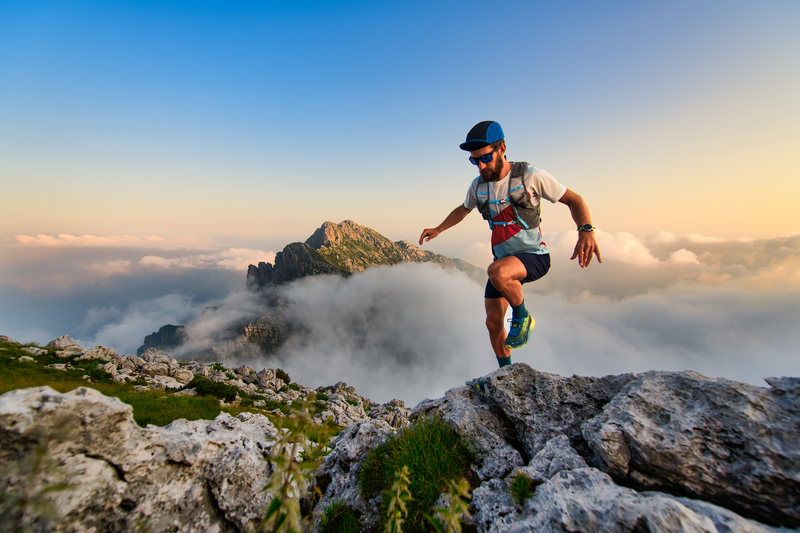
94% of researchers rate our articles as excellent or good
Learn more about the work of our research integrity team to safeguard the quality of each article we publish.
Find out more
ORIGINAL RESEARCH article
Front. Endocrinol. , 21 December 2023
Sec. Adrenal Endocrinology
Volume 14 - 2023 | https://doi.org/10.3389/fendo.2023.1285631
Aim: To develop and internally validate a novel predictive model for SDHB mutations in pheochromocytomas and retroperitoneal paragangliomas (PPGLs).
Methods: Clinical data of patients with PPGLs who presented to Peking Union Medical College Hospital from 2013 to 2022 and underwent genetic testing were retrospectively collected. Variables were screened by backward stepwise and clinical significance and were used to construct multivariable logistic models in 50 newly generated datasets after the multiple imputation. Bootstrapping was used for internal validation. A corresponding nomogram was generated based on the model. Sensitivity analyses were also performed.
Results: A total of 556 patients with PPGLs were included, of which 99 had a germline SDHB mutation. The prediction model revealed that younger age of onset [Odds ratio (OR): 0.93, 95% CI: 0.91-0.95], synchronous metastasis (OR: 6.43, 95% CI: 2.62-15.80), multiple lesion (OR: 0.22, 95% CI: 0.09-0.54), retroperitoneal origin (OR: 5.72, 95% CI: 3.13-10.47), negative 131I-meta-iodobenzylguanidine (MIBG) (OR: 0.34, 95% CI: 0.15-0.73), positive octreotide scintigraphy (OR: 3.24, 95% CI: 1.25-8.43), elevated 24h urinary dopamine (DA) (OR: 1.72, 95% CI: 0.93-3.17), NE secretory type (OR: 2.83, 95% CI: 1.22- 6.59), normal secretory function (OR: 3.04, 95% CI: 1.04-8.85) and larger tumor size (OR: 1.09, 95% CI: 0.99-1.20) were predictors of SDHB mutations in PPGLs, and showed good and stable predictive performance with a mean area under the ROC curve (AUC) of 0.865 and coefficient of variation of 2.2%.
Conclusions: This study provided a novel and useful tool for predicting SDHB mutations by integrating easily obtained clinical data. It may help clinicians select suitable genetic testing methods and make appropriate clinical decisions for these high-risk patients.
Pheochromocytomas and paragangliomas (PPGLs), a kind of rare neuroendocrine tumor originating from adrenal chromaffin tissue and sympathetic and parasympathetic ganglia, are recognized to have the highest heritability rate among all tumors, with almost 40% of patients found to carry germline mutations in susceptibilitygenes, including SDHx, RET, VHL, FH, EPAS1, and NF1 (1–4). Among them, SDHB mutations are the most relevant for the treatment and prognosis of PPGLs. It is recommended that total adrenalectomy should be preferred to adrenal-sparing surgery for SDHB-mutated patients (5) due to their higher risk of metastasis and poorer prognosis (6–13). Therapeutic options for metastatic PPGLs are usually limited, but some retrospective clinical studies (14) have revealed that metastatic PPGLs with SDHB germline mutations may respond better than others to CVD (cyclophosphamide, vincristine, and dacarbazine) therapy (15–17), temozolomide (18), peptide receptor radionuclide therapy (PRRT) (19–27), and tyrosine kinase inhibitors (28–31). Importantly, patients’ SDHB mutations can be inherited by their offspring. It is reported that the penetrance of SDHB-related PPGLs is 21% by the age of 50, 42% by the age of 60 (32) and < 50% over a lifetime (33, 34). Therefore, it is necessary to identify patients with SDHB germline mutations as early as possible and then provide personalized management and follow-up for such high-risk patients and their families (35–37).
After the diffusion of Next Generation Sequencing (NGS), SDHB mutations are mainly detected through a panel of PPGL susceptibility genes, but genetic testing is expensive and time-consuming and sometimes restricted by barriers of availability, patient privacy, insurance coverage, or technical reasons. In addition, the results of genetic testing often lag behind the clinical decision, indicating that a convenient model that integrates easily obtained clinical characteristics may be useful for predicting SDHB mutations prior to the clinical decision itself.
Therefore, this cross-sectional study aimed to compare the clinical characteristics and prognosis between patients with and without SDHB mutations and to internally develop and validate a novel predictive model for SDHB mutations by integrating easily obtained clinical characteristics, biochemical levels, and functional imaging results.
Patients who presented to Peking Union Medical College Hospital from 2013 to 2022 and met the following inclusion criteria were enrolled in this study:
1. A diagnosis of pheochromocytoma or retroperitoneal paraganglioma made by experienced physicians based on clinical characteristics, biochemical tests, and functional imaging results and confirmed by pathological findings for patients undergoing surgical resection.
2. Availability of genetic tests involving the SDHB gene, including Sanger sequencing, a panel test of PPGL susceptibility genes, Whole Exome Sequencing (WES), and the Multiplex Ligation Dependent Probe Amplification (MLPA) test.
3. Patients with clinical information available.
Exclusion criteria were as follows:
Patients diagnosed with Multiple Endocrine Neoplasia Type 2 (MEN2) or von Hippel-Lindau (VHL) disease based on their unique clinical features and found to carry pathogenic mutations of RET or VHL, respectively.
Clinical data of patients were collected retrospectively without knowing their gene mutations and included sex, age of onset, age at diagnosis, presence of synchronous metastases, number and site of lesions, size and location of the primary tumor, family history of PPGLs, clinical symptoms, the highest systolic and diastolic blood pressure (SBPmax and DBPmax), information on surgery, results of immunohistochemistry (IHC) staining for SDHB and KI-67 index on resected tumors, duration of follow-up, the occurrence of recurrence and metastasis during the follow-up. Results of 24-h urinary catecholamines (CAs) tests [including norepinephrine (NE), epinephrine (E), and dopamine (DA)] and functional imaging [including 131I-meta-iodobenzylguanidine (MIBG) and octreotide scintigraphy] were also extracted.
The duration of follow-up was calculated from surgery to the last follow-up for patients undergoing surgery and from the diagnosis for those not undergoing surgery. Measurements of 24-h urinary catecholamines were done by HPLC before 01/08/2021 and by LC-MS/MS after 01/08/2021. Elevated 24-h urinary NE, E, and DA were defined as levels higher than 40.7 μg/24h, 6.4 μg/24h, and 330.5 μg/24h, respectively, when measured by HPLC and higher than 76.9 μg/24h, 11.0 μg/24h, and 459.9 μg/24h, respectively, when measured by LC-MS/MS. The secretory type was determined by the 24-h urinary CAs. If patients had elevated 24-h urinary NE but normal 24-h urinary E, their secretory type was considered NE, whereas if they had elevated 24-h urinary E regardless of NE, their secretory type was considered E (38, 39). Tumor size was calculated as the largest diameter by enhanced computed tomography (CT) or pathology results.
Metastasis was defined as the occurrence of PPGLs in distant non-chromaffin tissues, such as lung, liver, bone, and lymph nodes (40), while synchronous metastasis was defined as the occurrence of metastasis at the first diagnosis or within six months after surgical resection. Recurrence-free survival (RFS) and metastasis-free survival (MFS) were calculated from the surgery or the first diagnosis to the occurrence of recurrence and metastasis or to the last follow-up.
This study was approved by the Ethics Committee of the Peking Union Medical College Hospital in Beijing, China. Written informed consent was obtained from all the patients.
All patients included in this study underwent germline genetic testing involving the SDHB gene. The genetic testing procedure has been described previously (13). The pathogenicity of SDHB germline mutations and deletions was classified into five grades according to the consensus of the American College of Medical Genetics and Genomics (ACMG), including “Pathogenic (P)”, “Likely Pathogenic (LP)”, “Uncertain significance (VUS)”, “Likely Benign (LB)”, and “Benign (B)”. If SDHB germline mutations found in patients were assessed as “P” or “LP”, their carriers could be classified into the SDHB group. However, if SDHB mutations were assessed as “VUS”, which indicated that it is unclear whether these mutations could cause PPGLs, their corresponding formalin-fixed, paraffin-embedded (FFPE) tumor tissues were used to detect the expression of SDHB, the protein encoded by the SDHB gene, by immunohistochemical (IHC) staining. Patients in whom the IHC on FFPE tumor tissue showed loss of SDHB protein expression were classified into the SDHB group, whereas patients with a preserved SDHB protein expression were classified as non-mutated. In addition, if SDHB mutations were assessed as “LB” or “B”, their carriers were classified into the non-SDHB group. Patients from both the SDHB group and non-SDHB groups were used to construct the predictive model.
MLPA testing was performed to assess the presence of SDHB gene deletions only in a subset of patients. If patients were found not to carry a PPGL susceptibility gene mutation but did not undergo MLPA testing and IHC staining of SDHB on FFPE tissue sections, they could not be excluded from carrying a deletion of SDHB, with a consequent potential misclassification bias. Therefore, to further analyze the impact of this misclassification bias on the predictive capacity of the established model, patients in the non-SDHB group were again divided into two groups: the identified non-SDHB group, with patients who underwent MLPA testing or had positive staining for SDHB on FFPE tissue sections, and the unidentified non-SDHB group, with patients who did not undergo MLPA testing and IHC staining for SDHB on FFPE tissue sections.
The sample size was not calculated during the study design stage but was determined by the pragmatic availability of eligible patients. Univariable logistic regression was used to recognize possible predictors of SDHB mutations. For missing data, the missing pattern was analyzed (Supplementary Figure 1; Supplementary Table 1). Namely, the clinical characteristics involved in the subsequent logistic regression models were compared between the groups with and without missing data. Wilcoxon and Chi-square tests, or Fisher’s exact test, were performed for continuous and categorical variables, respectively. If the results did not reach statistical significance (P>=0.05), their missing module was considered to be Missing Completely at Random (MCAR). Other variables (P<0.05) were further evaluated for the missing module. If the probability of these variables being missing could be predicted by known clinical characteristics, their missing module would be considered to be Missing At Random (MAR). After evaluation, some variables were MCAR and others were MAR. Therefore, the multiple imputation method (41–43) was used to impute these missing data, and then 50 new datasets were generated for further analysis. Subsequently, multivariable logistic models were established in 50 newly generated datasets using variables screened by backward stepwise methods (44) and clinical significance, and, finally, the coefficient of each factor was calculated as the mean of that in each model.
The model was internally validated by using the 1000-replicate bootstrapping method (45, 46) with the “mice” package. Discrimination, stability, and calibration were assessed using the mean area under the receiver operating characteristic (ROC) curve (AUC), its standard error (SD), coefficient of variation (CV), and the calibration curve. No adjustment was made to the model after evaluation, and its corresponding nomogram was built.
Moreover, the sensitivity analysis was performed to assess the predictive capacity of the model only in patients from the SDHB group and the identified non-SDHB group in order to identify the impact of patients from the unidentified non-SDHB group. The predictive capacity of the model was also evaluated in the dataset, where patients with missing data were excluded.
The Shapiro-Wilk test was used to assess the normality of the distribution of continuous variables. Continuous variables with a standard normal distribution were presented as mean± standard deviation (SD) and others as median (interquartile range [IQR]). Categorical variables were presented as frequencies (percentages). For all statistical analyses, a two-sided P<0.05 was considered statistically significant, and its 95% confidence interval (95% CI) was reported. All statistical analyses were performed using R-4.1.3 (R Foundation, www.r-project.org, Vienna, Austria).
The study enrolled 556 patients in total (Figure 1), of whom 251 (45.1%) were men. The median follow-up was 4.0 (1.5-7.0) years. The median ages of onset and diagnosis were 36.5 (26.0-47.0) and 39.0 (28.0-51.0) years, respectively. A total of 419 patients (75.4%) had one of the classic symptoms, and 454 (81.7%) patients had hypertension with a median SBPmax and DBPmax of 180 (160-209) and 110 (96.75-120) mmHg, respectively. Only 13 (2.3%) patients were recorded to have a family history of PPGLs.
Figure 1 The flow diagram of the study design. WES, Whole Exome Sequencing; MLPA, Multiplex Ligation Dependent Probe Amplification; MEN2, multiple endocrine neoplasia Type 2; VHL, von Hippel-Lindau.
Tumors in 244 patients (43.9%) originated from retroperitoneal ganglia and 312 (56.1%) from adrenal glands, of which 34 (10.9%) were bilateral. The median size of the primary tumor was 5.4 (4.0-7.5) cm. In terms of functional imaging, MIBG and octreotide scintigraphy were performed in 425 (76.4%) and 433 (77.9%) patients, respectively, of which 359 (64.6%) and 328 (59.0%) showed positive results.
After the evaluation of the SDHB gene, 99 patients (17.8%) were classified into the SDHB group, and 457 (82.2%) were classified into the non-SDHB group, of which 290 (63.5%) were grouped into the identified non-SDHB group. Clinical characteristics were compared between patients in the SDHB and the non-SDHB groups, as shown in Table 1.
Synchronous metastases were found in 37 (6.7%) patients. During the follow-up, 134 (24.1%) and 139 (25.0%) patients developed recurrence and distant metastasis, respectively. Compared with patients in the non-SDHB group, those in the SDHB group had worse RFS (P=0.019, Figure 2A) and MFS (P<0.001, Figure 2B), as well as a higher KI67 index [3.0% (2.0-8.0) vs. 2.0% (1.0- 3.0), P<0.001], which was regarded as a risk factor for poor prognosis.
Figure 2 Kaplan-Meier curves of (A) recurrence-free survival (RFS)" between patients with and without SDHB germline mutations (with only 555 patients' information available) and of (B) metastasis-free survival (MFS) between patients with and without SDHB germline mutations (with only 518 patients' information available after excluding 37 patients with synchronous metastases).
Univariable logistic analysis revealed that younger age of onset [odds ratio (OR): 0.94, 95% CI: 0.93-0.96; P<0.001], synchronous metastasis (OR: 7.4, 95% CI: 3.72-15.02; P<0.001), retroperitoneal origin (OR: 4.42, 95% CI: 2.76-7.25; P<0.001), negative MIBG (OR: 0.35, 95% CI: 0.2-0.63; P<0.001), positive octreotide scintigraphy (OR: 4.15, 95% CI: 1.97-10.19; P<0.001), secretory type of NE [OR: 4.12 (compared with secretory type of E), 95% CI: 2.09-9.09; P<0.001], normal 24-h urinary E and NE [OR: 3.81 (compared with secretory type of E), 95% CI: 1.64-9.39; P=0.002], larger primary tumor size (OR: 1.09, 95% CI: 1.02-1.17; P=0.009), absence of classic symptoms (OR: 0.62, 95% CI: 0.39-1.01; P=0.052), elevated urinary DA (OR: 1.62, 95% CI: 0.99-2.62; P=0.052), family history of PPGLs (OR: 2.99, 95% CI: 0.89-9.15; P=0.060), higher DBPmax (OR: 1.01, 95% CI: 1-1.02; P=0.061), and single lesion (OR: 0.49, 95% CI: 0.21-1.01; P=0.072) were predictors of SDHB mutations (Table 2).
Table 2 Univariate and multivariate logistic regression analysis of predictors for PPGLs with SDHB mutations.
In the multivariable logistic model, younger age of onset (OR: 0.93, 95% CI: 0.91-0.95; P<0.001), synchronous metastasis (OR: 6.43, 95% CI: 2.62- 15.80; P<0.001), single lesion (OR: 0.22, 95% CI: 0.09-0.54; P<0.001), retroperitoneal origin (OR: 5.72, 95% CI: 3.13-10.47; P<0.001), negative MIBG (OR: 0.34, 95% CI: 0.15-0.73; P=0.006), positive octreotide scintigraphy (OR: 3.24, 95% CI: 1.25-8.43; P=0.016), elevated 24-h urinary DA (OR: 1.72, 95% CI: 0.93-3.17; P=0.083), secretory type of NE [OR: 2.83 (compared with the secretory type of E), 95% CI: 1.22- 6.59; P=0.016], normal 24h urinary E and NE [OR: 3.04 (compared with the secretory type of E), 95% CI: 1.04-8.85; P=0.041], and larger primary tumor size (OR: 1.09, 95% CI: 0.99-1.20; P=0.075) were independent predictors of SDHB mutations (Table 2).
The model showed rather good discrimination with an AUC of 0.866 (95% CI: 0.828-0.903) (Supplementary Figure 2A) and calibration (R2 = 0.417, P = 0.775) (Supplementary Figure 2B) in a randomly selected dataset. After internal validation with 1000 bootstrap samples, the model still showed good discrimination with a mean AUC of 0.865 and good stability with an SD and CV of the AUC of 0.019 and 2.2%, respectively. Therefore, the model was not adjusted and was presented as a nomogram (Figure 3).
Figure 3 Nomogram of the established prediction model for SDHB mutations. MIBG, 131I-meta-iodobenzylguanidine; If patients had elevated 24-h urinary NE but normal 24-h urinary E, their secretory type was considered to be NE, whereas if they had elevated 24-h urinary E regardless of NE, their secretory type was considered to be E.
The results of the sensitivity analysis revealed an AUC of 0.873 (95% CI: 0.834-0.912) in 389 patients after excluding 167 unidentified non-SDHB patients and 0.871 (95% CI: 0.822-0.921) in 324 patients after excluding those with missing data.
This cross-sectional study retrospectively summarized the differences in clinical characteristics and prognosis between patients with and without SDHB mutations based on a large population of 556 PPGL cases. Importantly, we proposed a novel prediction model for SDHB mutations that integrates easily obtained clinical characteristics, functional imaging results, and biochemical parameters and showed good predictive performance and stability. The corresponding nomogram will be convenient and informative for clinicians to make an appropriate clinical decision for these high-risk patients when genetic testing is not available or the report is delayed.
Our results are consistent with the previous findings revealing that SDHB-mutated PPGLs are more likely to originate from extra-adrenal locations (13, 47, 48), have a younger age of onset (48), have the secretory type of NE (8), have a larger size (8), and express high levels of SSTR2 (19, 49). In addition, previous studies have mostly focused on the differences between pseudohypoxia type (PHT) PPGLs and non-PHT PPGLs and found that most PHT PPGLs presented with a younger age of onset (50), a noradrenergic phenotype (51, 52), and elevated DA (52). A recent study also developed a nomogram based on age ≤35 years, hypertension, 24-h urinary vanillylmandelic acid output (VMA) ≥100 umol/24-h and urinary 17-ketosteroid (17 KS) ≤50 umol/24-h levels to discriminate PHT from non-PHT PPGLs, which showed good discriminatory performance with an AUC of 0.829 (95% CI, 0.767–0.891) (53). However, hypertension was not regarded as a significant predictor in our study, possibly because it is a relatively nonspecific clinical finding (14). Another study used pre-operative weight loss > 10% body weight, elevated pre-operative hematocrit > 50%, normal baseline heart rate < 100 bpm, and normal plasma metanephrines < 0.60 nmol/L to predict the PHT PCCs and achieved an AUC of 0.831 (50). However, the above parameters were not collected in the study design, so we could not assess their predictive performance in our patients.
Our study also demonstrated that PPGLs with SDHB mutations had a higher proportion of recurrence and metastasis and a poorer MFS and RFS, as commonly reported in other studies (48). It is suggested that, compared with other genotypes, PPGLs with SDHB mutations should have more frequent and longer follow-ups and a more aggressive surveillance plan due to their poorer prognosis.
However, there were also some limitations in our study. First, as some patients were not initially diagnosed at our hospital, the clinical characteristics of their primary tumors were partially missing. However, after excluding patients with missing data, the results of the sensitivity analysis showed good predictive performance with an AUC of 0.871 (95% CI: 0.822- 0.921), indicating the low impact of missing data on the discrimination of the model. Second, among the patients in the non-SDHB group, 167 were grouped into the unidentified non-SDHB group due to the uncertainty of SDHB gene deletion, which might cause misclassification bias. However, the model also showed a good performance with an AUC of 0.873 (95% CI: 0.834- 0.912) in predicting SDHB mutations when considering only the 389 patients from the SDHB group and the identified non-SDHB group to exclude the influence of a potential misclassification bias. In fact, deletion in SDHB is rare in the Chinese population, with only 1.6% (1/61) described in our previous study (13). Third, our study only included patients with retroperitoneal paragangliomas and pheochromocytomas, but not those with paragangliomas originating from other organs, such as the head and neck [mostly associated with SDHD mutations and less commonly with SDHB mutations (54)], mediastinum, pelvic cavity, and bladder. For this reason, the application of the predictive model proposed in this study may be limited to retroperitoneal pheochromocytomas and PGLs only, which, however, represent the vast majority of PPGLs. In addition, the model proposed in this study included 24-h urinary CAs and octreotide scintigraphy, which have been progressively replaced in clinical practice by plasma-free metanephrines and 24-h urinary fractionated metanephrines, and PET/CT with 68Ga-somatostatin analogs (68Ga-SSA) due to their higher sensitivity and accuracy in the diagnosis of PPGLs. However, these data were only available in a few patients, which prevented their application in the predictive model construction. It is possible that the use of these parameters will further improve the discrimination of the model. Finally, this is a retrospective and single-center study that only included patients who underwent genetic testing, so selection bias may exist. Although our model included a large population and showed relatively good predictive performance, external validation is still required.
This retrospective study proposed a convenient and informative tool for predicting SDHB mutations in pheochromocytoma and retroperitoneal PGLs by integrating easily obtained clinical, biochemical, and functional imaging characteristics. The proposed model could help clinicians make an appropriate decision and plan a personalized follow-up for these high-risk patients.
The dataset included privacy clinical information of patients. Requests to access these datasets should be directed todG9uZ2FsQHB1bWNoLmNu.
This study was approved by the Ethics Committee of the Peking Union Medical College Hospital in Beijing, China. Written informed consent was obtained from all the patients.
YZ: Data curation, Formal analysis, Software, Validation, Visualization, Writing – original draft, Writing – review & editing. YG: Data curation, Project administration, Resources, Writing – review & editing. XM: Data curation, Resources, Writing – review & editing. TL: Data curation, Resources, Writing – review & editing. YC: Data curation, Investigation, Project administration, Writing – review & editing. YW: Investigation, Resources, Writing – review & editing. ML: Methodology, Resources, Writing – review & editing. DZ: Conceptualization, Methodology, Software, Supervision, Validation, Writing – review & editing. AT: Conceptualization, Funding acquisition, Project administration, Resources, Supervision, Writing – review & editing.
The author(s) declare financial support was received for the research, authorship, and/or publication of this article. The study was supported by the CAMS Innovation Fund for Medical Sciences (CIFMS) (grant number 2021-I2M-C&T-B-002), the National High Level Hospital Clinical Research Funding (grant number 2022-PUMCH-C-028), and the National Key Research and Development Program of China (grant numbers 2021YFC2501600 and 2021YFC2501603).
The authors declare that the research was conducted in the absence of any commercial or financial relationships that could be construed as a potential conflict of interest.
All claims expressed in this article are solely those of the authors and do not necessarily represent those of their affiliated organizations, or those of the publisher, the editors and the reviewers. Any product that may be evaluated in this article, or claim that may be made by its manufacturer, is not guaranteed or endorsed by the publisher.
The Supplementary Material for this article can be found online at: https://www.frontiersin.org/articles/10.3389/fendo.2023.1285631/full#supplementary-material
1. Dahia PL. Pheochromocytoma and paraganglioma pathogenesis: learning from genetic heterogeneity. Nat Rev Cancer (2014) 14(2):108–19. doi: 10.1038/nrc3648
2. Favier J, Amar L, Gimenez-Roqueplo AP. Paraganglioma and phaeochromocytoma: from genetics to personalized medicine. Nat Rev Endocrinol (2015) 11(2):101–11. doi: 10.1038/nrendo.2014.188
3. Neumann HPH, Young WF Jr., Eng C. Pheochromocytoma and paraganglioma. New Engl J Med (2019) 381(6):552–65. doi: 10.1056/NEJMra1806651
4. Casey R, Neumann HPH, Maher ER. Genetic stratification of inherited and sporadic phaeochromocytoma and paraganglioma: implications for precision medicine. Hum Mol Genet (2020) 29(R2):R128–r137. doi: 10.1093/hmg/ddaa201
5. Nockel P, El Lakis M, Gaitanidis A, Yang L, Merkel R, Patel D, et al. Preoperative genetic testing in pheochromocytomas and paragangliomas influences the surgical approach and the extent of adrenal surgery. Surg Jan (2018) 163(1):191–6. doi: 10.1016/j.surg.2017.05.025
6. Martucci VL, Pacak K. Pheochromocytoma and paraganglioma: diagnosis, genetics, management, and treatment. Curr Probl Cancer (2014) 38(1):7–41. doi: 10.1016/j.currproblcancer.2014.01.001
7. Lee H, Jeong S, Yu Y, Kang J, Sun H, Rhee JK, et al. Risk of metastatic pheochromocytoma and paraganglioma in SDHx mutation carriers: a systematic review and updated meta-analysis. J Med Genet (2020) 57(4):217–25. doi: 10.1136/jmedgenet-2019-106324
8. Crona J, Lamarca A, Ghosal S, Welin S, Skogseid B, Pacak K. Genotype-phenotype correlations in pheochromocytoma and paraganglioma: a systematic review and individual patient meta-analysis. Endocrine-Related Cancer (2019) 26(5):539–50. doi: 10.1530/erc-19-0024
9. Jochmanova I, Abcede AMT, Guerrero RJS, Malong CLP, Wesley R, Huynh T, et al. Clinical characteristics and outcomes of SDHB-related pheochromocytoma and paraganglioma in children and adolescents. J Cancer Res Clin Oncol (2020) 146(4):1051–63. doi: 10.1007/s00432-020-03138-5
10. Crona J, Taïeb D, Pacak K. New perspectives on pheochromocytoma and paraganglioma: toward a molecular classification. Endocr Rev (2017) 38(6):489–515. doi: 10.1210/er.2017-00062
11. van Hulsteijn LT, Dekkers OM, Hes FJ, Smit JW, Corssmit EP. Risk of Malignant paraganglioma in SDHB-mutation and SDHD-mutation carriers: a systematic review and meta-analysis. J Med Genet Dec (2012) 49(12):768–76. doi: 10.1136/jmedgenet-2012-101192
12. Turkova H, Prodanov T, Maly M, Martucci V, Adams K, Widimsky J Jr, et al. Characteristics and outcomes of metastatic sdhb and sporadic pheochromocytoma/paraganglioma: an national institutes of health study. Endocr practice: Off J Am Coll Endocrinol Am Assoc Clin Endocrinol (2016) 22(3):302–14. doi: 10.4158/ep15725.Or
13. Ma X, Li M, Tong A, Wang F, Cui Y, Zhang X, et al. Genetic and clinical profiles of pheochromocytoma and paraganglioma: A single center study. Front Endocrinol (2020) 11:574662. doi: 10.3389/fendo.2020.574662
14. Nölting S, Bechmann N, Taieb D, Beuschlein F, Fassnacht M, Kroiss M, et al. Personalized management of pheochromocytoma and paraganglioma. Endocr Rev (2022) 43(2):199–239. doi: 10.1210/endrev/bnab019
15. Jawed I, Velarde M, Därr R, Wolf KI, Adams K, Venkatesan AM, et al. Continued tumor reduction of metastatic pheochromocytoma/paraganglioma harboring succinate dehydrogenase subunit B mutations with cyclical chemotherapy. Cell Mol Neurobiol (2018) 38(5):1099–106. doi: 10.1007/s10571-018-0579-4
16. Hadoux J, Favier J, Scoazec JY, Leboulleux S, Al Ghuzlan A, Caramella C, et al. SDHB mutations are associated with response to temozolomide in patients with metastatic pheochromocytoma or paraganglioma. Int J Cancer (2014) 135(11):2711–20. doi: 10.1002/ijc.28913
17. Ayala-Ramirez M, Feng L, Habra MA, Habra MA, Rich T, Dickson PV, et al. Clinical benefits of systemic chemotherapy for patients with metastatic pheochromocytomas or sympathetic extra-adrenal paragangliomas: insights from the largest single-institutional experience. Cancer (2012) 118(11):2804–12. doi: 10.1002/cncr.26577
18. Tena I, Gupta G, Tajahuerce M, Benavent M, Cifrián M, Falcon A, et al. Successful second-line metronomic temozolomide in metastatic paraganglioma: case reports and review of the literature. Clin Med Insights Oncol (2018) 12:1179554918763367. doi: 10.1177/1179554918763367
19. Ziegler CG, Brown JW, Schally AV, Erler A, Gebauer L, Treszl A, et al. Expression of neuropeptide hormone receptors in human adrenal tumors and cell lines: antiproliferative effects of peptide analogues. Proc Natl Acad Sci USA (2009) 106(37):15879–84. doi: 10.1073/pnas.0907843106
20. Van Essen M, Krenning EP, De Jong M, Valkema R, Kwekkeboom DJ. Peptide Receptor Radionuclide Therapy with radiolabelled somatostatin analogues in patients with somatostatin receptor positive tumours. Acta Oncol (Stockholm Sweden). (2007) 46(6):723–34. doi: 10.1080/02841860701441848
21. van Hulsteijn LT, Niemeijer ND, Dekkers OM, Corssmit EP. (131)I-MIBG therapy for Malignant paraganglioma and phaeochromocytoma: systematic review and meta-analysis. Clin Endocrinol (2014) 80(4):487–501. doi: 10.1111/cen.12341
22. van Essen M, Krenning EP, Kooij PP, Bakker WH, Feelders RA, de Herder WW, et al. Effects of therapy with [177Lu-DOTA0, Tyr3]octreotate in patients with paraganglioma, meningioma, small cell lung carcinoma, and melanoma. J Nucl Med: Off Publ Soc Nucl Med (2006) 47(10):1599–606.
23. Zovato S, Kumanova A, Demattè S, Sansovini M, Bodei L, Di Sarra D, et al. Peptide receptor radionuclide therapy (PRRT) with 177Lu-DOTATATE in individuals with neck or mediastinal paraganglioma (PGL). Hormone Metab Res = Hormon- und Stoffwechselforschung = Hormones metabol (2012) 44(5):411–4. doi: 10.1055/s-0032-1311637
24. Forrer F, Riedweg I, Maecke HR, Mueller-Brand J. Radiolabeled DOTATOC in patients with advanced paraganglioma and pheochromocytoma. Q J Nucl Med Mol imaging: Off Publ Ital Assoc Nucl Med (AIMN) [and] Int Assoc Radiopharmacol (IAR) [and] Section So. (2008) 52(4):334–40.
25. Kong G, Grozinsky-Glasberg S, Hofman MS, Callahan J, Meirovitz A, Maimon O, et al. Efficacy of peptide receptor radionuclide therapy for functional metastatic paraganglioma and pheochromocytoma. J Clin Endocrinol Metab (2017) 102(9):3278–87. doi: 10.1210/jc.2017-00816
26. Vyakaranam AR, Crona J, Norlén O, Granberg D, Garske-Román U, Sandström M, et al. Favorable outcome in patients with pheochromocytoma and paraganglioma treated with (177)Lu-DOTATATE. Cancers (Basel) (2019) 11(7):909. doi: 10.3390/cancers11070909
27. Satapathy S, Mittal BR, Bhansali A. ‘Peptide receptor radionuclide therapy in the management of advanced pheochromocytoma and paraganglioma: A systematic review and meta-analysis’. Clin Endocrinol (2019) 91(6):718–27. doi: 10.1111/cen.14106
28. Ayala-Ramirez M, Chougnet CN, Habra MA, Palmer JL, Leboulleux S, Cabanillas ME, et al. Treatment with sunitinib for patients with progressive metastatic pheochromocytomas and sympathetic paragangliomas. J Clin Endocrinol Metab (2012) 97(11):4040–50. doi: 10.1210/jc.2012-2356
29. O’Kane GM, Ezzat S, Joshua AM, Leibowitz-Amit R, Olney HJ, Krzyzanowska M, et al. A phase 2 trial of sunitinib in patients with progressive paraganglioma or pheochromocytoma: the SNIPP trial. Br J Cancer (2019) 120(12):1113–9. doi: 10.1038/s41416-019-0474-x
30. Zhou Y, Cui Y, Zhang D, Tong A. Efficacy and safety of tyrosine kinase inhibitors in patients with metastatic pheochromocytomas/paragangliomas. J Clin Endocrinol Metab (2023) 108(3):755–66. doi: 10.1210/clinem/dgac657
31. Wang K, Schütze I, Gulde S, Bechmann N, Richter S, Helm J, et al. Personalized drug testing in human pheochromocytoma/paraganglioma primary cultures. Endocrine-Related Cancer (2022) 29(6):285–306. doi: 10.1530/erc-21-0355
32. Rijken JA, Niemeijer ND, Jonker MA, Eijkelenkamp K, Jansen JC, van Berkel A, et al. The penetrance of paraganglioma and pheochromocytoma in SDHB germline mutation carriers. Clin Genet (2018) 93(1):60–6. doi: 10.1111/cge.13055
33. Jafri M, Whitworth J, Rattenberry E, Vialard L, Kilby G, Kumar AV, et al. Evaluation of SDHB, SDHD and VHL gene susceptibility testing in the assessment of individuals with non-syndromic phaeochromocytoma, paraganglioma and head and neck paraganglioma. Clin Endocrinol (2013) 78(6):898–906. doi: 10.1111/cen.12074
34. Benn DE, Zhu Y, Andrews KA, Wilding M, Duncan EL, Dwight T, et al. Bayesian approach to determining penetrance of pathogenic SDH variants. J Med Genet (2018) 55(11):729–34. doi: 10.1136/jmedgenet-2018-105427
35. Buffet A, Ben Aim L, Leboulleux S, Drui D, Vezzosi D, Libé R, et al. Positive impact of genetic test on the management and outcome of patients with paraganglioma and/or pheochromocytoma. J Clin Endocrinol Metab (2019) 104(4):1109–18. doi: 10.1210/jc.2018-02411
36. Kiernan CM, Solórzano CC. Pheochromocytoma and paraganglioma: diagnosis, genetics, and treatment. Surg Oncol Clin N Am (2016) 25(1):119–38. doi: 10.1016/j.soc.2015.08.006
37. Amar L, Bertherat J, Baudin E, Ajzenberg C, Bressac-de Paillerets B, Chabre O, et al. Genetic testing in pheochromocytoma or functional paraganglioma. J Clin Oncol (2005) 23(34):8812–8. doi: 10.1200/jco.2005.03.1484
38. Kimura N, Takayanagi R, Takizawa N, Itagaki E, Katabami T, Kakoi N, et al. Pathological grading for predicting metastasis in phaeochromocytoma and paraganglioma. Endocrine-Related Cancer (2014) 21(3):405–14. doi: 10.1530/erc-13-0494
39. Cho YY, Kwak MK, Lee SE, Ahn SH, Kim H, Suh S, et al. A clinical prediction model to estimate the metastatic potential of pheochromocytoma/paraganglioma: ASES score. Surg Sep (2018) 164(3):511–7. doi: 10.1016/j.surg.2018.05.001
40. Lam AK. Update on adrenal tumours in 2017 world health organization (WHO) of endocrine tumours. Endocr Pathol (2017) 28(3):213–27. doi: 10.1007/s12022-017-9484-5
41. Blazek K, van Zwieten A, Saglimbene V, Teixeira-Pinto A. A practical guide to multiple imputation of missing data in nephrology. Kidney Int (2021) 99(1):68–74. doi: 10.1016/j.kint.2020.07.035
42. Beesley LJ, Bondarenko I, Elliot MR, Kurian AW, Katz SJ, Taylor JM. Multiple imputation with missing data indicators. Stat Methods Med Res (2021) 30(12):2685–700. doi: 10.1177/09622802211047346
43. Donders AR, van der Heijden GJ, Stijnen T, Moons KG. Review: a gentle introduction to imputation of missing values. J Clin Epidemiol (2006) 59(10):1087–91. doi: 10.1016/j.jclinepi.2006.01.014
44. Moons KG, Altman DG, Reitsma JB, Ioannidis JP, Macaskill P, Steyerberg EW, et al. Transparent Reporting of a multivariable prediction model for Individual Prognosis or Diagnosis (TRIPOD): explanation and elaboration. Ann Internal Med (2015) 162(1):W1–73. doi: 10.7326/m14-0698
45. Steyerberg EW, Harrell FE Jr., Borsboom GJ, Eijkemans MJ, Vergouwe Y, Habbema JD. Internal validation of predictive models: efficiency of some procedures for logistic regression analysis. J Clin Epidemiol Aug (2001) 54(8):774–81. doi: 10.1016/s0895-4356(01)00341-9
46. Jaki T, Su TL, Kim M, Lee Van Horn M. An evaluation of the bootstrap for model validation in mixture models. Commun Stat: Simulation Computation (2018) 47(4):1028–38. doi: 10.1080/03610918.2017.1303726
47. Else T, Marvin ML, Everett JN, Gruber SB, Arts HA, Stoffel EM, et al. The clinical phenotype of SDHC-associated hereditary paraganglioma syndrome (PGL3). J Clin Endocrinol Metab (2014) 99(8):E1482–6. doi: 10.1210/jc.2013-3853
48. Cui Y, Ma X, Wang F, Wang H, Zhou T, Chen S, et al. Differences in clinical manifestations and tumor features between metastatic pheochromocytoma/paraganglioma patients with and without germline SDHB mutation. Endocr Pract (2021) 27(4):348–53. doi: 10.1016/j.eprac.2020.09.015
49. Taïeb D, Hicks RJ, Hindié E, Guillet BA, Avram A, Ghedini P, et al. European Association of Nuclear Medicine Practice Guideline/Society of Nuclear Medicine and Molecular Imaging Procedure Standard 2019 for radionuclide imaging of phaeochromocytoma and paraganglioma. Eur J Nucl Med Mol Imaging (2019) 46(10):2112–37. doi: 10.1007/s00259-019-04398-1
50. Baechle JJ, Smith PM, Ortega CA, Wang TS, Solórzano CC, Kiernan CM. Clinical predictors of pseudohypoxia-type pheochromocytomas. Ann Surg Oncol (2022) 29(6):3536–46. doi: 10.1245/s10434-022-11419-1
51. Eisenhofer G, Huynh TT, Pacak K, Brouwers FM, Walther MM, Linehan WM, et al. Distinct gene expression profiles in norepinephrine- and epinephrine-producing hereditary and sporadic pheochromocytomas: activation of hypoxia-driven angiogenic pathways in von Hippel-Lindau syndrome. Endocrine-Related Cancer (2004) 11(4):897–911. doi: 10.1677/erc.1.00838
52. Eisenhofer G, Deutschbein T, Constantinescu G, Langton K, Pamporaki C, Calsina B, et al. Plasma metanephrines and prospective prediction of tumor location, size and mutation type in patients with pheochromocytoma and paraganglioma. Clin Chem Lab Med (2020) 59(2):353–63. doi: 10.1515/cclm-2020-0904
53. Wang Y, Liu L, Chen D, Pang Y, Xu X, Liu J, et al. Development and validation of a novel nomogram predicting pseudohypoxia type pheochromocytomas and paragangliomas. J Endocrinol Invest (2022) 46(7):1361–71. doi: 10.1007/s40618-022-01984-3
54. Hescot S, Curras-Freixes M, Deutschbein T, van Berkel A, Vezzosi D, Amar L, de la Fouchardière C, et al. Prognosis of Malignant pheochromocytoma and paraganglioma (MAPP-prono study): A european network for the study of adrenal tumors retrospective study. J Clin Endocrinol Metab (2019) 104(6):2367–74. doi: 10.1210/jc.2018-01968
Keywords: SDHB mutations, pheochromocytomas and paragangliomas, prediction model, PPGLs, genetic testing
Citation: Zhou Y, Gao Y, Ma X, Li T, Cui Y, Wang Y, Li M, Zhang D and Tong A (2023) Development and internal validation of a novel predictive model for SDHB mutations in pheochromocytomas and retroperitoneal paragangliomas. Front. Endocrinol. 14:1285631. doi: 10.3389/fendo.2023.1285631
Received: 30 August 2023; Accepted: 20 November 2023;
Published: 21 December 2023.
Edited by:
Mirko Parasiliti-Caprino, University of Turin, ItalyReviewed by:
Delmar Muniz Lourenco Jr., University of São Paulo, BrazilCopyright © 2023 Zhou, Gao, Ma, Li, Cui, Wang, Li, Zhang and Tong. This is an open-access article distributed under the terms of the Creative Commons Attribution License (CC BY). The use, distribution or reproduction in other forums is permitted, provided the original author(s) and the copyright owner(s) are credited and that the original publication in this journal is cited, in accordance with accepted academic practice. No use, distribution or reproduction is permitted which does not comply with these terms.
*Correspondence: Anli Tong, dG9uZ2FubGlAaG90bWFpbC5jb207; dG9uZ2FsQHB1bWNoLmNu; Dingding Zhang, ZGluZ2Rpbmd6aDE5ODdAMTYzLmNvbQ==
†ORCID: Dingding Zhang, orcid.org/0000-0002-5234-752X
Anli Tong, orcid.org/0000-0002-1418-1012
Disclaimer: All claims expressed in this article are solely those of the authors and do not necessarily represent those of their affiliated organizations, or those of the publisher, the editors and the reviewers. Any product that may be evaluated in this article or claim that may be made by its manufacturer is not guaranteed or endorsed by the publisher.
Research integrity at Frontiers
Learn more about the work of our research integrity team to safeguard the quality of each article we publish.