicle growth, through transvaginal ultrasound, and adjustments are made according to the size of the dominant follicle and hormone levels. When the appropriate criteria are met, the patient is injected with human chorionic gonadotropin (hCG) or gonadotropin releasing hormone (GnRH-a), followed by a vaginal vault puncture to retrieve the oocytes. This procedure is conducted 36 hours after the trigger day.
2.2.2 Semen treatment and in vitro fertilization
On the day of oocyte retrieval, the male partner provided sperm by masturbating. After that, the sperm underwent density gradient centrifugation. Afterwards, the oocytes and sperm were incubated together for 4 hours. Then, the granulosa cells surrounding the oocytes were removed to make it easier to observe the discharge of the second polar body and the fertilization process (10). Fertilization failure is defined as a discharge rate of the second polar body of less than 30%, or when no discharge of the second polar body is apparent. In such cases, rescue-ICSI intervention becomes necessary.
2.3 Data collection
Age, Body Mass Index (BMI), occupation, type of infertility, years of infertility, and clinical diagnosis were recorded as the clinical parameters for both infertile couples. The levels of basal estrogen, luteinizing hormone (LH), and follicle stimulating hormone (FSH) in females, as well as estrogen, progesterone, and LH levels on the trigger day, were also measured. The mature follicular rate was assessed, along with semen parameters on the day of oocyte retrieval, including semen volume, percentage of morphologicaliy abnormal sperm, sperm vitality, sperm concentration, and PR%. A fertilization rate of less than 30%, or the expulsion of the second polar body of less than 30%, referred to as an IVF fertilization failure, was considered a positive event.
2.4 Statistical analysis
According to the random sampling technology, the infertile couples were divided into training set and validation set in a 6:4 ratio. The continuous variables were represented as the mean ± standard deviation (SD), while non-normally distributed data were presented as the median (interquartile range). To compare variables between groups, Student’s t-tests (for normally distributed data) or the Mann–Whitney U-test (for non-normally distributed data) were employed. Categorical variables were expressed as percentages, and the chi-squared test was used for statistical comparison. These data were analyzed using SPSS 23.0.
Univariate logistic regression was used to identify predictive factors associated with fertilization failure. The variables with P<0.05 were entered into the next multifactor analysis. To reduce overfit bias, internal validation was performed using bootstrap resampling. Bootstrapping repeated the process of drawing samples with replacement from the original dataset 500 times. The closer the original and corrected statistics, the better the fit of the regression model (11). The area under the ROC curve was used to assess the accuracy of the nomogram. Additionally, a decision curve analysis was performed to determine the clinical utility of this model. The statistical analysis mentioned above was conducted using IBM SPSS Statistics for Windows (version 23.0) and R (version 4.3.1). P value less than 0.05 was considered statistically significant.
3 Results
3.1 Baseline characteristics
A total of 1770 groups of infertile couples were included in the study and analyzed after applying the inclusion and exclusion criteria. Out of these, 264 couples (14.9%) experienced failed fertilization, which was defined as a fertilization rate of less than 30%. The infertile couples were divided into a training set (n=1062) and a validation set (n=708) in a 6:4 ratio for constructing and testing the model. The basic characteristics, summarized in Table 1, showed no statistically significant difference in baseline characteristics between the two groups (P<0.05).
3.2 Logistic regression analysis
Table 2 displays the results of the univariate logistic regression analysis for fertilization failure. It reveals that in the failed fertilization group, the percentage of abnormal morphological sperm, P on the day of HCG trigger, oligoasthenozoospermia, teratozoospermia and the number of infertility years for couple significantly increased. On the other hand, the basal estrogen in females, PR%, sperm vitality, sperm concentration, number of oocytes retrieved, and the rate of mature oocytes significantly reduced the likelihood of fertilization failure. Variables with a P value <0.05 were considered statistically significant and included in the subsequent multivariate analysis.
The results of the multiple logistic regression analysis presented in Table 3. According to Table 3, the independent predictors of fertilization failure were infertility years (OR: 1.063, 95%CI: 1.002,1.126, P=0.038), the rate of mature oocytes (OR: 0.106, 95%CI:0.046,0.239, P<0.001), number of oocytes retrieved (OR: 0.939, 95%CI:0.906,0.971, P<0.001), basal estrogen level (OR: 0.991, 95%CI:0.984,0.998, P=0.015), oligoasthenozoospermia (OR: 1.512, 95%CI:1.013,2.241, P=0.041), sperm concentration (OR: 0.982, 95%CI: 0.971,0.993, P=0.001), sperm vitality (OR: 1.177, 95%CI: 1.086,1.282, P<0.001), percentage of abnormal morphological sperm (OR: 1.312, 95%CI: 1.122,1.543, P=0.001) and PR% value of male semen (OR: 0.821, 95%CI: 0.751,0.894, P<0.001).
3.3 Development and Validation of the clinical prediction model
The equations were constructed using regression coefficients to determine the probability of fertilization failure (P) = -24.78 + 0.061* Infertility years - 0.008 *level of female basic estrogen -2.25 *rate of mature oocytes -0.63* number of occytes retrieved- 0.018 * sperm concentration + 0.163 * sperm vitality - 0.197 * percentage of progressive motility (PR%) + 0.27 * percentage of abnormal morphological sperm + 0.413 * Oligoasthenozoospermia. To predict the probability of fertilization failure in conventional IVF assisted couples, we developed a nomogram (Figure 1), that includes of the above independent predictors. The area under the receiver operating characteristic curve (AUC) for the training set (Figure 2A) is 0.776 (95% CI: 0.740,0.812), indicating good clinical predictive ability. Similarly, the validation set (Figure 2B) has an AUC of 0.756 (95% CI: 0.708,0.805). The calibration curves for the training set (Figure 3A) and validation set (Figure 3B) have slopes of 1.000 and 0.891, respectively, indicating good calibration ability. Furthermore, the decision curve analysis of the training set (Figure 4A) and validation set (Figure 4B) demonstrates that the prediction model has high net income and clinical application value, as it is positioned higher on the decision curve.
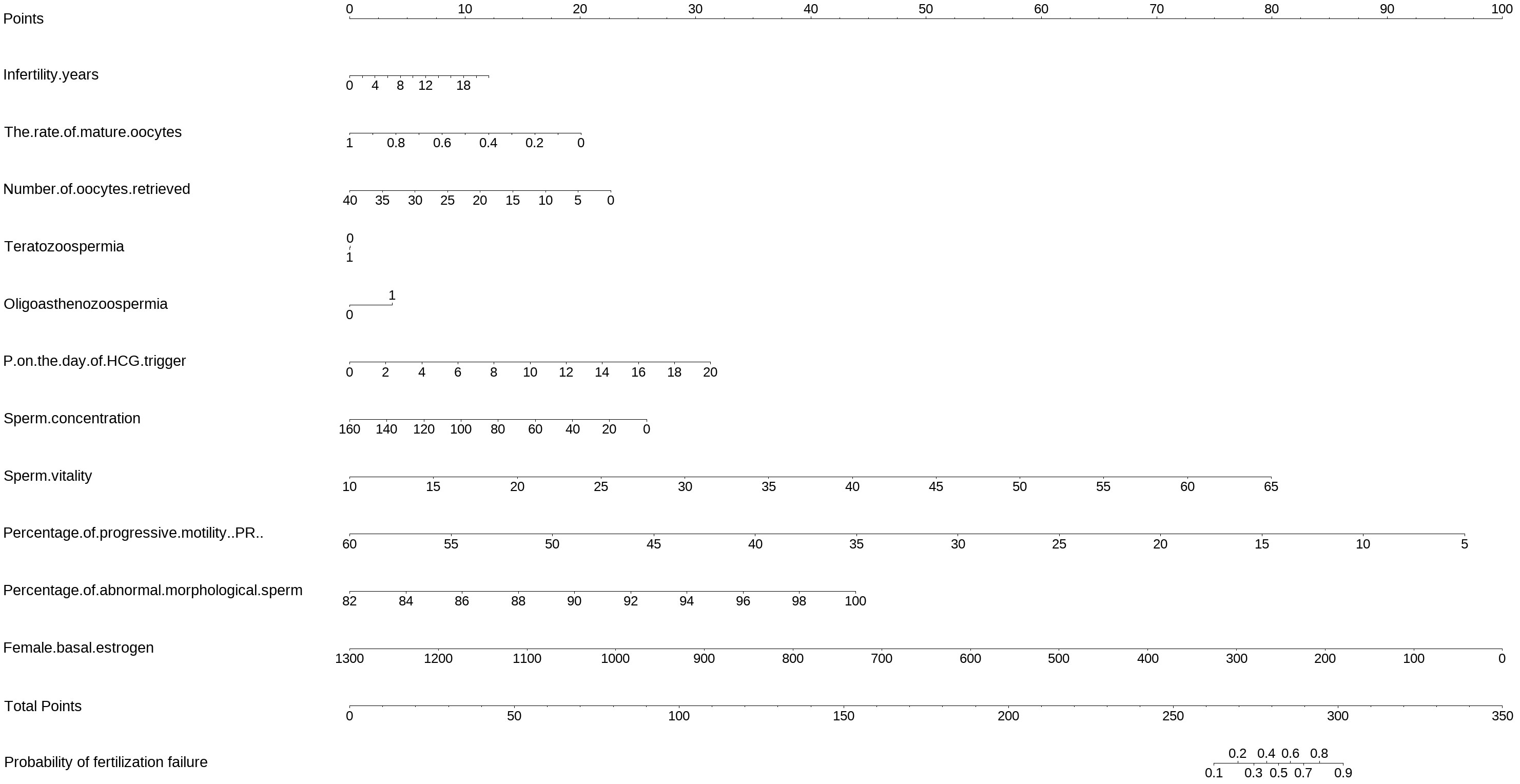
Figure 1 The nomogram to predict the probability of fertilization failure occurring in routine in-vitro fertilization (IVF) cycles. The nomogram can be applied by following procedures: draw a line perpendicular from the corresponding axis of each risk factor until it reaches the top line labeled “Points”; sum up the points for all risk factors and recorded as the total score; and draw a line descending from the axis labeled “Total points” until it intercepts the lower line to determine the probability of failed fertilization. The optimal threshold point was calculated using receiver operating characteristic (ROC) curve.
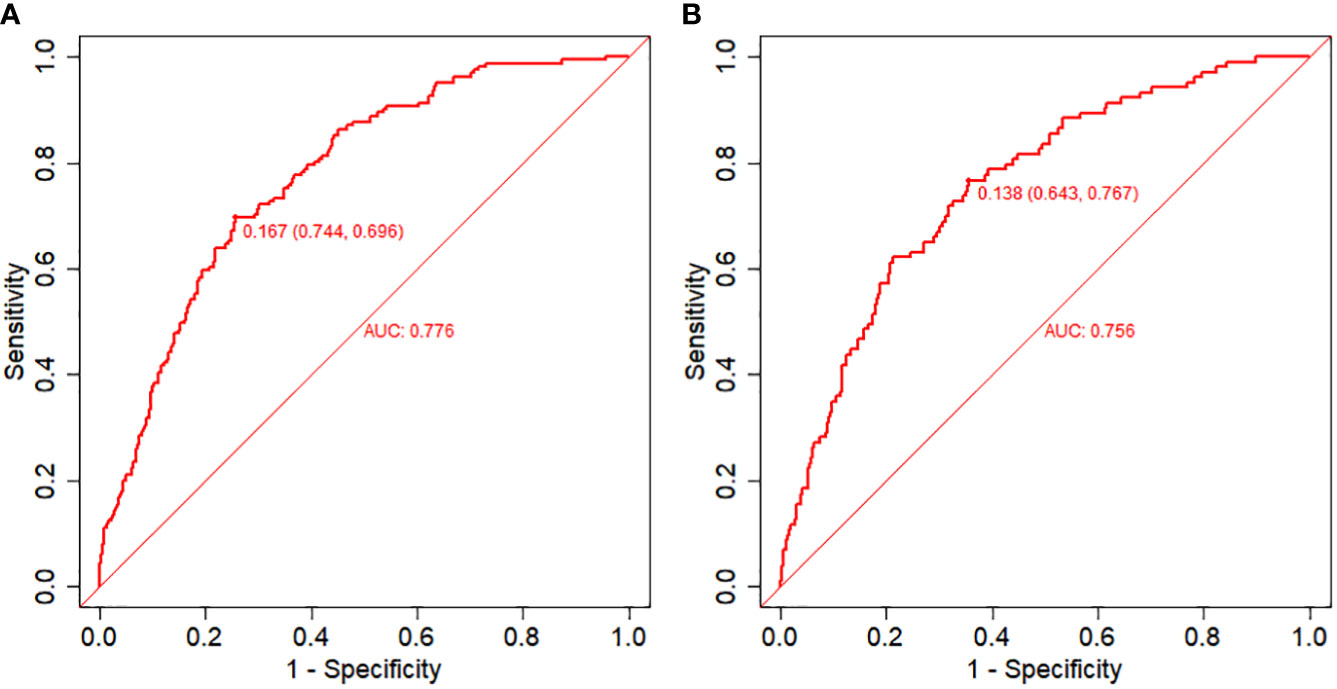
Figure 2 Receiver operating characteristic (ROC) curves and calibration plots of the training and validation sets. (A) Area under the ROC curve (AUC) of the training set is 0.776 (95% CI: 0.740,0.812). (B) AUC of the validation set is 0.756 (95% CI: 0.708,0.805). .
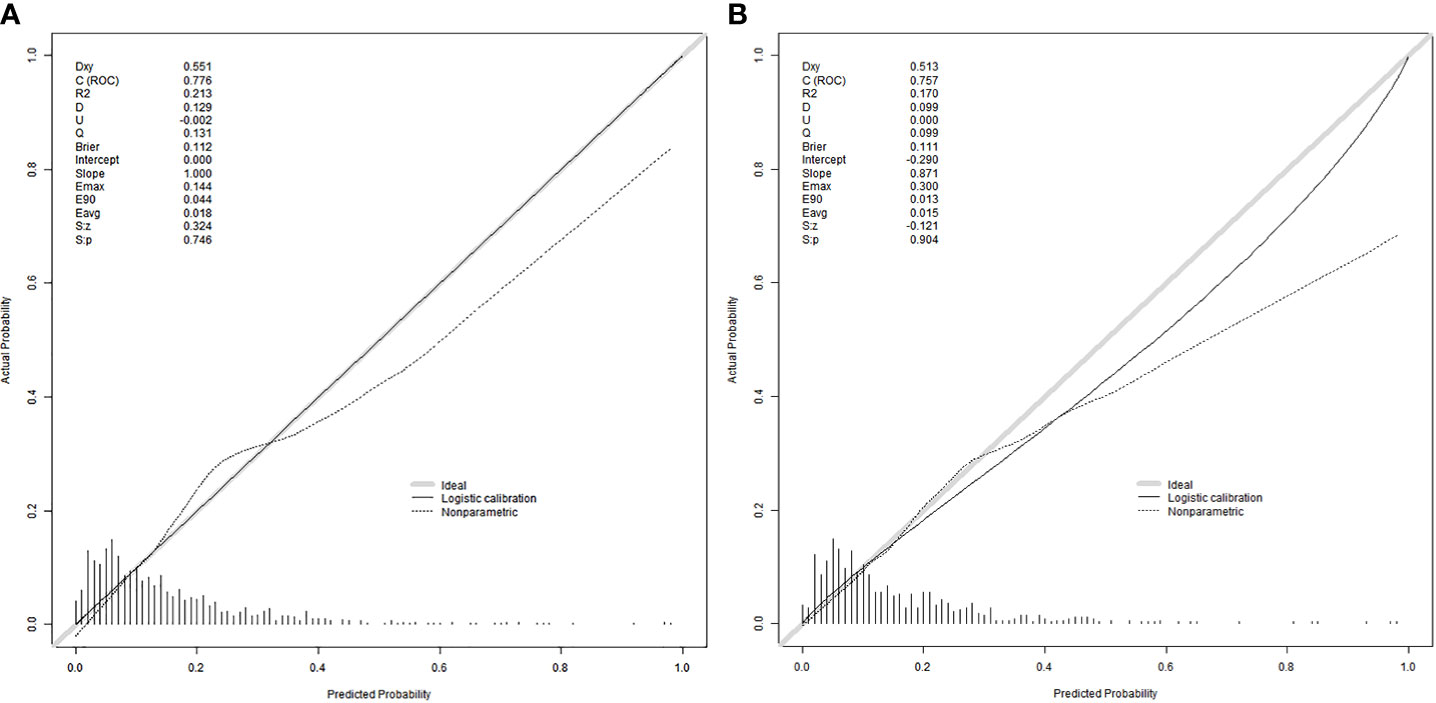
Figure 3 Calibration curves were used to evaluate the calibration of the model. The horizontal axis is the predicted probability provided by this model, and the vertical axis is the observed incidence of pregnancy failure. The ideal line with 45° slope represents a perfect prediction (the predicted probability equals the observed probability). The lower the Brier score for a set of predictions, the better the prediction calibration. When the slope was closer to 1.00, the prediction model had better calibration power. (A) Calibration curve for training set (Brier = 0.112, Slope = 1.000). (B) Calibration curve for validation set (Brier = 0.111, Slope = 0.871).
4 Discussion
4.1 Multivariate analysis affecting IVF fertilization failure
With the advancement of assisted reproductive technology, the successful implementation of IVF technology in clinical practice has brought hope to couples struggling with infertility. However, the success rate of in vitro fertilization is still not promising. Research has indicated that factors such as age (12), sperm quality (13) and oocyte quality play a crucial role in determining the success rate of IVF-ET.
This study is a retrospective analysis based on the assisted reproductive population. Infertile couples who underwent by conventional IVF and rescue ICSI were selected from the Department of Reproductive Medicine at the Fourth Hospital of Hebei Medical University, between June 2015 and June 2023. The objective of this study was to compare the basic information and semen parameters of husbands in failed and successful couples. The results revealed that several factors, including the percentage of progressive motility, sperm concentration, sperm vitality, percentage of abnormal morphological sperm, oligoasthenozoospermia, basal estrogen level in females, rate of mature oocytes, and duration of infertility, were identified as independent predictors of fertilization failure.
It has been suggested that fertilization failure may be attributed to disruptions in the interaction between sperm and oocytes at the level of the cumulus cell and oocyte zona pellucida (14). In this study, it is believed that the rate of sperm motility plays a crucial role in the success of fertilization, as there are varying rates of sperm survival and significant differences in the fertilization rate (15). Our study demonstrates that males with oligoasthenospermia or decreased sperm density, motility, and PR% have a significantly lower fertilization rate, which is consistent with previous findings (16, 17). Additionally, our study found that female factors also influence the likelihood of fertilization failure. Through the analysis in Table 3, it was observed that an increase in female baseline estrogen levels and the rate of mature oocytes decreased the probability of fertilization failure, aligning with previous research (18). We hypothesize that estrogen levels impact oocyte quality, and mature oocytes contribute to the successful binding of sperm and oocytes. Several studies have emphasized differences in the number of follicles, the total number of obtained oocytes, and the number of mature oocytes in the fertilization failure group (19). This could be linked to mechanisms such as meiotic spindle abnormalities in oocytes (20). Furthermore, this study revealed a significant difference in the number of years of infertility between the two groups, with a statistically significant distinction.
In the occurrence factors of infertility in China, 20-30% are caused by both couples. Semen parameters are important indicators used to evaluate male fertility and commonly employed in clinical practice. According to the WHO guidelines, important parameters for evaluating semen quality include the percentage of progressive motility, sperm concentration, sperm vitality, and the percentage of abnormal morphological sperm. The predictive value of these semen analysis parameters in forecasting the outcome of IVF fertilization has been established (21). Approximately 20% of IVF treatment cases are associated with a low fertilization rate or complete fertilization failure. Studies have indicated that fertilization failure is often linked to poor semen quality, specifically oligoasthenospermia, hypomospermia, and low sperm and oocyte union. However, the exact cause is still unclear (22, 23).
While sperm factors are the primary cause of fertilization disorders, oocyte abnormalities also contribute to the issue. Research has shown a correlation between the maturity and quality of the oocytes and the success rate of fertilization (24).
4.2 Development and validation of the nomogram prediction model
The successful combination of sperm and eggs marks the beginning of embryo formation and is a crucial step in in vitro fertilization embryo transfer. To assist clinical IVF in selecting appropriate insemination methods and guiding clinical work, we developed prediction models based on retrospective clinical studies, which include independent predictive factors that affect fertilization failure. In the field of reproductive medicine, there have been numerous previous studies on clinical prediction models of assisted reproductive technology (ART) for pregnancy, and most models have shown an area under the curve ranging between 0.59 and 0.8 (25). The area under the curve (AUC) of the modeling set in this study is 0.776, indicating a moderate predictive effect. An article published in JAMA in 2022 emphasizes calibration as an important indicator of predictive models, as it reflects their ability to accurately estimate absolute risk. The article also suggests the inclusion of a calibration curve in clinical papers on predictive models (26). For this study, we randomly allocated 60% of the samples to the modeling group and 40% to the validation group, and the model performed well in both groups. In recent years, the clinical application value of prediction models has been emphasized, with the evaluation mainly based on decision curve analysis, which assesses whether the model can benefit patients by influencing clinical decisions (27). We generated decision curves for both groups and demonstrated that the nomogram model yielded a substantial net benefit.
The occurrence of fertilization failure in routine IVF cycles is influenced by factors such as semen quality, ovarian function, egg quality, and maturity. However, the impact of these factors is still being investigated. Considering this limitation, our objective was to develop a clinical model using retrospective data to predict fertilization failure. This model aims to assist in selecting appropriate insemination methods, thus avoiding the wastage of eggs. Furthermore, there is potential to incorporate additional variables in future iterations of the model. Given the relatively low rate of fertilization failure in regular IVF cycles (approximately 5%-15%), we analyzed the final nomogram, which demonstrated a maximum net gain of approximately 15% according to the decision curve.
Furthermore, the clinical selection of appropriate insemination methods for infertile couples is a topic under active investigation. Our model uses internal verification of the results of the nomogram, without external verification, which is a significant limitation of this paper. We hope to address this limitation by continuing the multicenter retrospective study to improve the generalizability of the results. Nonetheless, our exploration offers valuable insights for future research. We aim to conduct more comprehensive and in-depth studies to develop a systematic and comprehensive clinical prediction model.
5 Conclusions
We discovered that factors such as female infertility duration, basal estrogen levels, rate of mature oocytes, number of oocytes retrieved, oligoasthenozoospermia, sperm concentration, sperm vitality, percentage of abnormal morphological sperm, and PR% independently predicted the likelihood of fertilization failure. Our retrospective study has developed a well-calibrated model that accurately predicts the probability of fertilization failure in infertile couples undergoing routine IVF treatment. This model carries significant clinical implications.
Data availability statement
The original contributions presented in the study are included in the article/Supplementary Material. Further inquiries can be directed to the corresponding author.
Ethics statement
The studies involving humans were approved by Ethics Committee of Reproductive Medicine Department, The Fourth Hospital, Hebei Medical University. The studies were conducted in accordance with the local legislation and institutional requirements. The participants provided their written informed consent to participate in this study.
Author contributions
ZN: Writing – review & editing. LX: Writing – original draft.
Funding
The author(s) declare that no financial support was received for the research, authorship, and/or publication of this article.
Acknowledgments
Acknowledgments my deepest gratitude goes to professor ZN, my supervisor, for her constant encouragement and guidance on writing this article. Thanks to the teachers and senior sisters in the department for their help.
Conflict of interest
The authors declare that the research was conducted in the absence of any commercial or financial relationships that could be construed as a potential conflict of interest.
Publisher’s note
All claims expressed in this article are solely those of the authors and do not necessarily represent those of their affiliated organizations, or those of the publisher, the editors and the reviewers. Any product that may be evaluated in this article, or claim that may be made by its manufacturer, is not guaranteed or endorsed by the publisher.
Supplementary material
The Supplementary Material for this article can be found online at: https://www.frontiersin.org/articles/10.3389/fendo.2023.1331640/full#supplementary-material
References
1. Zhu L, Xi Q, Nie R, Chen W, Zhang H, Li Y, et al. Rescue intracytoplasmic sperm injection: a prospective randomized study. J Reprod Med (2011) 56(9-10):410–4.
2. Kuczyński W, Dhont M, Grygoruk C, Pietrewicz P, Redzko S, Szamatowicz M, et al. Rescue ICSI of unfertilized oocytes after IVF. Hum Reprod (2002) 17(9):2423–7. doi: 10.1093/humrep/17.9.2423
3. Vanden Meerschaut F, Nikiforaki D, Heindryckx B, De Sutter P. Assisted oocyte activation following ICSI fertilization failure. Reprod BioMed ONLINE (2014) 28(5):560–71. doi: 10.1016/j.rbmo.2014.01.008
4. Liu DY, Baker HW. Defective sperm-zona pellucida interaction: a major cause of failure of fertilization in clinical in-vitro fertilization. Hum Reprod (2000) 15(3):702–8. doi: 10.1093/humrep/15.3.702
5. Krog M, Prior M, Carlsen E, Loft A, Forman J, Pinborg A, et al. Fertilization failure after IVF in 304 couples–a case-control study on predictors and long-term prognosis. Eur J Obstet Gyn R B (2014) 184:32–7. doi: 10.1016/j.ejogrb.2014.10.037
6. Morton PC, Yoder CS, Tucker MJ, Wright G, Brockman WD, Kort HI. Reinsemination by intracytoplasmic sperm injection of 1-day-old oocytes after complete conventional fertilization failure. Fertil Steril (1997) 68(3):488–91. doi: 10.1016/S0015-0282(97)00223-9
7. Esteves SC. Intracytoplasmic sperm injection versus conventional IVF. Lancet (2021) 397(10284):1521–3. doi: 10.1016/S0140-6736(21)00843-6
8. Montoya JM, Bernal A, Borrero C. Diagnostics in assisted human reproduction. Reprod BioMed ONLINE (2002) 5(2):198–210. doi: 10.1016/S1472-6483(10)61624-0
9. 陈子江. 石玉华.现代不孕症的诊断治疗及研究进展. 现代妇产科进展 (2004) 13(1):73–74,76. doi: 10.3969/j.issn.1004-7379.2004.01.038
10. 张春雨. 多精受精胚胎的发育潜能及临床应用价值研究. 中外女性健康研究 (2016) 17):163–164,186. doi: 10.3969/j.issn.2096-0417.2016.17.107
11. Steyerberg EW, Harrell FE, Borsboom GJ, Eijkemans MJ, Vergouwe Y, Habbema JD, et al. Internal validation of predictive models: efficiency of some procedures for logistic regression analysis. J Clin Epidemiol (2001) 54(8):774–81. doi: 10.1016/S0895-4356(01)00341-9
12. Sohn J, Jun J, Kim S, Ji KM, Mook LJ, Jin SH, et al. Does vitamin D deficiency affect the IVF-ET outcomes in infertile Korean women? Reprod BioMed Online (2018) 37:e4. doi: 10.1016/j.rbmo.2018.04.002
13. Matsukawa Y, Kanada Y, Takai S, Inoue S, Majima T, Funahashi Y, et al. Pre-treatment serum testosterone level can be a useful factor to predict the improvement in bladder outlet obstruction by tadalafil for male patients with lower urinary tract symptoms induced by benign prostatic obstruction. Aging Male (2019) 23(5):641–7. doi: 10.1080/13685538.2018.1563777
14. Coticchio G, Dal Canto M, Mignini Renzini M, Guglielmo MC, Brambillasca F, Turchi D, et al. Oocyte maturation: gamete-somatic cells interactions, meiotic resumption, cytoskeletal dynamics and cytoplasmic reorganization. Hum Reprod UPDATE (2015) 21(4):427–54. doi: 10.1093/humupd/dmv011
15. van der Westerlaken L, Helmerhorst F, Dieben S, Naaktgeboren N. Intracytoplasmic sperm injection as a treatment for unexplained total fertilization failure or low fertilization after conventional in vitro fertilization. Fertil Steril (2005) 83(3):612–7. doi: 10.1016/j.fertnstert.2004.08.029
16. Yang YS, Chen SU, Hwang JL, Ho HN, Lin HR, Lee TY. Analysis of human in vitro fertilization failure. J FORMOS Med Assoc (1993) 92(2):122–7.
17. Zhu J, Jiang H, He RB, Yin H-Q, Wang C-L, Li Y, et al. Association between etiologic factors in infertile couples and fertilization failure in conventional in vitro fertilization cycles. ANDROLOGY-US. (2015) 3(4):717–22. doi: 10.1111/andr.12048
18. Xia Q, Li Y, Zhang Y, Tian F, Zhang Q, Yao Z, et al. Identification of factors related to fertilization failure in in vitro fertilization-embryo transfer. Zhong Nan Da Xue Xue Bao Yi Xue Ban (2020) 45(8):960–5. doi: 10.11817/j.issn.1672-7347.2020.200076
19. Frapsauce C, Pionneau C, Bouley J, de Larouzière V, Berthaut I, Ravel C, et al. [Unexpected in vitro fertilization failure in patients with normal sperm: a proteomic analysis]. Gynecol Obstet Ferti (2009) 37(10):796–802. doi: 10.1016/j.gyobfe.2009.07.014
20. Combelles CM, Morozumi K, Yanagimachi R, Zhu L, Fox Janis H, Racowsky C. Diagnosing cellular defects in an unexplained case of total fertilization failure. Hum Reprod (2010) 25(7):1666–71. doi: 10.1093/humrep/deq064
21. Joshi N, Kodwany G, Balaiah D, Parikh M, Parikh F. The importance of computer-assisted semen analysis and sperm function testing in an IVF program. Int J FERTIL MENOP S (1996) 41(1):46–52.
22. Guzick DS, Overstreet JW, Factor-Litvak P, Brazil CK, Nakajima ST, Coutifaris C. Sperm morphology, motility, and concentration in fertile and infertile men. New Engl J Med (2001) 345(19):1388–93. doi: 10.1056/NEJMoa003005
23. Swain JE, Pool TB. ART failure: oocyte contributions to unsuccessful fertilization. Hum Reprod Update (2008) 14(5):431–46. doi: 10.1093/humupd/dmn025
24. Wessel GM, Conner SD, Berg L. Cortical granule translocation is microfilament mediated and linked to meiotic maturation in the sea urchin oocyte. Development (2002) 129(18):4315–25. doi: 10.1242/dev.129.18.4315
25. Curchoe CL, Flores-Saiffe Farias A, Mendizabal-Ruiz G, Chavez-Badiola A, et al. Evaluating predictive models in reproductive medicine. Fertil Steril (2020) 114(5):921–6. doi: 10.1016/j.fertnstert.2020.09.159
26. Alba AC, Agoritsas T, Walsh M, Hanna S, Iorio A, Devereaux PJ, et al. Discrimination and calibration of clinical prediction models: users' Guides to the medical literature. JAMA-J Am Med Assoc (2017) 318(14):1377–84. doi: 10.1001/jama.2017.12126
Keywords: in vitro fertilization, rescue ICSI, clinical prediction model, fertilization failure, nomogram
Citation: Xingnan L and Na Z (2024) Development and validation of a clinical prediction model of fertilization failure during routine IVF cycles. Front. Endocrinol. 14:1331640. doi: 10.3389/fendo.2023.1331640
Received: 01 November 2023; Accepted: 26 December 2023;
Published: 19 January 2024.
Edited by:
Jan Tesarik, MARGen Clinic, SpainReviewed by:
Chong Liu, Huazhong University of Science and Technology, ChinaGuilan Xie, Peking University, China
Copyright © 2024 Xingnan and Na. This is an open-access article distributed under the terms of the Creative Commons Attribution License (CC BY). The use, distribution or reproduction in other forums is permitted, provided the original author(s) and the copyright owner(s) are credited and that the original publication in this journal is cited, in accordance with accepted academic practice. No use, distribution or reproduction is permitted which does not comply with these terms.
*Correspondence: Zhang Na, MTM4MzMxMTY4MDZAMTYzLmNvbQ==