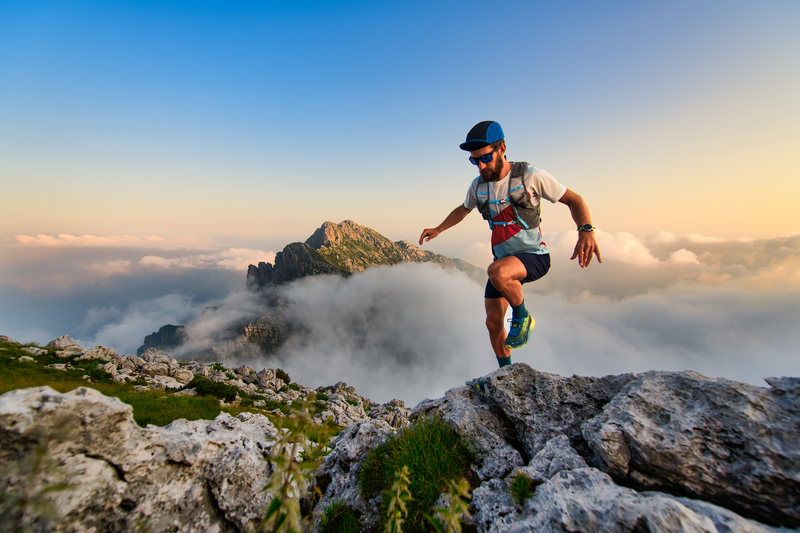
95% of researchers rate our articles as excellent or good
Learn more about the work of our research integrity team to safeguard the quality of each article we publish.
Find out more
EDITORIAL article
Front. Endocrinol. , 18 December 2023
Sec. Systems Endocrinology
Volume 14 - 2023 | https://doi.org/10.3389/fendo.2023.1305897
This article is part of the Research Topic Machine Learning-Assisted Diagnosis and Treatment of Endocrine-Related Diseases View all 13 articles
Editorial on the Research Topic
Machine learning-assisted diagnosis and treatment of endocrine-related diseases
The endocrine system is an important regulatory system in the human body. It regulates physiological processes such as growth, development, metabolism, and reproduction through the secretion, transfer, and feedback of hormones. Various factors, including environmental, genetic, and lifestyle factors, can lead to the development of endocrine diseases associated with dysfunction of the pituitary, adrenal, and thyroid glands, as well as diabetes. Changes in living environments and lifestyles have led to steady increases in the incidence of endocrine diseases. If not detected and treated in time, these can lead to complications and cause irreversible damage to health.
The application of machine learning in medical fields has great prospects (1). This Research Topic is the use of machine learning to establish models for the prediction, diagnosis, and management of endocrine-related diseases for the benefit of both patients and clinical practice. The following is a brief overview of some of the included articles:
The model built by Wang et al. using the Gaussian Naive Bayes (GNB) algorithm allows improved prediction of prolonged hospitalization of patients following acute ischemic stroke (AIS). This will assist in policy adjustments for improved resource utilization, thereby alleviating the increasingly heavy economic burden caused by AIS.
Chen et al. used a two-sample Mendelian randomization (MR) study to explore the relationship between thyroid function and cholelithiasis. Their findings showed that low-density lipoprotein cholesterol (LDL-C) and apolipoprotein B mediated the effects of FT4 on cholelithiasis risk, with patients with high FT4 levels showing delayed or reduced risk of the long-term effects of cholelithiasis.
Zeng et al. analyzed data from the Genomics of Drug Sensitivity in Cancer (GDSC) and The Cancer Genome Atlas (TCGA) databases, using Spearman correlation analysis to identify the key genes that influence Gemcitabine (GEM) efficacy. It was found that CALB2 and GPX3 could be used as biomarkers for prognosis prediction in some forms of colorectal cancer (CRC) as well as potential target genes of GEM, providing new ideas for the development of new combined targeted drugs for colorectal cancer.
Liu et al. established a machine learning-based choice for some type 2 diabetic kidney disease(T2DKD) risk prediction model based on clinical data from a multi-center retrospective database and verified its effectiveness. While the model was found to be helpful for the diagnosis of T2DKD, further investigation using additional data is required.
Huang et al. used the ESTIMATE algorithm to conduct a series of bioinformatics analyses on breast cancer (BC) samples from the TCGA database to identify genes associated with the tumor microenvironment (TME). The authors found a significant correlation between KLRB1 and the BC TME, suggesting its use as a prognostic marker and therapeutic target, providing a new direction for the treatment of BC.
Li et al. identified stratified prognostic biomarkers for serous ovarian cancer (SOC) after investigation of immune infiltration, drug sensitivity, and genes associated with the epithelial-mesenchymal transition (EMT). This lays a foundation for in-depth investigation of the role of the EMT in SOC immune regulation and changes in related pathways. It also suggests effective solutions for the early diagnosis and clinical treatment of ovarian cancer.
Xu et al. identified the C11 cluster that specifically expresses HSPB6 in fibroblasts as key to the development of pancreatic adenocarcinoma (PAAD). The authors used comprehensive bioinformatics analyses and constructed a nine-gene prognostic model using tumor-related PAAD prognostic genes in the C11 subgroup. The RiskScore may have reliable clinical potential for the prognostic prediction of PAAD.
With the increased informatization of society and further accumulation of data, the use of machine learning will become more common in medical applications requiring the processing of large amounts of data. Machine learning can process large amounts of data that humans cannot handle and is a powerful and versatile tool for future medicine (2). The application of machine learning in the diagnosis and treatment of endocrine diseases will become increasingly widespread and mature, as is currently seen in the prediction, diagnosis, and management of diseases such as diabetes (3), thyroid disease (4), and neuroendocrine tumors (5).
HZ: Formal analysis, Investigation, Methodology, Resources, Writing – original draft. UK: Conceptualization, Data curation, Project administration, Resources, Writing – review & editing. WS: Conceptualization, Investigation, Methodology, Project administration, Resources, Supervision, Writing – review & editing.
The authors declare that the research was conducted in the absence of any commercial or financial relationships that could be construed as a potential conflict of interest.
All claims expressed in this article are solely those of the authors and do not necessarily represent those of their affiliated organizations, or those of the publisher, the editors and the reviewers. Any product that may be evaluated in this article, or claim that may be made by its manufacturer, is not guaranteed or endorsed by the publisher.
1. Handelman GS, Kok HK, Chandra RV, Razavi AH, Lee MJ, Asadi H. eDoctor: machine learning and the future of medicine. J Intern Med (2018) 284(6):603–19. doi: 10.1111/joim.12822
2. Komuro J, Kusumoto D, Hashimoto H, Yuasa S. Machine learning in cardiology: Clinical application and basic research. J Cardiol (2023) 82(2):128–33. doi: 10.1016/j.jjcc.2023.04.020
3. Afsaneh E, Sharifdini A, Ghazzaghi H, Ghobadi MZ. Recent applications of machine learning and deep learning models in the prediction, diagnosis, and management of diabetes: a comprehensive review. Diabetol Metab Syndr (2022) 14(1):196. doi: 10.1186/s13098-022-00969-9
4. Cao CL, Li QL, Tong J, Shi LN, Li WX, Xu Y, et al. Artificial intelligence in thyroid ultrasound. Front Oncol (2023) 13:1060702. doi: 10.3389/fonc.2023.1060702
Keywords: machine learning, endocrine disorders, diagnosis, treatment, artificial intelligence
Citation: Zhang H, Kahlert UD and Shi W (2023) Editorial: Machine learning-assisted diagnosis and treatment of endocrine-related diseases. Front. Endocrinol. 14:1305897. doi: 10.3389/fendo.2023.1305897
Received: 02 October 2023; Accepted: 06 December 2023;
Published: 18 December 2023.
Edited and Reviewed by:
Tom Michoel, University of Bergen, NorwayCopyright © 2023 Zhang, Kahlert and Shi. This is an open-access article distributed under the terms of the Creative Commons Attribution License (CC BY). The use, distribution or reproduction in other forums is permitted, provided the original author(s) and the copyright owner(s) are credited and that the original publication in this journal is cited, in accordance with accepted academic practice. No use, distribution or reproduction is permitted which does not comply with these terms.
*Correspondence: Ulf D. Kahlert, dWxmLmthaGxlcnRAbWVkLm92Z3UuZGU=; Wenjie Shi, d2VuamllLnNoaUBvdmd1LmRl
Disclaimer: All claims expressed in this article are solely those of the authors and do not necessarily represent those of their affiliated organizations, or those of the publisher, the editors and the reviewers. Any product that may be evaluated in this article or claim that may be made by its manufacturer is not guaranteed or endorsed by the publisher.
Research integrity at Frontiers
Learn more about the work of our research integrity team to safeguard the quality of each article we publish.