- 1Section on Endocrinology and Genetics, Program on Developmental Endocrinology and Genetics, Eunice Kennedy Shriver National Institute of Child Health and Human Development (NICHD), Bethesda, MD, United States
- 2Biostatistics and Clinical Epidemiology Service, National Institutes of Health (NIH) Clinical Center, Bethesda, MD, United States
- 3Molecular Genomics Core, National Institute of Child Health and Human Development (NICHD), Bethesda, MD, United States
- 4Section on Pediatric Endocrinology and Diabetes, Yale University, New Haven, CT, United States
- 5Department of Medicine and Health Sciences, “V. Tiberio” University of Molise, Campobasso, Italy
- 6Human Genetics and Precision Medicine, Institute for Molecular Biology and Biotechnology (IMBB), Foundation for Research & Technology Hellas (FORTH), Heraklion, ELPEN Research Institute, Athens, Greece
Introduction: Pediatric obesity has steadily increased in recent decades. Large-scale genome-wide association studies (GWAS) conducted primarily in Eurocentric adult populations have identified approximately 100 loci that predispose to obesity and type II diabetes. GWAS in children and individuals of non-European descent, both disproportionately affected by obesity, are fewer. Rare syndromic and monogenic obesities account for only a small portion of childhood obesity, so understanding the role of other genetic variants and their combinations in heritable obesities is key to developing targeted and personalized therapies. Tight and responsive regulation of the cAMP-dependent protein kinase (PKA) signaling pathway is crucial to maintaining healthy energy metabolism, and mutations in PKA-linked genes represent the most common cause of monogenic obesity.
Methods: For this study, we performed targeted exome sequencing of 53 PKA signaling-related genes to identify variants in genomic DNA from a large, ethnically diverse cohort of obese or metabolically challenged youth.
Results: We confirmed 49 high-frequency variants, including a novel variant in the PDE11A gene (c.152C>T). Several other variants were associated with metabolic characteristics within ethnic groups.
Discussion: We conclude that a PKA pathway-specific variant search led to the identification of several new genetic associations with obesity in an ethnically diverse population.
Introduction
Over the past decade, several genome-wide association studies (GWAS) in adults, but only a few in pediatric patient cohorts, have been conducted to uncover genetic loci associated with obesity and other increasingly prevalent pediatric metabolic diseases including non-alcoholic fatty liver disease (NAFLD) and type II diabetes (T2D). From these studies, approximately 100 identified genetic loci have been associated with body mass index (BMI) variability, the main correlating factor to obesity used in GWAS (1). However, investigation into the translatability of genetic risk loci in adults to pediatric patients has been spotty, with few genetic loci being associated with BMI variability in pediatric patients (1). While obesity is the most important risk factor for NAFLD, not all obese children develop NAFLD, demonstrating the importance of genetic factors in disease susceptibility. Additionally, most large-scale studies and genetic databanks are Eurocentric (2, 3), despite the 58% and 49% increased prevalence of obesity among Hispanic and non-Hispanic Black compared to non-Hispanic White children in the U.S (4). With the alarming, unabated increase in prevalence of childhood and adolescent obesity and other metabolic diseases, thorough investigation of all avenues leading to understanding the mechanistic underpinnings and genetic contributors is warranted.
The prevalence of T2D increased from 0.34 to 0.67 per 1,000 youths aged 10 to 19 years between 2001 and 2017, with the greatest increases observed in non-Hispanic Black and Hispanic youths (5). The prevalence of T2D is higher among those 10 to 19 years old compared to younger children, in part because of pubertal surges in growth hormone and insulin-like growth factor that promote insulin resistance (6). Youth-onset T2D is typically more aggressive, involves more frequent complications, and is less responsive to treatment than adult-onset T2D. As with NAFLD, obesity is the most important risk factor for T2D, yet not all obese children develop the disease, urging a better understanding of genetic differences in disease susceptibility (7). A number of common genetic loci that predispose to the development of T2D include genes involved in glucose production, insulin signaling or sensitivity, β-cell function, pancreatic development, and those that cause monogenic T2D (8). The use of polygenic risk scores (PRS) to predict T2D risk is an effective tool that gains utility as knowledge of risk variants expands. The cAMP-dependent protein kinase (PKA)/cAMP signaling pathway is an important target for T2D therapy development because of its central role in glucose homeostasis, including insulin and glucagon secretion, glycogen synthesis and breakdown, and gluconeogenesis (9).
NAFLD is the leading cause of chronic liver disease among children and adults and one of the main manifestations of metabolic syndrome (10, 11). NAFLD is a disorder with a wide spectrum, ranging from fat accumulation in >5% of hepatocytes to non-alcoholic steatohepatitis (NASH), steatofibrosis, and potentially cirrhosis, although the progression of disease is not well-documented in children (11). Genetic variation in the gene patatin-like phospholipase domain-containing protein 3 (PNPLA3) confers susceptibility to nonalcoholic fatty liver disease. The PNPLA3 I148M variant, common among Hispanic populations, is associated with two-fold higher hepatic fat content than non-carriers while the S453I variant, common in African Americans (AAs), is associated with decreased hepatic fat (12). Several other well-known variants in the genes transmembrane 6 superfamily member 2 (TM6SF2) (variant: E167K), glucokinase regulator (GCKR), membrane bound O-acyltransferase domain containing 7 gene–transmembrane channel-like 4 (MBOAT7-TMC4) (variant: rs641738), and hydroxysteroid 17-beta dehydrogenase 13 (HSD17B13) (variant: rs72613567:TA), in addition to gut microbiota composition, contribute to NAFLD susceptibility in children (13). The etiology of NAFLD involves genetic and non-genetic factors, including oxidative stress; altered lipid metabolism, cytokines, and adipokines; and mitochondrial dysfunction that promotes inflammation, cell death, and fibrosis, that interact in parallel to increase NAFLD susceptibility (14). Dysregulated hepatic lipid metabolism, a key component of NAFLD, by default involves changes along the PKA/cAMP signaling pathway. Liver-specific PKA inhibition in mice altered the regulation of nearly 100 genes involved in hepatic lipid metabolism and reduced hepatic triglyceride accumulation significantly after chronic high-fat diet exposure (15). Other work showed that hepatic activation of PKA and, in turn, AMP-activated protein kinase (AMPK), through the PKA catalytic subunit α, stimulates lipolysis and protects against dysregulated hepatic lipid metabolism and apoptosis typically induced by free fatty acids or high-fat/high-sugar diet (16). Atherosclerotic cardiovascular disease (ASCVD) is the leading cause of adult morbidity and mortality in developed countries and begins in childhood with subclinical vascular changes (17). Dyslipidemia, diabetes, and obesity are primary risk factors for ASCVD and NAFLD, and the etiologies of both diseases involve combined genetic and lifestyle factors.
With multiple roles in glucose homeostasis and in lipid metabolism, storage, and trafficking, appropriate regulation of PKA/cAMP signaling is a common thread underlying the maintenance of healthy metabolic functions. Targeting PKA and cAMP signaling to develop obesity, T2D and dyslipidemia therapeutics is not a novel idea (18). Nearly 20% of the 361 drugs in clinical trials documented by the U.S Food and Drug Administration in 2017 were compounds targeting G-protein-coupled receptors (GPCRs) that act just upstream of the PKA/cAMP signaling pathway (19). PKA signaling is integral in regulating various aspects of energy homeostasis from adipocyte and liver biology to the central control of intake (20). Because of the intimate relationship between PKA and the maintenance of metabolic health, we sought to identify variants in PKA signaling-related genes (Table 1) that are involved in metabolic regulation among our predominantly obese pediatric cohort. The diversity of the cohort lends strength to the study and highlights the importance of ethnic background on the metabolic phenotype.
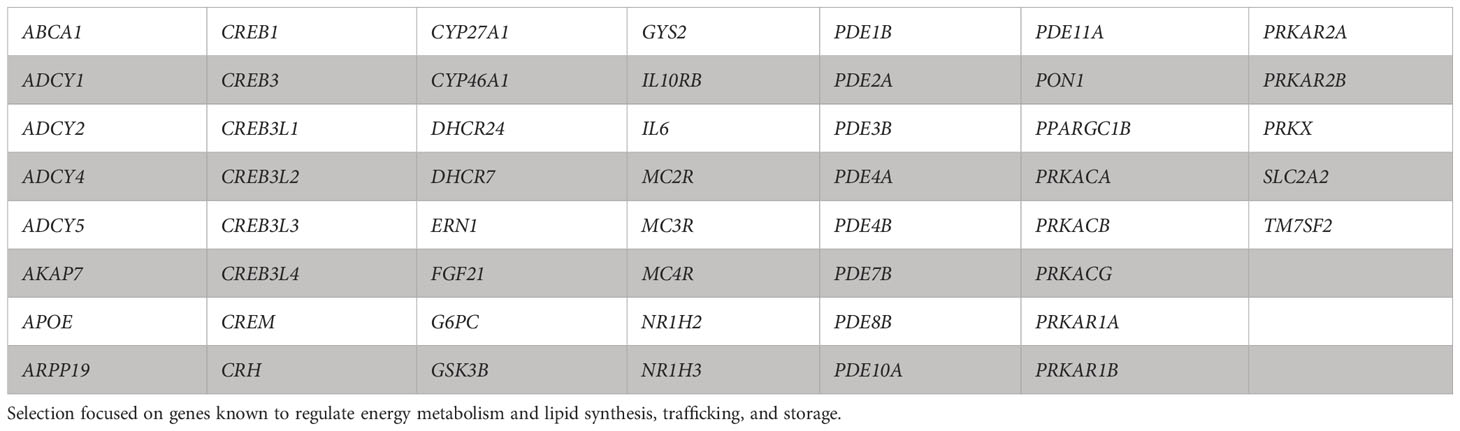
Table 1 Gene list for targeted exome sequencing including PKA subunit genes and other genes coding for proteins involved in the PKA signaling pathway (n = 53).
Methods
Patient studies
The patients in this study were recruited from the Yale Obesity Clinic as part of the Pathogenesis of Youth Onset Diabetes (PYOD) cohort (NCT01967849). Participants were excluded if they had been previously treated for other endocrinopathies, were on any chronic medications known to alter glucose or insulin metabolism, or had been or were currently being treated with oral glucocorticoids, antirejection medication, or chemotherapy. Youth with a BMI between the 85th and 95th percentiles were defined overweight and youth with a BMI greater than the 95th percentile were defined obese. BMI percentiles were derived from age and sex national standards. Our study protocol was approved by the Human Investigations Committee of the Yale School of Medicine. Participants provided assent and parents provided written informed consent to participate in the study.
Cohort demographics and clinical features
Genomic DNA from a cohort of 460 patients was sequenced and association analyses based on known gene functions were performed. Data for 86 clinical parameters were available for most patients including hepatic fat fraction (HFF); anthropometric data; blood pressure; fasting levels of glucose, insulin, and C-peptide and their levels during oral glucose tolerance test (OGTT); homeostatic model assessment (HOMA), whole-body insulin sensitivity index (WBISI), insulinogenic index (IGI), disposition index (DI), and glycosylated hemoglobin (HbA1c); expanded cholesterol, chylomicron, and triglyceride values including very-low-density lipoprotein (VLDL), high-density lipoprotein (HDL), and low-density lipoprotein (LDL) size; serum glutamic oxaloacetic transaminase (SGOT), serum glutamic pyruvate transaminase (SGPT), gamma glutamyl transferase (γ-GTT), alkaline phosphatase; and apolipoprotein B (ApoB).
Clinical data collection
Magnetic resonance imaging (MRI) studies were performed on a GE or Siemens Sonata 1.5 Tesla system. Measurement of liver fat content was performed by MRI using the two-point Dixon (2PD) method as modified by Fishbein et al. (21). HFF% was calculated in duplicate from the mean pixel signal intensity data using the formula: [(Sin−Sout)/(2×Sin)]×100. The imaging parameters included the following: matrix size = 128 × 256, flip angle = 30°, TR = 18 ms, TEs = 2.38/4.76 ms out-of-phase and in-phase, respectively, bandwidth = 420 Hz/pixel, six averages, slice thickness = 10 mm, one slice, 2.3 s/slice (for 2 points), scan time = 14 s in a single breath-hold.
A standard OGTT (1.75 g/kg body weight, up to 75 g) was performed. Blood samples were drawn at −15-, 0-, 30-, 60-, 90-, 120-, and 180-min time points for determination of glucose, insulin, and C-peptide. Insulin sensitivity was assessed by the WBISI (22). The WBISI was calculated as 10,000/square root of [(fasting glucose × fasting insulin) × (mean glucose × mean insulin during OGTT)] (2). The IGI, an index of early-phase insulin secretion, was calculated as: IGI = insulin (0–30 min) in microunits per milliliter divided by the glucose (0–30 min) in milligrams per deciliter. The DI, which provides a picture of the beta cell response in an insulin-resistant milieu, was calculated as the product of the IGI and the WBISI, based on the curvilinear relation of these OGTT-derived parameters previously described by our group in obese children and adolescents (22, 23).
Plasma glucose was determined using a glucose analyzer by the glucose oxidase method (Beckman Instruments, Brea, CA). Plasma insulin was measured by the Linco radioimmunoassay, lipid levels were determined with an auto-analyzer (model 747-200), and liver enzymes were measured using standard automated kinetic enzymatic assays.
Fasting plasma samples were obtained to determine lipoprotein particle concentrations and sizes. The analyses were conducted with a 400-MHz proton NMR analyzer at Liposcience (Raleigh, NC), and the methodology used has been previously described (24). Briefly, each lipoprotein subclass concentration was measured by the amplitudes of the characteristic lipid-methyl group NMR signals that they emit. The intensity of each signal was proportional to the quantity of the subclass, which was reported in particle concentration units (nmol/L for VLDL and LDL and μmol/L for HDL). VLDL, LDL, and HDL were separated into 10 subclass categories: large VLDL (including chylomicrons) (>60 nm), medium VLDL (35–60 nm), small VLDL (27–35 nm), intermediate-density lipoprotein (IDL) (23–27 nm), large LDL (21.2–23 nm), medium-small LDL (19.8–21.2 nm), very small LDL (18–19.8 nm), large HDL (8.8–13 nm), medium HDL (8.2–8.8 nm), and small HDL (7.3–8.2 nm). Average particle sizes were computed as the sum of the diameter of each subclass multiplied by its relative mass percentage as estimated from the amplitude of its methyl NMR signal.
Classification of NAFLD, glucose tolerance status, and dyslipidemia
NAFLD was defined as having greater than or equal to 5% of hepatocytes containing macrovesicular fat (HFF% ≥ 5) as quantified by the MRI method previously described. Glucose tolerance status was considered normal, impaired, or T2D at the following fasting glucose levels: 60–100 mg/dL, 100–125 mg/dL, and greater than 125mg/dL. Dyslipidemia was characterized as having any of the following: total cholesterol of greater than or equal to 200 mg/dL; low density lipoprotein (LDL) cholesterol of greater than or equal to 130 mg/dL; triglycerides greater than or equal to 100 mg/dL (0–9 years old) and greater than or equal to 130 mg/dL (10–18 years old); or high-density lipoprotein (HDL) cholesterol less than 40 mg/dL.
Targeted exome sequencing and data compilation
Targeted exome sequencing and its pursuant data compilation were completed as previously described (25). In short, custom amplicons for 53 PKA signaling-related genes (Table 1) were created with Illumina DesignStudio; TruSeq Custom Amplicon Library Preparation Kit was used to generate the cDNA libraries that were then sequenced with MiSeq (Illumina; v2 chemistry). Call sets were generated and annotated as previously reported (25).
Data processing and in silico prediction
All data compiled from the targeted exome sequencing was systematically reviewed and categorized as described before (25). Briefly, only variants with a high allele frequency (defined as a frequency greater than or equal to 0.004) and a predicted high or moderate physiological impact were further investigated. The database Varsome.com was used to gather detailed information on variants of interest and Integrative Genomics Viewer (IGV_2.4.16, Broad Institute) was used to determine the legitimacy of variants. Variants of interest that passed this scrutiny were confirmed with Sanger sequencing.
Statistical analyses
Data are described using frequency (percentages), and simple descriptive statistics of mean ± standard deviation (SD) or median (inter-quartile range, IQR). Data were assessed for distributional assumptions and analyzed accordingly. Mixed models were used for comparing demographic and outcome data by race/ethnicity reported in Table 2. In addition, they tested associations between the main effect of race/ethnicity and outcomes (e.g., WBSI, HDL, and VLDL) and adjusted for appropriate covariates (e.g., BMI and NAFLD) as necessary. Statistical evidence was based on effect sizes, variability, and p-values. Data were analyzed using SAS v9.4 (SAS Institute, Inc, Cary, NC).
Results
Cohort demographics and metabolic characteristics
In our cohort, 38% of patients (n = 175) were Caucasian, 26.7% (n = 118) were AA, 30% (n = 138) were Hispanic, and 6.3% (n = 29) were Asian or “other” (Table 2). Female and male patients represented 56.7% and 43.3% of the patient cohort, respectively (p < 0.001). Median patient age was 13.3 (IQR 11.1–16.0) years old. Within the cohort, 36.0% of patients had NAFLD, 20.9% had impaired glucose tolerance, 2.6% had T2D, and 50.3% had dyslipidemia. Most of the cohort was obese (81.8%) with a median BMI percentile of 98.7. Finally, 139 patients harbored at least one confirmed variant in the targeted PKA signaling-related genes (Table 3). Of these patients, 18% (n = 25) were Caucasian, 58% (n = 81) were AA, 21% (n = 29) were Hispanic, and 3% (n = 4) were Asian or “other”. Breakdown by sex revealed that 58% (n = 81) of variant-harboring patients were female and 42% (n = 58) of variant-harboring patients were male. While the ratio by sex among Hispanic variant-harboring patients was 38% male (n = 11) to 62% female (n = 18), ratios by sex among other ethnic groups followed the trend seen in the total variant-harboring patient population.
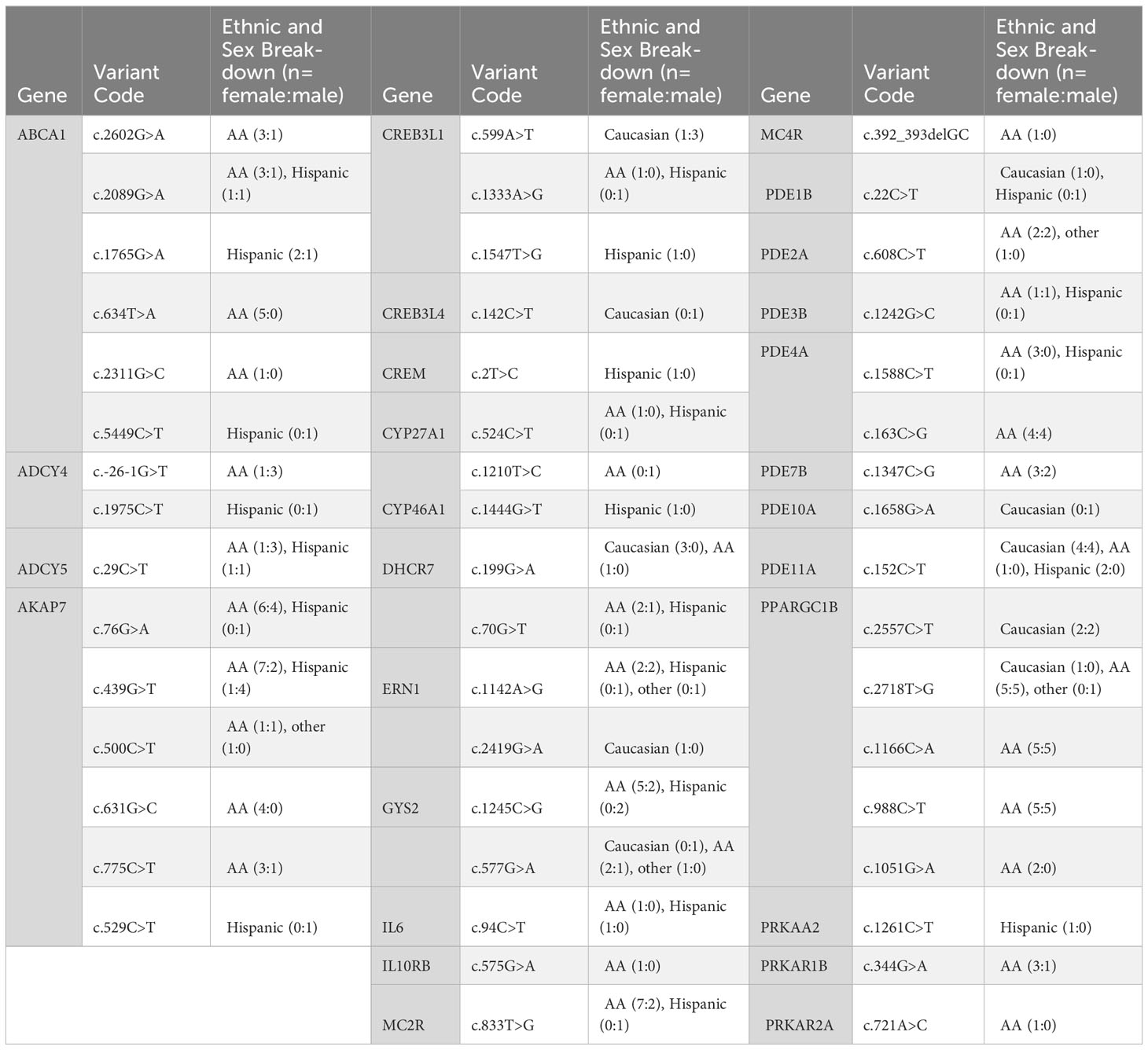
Table 3 Complete list of confirmed variants and their breakdown by ethnic group and sex, (n= female:male).
BMI percentile did not differ among racial/ethnic groups, nor did obesity, total body fat, waist circumference, or HOMA. In line with previous reports, Hispanic youth were disproportionately affected by NAFLD (26), and AAs were least affected (27). In our cohort, 36% of all patients had NAFLD, yet 43.1% of Hispanic and 29.7% of AA youth had NAFLD (Table 2). A total of 20.9% of patients had impaired glucose tolerance and 2.2% of patients had T2D that were similarly distributed among racial groups. Median DI was highest in the AA group [7.2 (IQR 4.2–11.3)] relative to the medians of the other racial groups (p = 0.043) or the entire cohort [5.7 (IQR 3.7–8.7)]. Visceral fat differed substantially by race (p = 0.002) and was highest in Caucasian and Hispanic groups [medians 54.2 (IQR 35.6–75.8) cm2 and 53 (IQR 37.2–75.4) cm2, respectively] and lowest in AAs [40.3 (IQR 24.1–59.2) cm2]. Among the cohort, 50.3% had dyslipidemia and there was an uneven distribution across races (p = 0.006), with 50.3%, 40.4%, 61.2%, and 38.5% of Caucasians, AAs, Hispanics, and “others” affected, respectively. The median circulating triglyceride value in our cohort was 85 (IQR 61–126) mg/dL, yet was only 64 (IQR 49–95) mg/dL in the AA group (p < 0.001).
In silico prediction analyses
Sequencing followed by in silico prediction analyses identified 49 potentially meaningful variants based on the previously described criteria. Of these, 29 variants occurred in at least three patients in our cohort and were thus considered high-frequency variants and subjected to focused correlation analysis based on known gene function (Table 3).
Association analyses of high-frequency variants
We performed focused association analyses among high-frequency variants within racial/ethnic groups. This entailed assessing potential effects only among clinical parameters relevant to known gene functions. Analyses included variants identified in the following genes: ATP binding cassette subfamily A member 1 (ABCA1), A-kinase anchoring protein 7 (AKAP7), glycogen synthase 2 (GYS2), melanocortin 2 receptor (MC2R), phosphodiesterase 4A (PDE4A), phosphodiesterase 11A (PDE11A), peroxisome proliferator-activated receptor gamma (PPARG) and coactivator 1 beta (PPARGC1B), and protein kinase cAMP-dependent type I regulatory subunit beta (PRKAR1B). While several other variants were identified, analyses were not performed when there were less than three individuals with any one variant within the same ethnic group. Four variants in the ABCA1 gene were identified exclusively among AA and Hispanic patients: c.2602G>A, c.1765G>A, c.2089G>A, and c.634T>A. Presence of the c.2602G>A variant in four AA patients was associated with higher WBISI, indicating improved insulin sensitivity [5.34 ± 2.52 vs. 1.96 ± 1.39 in the AA without; p < 0.001]. The ABCA1 c.2089G>A variant, present in 4 AA patients, was associated with increased visceral adipose tissue (AT) [66.0 ± 24.0 cm2 vs. 42.6 ± 25.3 cm2 in those without the variant; p = 0.014]. The two Hispanic patients confirmed to have the c.2089G>A variant had a mean BMI percentile of 99.67 compared to 93.58 in the entire Hispanic cohort, but the statistical analysis was underpowered for meaningful comparisons. No associations were found with the ABCA1 c.1765G>A variant that was identified in three Hispanic patients. The ABCA1 c.634T>A variant was confirmed in five AA patients, three of whom also harbored the PRKAR1B c.344G>A variant that was found to alter PKA Cα interaction with PKA regulatory subunits and was associated with a favorable lipoprotein profile (25). After adjusting for the presence of PRKAR1B c.344G>A, ABCA1 c.634T>A was associated with increased total small LDL particles (556.7 ± 601.5 mg/dL vs. 536.4 ± 263.9 mg/dL without; p = 0.014); its associations with several other lipid biomarkers approached significance but were not supported.
The AKAP7 c.439G>T variant was identified exclusively in AA (n = 9) and Hispanic patients (n = 5). Among AA patients, c.439G>T was associated with higher basal insulin levels [medians 60.7 (IQR 46.9–74.5) pmol/L vs. 37.0 (IQR 32.4–41.7) pmol/L without the variant; p = 0.001], higher 30-min insulin during OGTT [411.2 (IQR 281.7–540.7) mg/dL vs. 270.0 (IQR 226.0–313.9) mg/dL without; p = 0.033], and higher insulin AUC during OGTT [321.2 (IQR 222.4–420.0) mg/dL vs. 216.4 (IQR 182.8–250.0) mg/dL without; p = 0.038]. Hispanic youth who harbored the AKAP7 c.439G>T variant had a median body fat percentage of 33.8% (IQR 28.2–39.4%) compared to 40.0% (IQR 38.8–41.1%; p = 0.035) in those without. AKAP7 c.76G>A was confirmed in nine AA and one Hispanic patient. Among the AA cohort, it was associated with higher HDL [medians 46.4 (IQR 39.6–53.1) mg/dL vs. 38.1 (IQR 34.9–41.3) mg/dL in those without; p = 0.021] and lower IDL [medians 10.8 mg/dL (IQR −12.0-33.5) mg/dL vs. 35.7 (IQR 23.4–48.0) mg/dL; p = 0.044].
PDE11A c.152C>T was confirmed in 11 patients, 8 of whom were Caucasian, 1 was AA, and another 2 were Hispanic. Among Caucasian youth, the c.152C>T variant was associated with higher glucose AUC values [medians 155.2 (IQR 133.0–177.4) mg/dL vs. 131.4 (IQR 126.1–136.6) mg/dL in those without; p = 0.040] obtained during OGTT. HFF in the two Hispanic patients with this variant tended to be higher, but analysis was underpowered [20.0% (IQR 9.6–30.4%) vs. 9.9% (IQR 8.6–11.1%); p = 0.058].
Several variants in the PPARGC1B gene were confirmed in AA youth: c.988C>T (n = 10), c.1051G>A (n = 2), c.1166C>T (n = 10), and c.2718T>G (n = 10). Additionally, PPARGC1B c.2557C>T was confirmed in four Caucasian youth; one Caucasian and one “other” had the c.2718T>G variant. The PPARGC1B c.988C>T and c.1166C>A variants were concomitantly harbored by the same 10 patients, with one of these individuals being homozygous for both variants. Presence of PPARGC1B c.988C>T and c.1166C>A variants was positively associated with higher insulin AUC [medians 308.4 (IQR 221.7–395.1) pmol/L vs. 214.0 (IQR 180.0–248.0) pmol/L in those without; p = 0.037] during OGTT. Presence of c.2718T>G among AA was associated with older age at the time of enrollment in the clinical protocol [medians 16.5 (IQR 14.6–18.5) years vs. 13.3 (IQR 12.5–14.1) years old in those without; p = 0.002] and with increased subcutaneous AT [medians 678.3 (IQR 553.8–802.8) cm2 vs. 467.8 (IQR 417.5–518.0) cm2 in those without; p = 0.001] and increased visceral AT [median 64.6 (IQR 48.8–80.3) cm2 vs. 44.1 (IQR 37.7–50.5) cm2 without; p = 0.011]. The patient homozygous for c.2718T>G had 1,134.2 cm2 and 93.4 cm2 of subcutaneous and visceral AT, respectively.
MC2R c.833T>G was confirmed in a total of 10 patients (9 AA, 1 Hispanic), 1 of whom was homozygous for the variant. Analyses within the AA cohort revealed a tendency to be younger at the time of entry into the clinical protocol [median 11.7 (IQR 9.6–13.8) years old vs. 13.7 (IQR 13.0–14.5) years old in those without; p = 0.052]. The presence of the c.833T>G variant was associated with increased HFF [medians 11.3% (IQR 8.4–14.2%) vs. 8.2% (IQR 7.1–9.2%) in those without; p = 0.032] and SGOT [median 25.6 (IQR 21.5–29.7) vs. 21.4 (IQR 19.8–23.0) without; p = 0.044]. The variant was also associated with higher total cholesterol [medians 172.1 (IQR 154.9–189.2) mg/dL vs. 153.6 (IQR 147.1–160.2) mg/dL without; p = 0.037] and small VLDL [medians 36.4 (IQR 25.8–47.0) mg/dL vs. 24.8 (IQR 20.6–29.1) mg/dL without; p = 0.027]. Associations with elevated ApoB and alkaline phosphatase levels were not supported by the data (p = 0.089 and p = 0.086, respectively). The GYS2 c.1245C>G was confirmed in seven AA and two Hispanic patients and PDE4A c. 163C>G was confirmed in 7 AA patients, but neither was associated with any of the clinical parameters examined.
Discussion
Among the panel of 53 PKA signaling pathway-related genes for which we performed targeted exome sequencing, we confirmed 49 variants in our pediatric cohort that included 29 that met criteria for high-frequency allele classification (Table 3). In most cases, and while relatively small in sample sizes, the variants segregated neatly by racial/ethnic background, enabling us to perform association analyses that exploited the unique metabolic characteristics of each ethnic group. We did not examine non-coding variants that might also contribute to genetic regulation of the target or neighboring genes. Analyses based on sex within each racial/ethnic background were not performed due to the diminished power of any conducted analyses on these further reduced sample sizes.
It is important to note that biological sex is a major factor influencing metabolism. Additionally, sexual dimorphism in the regulation of PKA signaling is well documented in Cushing syndrome, in which prevalence in female patients is nearly twofold that in male patients (28), and in glucocorticoid regulation of metabolism (29). Numerous human and animal studies have shown that large portions of genes are differentially expressed in female and male patients in a variety of tissues including adipose, liver, and brain, with female patients tending to have more favorable metabolic profiles (30), yet in the US, more adult women than men are obese or severely obese (31). Also relevant to this study, mouse models have demonstrated extensive sex differences in PKA signaling regulation of energy balance. Global knockout of the PKA regulatory subunits RIIβ (Prkar2b) (32), RIIα (Prkar2a) (33, 34), and the catalytic subunit beta (Prkacb) (35) in mice all caused distinct forms of diet-induced obesity resistance that conveyed a range of sex-dependent phenotypic characteristics.
In mice, targeted deletion of Abca1 with an adiponectin-Cre driver led to decreased adipocyte size and weight gain after high-fat diet feeding, supporting a role for ABCA1 in adipocyte biology and in maintenance of energy balance (36). In a cohort of Northwest Mexican adults, single-nucleotide polymorphisms (SNPs) in the ABCA1 gene as well as FTO alpha-ketoglutarate-dependent dioxygenase (FTO), adrenoceptor beta 3 (ADRB3), and PPARG were associated with increased risk of obesity (37). While ABCA1 variant c.2602G>A was associated with improved insulin sensitivity in AAs in our cohort, the c.2089G>A variant was associated with increased visceral AT, and c.634T>A, identified only in a subgroup of Hispanic youth, was associated with elevated small LDL cholesterol levels, exemplifying the messy interplay of genetic variation.
A-kinase anchoring proteins (AKAPs) and phosphodiesterases (PDEs) represent two important classes of molecules in the regulation of PKA signaling that play roles in the subcellular localization of the PKA enzyme and regulation of cAMP levels, respectively. AKAP genes have been studied in the pathophysiology of cardiovascular disease (38), and while the role of AKAPs in metabolic syndrome and obesity is not entirely clear, their importance in the spatiotemporal regulation of PKA signaling centrally and peripherally implicates these proteins indirectly (39). Deletion of Akap1 in mice, for example, conferred resistance to diet-induced obesity and increased energy expenditure and brown AT thermogenesis in obese mice (39). Additionally, disruption of either of the PKA type II regulatory subunits, Prkar2b (40) and Prkar2a (33), known for their interactions with AKAPs, cause resistance to diet-induced obesity in mice (by different mechanisms).
PDE11A defects have been linked to several cancers (adrenal, testicular, and prostate) and to major depression, bipolar disorder, and asthma, implicating specific PDE11A inhibitors as potential drug targets (41). Inactivating mutations in PDE11A cause isolated macronodular adrenal hyperplasia and Cushing syndrome that is characterized by central obesity and a panel of metabolic sequelae (42), confirming a role for this gene in metabolic regulation and energy balance. Inverse correlations between BMI and PDE activities have been observed in subcutaneous and omental AT (43) and inhibition of PDE4 has been identified as a therapeutic target for obese women with polycystic ovary syndrome (44). In our cohort, the novel c.152C>T variant in the PDE11A gene was associated with higher glucose AUC in Caucasian youth.
Over 150 different variants in the melanocortin-4 receptor (MC4R) gene that lead to impaired receptor function have been identified, which constitute the most common cause of monogenic obesity (45). Activation of MC4R potently inhibits feeding without altering activity or locomotion (46). We only identified one patient with an MC4R variant that did not permit correlation analysis, but not surprisingly, this patient was only 8.5 years old and had a BMI percentile of 99.3. While the homozygous or multiple heterozygous variants in melanocortin-2 (MC2R) have been associated with familial glucocorticoid deficiency due to unresponsiveness to adrenocorticotropic hormone (ACTH), (https://omim.org/entry/202200), we found that the c.833T>G variant was associated with the younger age of patients, suggesting more severe phenotype and earlier diagnosis. The AA patients harboring the MC2R c.833T>G variant additionally had higher HFF and less favorable lipoprotein profiles.
No functional data were available for the PPARGC1B variants (ClinVar), but variants in this gene have been associated with obesity in both pediatric and adult populations. In fact, recent research into microRNA-based therapeutics inhibiting a constellation of genes, including Ppargc1b in mice, has shown beneficial results protecting against diet-induced obesity (47). Our data associated the c.2718T>G variant and the combination of c.988C>T and c.1166C>A variants with increased subcutaneous and visceral fat mass and elevated insulin levels, respectively. While several other variants were identified, we were not able to perform association analyses on all due to the limited number of affected individuals either in total or within ethnic groupings (Table 3).
A refined understanding of the genetic variations within different racial/ethnic backgrounds that predispose or protect children and teens from obesity and other metabolic complications can improve early interventions and the identification of higher-risk subgroups. Because of the multifactorial nature of obesity and metabolic diseases, screening high-risk individuals represents an additional form of intervention along with promoting healthy lifestyle choices and pharmacologic therapies. Limitations include sample sizes that in some cases did not permit definitive comparisons and the lack of evaluation of these variants in cell or animal models. Despite these shortcomings, here we identified almost 50 variants linked to PKA pathway signaling regulation of metabolism, which is a primary regulator of energy balance, adipocyte function, glucose metabolism, and lipid storage and trafficking.
Data availability statement
All data generated and analyzed during this study are included in this published article or in the following data repository: doi: 10.6084/m9.figshare.14120888.v1 / https://figshare.com/articles/figure/PKA_NAFLD_Supplemental_Data_docx/14120888/1.
Ethics statement
The studies involving humans were approved by the Human Investigations Committee of the Yale School of Medicine. The studies were conducted in accordance with the local legislation and institutional requirements. Written informed consent for participation in this study was provided by the participants’ legal guardians/next of kin. Written informed consent was obtained from the individual(s), and minor(s)’ legal guardian/next of kin, for the publication of any potentially identifiable images or data included in this article.
Author contributions
MB: Investigation, Writing – original draft, Writing – review & editing, Formal Analysis. NSi: Formal Analysis, Investigation, Writing – review & editing, Methodology, Validation. FF: Investigation, Methodology, Validation, Writing – review & editing. JI: Investigation, Methodology, Writing – review & editing, Formal Analysis. SLC: Investigation, Methodology, Writing – review & editing, Project administration, Validation. SC: Writing – review & editing, Conceptualization, Funding acquisition, Supervision. NSa: Conceptualization, Funding acquisition, Writing – review & editing, Investigation, Methodology, Project administration. CS: Conceptualization, Funding acquisition, Writing – review & editing. EL: Conceptualization, Writing – review & editing, Data curation, Investigation, Project administration, Supervision, Validation, Writing – original draft.
Funding
The author(s) declare financial support was received for the research, authorship, and/or publication of this article. This work was in part supported by the research project Z01-HD008920 (PI: CS) of the Intramural Research Program of the Eunice Kennedy Shriver National Institute of Child Health and Human Development. SC is funded by R01HD028016 and R01DK111038, and NS is funded by R01DK114504 and R01MD015974. The clinical studies described were made possible also by the NIH National Center for Advancing Translational Science (CTSA grant UL1 TR001863) and the Diabetes Research Center at Yale (grant P30DK045735).
Conflict of interest
The authors declare that the research was conducted in the absence of any commercial or financial relationships that could be construed as a potential conflict of interest.
Publisher’s note
All claims expressed in this article are solely those of the authors and do not necessarily represent those of their affiliated organizations, or those of the publisher, the editors and the reviewers. Any product that may be evaluated in this article, or claim that may be made by its manufacturer, is not guaranteed or endorsed by the publisher.
Author disclaimer
The contents of this publication are solely the responsibility of the authors and do not necessarily represent the official view of the NIH.
References
1. Locke AE, Kahali B, Berndt SI, Justice AE, Pers TH, Day FR, et al. Genetic studies of body mass index yield new insights for obesity biology. Nature (2015) 518(7538):197–206. doi: 10.1038/nature14177
2. Chen J, Spracklen CN, Marenne G, Varshney A, Corbin LJ, Luan J, et al. The trans-ancestral genomic architecture of glycemic traits. Nat Genet (2021) 53(6):840–60. doi: 10.1038/s41588-021-00852-9
3. Langenberg C, Lotta LA. Genomic insights into the causes of type 2 diabetes. Lancet (2018) 391(10138):2463–74. doi: 10.1016/S0140-6736(18)31132-2
4. Stierman BA, Carroll MD, Chen T-C, Davy O, Fink S, Fryar CD, et al. “National health and nutrition examination survey 2017–march 2020 prepandemic data files—development of files and prevalence estimates for selected health outcomes” in National Health Statistics Reports; No. 158 (Hyattville, MD: National Center for Health) (2021). doi: 10.15620/cdc:106273.
5. Lawrence JM, Divers J, Isom S, Saydah S, Imperatore G, Pihoker C, et al. Trends in prevalence of type 1 and type 2 diabetes in children and adolescents in the US, 2001-2017. JAMA (2021) 326(8):717–27. doi: 10.1001/jama.2021.11165
6. Flint A, Arslanian S. Treatment of type 2 diabetes in youth. Diabetes Care (2011) 34 Suppl 2(Suppl 2):S177–83. doi: 10.2337/dc11-s215
7. Todd JN, Srinivasan S, Pollin TI. Advances in the genetics of youth-onset type 2 diabetes. Curr Diabetes Rep (2018) 18(8):57. doi: 10.1007/s11892-018-1025-1
8. Valaiyapathi B, Gower B, Ashraf AP. Pathophysiology of type 2 diabetes in children and adolescents. Curr Diabetes Rev (2020) 16(3):220–9. doi: 10.2174/1573399814666180608074510
9. Yang H, Yang L. Targeting cAMP/PKA pathway for glycemic control and type 2 diabetes therapy. J Mol Endocrinol (2016) 57(2):R93–R108. doi: 10.1530/JME-15-0316
10. Nobili V, Alisi A, Newton KP, Schwimmer JB. Comparison of the phenotype and approach to pediatric vs adult patients with nonalcoholic fatty liver disease. Gastroenterology (2016) 150(8):1798–810. doi: 10.1053/j.gastro.2016.03.009
11. Nobili V, Alisi A, Valenti L, Miele L, Feldstein AE, Alkhouri N. NAFLD in children: new genes, new diagnostic modalities and new drugs. Nat Rev Gastroenterol Hepatol (2019) 16(9):517–30. doi: 10.1038/s41575-019-0169-z
12. Romeo S, Kozlitina J, Xing C, Pertsemlidis A, Cox D, Pennacchio LA, et al. Genetic variation in PNPLA3 confers susceptibility to nonalcoholic fatty liver disease. Nat Genet (2008) 40(12):1461–5. doi: 10.1038/ng.257
13. Riccio S, Melone R, Vitulano C, Guida P, Maddaluno I, Guarino S, et al. Advances in pediatric non-alcoholic fatty liver disease: From genetics to lipidomics. World J Clin Pediatr (2022) 11(3):221–38. doi: 10.5409/wjcp.v11.i3.221
14. Noureddin M, Sanyal AJ. Pathogenesis of NASH: the impact of multiple pathways. Curr Hepatol Rep (2018) 17(4):350–60. doi: 10.1007/s11901-018-0425-7
15. Yang J, Zhang X, Yi L, Yang L, Wang WE, Zeng C, et al. Hepatic PKA inhibition accelerates the lipid accumulation in liver. Nutr Metab (Lond) (2019) 16:69. doi: 10.1186/s12986-019-0400-5
16. Liang C, Li Y, Bai M, Huang Y, Yang H, Liu L, et al. Hypericin attenuates nonalcoholic fatty liver disease and abnormal lipid metabolism via the PKA-mediated AMPK signaling pathway in vitro and in vivo. Pharmacol Res (2020) 153:104657. doi: 10.1016/j.phrs.2020.104657
17. de Ferranti SD, Steinberger J, Ameduri R, Baker A, Gooding H, Kelly AS, et al. Cardiovascular risk reduction in high-risk pediatric patients: A scientific statement from the American heart association. Circulation (2019) 139(13):e603–34. doi: 10.1161/CIR.0000000000000618
18. London E, Stratakis CA. The regulation of PKA signaling in obesity and in the maintenance of metabolic health. Pharmacol Ther (2022) 237:108113. doi: 10.1016/j.pharmthera.2022.108113
19. Hauser AS, Attwood MM, Rask-Andersen M, Schiöth HB, Gloriam DE. Trends in GPCR drug discovery: new agents, targets and indications. Nat Rev Drug Discovery (2017) 16(12):829–42. doi: 10.1038/nrd.2017.178
20. London E, Bloyd M, Stratakis CA. PKA functions in metabolism and resistance to obesity: lessons from mouse and human studies. J Endocrinol (2020) 246(3):R51–64. doi: 10.1530/JOE-20-0035
21. Fishbein MH, Gardner KG, Potter CJ, Schmalbrock P, Smith MA. Introduction of fast MR imaging in the assessment of hepatic steatosis. Magn Reson Imaging (1997) 15(3):287–93. doi: 10.1016/S0730-725X(96)00224-X
22. Yeckel CW, Weiss R, Dziura J, Taksali SE, Dufour S, Burgert TS. Validation of insulin sensitivity indices from oral glucose tolerance test parameters in obese children and adolescents. J Clin Endocrinol Metab (2004) 89(3):1096–101. doi: 10.1210/jc.2003-031503
23. Giannini C, Weiss R, Cali A, Bonadonna R, Santoro N, Pierpont B, et al. Evidence for early defects in insulin sensitivity and secretion before the onset of glucose dysregulation in obese youths: a longitudinal study. Diabetes (2012) 61(3):606–14. doi: 10.2337/db11-1111
24. D'Adamo E, Northrup V, Weiss R, Santoro N, Pierpont B, Savoye M, et al. Ethnic differences in lipoprotein subclasses in obese adolescents: importance of liver and intraabdominal fat accretion. Am J Clin Nutr (2010) 92(3):500–8. doi: 10.3945/ajcn.2010.29270
25. Bloyd M, Settas N, Faucz FR, Sinaii N, Bathon K, Iben J, et al. The PRKAR1B p.R115K variant is associated with lipoprotein profile in African American youth with metabolic challenges. J Endocr Soc (2021) 5(8):bvab071. doi: 10.1210/jendso/bvab071
26. Saab S, Manne V, Nieto J, Schwimmer JB, Chalasani NP. Nonalcoholic fatty liver disease in Latinos. Clin Gastroenterol Hepatol (2016) 14(1):5–12; quiz e9-10. doi: 10.1016/j.cgh.2015.05.001
27. Sherif ZA, Saeed A, Ghavimi S, Nouraie SM, Laiyemo AO, Brim H, et al. Global epidemiology of nonalcoholic fatty liver disease and perspectives on US minority populations. Dig Dis Sci (2016) 61(5):1214–25. doi: 10.1007/s10620-016-4143-0
28. Stratakis CA. Cushing syndrome caused by adrenocortical tumors and hyperplasias (corticotropin- independent Cushing syndrome). Endocr Dev (2008) 13:117–32. doi: 10.1159/000134829
29. Kroon J, Pereira AM, Meijer OC. Glucocorticoid sexual dimorphism in metabolism: dissecting the role of sex hormones. Trends Endocrinol Metab (2020) 31(5):357–67. doi: 10.1016/j.tem.2020.01.010
30. Chella Krishnan K, Mehrabian M, Lusis AJ. Sex differences in metabolism and cardiometabolic disorders. Curr Opin Lipidol (2018) 29(5):404–10. doi: 10.1097/MOL.0000000000000536
31. Hales CM, Fryar CD, Carroll MD, Freedman DS, Ogden CL. Trends in obesity and severe obesity prevalence in US youth and adults by sex and age, 2007-2008 to 2015-2016. JAMA (2018) 319(16):1723–5. doi: 10.1001/jama.2018.3060
32. Nolan MA, Sikorski MA, McKnight GS. The role of uncoupling protein 1 in the metabolism and adiposity of RII beta-protein kinase A-deficient mice. Mol Endocrinol (2004) 18(9):2302–11. doi: 10.1210/me.2004-0194
33. London E, Nesterova M, Sinaii N, Szarek E, Chanturiya T, Mastroyannis SA, et al. Differentially regulated protein kinase A (PKA) activity in adipose tissue and liver is associated with resistance to diet-induced obesity and glucose intolerance in mice that lack PKA regulatory subunit type IIalpha. Endocrinology (2014) 155(9):3397–408. doi: 10.1210/en.2014-1122
34. London E, Wester JC, Bloyd M, Bettencourt S, McBain CJ, Stratakis CA. Loss of habenular Prkar2a reduces hedonic eating and increases exercise motivation. JCI Insight (2020) 5(23):e141670. doi: 10.1172/jci.insight.141670
35. London E, Noguchi A, Springer D, Faidas M, Gavrilova O, Eisenhofer G, et al. The catalytic subunit beta of PKA affects energy balance and catecholaminergic activity. J Endocr Soc (2019) 3(5):1062–78. doi: 10.1210/js.2019-00029
36. Cuffe H, Liu M, Key CC, Boudyguina E, Sawyer JK, Weckerle A, et al. Targeted deletion of adipocyte abca1 (ATP-binding cassette transporter A1) impairs diet-induced obesity. Arterioscler Thromb Vasc Biol (2018) 38(4):733–43. doi: 10.1161/ATVBAHA.117.309880
37. Velazquez-Roman J, Angulo-Zamudio UA, León-Sicairos N, Medina-Serrano J, DeLira-Bustillos N, Villamil-Ramírez H, et al. Association of FTO, ABCA1, ADRB3, and PPARG variants with obesity, type 2 diabetes, and metabolic syndrome in a Northwest Mexican adult population. J Diabetes Complications (2021) 35(11):108025. doi: 10.1016/j.jdiacomp.2021.108025
38. Suryavanshi SV, Jadhav SM, McConnell BK. Polymorphisms/mutations in A-kinase anchoring proteins (AKAPs): role in the cardiovascular system. J Cardiovasc Dev Dis (2018) 5(1):7. doi: 10.3390/jcdd5010007
39. Ji L, Zhao Y, He L, Zhao J, Gao T, Liu F, et al. AKAP1 deficiency attenuates diet-induced obesity and insulin resistance by promoting fatty acid oxidation and thermogenesis in brown adipocytes. Adv Sci (Weinh) (2021) 8(6):2002794. doi: 10.1002/advs.202002794
40. Cummings DE, Brandon EP, Planas JV, Motamed K, Idzerda RL, McKnight GS. Genetically lean mice result from targeted disruption of the RII beta subunit of protein kinase A. Nature (1996) 382(6592):622–6. doi: 10.1038/382622a0
41. Ceyhan O, Birsoy K, Hoffman CS. Identification of biologically active PDE11-selective inhibitors using a yeast-based high-throughput screen. Chem Biol (2012) 19(1):155–63. doi: 10.1016/j.chembiol.2011.12.010
42. Horvath A, Boikos S, Giatzakis C, Robinson-White A, Groussin L, Griffin KJ, et al. A genome-wide scan identifies mutations in the gene encoding phosphodiesterase 11A4 (PDE11A) in individuals with adrenocortical hyperplasia. Nat Genet (2006) 38(7):794–800. doi: 10.1038/ng1809
43. Omar B, Banke E, Ekelund M, Frederiksen S, Degerman E. Alterations in cyclic nucleotide phosphodiesterase activities in omental and subcutaneous adipose tissues in human obesity. Nutr Diabetes (2011) 1(8):e13. doi: 10.1038/nutd.2011.9
44. Jensterle M, Kocjan T, Janez A. Phosphodiesterase 4 inhibition as a potential new therapeutic target in obese women with polycystic ovary syndrome. J Clin Endocrinol Metab (2014) 99(8):E1476–81. doi: 10.1210/jc.2014-1430
45. van den Berg L, van Beekum O, Heutink P, Felius BA, van de Heijning MP, Strijbis S, et al. Melanocortin-4 receptor gene mutations in a Dutch cohort of obese children. Obes (Silver Spring) (2011) 19(3):604–11. doi: 10.1038/oby.2010.259
46. Fan W, Boston BA, Kesterson RA, Hruby VJ, Cone RD. Role of melanocortinergic neurons in feeding and the agouti obesity syndrome. Nature (1997) 385(6612):165–8. doi: 10.1038/385165a0
Keywords: obesity, protein kinase A, pediatric, liver disease, targeted exome sequencing, GPCRs, T2DM, genetics
Citation: Bloyd M, Sinaii N, Faucz FR, Iben J, Coon SL, Caprio S, Santoro N, Stratakis CA and London E (2023) High-frequency variants in PKA signaling-related genes within a large pediatric cohort with obesity or metabolic abnormalities. Front. Endocrinol. 14:1272939. doi: 10.3389/fendo.2023.1272939
Received: 04 August 2023; Accepted: 05 October 2023;
Published: 13 November 2023.
Edited by:
Chunye Zhang, University of Missouri, United StatesReviewed by:
Jianjie Wang, Missouri State University, United StatesShuai Liu, Zhejiang University, China
Copyright © 2023 Bloyd, Sinaii, Faucz, Iben, Coon, Caprio, Santoro, Stratakis and London. This is an open-access article distributed under the terms of the Creative Commons Attribution License (CC BY). The use, distribution or reproduction in other forums is permitted, provided the original author(s) and the copyright owner(s) are credited and that the original publication in this journal is cited, in accordance with accepted academic practice. No use, distribution or reproduction is permitted which does not comply with these terms.
*Correspondence: Michelle Bloyd, YmxveWRAbXVzYy5lZHU=