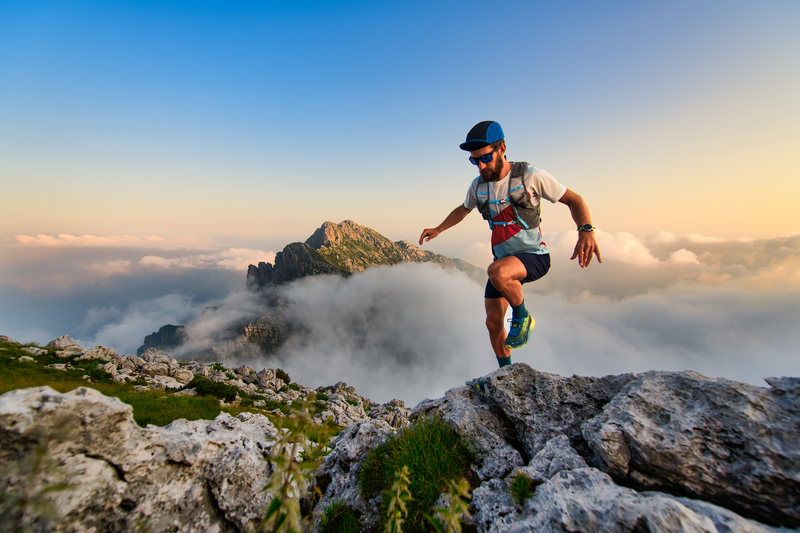
94% of researchers rate our articles as excellent or good
Learn more about the work of our research integrity team to safeguard the quality of each article we publish.
Find out more
ORIGINAL RESEARCH article
Front. Endocrinol. , 19 December 2023
Sec. Diabetes: Molecular Mechanisms
Volume 14 - 2023 | https://doi.org/10.3389/fendo.2023.1293124
Introduction: Type 2 diabetes (T2D) is a multifactorial disease involving genetic and environmental components. Several genome-wide association studies (GWAS) have been conducted to decipher potential genetic aberrations promoting the onset of this metabolic disorder. These GWAS have identified over 400 associated variants, mostly in the intronic or intergenic regions. Recently, a growing number of exome genotyping or exome sequencing experiments have identified coding variants associated with T2D. Such studies were mainly conducted in European populations, and the few candidate-gene replication studies in North African populations revealed inconsistent results. In the present study, we aimed to discover the coding genetic etiology of T2D in the Tunisian population.
Methods: We carried out a pilot Exome Wide Association Study (EWAS) on 50 Tunisian individuals. Single variant analysis was performed as implemented in PLINK on potentially deleterious coding variants. Subsequently, we applied gene-based and gene-set analyses using MAGMA software to identify genes and pathways associated with T2D. Potential signals were further replicated in an existing large in-silico dataset, involving up to 177116 European individuals.
Results: Our analysis revealed, for the first time, promising associations between T2D and variations in MYORG gene, implicated in the skeletal muscle fiber development. Gene-set analysis identified two candidate pathways having nominal associations with T2D in our study samples, namely the positive regulation of neuron apoptotic process and the regulation of mucus secretion. These two pathways are implicated in the neurogenerative alterations and in the inflammatory mechanisms of metabolic diseases. In addition, replication analysis revealed nominal associations of the regulation of beta-cell development and the regulation of peptidase activity pathways with T2D, both in the Tunisian subjects and in the European in-silico dataset.
Conclusions: The present study is the first EWAS to investigate the impact of single genetic variants and their aggregate effects on T2D risk in Africa. The promising disease markers, revealed by our pilot EWAS, will promote the understanding of the T2D pathophysiology in North Africa as well as the discovery of potential treatments.
Type 2 diabetes (T2D) is a multifactorial disease involving genetic and environmental components (1). A large number of Genome-Wide Association Studies (GWAS) have been carried out to investigate its genetic basis. These studies, performed mostly in Europeans, identified more than 240 susceptibility loci comprising over 400 association signals with T2D (2–4). Despite these findings, the understanding of the biological mechanisms of this disease is still limited. In fact, GWAS-derived SNPs always have small effects on complex traits as they can be in high linkage disequilibrium (LD) with the real causal variants. In addition, most of them are located within intronic and intergenic regions, which makes the interpretation of their roles very difficult (5).
In order to overcome these problems, advances in genome sequencing have enabled the investigation of coding variants, providing further functional insights of disease mechanisms. In this context, recent exome genotyping chips and whole exome sequencing (WES) based studies have identified coding variants associated with T2D (6–13).
Many studies have reported differences in LD structure, minor allele frequencies (MAF), as well as effect sizes of several T2D susceptibility variants between different ethnicities (14). Therefore, conducting association studies in underrepresented populations is crucial to uncover novel insights about genetic diseases (15). For instance, Barbitoff et al. identified five susceptibility loci for T2D in the Russian population upon analysis of exome sequencing data of 89 individuals (49 patients with T2D and 40 controls) (6).
T2D is a public health problem in Tunisia, with a prevalence estimation of 23% according to the last national epidemiological study (16). This prevalence is predicted to reach 26.6% by 2027 (17). Hence, urgent measures are needed to better understand population specific risk factors of T2D and to alleviate its burden.
Here, we conducted the first pilot Exome Wide Association study (EWAS) on T2D in the Tunisian population. Our aims were: 1) to identify novel genetic loci associated to T2D in Tunisian subjects; 2) to study the aggregate effects of variants and genes on the predisposition to T2D; 3) to replicate these analyses in a large European in-silico dataset.
This study was carried out in the frame of the ACIP 2017_45 project. The Institutional Review Board at Institut Pasteur of Tunis approved the study (reference number: 2018/08/I/LR11IPT05/V2). It was conducted in accordance with the declaration of Helsinki.
A total of 50 subjects (33 cases with T2D and 17 controls), aged between 35 and 65 years old, were enrolled in the study. T2D was diagnosed based on the American Diabetes Association criteria, including fasting plasma glucose (FPG) ≥ 1,26 g/l and/or glycated hemoglobin A1C (HBA1c) ≥ 6.5% (18). After obtaining written informed consents, all patients were interviewed to obtain their family history of diseases, as well as anthropometric (weight, height, body mass index (BMI=weight (Kg)/height2(m2))) and clinical data. Subsequently, blood samples were collected for biochemical and molecular analyses.
DNA samples of all participants were extracted using the Flexigen DNA kit (QIAGEN). The quantity and the quality of the extracted DNA were measured using the DENOVIX DS-11 nanodrop spectrophotometer. Then, WES was performed at Macrogen (South Korea). Briefly, exome capture was carried out using Agilent SureSelect V6, and massive parallel sequencing was then performed on an Illumina Novaseq 6000 system to generate 151-bp paired-end reads.
Quality control of the raw sequencing data was performed using the FastQC program (http://www.bioinformatics.babraham.ac.uk/projects/fastqc) with default settings. Then, paired-end reads were mapped to the hg38 human reference genome, and the resulting alignments were prepared for variant calling according to the GATK best practices. Subsequently, genetic variants were called using GATK in a single merged VCF file, followed by an application of variant score quality recalibration using the GATK VQSR algorithm (19). Finally, we used Annovar, implemented in Varaft tool, to annotate the variants for all the samples in the merged VCF file (20, 21). The variants were assigned to genes and functionally annotated with allele frequencies in 1000 Genomes project (https://www.internationalgenome.org/) and GnomAD (https://gnomad.broadinstitute.org/) (22, 23). In addition, we assessed the effects of non-synonymous variants by 12 pathogenicity prediction algorithms: SIFT (24), Polyphen HDIV (25), MutationTaster (26), Mutation Assessor (27), PROVEAN (28), MetaSVM, MetaLR (29), LRT (30), FATHMM (31), DANN (32), CADD (33) and VEST3 (34).
In the present study, we focused on autosomal coding variants, including non-synonymous, stop-gain, stop-loss variants and frameshift indels. The impact of the non-synonymous genetic variants was assessed by an approach similar to that developed by Pezzilli et al. (35). It comprises 12 pathogenicity-prediction software packages, that were chosen because of their performance and high classification records (36, 37). The scores generated by these packages were binarized to 1 when the following conditions were met, and to 0 otherwise: SIFT score < 0.05, PolyPhen2 HDIV score > 0.453, FATHMM score < 0, MetaLR score > 0.5, MetaSVM score > 0, DANN score > 0.8, VEST3 score > 0.75, CADD score > 20, PROVEAN score < -2.5, Mutation Assessor score > 1.9, LRT=D and Mutation Taster=A or D. Finally, we summed the single 12 binary scores to get a total pathogenicity score for each non-synonymous variant. The genetic variants with a total pathogenicity score > 6 were filtered in for further investigation.
In addition, we excluded variants with call rate < 98% as well as those that deviated from the Hardy-Weinberg equilibrium (P < 10−6).
We performed single-variant analysis (SVA), using a logistic regression model with adjustment for age, as implemented in PLINK 1.9 (https://www.cog-genomics.org/plink/1.9/) (38). To do, we incorporated the ages of the 50 participants as a covariate into the model. Statistical significance was set using Bonferroni correction at 4 × 10-6 (0.05/12516 variants tested, after surviving the filtration process). Manhattan plot was generated using CMplot R package (39).
In order to increase the statistical power, we applied gene and pathway-based tests which combine associations within genes and gene-sets, respectively.
Gene and pathway analyses were conducted using the Multimarker Analysis on GenoMic Annotation (MAGMA) v1.10 software package (40), in a three-step analytic process. First, we mapped the SNPs to genes based on the NCBI 38 human gene reference build. Second, SNP-based EWAS summary statistics, generated by PLINK 1.9, served as input for MAGMA to perform gene-based association analysis. Bonferroni correction was applied for multiple testing correction (p-value = 0.05/2263 genes= 2.2 x 10-5). Finally, a competitive gene-set analysis was applied to test for the association of biological pathways with T2D. We used two collections of predefined gene-sets. The first collection was downloaded from the MsigDB v2022.1.Hs database, including Gene Ontology (GO) biological processes, KEGG and Reactome canonical pathways (41, 42). Significant pathways were called with p-value of 8.99 x 10-6 (0.05/5557 gene-sets), according to Bonferroni correction for multiple testing. The second collection consisted of 16 sets of candidate T2D-relevant genes, that were curated by Flannick et al. on their EWAS carried out on 2019 (10).
We carried out a replication study using the summary statistics of the European ExTexT2D in-silico dataset, downloaded from the DIAGRAM consortium (http://diagram-consortium.org/downloads.html). This dataset consists of ExomeChip meta-analysis of T2D in Europeans, involving up to 177116 individuals, that was previously published in Mahajan et al. (43). We selected a European replication dataset due to the close genetic relationship between Tunisians and Europeans. In fact, previous studies showed that the genetic landscape of the Tunisian population is a mosaic of Eurasian and North African lineages (44–47). This observation may be explained by the migratory waves that occurred in the Mediterranean region since the prehistoric period (44). Gene and pathway-based association p-values were computed with MAGMA using the same code employed in the analysis on the Tunisian subjects. The flowchart of this study is shown in Figure 1.
Baseline characteristics of our study participants are summarized in Table 1. BMI, fasting plasma glucose and HBA1c were significantly higher in T2D cases compared to controls.
A total of 12694 variants passed the quality control and variant filtration process to undergo statistical analyses. The Manhattan plot showed that none of the variants reached the exome-significance level (Figure 2). The highest peak has an association signal below a nominal threshold of 1 x 10-2. This signal stands for the stop-gain variant rs4879782:c.69C>G/p.Tyr23Ter, located in the MYORG (Myogenesis regulating glycosidase) gene, with a p-value equal to 9.58 x 10-3. However, this signal was not observed in our replication analysis performed on the European in-silico exome-chip dataset. Summary statistics of SVA are provided as Supplementary Table 1.
Figure 2 Manhattan plot of single variant analysis. The orange continuous line corresponds to the exome wide significance threshold for single variant analysis (4 x 10-6), while the green dashed line corresponds to a nominal association threshold (1 x 10-2). The promising variant is highlighted in red.
Gene-based analysis using MAGMA, revealed no signals that reached exome-wide significance (p-value < 1.47 x 10-5). The top most associated gene was MYORG (p-value=9.58 x 10-3), as it was the case with the SVA results (Table 2; Figure 3).
Figure 3 Manhattan plot of the gene-based analysis. The orange continuous line corresponds to the exome wide significance threshold for gene-based analysis (2.2 x 10-5), while the green dashed line corresponds to a nominal association threshold (2 x 10-3). The promising gene is highlighted in red.
We identified two nominal associations of two gene sets from the Gene Ontology database in our study samples (p-value < 2 x 10-3). They consist of the positive regulation of neuron apoptotic process and the regulation of mucus secretion (Table 3). In addition, our EWAS identified two pathways having nominal association with T2D (p-value < 1 x 10-2), both in our study samples and in the European replication dataset (Table 4).
Table 3 Two candidate pathways that exhibited nominal association with type 2 diabetes in the present study.
Table 4 Two candidate pathways that exhibited significant association with type 2 diabetes in the present study and in the replication in-silico dataset.
Regarding pathway analysis using the known T2D-relevant gene-sets, there was not a significant association (all p-values > 0.05).
The clinical heterogeneity of T2D and the development of multisystemic complications suggest that multiple genetic variations are involved in the manifestation of the disease phenotype.
In the present study, we performed a pilot EWAS to interrogate the association of variants, genes and pathways with T2D in Tunisia. The key findings of our study are as follows: 1) single variant and gene-based analyses revealed a promising association signal in MYORG gene, although not reaching exome wide significance threshold, 2) gene-set analysis identified two pathways with nominal association to T2D: positive regulation of neuron apoptotic process, and regulation of mucus secretion. However, these pathways’ associations were not replicated in an independent European in-silico exome chip dataset comprising up to 177116 individuals. In addition, our EWAS identified two pathways with nominal association to T2D, both in our study samples and in the European replication dataset.
To date, the majority of high throughput association studies of T2D have been performed in Europeans. There were only a few candidate gene studies of T2D in North African populations, and they yielded conflicting results. For instance, Cauchi et al. reported that 10 among 37 validated European loci were associated with T2D in the Tunisian population (48).
In our pilot EWAS, the SVA and gene-based analysis did not reveal any significant association with T2D in the Tunisian study population. However, they both revealed a promising stop-gain variant, rs4879782, located in the MYORG gene. This variant was not previously associated with a clinical condition. On the cellular level, MYORG encodes a glycosyl hydrolase, hydrolyzing O-glycosyl compounds in the endoplasmic reticulum. Interestingly, it was reported that MYORG is involved in the fiber development of skeletal muscle, the major site for postprandial glucose uptake from the blood circulation (49). Progressive loss of muscle mass is associated with impairment of its roles in regulating glucose homeostasis, which was shown to contribute to the pathogenesis of T2D (50, 51). Based on these observations, further studies are warranted to examine the relationships between MYORG mutations and the glucose metabolism.
For pathway-based analysis, we conducted a competitive, regression-based test as implemented by MAGMA (40), to investigate the enrichment of genes associated with T2D.
It is known that T2D is a polygenic trait that is influenced by hundreds of variants across many genes (52). Hence, any random combination of genes is likely to be associated with T2D. We opted for competitive tests that compare the association with T2D of genes in a specific gene-set against genes that are not in this specific gene-set.
Pathway association analysis revealed nominal associations between T2D and two pathways. The first association was with the “positive regulation of neuron apoptotic process”. This pathway is implicated in the maintenance of the neuronal system in adult life by the tight regulation of apoptotic mechanisms. However, excessive activation of this process could lead to pathological conditions such as neurodegenerative diseases (53). Neuronal apoptosis can be triggered through mitochondrial pathways, including the release of caspase proteins or activation of death receptors by the tumor necrosis factor receptor (54). In this context, a recent study has demonstrated excessive activation of caspase and tumor necrosis factor-alpha in T2D rat brains (55). Moreover, it has been shown that apoptosis can be induced by oxidative stress and inflammatory mechanisms triggered by insulin resistance, leading to neurodegenerative diseases such as ischemic stroke and vascular dementia (56, 57). These observations emphasize the relevance of conducting further studies on the effects of genetic alterations in this pathway on the development of T2D neurodegenerative complications. The second association was with the “regulation of mucus secretion” pathway. Intestinal mucus forms a highly organized protective barrier against harmful substances (58). Under perturbed conditions such as infections, pathogens can disrupt the mucus barrier, which facilitates the translocation of microbial products to the epithelial surface. This can cause inflammatory response, which sets favorable conditions for the development of metabolic diseases such as diabetes (59). In addition, a recent study demonstrated that hyperglycemia alters the intestinal barrier, which results in a systemic influx of microbial products, leading to inflammatory consequences of diabetes and obesity (60). In perspective, further studies are needed to decipher the links between the regulation of the gut mucus secretion and the development of metabolic disorders.
Besides these two pathways, MAGMA analysis identified two pathways that showed nominal association (p-value < 1 x 10 -2) with T2D pathogenesis in Tunisians and in a European replication dataset. The first identified pathway was the regulation of beta-cell development. It is known that pancreatic beta-cells ensure the production, the storage and the secretion of the insulin used by the organism to maintain normoglycemia (61). Changes in nutrient state affect the insulin secretory response according to an interplay between extracellular signals and beta cell-specific transcriptional programs. Defects in this interplay process will alter the beta-cells function, leading to a relative insufficiency of insulin blood levels, which is characteristic of T2D (62). Thus, it is undeniable that a deeper understanding of beta-cell transcriptional programs and their associated signals will have direct implications for diabetes therapies. The second pathway was the regulation of peptidase activity. The most known peptidase that affects glucose metabolism is the dipeptidyl peptidase-4 (DPP-4). This protein inhibits the glucagon-like-peptide-1, an incretin expressed in the post-prandial phase in order to stimulate insulin secretion (63). Interestingly, it has been reported that obese and type 2 diabetic subjects have high circulating DPP-4 levels (64). Moreover, it has been proposed that DPP-4 triggers inflammation and insulin resistance in adipose and hepatic tissue (65, 66). These observations promoted the development of DPP-4 inhibitors, used nowadays as oral anti-diabetic medications. However, the overall significance of DPP-4 activity for the regulation of glucose homeostasis remains to be resolved.
A potential limitation of our study resides in the relatively small sample size of the discovery cohort. This limitation may induce a lack of sufficient statistical power to detect all associations. To overcome this limitation, we applied a three-step approach in order to reduce the number of statistical tests, and thus increase the statistical power. First, we prioritized candidate markers that potentially damage protein’s structure and function. Second, we applied gene-based and pathway-based analyses in order to test the aggregate effects of combinations of genetic variants on the regulation of the glycemic homeostasis. Finally, we replicated our analyses in a large existing in-silico dataset. Overall, our strategy led to identify nominal variant and gene associations, as well as biologically relevant pathways associated with T2D in the Tunisian population. Therefore, it seems suitable to discover candidate disease markers in underrepresented populations.
In summary, the present study is the first EWAS on T2D in Africa. Variant and gene-based analyses highlighted changes in MYORG gene as new promising markers of T2D in Tunisians, although not reaching exome wide significance. Further gene-set analysis highlighted the potential role of the positive regulation of neuron apoptotic process, the regulation of mucus secretion, beta-cell development and peptidase activity pathways in T2D development and/or progression. These candidate loci and pathways will promote the understanding of T2D pathophysiology in North-African populations. Moreover, they may be the targets for functional studies to discover potential treatments for T2D.
The data presented in the study are deposited in the European Variation Archive (EVA) repository, accession number PRJEB70960. Publicly available datasets were analyzed in this study. This data can be found here: http://diagram-consortium.org/downloads.html.
The studies involving humans were approved by Comité d’éthique Bio-Médicale de l’Institut Pasteur de Tunis. The studies were conducted in accordance with the local legislation and institutional requirements. The participants provided their written informed consent to participate in this study.
HD: Conceptualization, Data curation, Formal Analysis, Investigation, Methodology, Resources, Software, Validation, Visualization, Writing – original draft. WB: Data curation, Formal Analysis, Methodology, Resources, Validation, Writing – review & editing. NK: Data curation, Methodology, Resources, Validation, Writing – review & editing. MF: Methodology, Resources, Validation, Writing – review & editing. HJ: Data curation, Methodology, Resources, Validation, Writing – review & editing. MH: Data curation, Methodology, Resources, Validation, Writing – review & editing. IG: Data curation, Methodology, Resources, Validation, Writing – review & editing. MG: Data curation, Methodology, Resources, Validation, Writing – review & editing. WK: Data curation, Methodology, Resources, Validation, Writing – review & editing. MM: Methodology, Validation, Writing – review & editing. MT: Methodology, Validation, Writing – review & editing. AK: Methodology, Validation, Writing – review & editing. HT: Methodology, Validation, Writing – review & editing. AB: Methodology, Validation, Writing – review & editing. HJ: Investigation, Methodology, Resources, Validation, Writing – review & editing. OM: Validation, Writing – review & editing. AA: Investigation, Validation, Writing – review & editing. RK: Conceptualization, Funding acquisition, Investigation, Project administration, Resources, Supervision, Validation, Writing – review & editing.
The author(s) declare financial support was received for the research, authorship, and/or publication of this article. This study was funded by the Institut Pasteur International Network in the frame of the “Actions Concertées InterPasteuriennes” (ACIP 45-17), the Tunisian Ministry of Higher Education and Scientific Research (LR16IPT05) and the Tunisian Ministry of Health. Hamza Dallali, Nadia Kheriji and Haifa Jmel are recipients of MOBIDOC fellowships, funded by The Tunisian Ministry of Higher Education and Scientific Research through the PromEssE project and managed by the ANPR.
We thank the study participants for their collaboration. We acknowledge Ahmed S. Chakroun from RAN BioLinks (Tunis, Tunisia) for his assistance in the shipment of DNA samples as well as the gathering of the raw data from the sequencing platform at Macrogen, Inc. (Seoul, South Korea). The authors thank the Clinical Core and the Department for Scientific Programming and Incentive Actions (SPAIS) of the Institut Pasteur (Paris, France) for their support in the implementation of the ACIP 45-17 project.
The authors declare that the research was conducted in the absence of any commercial or financial relationships that could be construed as a potential conflict of interest.
All claims expressed in this article are solely those of the authors and do not necessarily represent those of their affiliated organizations, or those of the publisher, the editors and the reviewers. Any product that may be evaluated in this article, or claim that may be made by its manufacturer, is not guaranteed or endorsed by the publisher.
The Supplementary Material for this article can be found online at: https://www.frontiersin.org/articles/10.3389/fendo.2023.1293124/full#supplementary-material
1. McCarthy MI. Painting a new picture of personalised medicine for diabetes. Diabetologia (2017) 60:793–9. doi: 10.1007/S00125-017-4210-X/FIGURES/1
2. Mahajan A, Taliun D, Thurner M, Robertson NR, Torres JM, Rayner NW, et al. Fine-mapping type 2 diabetes loci to single-variant resolution using high-density imputation and islet-specific epigenome maps. Nat Genet (2018) 50:1505–13. doi: 10.1038/s41588-018-0241-6
3. Scott RA, Scott LJ, Mägi R, Marullo L, Gaulton KJ, Kaakinen M, et al. An expanded genome-wide association study of type 2 diabetes in Europeans. Diabetes (2017) 66:2888–902. doi: 10.2337/DB16-1253/-/DC1
4. Xue A, Wu Y, Zhu Z, Zhang F, Kemper KE, Zheng Z, et al. Genome-wide association analyses identify 143 risk variants and putative regulatory mechanisms for type 2 diabetes. Nat Commun (2018) 9:1–14. doi: 10.1038/s41467-018-04951-w
5. Grotz AK, Gloyn AL, Thomsen SK. Prioritising causal genes at type 2 diabetes risk loci. Curr Diabetes Rep (2017) 17:1–9. doi: 10.1007/S11892-017-0907-Y/FIGURES/1
6. Barbitoff YA, Serebryakova EA, Nasykhova YA, Predeus AV, Polev DE, Shuvalova AR, et al. Identification of novel candidate markers of type 2 diabetes and obesity in Russia by exome sequencing with a limited sample size. Genes (Basel) (2018) 9:415. doi: 10.3390/GENES9080415
7. Cheung CYY, Tang CS, Xu A, Lee CH, Au KW, Xu L, et al. Exome-chip association analysis reveals an Asian-specific missense variant in PAX4 associated with type 2 diabetes in Chinese individuals. Diabetologia (2017) 60:107–15. doi: 10.1007/S00125-016-4132-Z/TABLES/3
8. Curtis D. Analysis of rare coding variants in 200,000 exome-sequenced subjects reveals novel genetic risk factors for type 2 diabetes. Diabetes Metab Res Rev (2022) 38. doi: 10.1002/DMRR.3482
9. Deaton AM, Parker MM, Ward LD, Flynn-Carroll AO, BonDurant L, Hinkle G, et al. Gene-level analysis of rare variants in 379,066 whole exome sequences identifies an association of GIGYF1 loss of function with type 2 diabetes. Sci Rep (2021) 11:1–16. doi: 10.1038/s41598-021-99091-5
10. Flannick J, Mercader JM, Fuchsberger C, Udler MS, Mahajan A, Wessel J, et al. Exome sequencing of 20,791 cases of type 2 diabetes and 24,440 controls. Nature (2019) 570:71–6. doi: 10.1038/s41586-019-1231-2
11. Fuchsberger C, Flannick J, Teslovich TM, Mahajan A, Agarwala V, Gaulton KJ, et al. The genetic architecture of type 2 diabetes. Nature (2016) 536:41–7. doi: 10.1038/nature18642
12. Kwak SH, Chae J, Lee S, Choi S, Koo BK, Yoon JW, et al. Nonsynonymous variants in PAX4 and GLP1R are associated with type 2 diabetes in an East Asian population. Diabetes (2018) 67:1892–902. doi: 10.2337/DB18-0361
13. O’Beirne SL, Salit J, Rodriguez-Flores JL, Staudt MR, Khalil CA, Fakhro KA, et al. Exome sequencing-based identification of novel type 2 diabetes risk allele loci in the Qatari population. PLoS One (2018) 13. doi: 10.1371/JOURNAL.PONE.0199837
14. Mahajan A, Go MJ, Zhang W, Below JE, Gaulton KJ, Ferreira T, et al. Genome-wide trans-ancestry meta-analysis provides insight into the genetic architecture of type 2 diabetes susceptibility. Nat Genet (2014) 46:234–44. doi: 10.1038/ng.2897
15. Zheng Q, Zhang Y, Jiang J, Jia J, Fan F, Gong Y, et al. Exome-wide association study reveals several susceptibility genes and pathways associated with acute coronary syndromes in Han Chinese. Front Genet (2020) 11:336/BIBTEX. doi: 10.3389/FGENE.2020.00336/BIBTEX
16. Jemaa R, Razgallah R, Rais L, ben Ghorbel I, Feki M, Kallel A. Prevalence of diabetes in the Tunisian population: Results of the ATERA-survey. Arch Cardiovasc Dis Suppl (2023) 15:131. doi: 10.1016/J.ACVDSP.2022.10.253
17. Saidi O, O’Flaherty M, Ben Mansour N, Aissi W, Lassoued O, Capewell S, et al. Forecasting Tunisian type 2 diabetes prevalence to 2027: validation of a simple model. BMC Public Health (2015) 15:104. doi: 10.1186/s12889-015-1416-z
18. American Diabetes Association. 2. Classification and diagnosis of diabetes: Standards of medical care in diabetes-2021. Diabetes Care (2021) 44:S15–33. doi: 10.2337/dc21-S002
19. Poplin R, Ruano-Rubio V, DePristo MA, Fennell TJ, Carneiro MO, Van der Auwera GA, et al. Scaling accurate genetic variant discovery to tens of thousands of samples. bioRxiv (2017), 201178. doi: 10.1101/201178
20. Wang K, Li M, Hakonarson H. ANNOVAR : functional annotation of genetic variants from high-throughput sequencing data. Nucleic Acids Res (2010) 38:1–7. doi: 10.1093/nar/gkq603
21. Desvignes J-P, Bartoli M, Delague V, Krahn M, Miltgen M, Béroud C, et al. VarAFT: a variant annotation and filtration system for human next generation sequencing data. Nucleic Acids Res (2018) 46:W545–53. doi: 10.1093/nar/gky471
22. Consortium T 1000 GP. A global reference for human genetic variation. Nature (2015) 526:68–74. doi: 10.1038/nature15393
23. Chen S, Francioli LC, Goodrich JK, Collins RL, Wang Q, Alföldi J, et al. A genome-wide mutational constraint map quantified from variation in 76,156 human genomes. bioRxiv (2022). doi: 10.1101/2022.03.20.485034
24. Kumar P, Henikoff S, Ng PC. Predicting the effects of coding non-synonymous variants on protein function using the SIFT algorithm. Nat Protoc (2009) 4:1073–82. doi: 10.1038/nprot.2009.86
25. Adzhubei IA, Schmidt S, Peshkin L, Ramensky VE, Gerasimova A, Bork P, et al. A method and server for predicting damaging missense mutations. Nat Methods (2010) 7:248–9. doi: 10.1038/nmeth0410-248
26. Schwarz JM, Rödelsperger C, Schuelke M, Seelow D. MutationTaster evaluates disease-causing potential of sequence alterations. Nat Methods (2010) 7:575–6. doi: 10.1038/nmeth0810-575
27. Reva B, Antipin Y, Sander C. Determinants of protein function revealed by combinatorial entropy optimization. Genome Biol (2007) 8:R232. doi: 10.1186/gb-2007-8-11-r232
28. Choi Y, Chan AP. PROVEAN web server: a tool to predict the functional effect of amino acid substitutions and indels. Bioinformatics (2015) 31:2745–7. doi: 10.1093/bioinformatics/btv195
29. Dong C, Wei P, Jian X, Gibbs R, Boerwinkle E, Wang K, et al. Comparison and integration of deleteriousness prediction methods for nonsynonymous SNVs in whole exome sequencing studies. Hum Mol Genet (2015) 24:2125–37. doi: 10.1093/hmg/ddu733
30. Chun S, Fay JC. Identification of deleterious mutations within three human genomes. Genome research (2009) 19:1553–61. doi: 10.1101/gr.092619.109.2001
31. Shihab HA, Gough J, Cooper DN, Stenson PD, Barker GLA, Edwards KJ, et al. Predicting the functional , molecular , and phenotypic consequences of amino acid substitutions using hidden. Hum Mutat (2012) 34:57–65. doi: 10.1002/humu.22225
32. Quang D, Chen Y, Xie X. DANN: a deep learning approach for annotating the pathogenicity of genetic variants. Bioinformatics (2015) 31:761–3. doi: 10.1093/BIOINFORMATICS/BTU703
33. Kircher M, Witten DM, Jain P, O’roak BJ, Cooper GM, Shendure J. A general framework for estimating the relative pathogenicity of human genetic variants. Nat Genet (2014) 46, 310–315. doi: 10.1038/ng.2892
34. Carter H, Douville C, Stenson PD, Cooper DN, Karchin R. Identifying Mendelian disease genes with the Variant Effect Scoring Tool. BMC Genomics (2013) 14:S3. doi: 10.1186/1471-2164-14-S3-S3
35. Pezzilli S, Ludovico O, Biagini T, Mercuri L, Alberico F, Lauricella E, et al. Insights from molecular characterization of adult patients of families with multigenerational diabetes. Diabetes (2018) 67:137–45. doi: 10.2337/db17-0867
36. Castellana S, Fusilli C, Mazza T. Chapter 22 A broad overview of computational methods for predicting the pathophysiological effects of non-synonymous variants. Methods Mol Biol (2016) 1415:423–40. doi: 10.1007/978-1-4939-3572-7
37. Grimm DG, Azencott CA, Aicheler F, Gieraths U, Macarthur DG, Samocha KE, et al. The evaluation of tools used to predict the impact of missense variants is hindered by two types of circularity. Hum Mutat (2015) 36:513–23. doi: 10.1002/humu.22768
38. Chang CC, Chow CC, Tellier LC, Vattikuti S, Purcell SM, Lee JJ. Second-generation PLINK: rising to the challenge of larger and richer datasets. Gigascience (2015) 4:7. doi: 10.1186/s13742-015-0047-8
39. Yin L, Zhang H, Tang Z, Xu J, Yin D, Zhang Z, et al. rMVP: A memory-efficient, visualization-enhanced, and parallel-accelerated tool for genome-wide association study. Genomics Proteomics Bioinf (2021) 19:619–28. doi: 10.1016/J.GPB.2020.10.007
40. de Leeuw CA, Mooij JM, Heskes T, Posthuma D. MAGMA: generalized gene-set analysis of GWAS data. PLoS Comput Biol (2015) 11:e1004219. doi: 10.1371/JOURNAL.PCBI.1004219
41. Liberzon A, Birger C, Thorvaldsdóttir H, Ghandi M, Mesirov JP, Tamayo P. The Molecular Signatures Database (MSigDB) hallmark gene set collection. Cell Syst (2015) 1:417. doi: 10.1016/J.CELS.2015.12.004
42. Subramanian A, Tamayo P, Mootha VK, Mukherjee S, Ebert BL, Gillette MA, et al. Gene set enrichment analysis: A knowledge-based approach for interpreting genome-wide expression profiles. Proc Natl Acad Sci U.S.A. (2005) 102:15545–50. doi: 10.1073/PNAS.0506580102/SUPPL_FILE/06580FIG7.JPG
43. Mahajan A, Wessel J, Willems SM, Zhao W, Robertson NR, Chu AY, et al. Refining the accuracy of validated target identification through coding variant fine-mapping in type 2 diabetes. Nat Genet (2018) 50:559–71. doi: 10.1038/s41588-018-0084-1
44. Kefi R, Hsouna S, Ben Halim N, Lasram K, Romdhane L, Messai H, et al. Phylogeny and genetic structure of Tunisians and their position within Mediterranean populations. Mitochondrial DNA (2015) 26:593–604. doi: 10.3109/19401736.2013.879649
45. Jmel H, Romdhane L, Halima YB, Hechmi M, Naouali C, Dallali H, et al. Pharmacogenetic landscape of metabolic syndrome components drug response in Tunisia and comparison with worldwide populations. PLoS One (2018) 13. doi: 10.1371/journal.pone.0194842
46. Hamdi Y, Ben Rekaya M, Jingxuan S, Nagara M, Messaoud O, Benammar Elgaaied A, et al. A genome wide SNP genotyping study in the Tunisian population: specific reporting on a subset of common breast cancer risk loci. BMC Cancer (2018) 18:1295. doi: 10.1186/s12885-018-5133-8
47. Ben Halima Y, Kefi R, Sazzini M, Giuliani C, De Fanti S, Nouali C, et al. Lactase persistence in Tunisia as a result of admixture with other Mediterranean populations. Genes Nutr (2017) 12:1–10. doi: 10.1186/S12263-017-0573-3/FIGURES/3
48. Cauchi S, Ezzidi I, El Achhab Y, Mtiraoui N, Chaieb L, Salah D, et al. European genetic variants associated with type 2 diabetes in North African Arabs. Diabetes Metab (2012) 38:316–23. doi: 10.1016/J.DIABET.2012.02.003
49. Murakami S, Fujita N, Kondo H, Takeda I, Momota R, Ohtsuka A, et al. Abnormalities in the fiber composition and capillary architecture in the soleus muscle of type 2 diabetic Goto-Kakizaki rats. Sci World J (2012) 2012. doi: 10.1100/2012/680189
50. Teng S, Huang P. The effect of type 2 diabetes mellitus and obesity on muscle progenitor cell function. Stem Cell Res Ther (2019) 10:1–15. doi: 10.1186/S13287-019-1186-0
51. Merz KE, Thurmond DC. Role of Skeletal Muscle in Insulin Resistance and Glucose Uptake Didactic Synopsis Major teaching points. Compr Physiol (2020) 10:785–809. doi: 10.1002/cphy.c190029
52. Langenberg C, Lotta LA. Genomic insights into the causes of type 2 diabetes. Lancet (2018) 391:2463–74. doi: 10.1016/S0140-6736(18)31132-2
53. Hollville E, Romero SE, Deshmukh M. Apoptotic cell death regulation in neurons. FEBS J (2019) 286:3276–3298 .doi: 10.1111/FEBS.14970
54. Moujalled D, Strasser A, Liddell JR. Molecular mechanisms of cell death in neurological diseases. Cell Death Differ (2021) 28:2029–44. doi: 10.1038/s41418-021-00814-y
55. Chen X, Famurewa AC, Tang J, Olatunde OO, Olatunji OJ. Hyperoside attenuates neuroinflammation, cognitive impairment and oxidative stress via suppressing TNF-α/NF-κB/caspase-3 signaling in type 2 diabetes rats. Nutr Neurosci (2022) 25:1774–84. doi: 10.1080/1028415X.2021.1901047
56. Ghowsi M, Qalekhani F, Farzaei MH, Mahmudii F, Yousofvand N, Joshi T. Inflammation, oxidative stress, insulin resistance, and hypertension as mediators for adverse effects of obesity on the brain: A review. Biomedicine (Taipei) (2021) 11:13. doi: 10.37796/2211-8039.1174
57. Ortiz GG, Huerta M, González-Usigli HA, Torres-Sánchez ED, Delgado-Lara DL, Pacheco-Moisés FP, et al. Cognitive disorder and dementia in type 2 diabetes mellitus. World J Diabetes (2022) 13:319. doi: 10.4239/WJD.V13.I4.319
58. Cornick S, Tawiah A, Chadee K. Roles and regulation of the mucus barrier in the gut. Tissue Barriers (2015) 3. doi: 10.4161/21688370.2014.982426
59. Schroeder BO. Fight them or feed them: How the intestinal mucus layer manages the gut microbiota. Gastroenterol Rep (Oxf) (2019) 7:3–12. doi: 10.1093/gastro/goy052
60. Thaiss CA, Levy M, Grosheva I, Zheng D, Soffer E, Blacher E, et al. Hyperglycemia drives intestinal barrier dysfunction and risk for enteric infection. Sci (1979) (2018) 359:1376–83. doi: 10.1126/SCIENCE.AAR3318/SUPPL_FILE/AAR3318_THAISS_SM.PDF
61. Bartolomé A. The pancreatic beta cell: editorial. Biomolecules (2023) 13. doi: 10.3390/BIOM13030495
62. Wortham M, Sander M. Transcriptional mechanisms of pancreatic β-cell maturation and functional adaptation. Trends Endocrinol Metab (2021) 32:474. doi: 10.1016/J.TEM.2021.04.011
63. Deacon CF. Physiology and pharmacology of DPP-4 in glucose homeostasis and the treatment of type 2 diabetes. Front Endocrinol (Lausanne) (2019) 10:80. doi: 10.3389/FENDO.2019.00080
64. Sarkar J, Nargis T, Tantia O, Ghosh S, Chakrabarti P. Increased plasma dipeptidyl peptidase-4 (DPP4) activity is an obesity-independent parameter for glycemic deregulation in type 2 diabetes patients. Front Endocrinol (Lausanne) (2019) 10:505. doi: 10.3389/FENDO.2019.00505
65. Deacon CF. Physiology and pharmacology of DPP-4 in glucose homeostasis and the treatment of type 2 diabetes. Front Endocrinol (Lausanne) (2019) 10:80/BIBTEX. doi: 10.3389/FENDO.2019.00080/BIBTEX
Keywords: type 2 diabetes, exome wide association study, Tunisian population, gene-based analysis, pathway-based analysis
Citation: Dallali H, Boukhalfa W, Kheriji N, Fassatoui M, Jmel H, Hechmi M, Gouiza I, Gharbi M, Kammoun W, Mrad M, Taoueb M, Krir A, Trabelsi H, Bahlous A, Jamoussi H, Messaoud O, Abid A and Kefi R (2023) The first exome wide association study in Tunisia: identification of candidate loci and pathways with biological relevance for type 2 diabetes. Front. Endocrinol. 14:1293124. doi: 10.3389/fendo.2023.1293124
Received: 12 September 2023; Accepted: 27 November 2023;
Published: 19 December 2023.
Edited by:
Yanshan Dai, Bristol Myers Squibb, United StatesReviewed by:
Qi Su, University of California, San Diego, United StatesCopyright © 2023 Dallali, Boukhalfa, Kheriji, Fassatoui, Jmel, Hechmi, Gouiza, Gharbi, Kammoun, Mrad, Taoueb, Krir, Trabelsi, Bahlous, Jamoussi, Messaoud, Abid and Kefi. This is an open-access article distributed under the terms of the Creative Commons Attribution License (CC BY). The use, distribution or reproduction in other forums is permitted, provided the original author(s) and the copyright owner(s) are credited and that the original publication in this journal is cited, in accordance with accepted academic practice. No use, distribution or reproduction is permitted which does not comply with these terms.
*Correspondence: Rym Kefi, cnltLmtlZmlAcGFzdGV1ci51dG0udG4=
Disclaimer: All claims expressed in this article are solely those of the authors and do not necessarily represent those of their affiliated organizations, or those of the publisher, the editors and the reviewers. Any product that may be evaluated in this article or claim that may be made by its manufacturer is not guaranteed or endorsed by the publisher.
Research integrity at Frontiers
Learn more about the work of our research integrity team to safeguard the quality of each article we publish.