- 1West China School of Public Health and West China Fourth Hospital, Sichuan University, Chengdu, Sichuan, China
- 2Department of Cardiology, Chinese People's Liberation Army of China (PLA) Medical School, Beijing, China
- 3Department of Biotherapy and National Clinical Research Center for Geriatrics, Cancer Center, West China Hospital, Sichuan University, Chengdu, Sichuan, China
Background: Septic patients with diabetes mellitus (DM) are more venerable to subsequent complications and the resultant increase in associated mortality. Therefore, it is important to make tailored clinical decisions for this subpopulation at admission.
Method: Data from large-scale real-world databases named the Medical Information Mart for Intensive Care Database (MIMIC) were reviewed. The least absolute selection and shrinkage operator (LASSO) was performed with 10 times cross-validation methods to select the optimal prognostic factors. Multivariate COX regression analysis was conducted to identify the independent prognostic factors and nomogram construction. The nomogram was internally validated via the bootstrapping method and externally validated by the MIMIC III database with receiver operating characteristic (ROC), calibration curves, decision curve analysis (DCA), and Kaplan-Meier curves for robustness check.
Results: A total of 3,291 septic patients with DM were included in this study, 2,227 in the MIMIC IV database and 1,064 in the MIMIC III database, respectively. In the training cohort, the 28-day all-cause mortality rate is 23.9% septic patients with DM. The multivariate Cox regression analysis reveals age (hazard ratio (HR)=1.023, 95%CI: 1.016-1.031, p<0.001), respiratory failure (HR=1.872, 95%CI: 1.554-2.254, p<0.001), Sequential Organ Failure Assessment score (HR=1.056, 95%CI: 1.018-1.094, p=0.004); base excess (HR=0.980, 95%CI: 0.967-0.992, p=0.002), anion gap (HR=1.100, 95%CI: 1.080-1.120, p<0.001), albumin (HR=0.679, 95%CI: 0.574-0.802, p<0.001), international normalized ratio (HR=1.087, 95%CI: 1.027-1.150, p=0.004), red cell distribution width (HR=1.056, 95%CI: 1.021-1.092, p=0.001), temperature (HR=0.857, 95%CI: 0.789-0.932, p<0.001), and glycosylated hemoglobin (HR=1.358, 95%CI: 1.320-1.401, p<0.001) at admission are independent prognostic factors for 28-day all-cause mortality of septic patients with DM. The established nomogram shows satisfied accuracy and clinical utility with AUCs of 0.870 in the internal validation and 0.830 in the external validation cohort as well as 0.820 in the septic shock subpopulation, which is superior to the predictive value of the single SOFA score.
Conclusion: Our results suggest that admission characteristics show an optimal prediction value for short-term mortality in septic patients with DM. The established model can support intensive care unit physicians in making better initial clinical decisions for this subpopulation.
Introduction
Sepsis is one of the leading life-threatening conditions caused by the dysregulated host response to infection (1–4). Due to the high incidence and subsequently, mortality risk, sepsis, and septic shock are the major medical problems, which affect millions of critically ill populations (3, 5). Sepsis is frequently observed in aging and cancer as well as immunosuppressive subpopulations (6). Despite recent improvements in diagnosis and treatment (including the use of organ support, antibiotics, and fluid resuscitation), sepsis remains a high hospitalization cost and fatal disease around the world (4, 7). How to make more precise clinical management decisions for septic patients with different comorbidities has raised wide concerns (8, 9).
Notably, diabetes mellitus (DM) is one of the most frequent comorbidities in critically ill patients (10). Globally, the prevalence of DM has quadrupled since the last 80s, which has become the ninth major cause of death (11, 12). The International Diabetes Federation (IDF) suggested that the number of DM would rise to almost 650 million by 2040 (11). To date, compelling evidence showed that patients with DM suffered from an increased risk of various infections (13–15) and represented the predominant population experiencing post-sepsis complications (16, 17). Moreover, septic patients with DM presented worse clinical outcomes during the hospitalization (10, 13, 17). The altered immune response and the hyperglycemia condition further assist the growth of microorganisms, which could lead to a more tough situation in septic patients with DM (15, 18). Therefore, identifying possible strategies to reduce in-hospital mortality and subsequent morbidity in such high-risk subpopulations would bring considerable clinical and social benefits. Several studies have explored the interaction between the blood glucose level or glycosylated hemoglobin or DM and sepsis (19–21). However, the results were inconsistent. Meanwhile, there was a persistent lack of studies on the evaluation of independent prognostic factors and models to guide the clinical decisions on septic patients with DM at initial admission.
To address the research gaps, we aim to determine the significant prognostic factors in septic patients with DM, based on a large-scale intensive care database involving hospitalized patients between 2001 and 2019. Furthermore, we aim to further establish and validate a feasible individualized model to assess ICU physicians to predict short-term mortality in septic patients with DM.
Materials and methods
Database
Two cohorts of septic patients admitted to the ICU coexisted with DM, from a publicly available real-world clinical database named Medical Information Mart for Intensive Care Database III (MIMIC III, version 1.4) and IV (MIMIC IV, version 2.2), and maintained by Beth Israel Deaconess Medical Center in Boston, MA, USA from 2001 to 2019 was included (22). The description of this large-scale database has been published in the previous literature in chapters (23–26). The first author was permitted to extract data from the database after passing the related examination. The reporting of this study followed the Strengthening the Reporting of Observational Studies in Epidemiology (STROBE) guidelines (27).
Ethical approval
The MIMIC-III and MIMIC-IV databases used in our study were approved by the Institutional Review Boards (IRB) of the Massachusetts Institute of Technology and do not contain protected health information. As the clinical data were extracted from the MIMIC-III and MIMIC-IV databases, they did not contain any individually identifiable information. Informed consent and ethical approval were not required from the Ethics Committee of the West China Fourth Hospital.
Study population
Initially, the study population was focused on septic patients with DM. Additionally, the medical records of all adult patients aged at least 18 years admitted to the ICU were analyzed. To reduce selection bias, we only use first-round ICU admission records for patients who were enrolled in the ICU more than once. Patients who were discharged <24 hours and encountered missing variable data (medication information), as well as outcome data (28-day in-hospital mortality), were excluded. The flow chart of the patient selection process is shown in Figure 1.
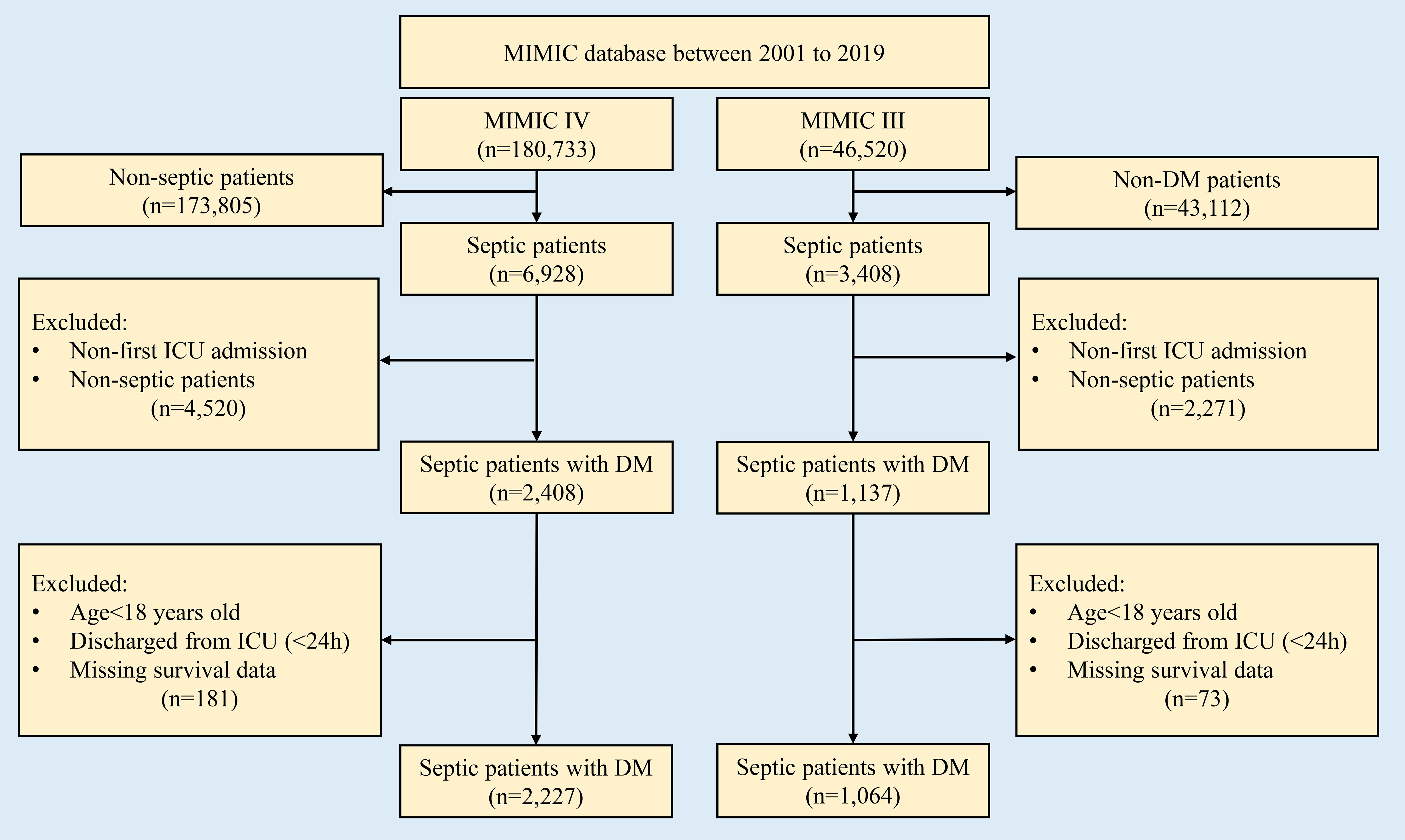
Figure 1 Flow chart for patient selection in this study. DM, diabetes mellitus; MIMIC, Medical Information Mart for Intensive Care Database.
Definition of sepsis, septic shock, and DM
Sepsis is defined as life-threatening organ dysfunction caused by a dysregulated host response to infection (3). The diagnoses of sepsis and septic shock were based on the ‘Third International Consensus Definitions for Sepsis and Septic Shock (sepsis-3)’ (3). The organ dysfunction can be identified as an acute change in total SOFA score ≥2 points consequent to the infection. The SQL language method and the dictionary of codes for the International Classification of Diseases and Ninth Revision (ICD-9) and International Classification of Diseases and Tenth Revision (ICD-10) codes dictionary were used to screen and extract septic and septic shock patients with DM from the MIMIC III and MIMIC IV databases, respectively.
Definition and selection of variables
The clinical characteristics of each patient were collected after admission to the ICU. Variables were divided into categorical and continuous variables. Categorical variables included sex (female and male), race (white, black, and other), weight, comorbidities (heart failure, respiratory failure, liver disease including hepatitis and cirrhosis, renal diseases including chronic kidney disease (CKD) and acute kidney injury (AKI), and malignancy), the medical usage records of continuous renal replacement therapy (CRRT), oxygen, ventilation, antibiotic, dopa, norepinephrine, and fluid input during the ICU stay.
Continuous variables included the age at admission, SOFA score, length of hospital stay, length of ICU stay, total fluids administrations, peripheral blood test data (red blood cell (RBC), platelets (PTL), white blood cell (WBC), albumin (Alb), alanine transaminase (ALT), glutamic transaminase (AST), hemoglobin (Hb), lactate (Lac), lymphocyte, neutrophil, international normalized ratio (INR), blood urea nitrogen (BUN), creatinine, potassium ion (K), chloride ion (Cl), sodium ion (Na), bicarbonate (HCO3-), prothrombin time (PT), mean erythrocyte volume (MCV), hematocrit (HCT), red cell distribution width (RDW)), arterial blood gas analysis (base excess (BE), total calcium (TCa), anion gap (AG)), glycosylated hemoglobin (HbA1c), fasting glucose, and general examination (heart rate (HR), mean blood pressure (MBP), temperature, respiration rate). There were thirty-eight variables at admission were selected for further analysis. The unit of the selected variable was summarized in Table S1.
Primary outcome
The primary endpoint of this study was the 28-day all-cause mortality of septic patients with DM.
Model construction and validation
Nomograms are widely used as clinical prognostic models in different fields of diseases (28). It can generate an individual probability of a clinical event by integrating diverse prognostic and determinant variables (29, 30). In this study, the patients from the MIMIC IV database were used for nomogram construction due to the larger sample size. Bootstrap analysis was used for internal validation. Moreover, patients from the MIMIC III database were further settled as the validation cohort.
Statistical analysis
The variables with a missing value (<20%) were filled out using the median interpolation method (31). Categorical variables were presented as the number of the subgroup population with the percentage (%). Continuous variables were expressed as the mean and standard deviation (Mean ± SD). LASSO regression was used to remove less important variables and reduce the potential overfitting between included variables via the regression coefficients penalizing the size of the parameters. The LASSO regression curtailed the coefficient estimates toward zero, with the degree of shrinkage dependent on an additional parameter (λ) (32). To determine the optimal values for λ, 10-time cross-validation was used, and the minimum criteria λ was selected for further analysis (32). The multivariate Cox regression analysis was conducted to determine the independent short-term prognostic factors in septic patients with DM. The comparisons of selected variables among survivor and non-survivor subpopulations were conducted by using the Pearson-Chi square test or Student’s t-text as appropriate. The LASSO and stepwise multivariate Cox regression analyses were conducted by using the “rms” package from the “R” software (http://www.r-project.org, R Foundation, Vienna, Austria, version 4.1.2). The nomogram of prognostic factors associated with 28-day mortality in septic patients with DM was based on the statistically significant different variables during the multivariate Cox regression analysis.
The C-index (33) and the area under the receiver operating characteristic (ROC) curves (AUCs) were calculated for evaluating the discrimination of the nomogram. Furthermore, the calibration curves and decision curve analysis (DCA), and Kaplan-Meier (KM) curves were performed to assess the utility of the nomogram. A two-tailed P-value < 0.05 was considered statistically significant.
Results
The clinical features of septic patients with DM
In this study, 2,227 patients in the MIMIC IV database and 1,064 in the MIMIC III database who met the inclusion criteria were enrolled. In the training data, the overall 28-day all-cause mortality rate of septic patients with DM was 23.9% (n=533) and most of the patients were white in race (61.1%) and the male subpopulation showed a relatively higher proportion (58.3%). The septic shock was identified in 57.6% of septic patients from the MIMIC IV database and 51.2% of septic patients from the MIMIC III database, respectively. Septic patients with DM were accompanied by various degrees of comorbidities at admission, with a more pronounced in renal diseases (82.4%), respiratory failure (46.9%), and heart failure (39.6%) in the training cohort. During ICU hospitalization, the majority of the septic patients with DM received ventilation (83.0%) and antibiotic (98.5%) therapeutic interventions but only 11.3% of the patients received CRRT management. In the external validation cohort from the MIMIC III database, several differences were noticed in the proportions of heart and liver comorbidities, ventilation intervention, dopa usage, total fluids administrations, and length of ICU stay, compared with the training cohort (p<0.001). In addition, the mean proportion of HbA1c was 7.27% in the training cohort and 8.38% in the validation cohort, respectively. The clinical characteristics of the entire study population are shown in Table 1.
LASSO regression analysis
A total of 38 variables were initially elected to the LASSO regression analysis with 10-fold cross-validation (Figures 2A, B; Table S1). Ultimately, ten variables including age, respiratory failure, SOFA score, Alb, BE, AG, INR, RDW, temperature, and HbA1c were selected for the stepwise multivariate Cox regression analysis (Table S2).
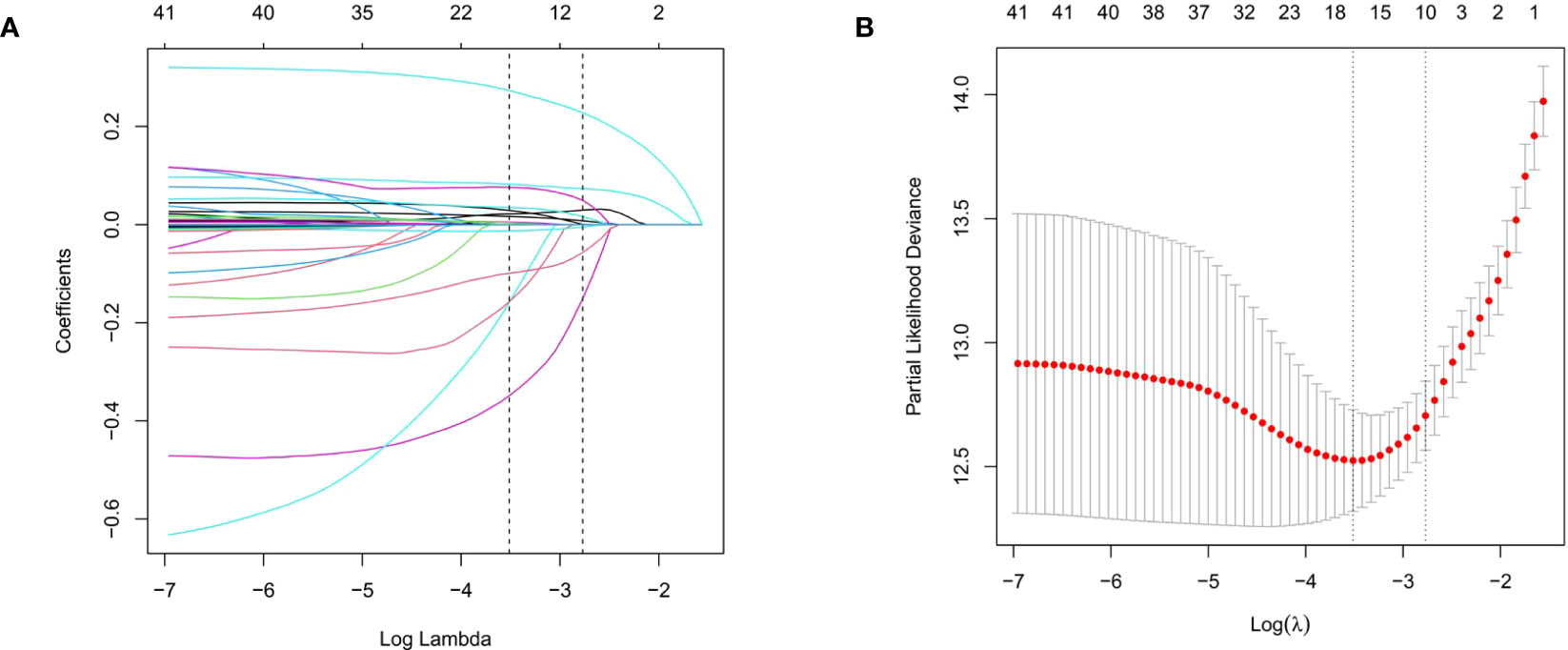
Figure 2 The LASSO regression analysis to select the potential variables. A total of thirty-eight variables are initially included and ten variables are finally selected for further analysis. (A) The LASSO coefficient analysis of the clinical features. (B) Tuning parameter selection in the LASSO Cox regression model.
The comparison of clinical variables between survivor and non-survivor
Based on the selected variables, we compared the clinical characteristics between the survivor and non-survivor subpopulations in 28-day all-cause mortality (Table 2). Regarding the age at admission, non-survivors were significantly older than the survivors (71.43 years vs. 66.89 years, p<0.001). Similarly, non-survivors showed a higher proportion of respiratory failure (691 cases (40.8%) vs. 354 (66.4%), p<0.001), SOFA score (4.08 vs. 3.13, p<0.001), RDW (16.53% vs. 15.45%, p<0.001), AG (18.44mEq/L vs. 14.17mEq/L, p<0.001) and prolonged INR (1.90s vs. 1.50s, p<0.001) as well as Hb1Ac (9.50% vs. 7.27%, p<0.001), when compared with the survivors. On the contrary, the non-survivors showed a decrease in Alb (2.67g/dL vs. 2.77g/dL, p<0.001), BE (-5.69mEq/L vs. -2.68mEq/L, p<0.001), and body temperature (36.61°C vs. 36.98°C, p<0.001) at admission, compared to the survivors. During the hospitalization, survivors showed longer hospitalization than non-survivors (length of hospital stay: 16.83 vs. 8.38 days, p<0.001; length of ICU stay: 5.57 vs. 5.21 days, p<0.001). There was a significant difference in total amounts of fluid input between survivors and non-survivors during the ICU stay (46720.13 ml vs. 59784.26 ml, p=0.004).
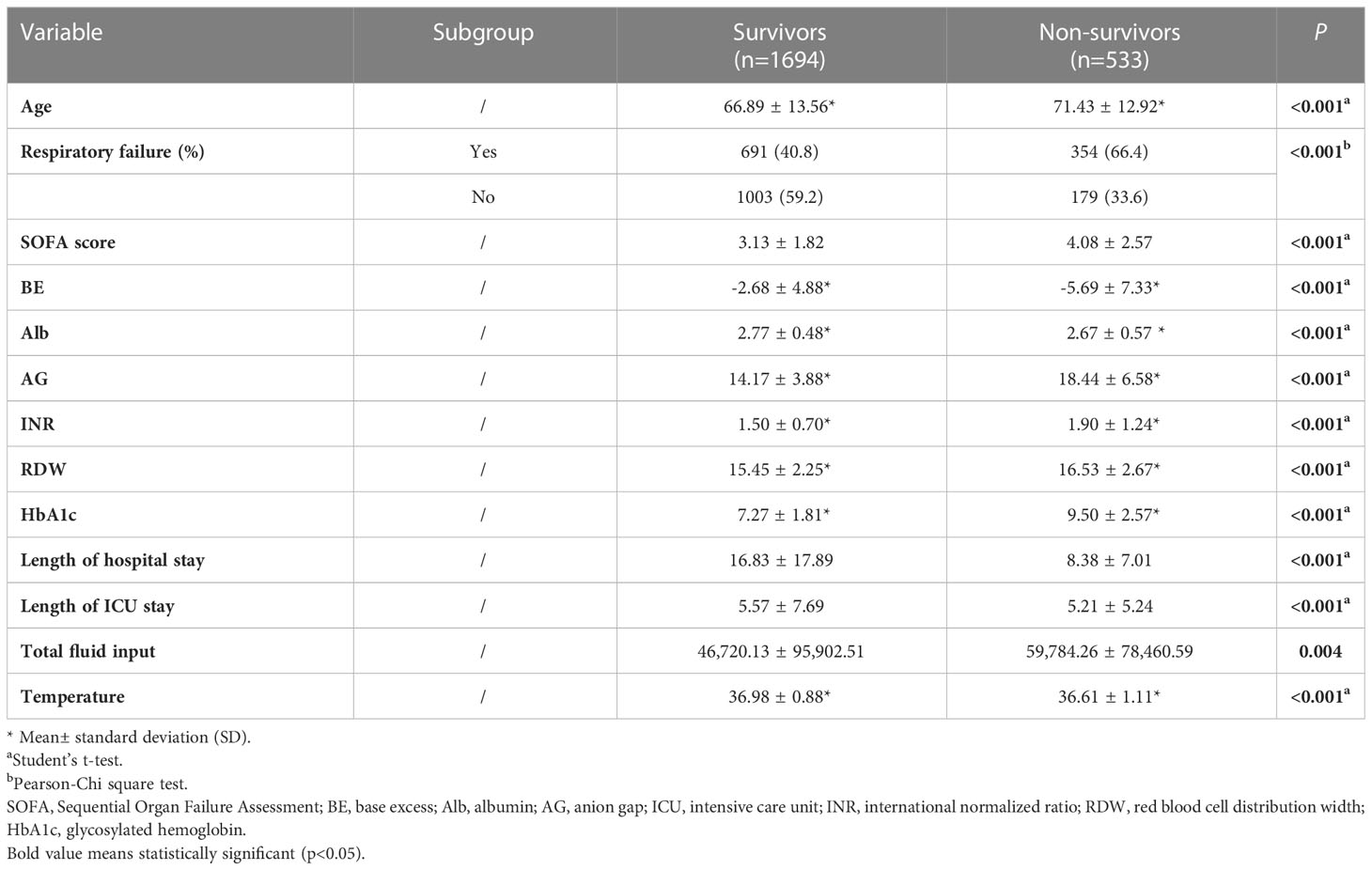
Table 2 Comparisons of clinical characteristics between survivors and non-survivors of the training cohort.
Multivariate Cox regression analyses
Multivariate Cox regression analysis revealed that age at admission (hazard ratio (HR)=1.029 per year, 95%CI: 1.022-1.036, p<0.001), respiratory failure (HR=1.872, 95%CI: 1.554-2.254, p<0.001), SOFA (HR=1.056, 95%CI: 1.018-1.094, p=0.004), levels of BE (HR=0.980, 95%CI: 0.967-0.992, p=0.002), AG (HR=1.100, 95%CI: 1.080-1.120, p<0.001), Alb (HR=0.679, 95%CI: 0.574-0.802, p<0.001), INR (HR=1.087, 95%CI: 1.027-1.150, p=0.004), RDW (HR=1.056, 95%CI: 1.021-1.092, p=0.001), and body temperature (HR=0.857, 95%CI: 0.789-0.932, p<0.001), as well as HbA1c (HR=1.358, 95%CI: 1.320-1.401, p<0.001), were the independent prognostic factors in 28-day mortality of septic patients with DM (Figure 3).
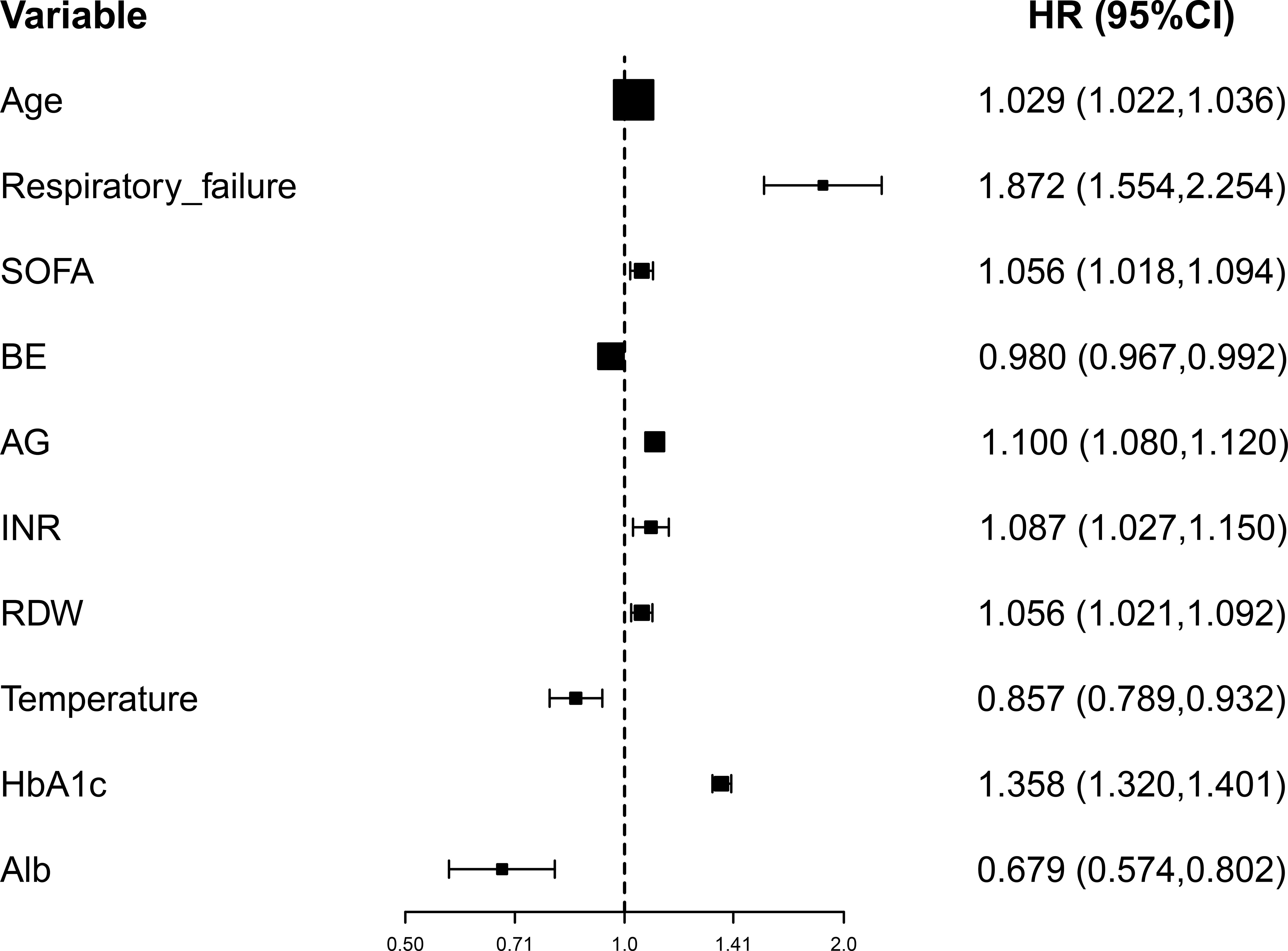
Figure 3 Forest plot for the multivariate Cox regression analysis. BE, base excess (mEq/L); SOFA, Sequential Organ Failure Assessment; AG, anion gap (mEq/L); INR, international normalized ratio (second); RDW, red blood cell distribution width (%), HbA1c, glycosylated hemoglobin (%).
Nomogram construction and validation
Depending on the prognostic factors determined by the multivariate Cox regression analysis, an individualized nomogram was subsequently established with the selected ten variables (Figure 4A). Each admitted septic patient with DM could get a total score and the corresponding risk for 28-day hospitalization mortality by adding the scores derived from the ten variables (Figure 4B).
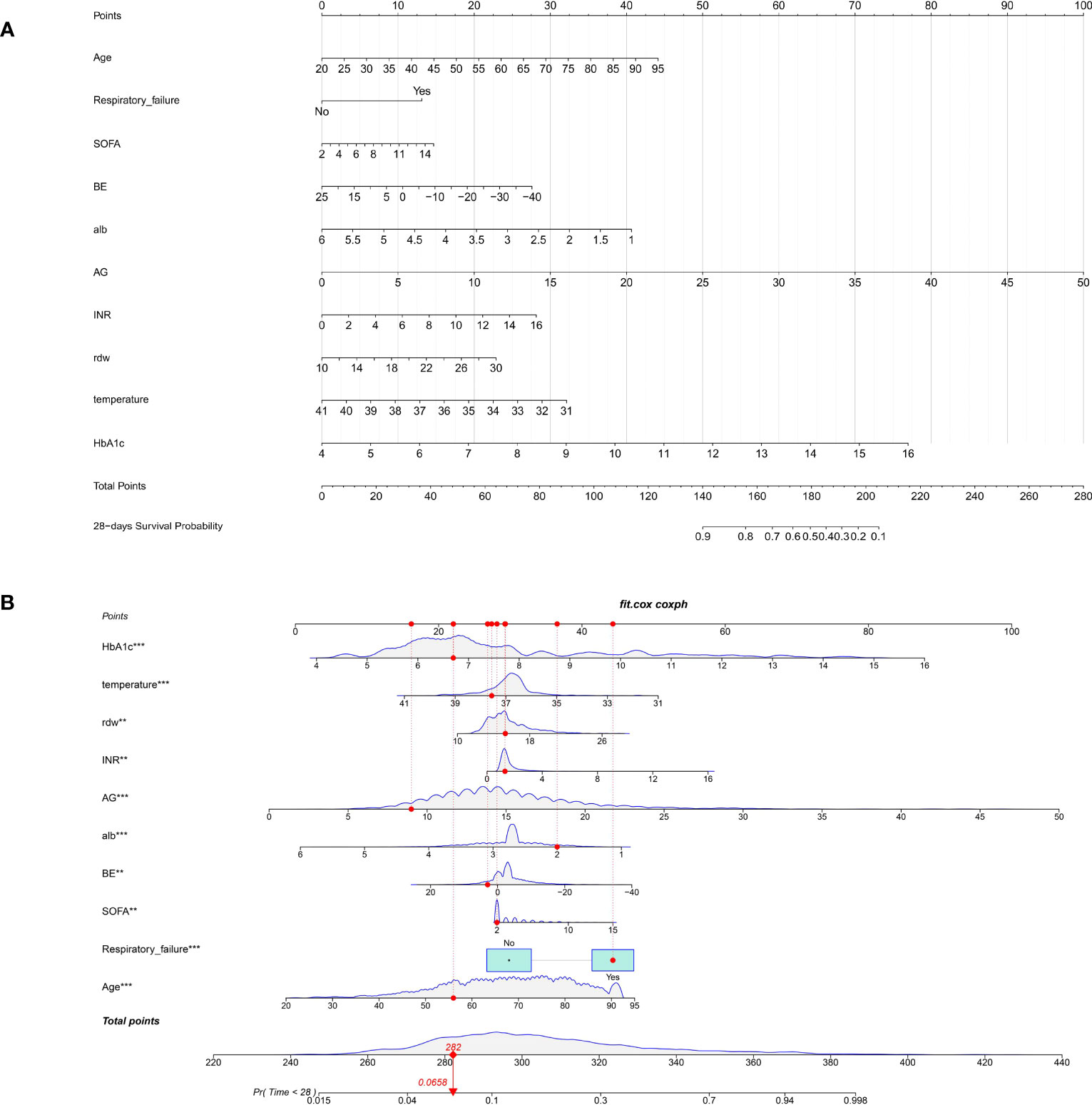
Figure 4 The nomogram for predicting the 28-day all-cause mortality in septic patients with diabetes mellitus. (A). The nomogram is constructed by ten admission clinical variables; (B). The example for explaining the clinical utility of the nomogram. The admitted patient would get an individualized score for each variable. By adding all scores, the clinicians could calculate the potential risk of 28-day all-cause mortality for each patient (red dot). BE, base excess (mEq/L); SOFA, Sequential Organ Failure Assessment; AG, anion gap (mEq/L); INR, international normalized ratio (second); RDW, red blood cell distribution width (%), HbA1c, glycosylated hemoglobin (%).
To validate the clinical utility of the nomogram, both internal bootstrap analysis and external cohort validation were performed. The C-indexes, which were consistent with the AUC of the ROCs, were all above 0.80, with 0.870 (95%CI: 0.850-0.880) in the internal bootstrap analysis and 0.830 (95%CI:0.810-0.860) in the external validation cohort, respectively (Figures 5A, B). Compared with the single SOFA score, our model was internally and externally validated to have better discrimination and accuracy in predicting the 28-day all-cause mortality of septic patients with DM (Figures 5A, B).
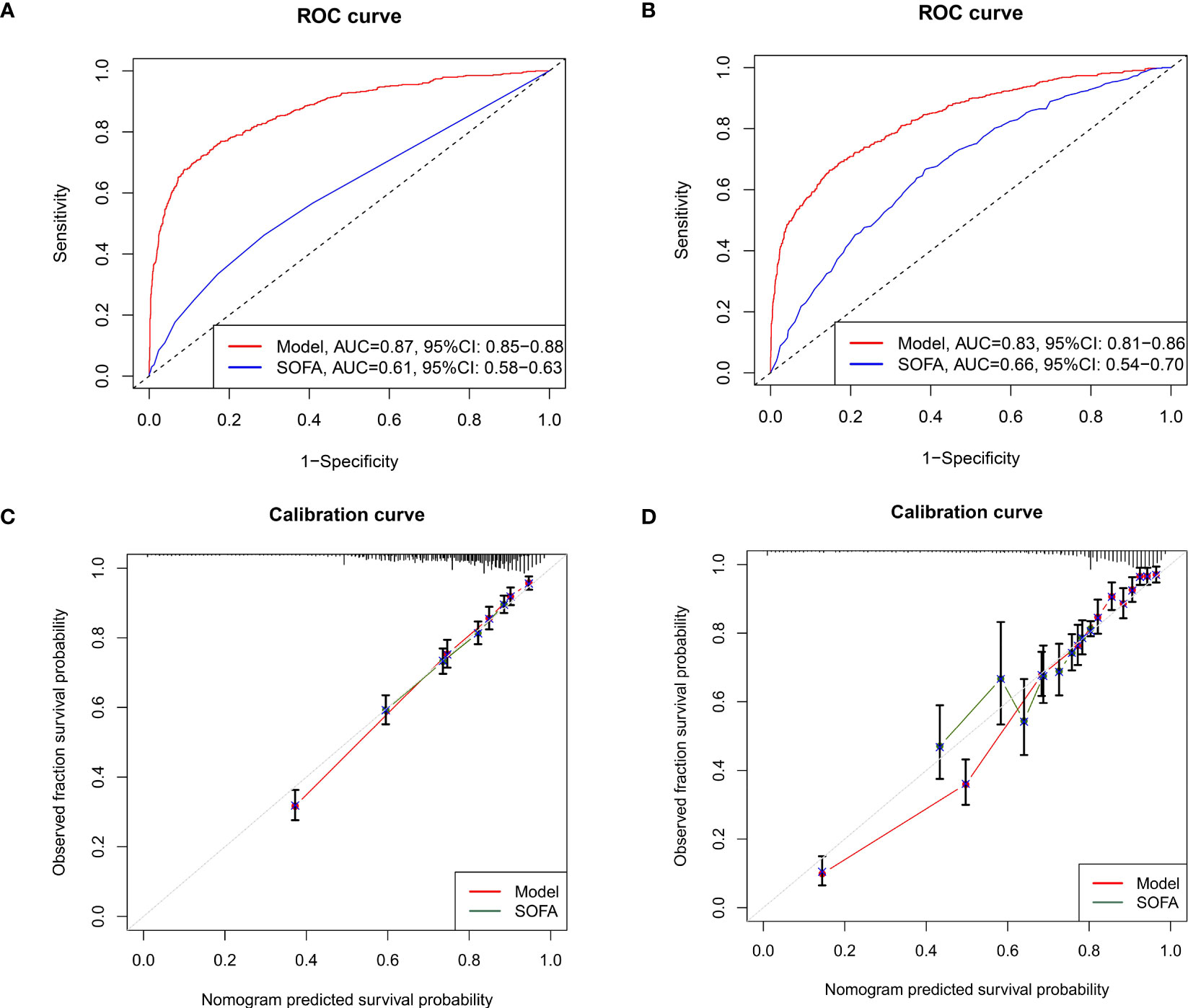
Figure 5 Methods to evaluate the accuracy and discrimination of the nomogram. (A) The ROC curves for evaluating the accuracy of the nomogram through internal bootstrapping analysis. (B) The ROC curves for evaluating the accuracy of the nomogram via external cohort analysis; (C) The calibration curves for evaluating the discrimination of the nomogram in the training cohort; (D) The calibration curves for evaluating the discrimination of the nomogram in the external validating cohort. ROC, receiver operating characteristic.
Besides, we applied the nomogram to the septic shock subpopulation to further validate the generalization of the model. The AUC of the ROC for predicting the 28-day mortality of the septic shock subpopulation reached 0.82 (95%CI: 0.790-0.850), which indicated the identified prognostic indicators were also pivotal in more severe subpopulations at admission (Figure S1).
Robustness check
To further evaluate the utility of the nomogram, calibration curves were applied. Detailly, the calibration curves showed promising agreement in the ideal and observation events in both training (Figure 5C) and validation cohorts (Figure 5D) as well as the septic shock cohorts (Figure S2).
Similarly, the DCA curves showed that the scores derived from the nomogram could be more accurate than a treat-none or treat-all strategy when the threshold probability was above 5% in the training cohort (Figure 6A) and 10% in the septic shock subpopulation (Figure S3), respectively. Meanwhile, the external validation cohort also supported an optimal clinical utility of the model at the threshold probability intervals greater than 5% (Figure 6C), which was also better than the prediction value of SOFA scores (Figures 6B, D) in the study population.
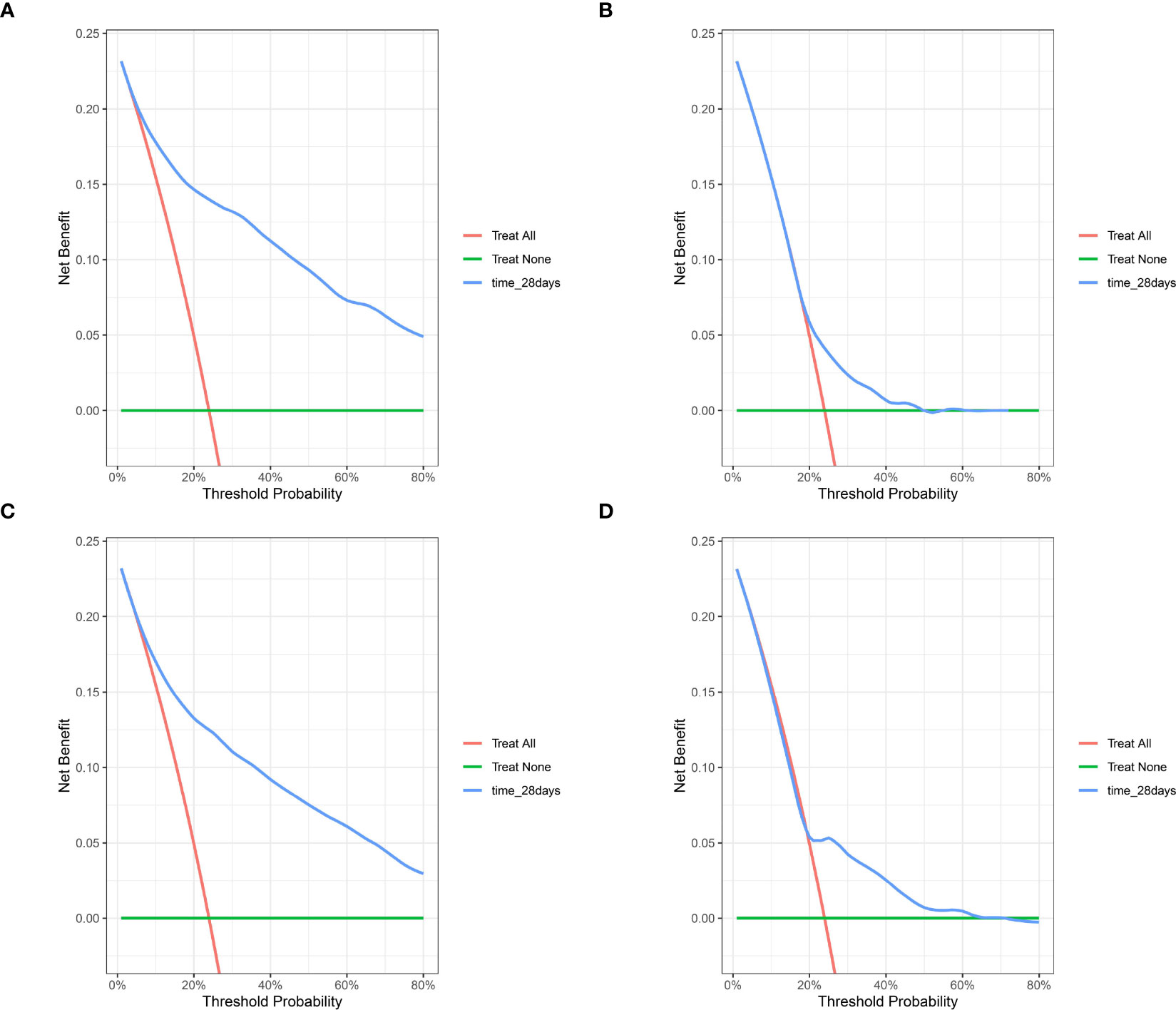
Figure 6 The DCA for evaluating the clinical utility of the nomogram. (A) the DCA of internal bootstrapping analysis; (B) The DCA of the training SOFA score; (C) The DCA of external validation cohort; (D) The DCA of the external SOFA score. DCA, decision curve analysis; SOFA, Sequential Organ Failure Assessment.
Based on the risk model, the KM curves showed good discrimination in identifying the real high-risk subpopulation (cutoff value: 186) in both the internal (Figures 7A, B) and external validating cohorts (Figures 7C, D).
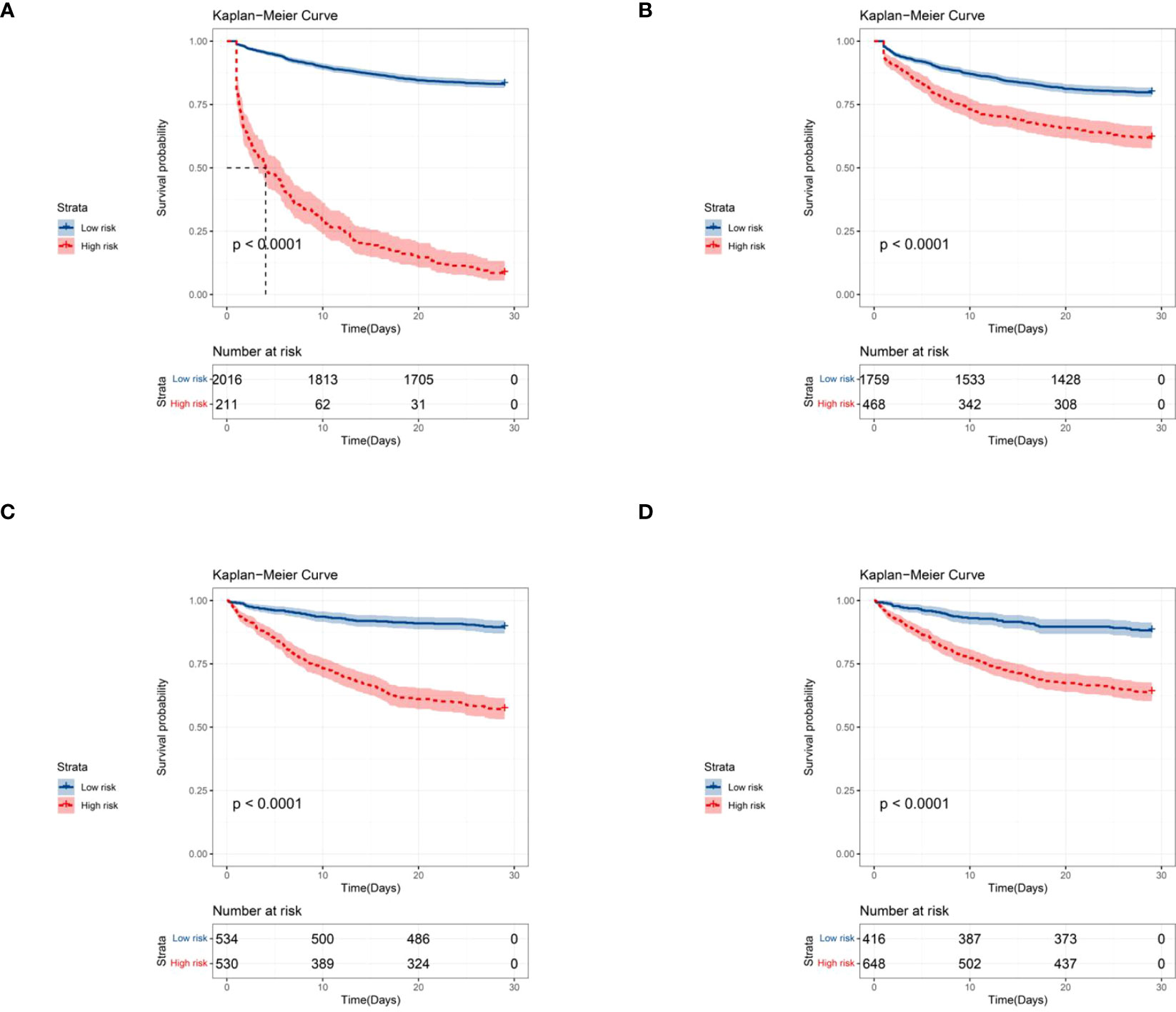
Figure 7 Kaplan-Meier curves for predicting the 28-day all-cause mortality in septic patients with DM by stratifying the different risk subgroups (the cut-off point was 186). (A) Kaplan-Meier curves based on the nomogram; (B) Kaplan-Meier curves based on the training SOFA score; (C) Kaplan-Meier curves based on the external validation cohort; (D) Kaplan-Meier curves based on the external validation SOFA score.
Discussion
Currently, prognostic factors for septic patients with DM remain limited. In this study, we fill this research gap and identify that various admission characteristics were significantly associated with the short-term mortality risk in septic patients with DM. Additionally, we developed an individualized prediction nomogram for the clinical management of septic patients with DM at the initial round evaluation. Robustness analyses showed good discrimination, calibration ability, and clinical usefulness of this predictive model.
Sepsis is still the leading cause of death in patients elected to the ICU (15, 18). In the present study, the 28-day mortality rate of septic patients with DM was 23.9% (n=533), which was higher than in general septic patients (10%-15%) (34–36). Regarding the hospitalization characteristics of the study population, we observed that survivors showed longer hospital and ICU stays than non-survivors, which was consistent with previous studies (24, 37). Besides, our study also determined that non-survivors received a higher volume of fluids input during the ICU stay than survivors. Interestingly, the optimal amount of fluid administration for septic and septic shock patients is still uncertain (24, 38–40). Notably, one meta-analysis included 13 trials that suggested that lower standard intravenous (IV) fluid volumes showed no significant difference in all-cause mortality compared with higher IV fluid volumes (41). Furthermore, the recent Conservative versus Liberal Approach to Fluid Therapy in Septic Shock in Intensive Care (CLASSIC) study also revealed that adult patients with septic shock might not get more survival or health-related quality of life benefits from restrictive fluid therapy when compared with IV fluid therapy (38). Thus, clinicians should trade off the risks and benefits of fluid administration in each stage of critical illness. Further works are needed to help clinicians to make tailored fluid management.
Among septic patients with DM, recent basic and clinical studies revealed that systemic immune dysfunctions including, but not limited to, deficiencies in neutrophil function and declining T-cell responses triggered by the hyperglycemia condition were associated with the infection development and sepsis mortality (10, 42). As one of the representative indicators in DM, HbA1c was mainly interpreted as a biomarker to reflect the short and intermediate terms of glycemic control. Of note, recent studies demonstrated the clinical predictive value of HbA1c in the development and prognosis of critically ill diseases, particularly sepsis. However, the findings were not always consistent. Notably, Anca et al. determined the nonlinear relationship between HbA1c and the risk of sepsis (43). However, HbA1c was not identified significantly associated with mortality in septic patients (43). On the contrary, the study by Guo et al. showed that the levels of HbA1c at admission were remarkably associated with morbidity and mortality in septic patients (44). In our analysis, high proportions of HbA1c were strongly associated with an increased risk of short-term mortality in septic patients with DM. Preclinical studies showed that chronic dysglycemia could impair the vascular endothelial glycocalyx and further cause complications and the mortality of sepsis (45). Especially, the lung was one of the most vulnerable organs in DM and septic patients. In our study, we observed that patients with respiratory failure showed a nearly two-fold risk (HR=1.872) of mortality, compared with patients without respiratory failure. Notably, several previous studies suggested that DM was associated with an increased risk of respiratory failure. For example, Gulcan et al. reported that half of the patients with DM exhibited respiratory failure during sleep, which might be due to restrictive impairment of respiratory function secondary to DM (46). Besides, the preclinical data also demonstrated that hyperglycemia could activate sodium-potassium-chloride cotransporter 1(NKCC1) related pathways and adversely affect alveolar fluid regulation and lung function (47). Therefore, septic patients with DM are expected to receive more active respiratory function surveillance during the ICU stay.
Compared with recent studies on general sepsis, we validated several clinical indicators in the subpopulation of patients with DM. Initially, we identified that age was a strong prognostic indicator of short-term mortality in septic patients with DM. There is a significant increase in the prevalence of DM in the elderly population (48–50), leading to exacerbation, poor prognosis, and increased mortality risk in sepsis (51). Additionally, serum biomarkers were important predictors in the surveillance of critically ill patients (34, 36, 52–55). Septic patients were often accompanied by hypoproteinemia due to capillary leakage, reperfusion injury, tissue ischemia, and inflammation (56, 57). More importantly, septic patients with hypoproteinemia showed worse clinical outcomes during hospitalization. Similarly, hypoproteinemia was also associated with multiple adverse complications and increased risk of adverse outcomes in DM (58). Therefore, septic patients with DM may suffer from a higher risk of mortality in the condition of hypoproteinemia. Furthermore, acid-base disorders are frequently observed in critically ill patients (59), especially in patients with DM (60). Of note, serum lac level was a tight biomarker for predicting the clinical outcome of patients with sepsis (61, 62). Interestingly, hyperlactatemia was a frequent metabolic condition in DM patients with ketoacidosis (63, 64). However, compared with other critically ill diseases, the elevated lac level was not determined to be associated with increased length of ICU stay or mortality in DM patients (65). Furthermore, some researchers even queried whether the standard hyperlactatemia cut-off value was also adapted for the survival prediction in DM patients (66). For these reasons, the value of lactate levels might be undermined in predicting short-term mortality in septic patients with DM, particularly when they were concurrent with diabetic ketoacidosis.
As a common biomarker for evaluating the acid-base balance, the AG levels were frequently used to evaluate the type of metabolic acidosis. However, acid-base imbalance, especially metabolic acidosis, frequently occurred in seriously ill patients and was significantly related to mortality. In our analysis, the levels of BE and AG were determined to be significantly associated with short-term mortality among septic patients with DM. Recent two studies from different regions also highlighted the predictive role of BE in the diagnosis and prognosis of sepsis (67, 68). Moreover, AG was recently identified to be an optimal serum biomarker for reflecting systemic dysfunctions in critically ill patients (69–71). In particular, high levels of AG were not only associated with impaired cardiorespiratory fitness (72) but also mediated more severe insulin resistance conditions (73). This could partially explain the underlying mechanism that AG played an important role in the prognosis of septic patients with DM. Currently, whether AG can accurately predict the prognosis of critically ill patients is still debatable. These discrepancies may be due to differences in varied study populations and measuring methods as well as other factors that influence AG values may also influence the exact correlations. Further studies are warranted to explore the underlying pathophysiological mechanism.
Consistent with the study by Liu et al. (74), we observed that prolonged INR was positively associated with short-term mortality in septic patients with DM. Some possible mechanisms might explain this finding. It is noteworthy that the activated coagulation system was regarded as the primary response of host systemic defense in sepsis (75). However, chronic septic conditions could adversely mediate the coagulation dysfunction with subsequent disseminated intravascular coagulation (DIC) (76). Besides, we also determined that a high percentage of RDW was an adverse prognostic factor for septic patients with DM. Most recently, the latest evidence showed that RDW could predict poor outcomes in various chronic diseases (77–81). In the DM population, Nada found that RDW was markedly higher in DM patients than in healthy subjects and particularly higher in uncontrolled glycemia (82). And in the population with sepsis, a meta-analysis of three studies revealed that a high level of RDW was associated with septic death (83). Thus, the percentage of RDW, affected by the hyperglycemia condition, showed a unique clinical value in critically ill patients, which could be a supplement indicator for assessing the prognosis of septic patients with DM.
Interestingly, the admitting body temperature was observed to be associated with the severity and prognosis of septic patients with DM. The latest two large-scale multi-center studies yielded the distinguished role of body temperature in the prognosis of critically ill patients, especially in terms of septic patients (84, 85). Similarly, our study supported that the patients with relatively higher body temperatures presented a lower risk for short-term mortality.
Based on identified prognostic factors, we established a new individualized nomogram to evaluate the condition of septic patients with DM at admission. The optimal C-index and AUCs in both the internal and external validation cohorts suggested the good discrimination and accuracy of the model in detecting high-risk patients. Furthermore, compared with the separate SOFA evaluation system, our model showed better predictive accuracy combined with various clinical characteristics. Additionally, we explored the utility of the nomogram in septic shock patients with DM, the subpopulation which was much more related to mortality. As expected, the model also presented promising predictive ability in detecting high-risk septic shock patients. Therefore, by using the predictive model, clinicians could better stratify risk for septic patients with DM at the initial evaluation.
Nevertheless, our study has some limitations. First, some factors, including laboratory indicators (such as fast plasma glucose, fasting insulin, fasting C-peptide, and C-reactive protein), site of infection, drugs, and interventions that could be related to prognosis, were not included in this study. Second, although this is a large-scale cohort study with over 3000 patients involved, the nature of the retrospective study design could inevitably lead to selection bias. Third, the MIMIC database was constructed from the medical records of admitted patients during the past two decades. However, intensive care medicine has developed substantially during the same period. Whether our findings would also be adapted to the current clinical practice needs further validation. Last, although we have successfully developed and validated a short-term mortality risk stratification model, data from other countries or regions are needed to verify this model in the future with more useful variables added.
Conclusions
In this study, ten clinical variables including age, respiratory failure, SOFA, BE, Alb, AG, INR, RDW, HbA1c, and temperature at admission were identified as independent prognostic factors in predicting the 28-day all-cause mortality in septic patients with DM. Based on these factors, we developed and externally validated a predictive nomogram, with optimal discrimination and accuracy to detect the high-risk subgroup. This model can be implemented for ICU physicians to quickly make the initial clinical decision for septic patients with DM in clinical practice.
Data availability statement
The original contributions presented in the study are included in the article/Supplementary Material. Further inquiries can be directed to the corresponding author.
Author contributions
(I) Conception and design: XH and CY. (II) Administrative support: XH. (III) Provision of study materials or patients: CY, YJ, and YM. (IV) Collection and assembly of data: CY, YJ, and YM. (V) Data analysis and interpretation: XH, CY, and CZ. (VI) Manuscript writing: All authors. (VII) Final approval of manuscript: All authors.
Acknowledgments
We acknowledged the contributions of the Medical Information Mart for Intensive Care (MIMIC) Program registries for creating and updating the MIMIC-III and MIMIC-IV databases.
Conflict of interest
The authors declare that the research was conducted in the absence of any commercial or financial relationships that could be construed as a potential conflict of interest.
Publisher’s note
All claims expressed in this article are solely those of the authors and do not necessarily represent those of their affiliated organizations, or those of the publisher, the editors and the reviewers. Any product that may be evaluated in this article, or claim that may be made by its manufacturer, is not guaranteed or endorsed by the publisher.
Supplementary material
The Supplementary Material for this article can be found online at: https://www.frontiersin.org/articles/10.3389/fendo.2023.1237866/full#supplementary-material
References
1. Cecconi M, Evans L, Levy M, Rhodes A. Sepsis and septic shock. Lancet (2018) 392(10141):75–87. doi: 10.1016/S0140-6736(18)30696-2
2. van der Poll T, van de Veerdonk FL, Scicluna BP, Netea MG. The immunopathology of sepsis and potential therapeutic targets. Nat Rev Immunol (2017) 17(7):407–20. doi: 10.1038/nri.2017.36
3. Singer M, Deutschman CS, Seymour CW, Shankar-Hari M, Annane D, Bauer M, et al. The third international consensus definitions for sepsis and septic shock (Sepsis-3). Jama (2016) 315(8):801–10. doi: 10.1001/jama.2016.0287
4. Liu D, Huang SY, Sun JH, Zhang HC, Cai QL, Gao C, et al. Sepsis-induced immunosuppression: mechanisms, diagnosis and current treatment options. Military Med Res (2022) 9(1):56. doi: 10.1186/s40779-022-00422-y
5. Prescott HC, Angus DC. Enhancing recovery from sepsis: A review. Jama (2018) 319(1):62–75. doi: 10.1001/jama.2017.17687
6. Gotts JE, Matthay MA. Sepsis: pathophysiology and clinical management. BMJ (2016) 353:i1585. doi: 10.1136/bmj.i1585
7. Trevelin SC, Carlos D, Beretta M, da Silva JS, Cunha FQ. Diabetes mellitus and sepsis: A challenging association. Shock (2017) 47(3):276–87. doi: 10.1097/SHK.0000000000000778
8. Liu Y, Sun R, Jiang H, Liang G, Huang Z, Qi L, et al. Development and validation of a predictive model for in-hospital mortality in patients with sepsis-associated liver injury. Ann Transl Med (2022) 10(18):997. doi: 10.21037/atm-22-4319
9. Tang JL, Xin M, Zhang LC. Protective effect of Astragalus membranaceus and Astragaloside IV in sepsis-induced acute kidney injury. Aging (2022) 14(14):5855–77. doi: 10.18632/aging.204189
10. Frydrych LM, Bian G, O’Lone DE, Ward PA, Delano MJ. Obesity and type 2 diabetes mellitus drive immune dysfunction, infection development, and sepsis mortality. J leukocyte Biol (2018) 104(3):525–34. doi: 10.1002/JLB.5VMR0118-021RR
11. Zheng Y, Ley SH, Hu FB. Global aetiology and epidemiology of type 2 diabetes mellitus and its complications. Nat Rev Endocrinol (2018) 14(2):88–98. doi: 10.1038/nrendo.2017.151
12. Worldwide trends in diabetes since 1980: a pooled analysis of 751 population-based studies with 4.4 million participants. Lancet (2016) 387(10027):1513–30. doi: 10.1016/s0140-6736(16)00618-8
13. Frydrych LM, Fattahi F, He K, Ward PA, Delano MJ. Diabetes and sepsis: risk, recurrence, and ruination. Front Endocrinol (2017) 8:271. doi: 10.3389/fendo.2017.00271
14. Muller LM, Gorter KJ, Hak E, Goudzwaard WL, Schellevis FG, Hoepelman AI, et al. Increased risk of common infections in patients with type 1 and type 2 diabetes mellitus. Clin Infect diseases: an Off Publ Infect Dis Soc America (2005) 41(3):281–8. doi: 10.1086/431587
15. Schuetz P, Castro P, Shapiro NI. Diabetes and sepsis: preclinical findings and clinical relevance. Diabetes Care (2011) 34(3):771–8. doi: 10.2337/dc10-1185
16. Laupland KB, Gregson DB, Zygun DA, Doig CJ, Mortis G, Church DL. Severe bloodstream infections: a population-based assessment. Crit Care Med (2004) 32(4):992–7. doi: 10.1097/01.CCM.0000119424.31648.1E
17. Costantini E, Carlin M, Porta M, Brizzi MF. Type 2 diabetes mellitus and sepsis: state of the art, certainties and missing evidence. Acta Diabetol (2021) 58(9):1139–51. doi: 10.1007/s00592-021-01728-4
18. Hotchkiss RS, Monneret G, Payen D. Sepsis-induced immunosuppression: from cellular dysfunctions to immunotherapy. Nat Rev Immunol (2013) 13(12):862–74. doi: 10.1038/nri3552
19. Liang H, Ding X, Li L, Wang T, Kan Q, Wang L, et al. Association of preadmission metformin use and mortality in patients with sepsis and diabetes mellitus: a systematic review and meta-analysis of cohort studies. Crit Care (2019) 23(1):50. doi: 10.1186/s13054-019-2346-4
20. Stegenga ME, Vincent JL, Vail GM, Xie J, Haney DJ, Williams MD, et al. Diabetes does not alter mortality or hemostatic and inflammatory responses in patients with severe sepsis. Crit Care Med (2010) 38(2):539–45. doi: 10.1097/CCM.0b013e3181c02726
21. Esper AM, Moss M, Martin GS. The effect of diabetes mellitus on organ dysfunction with sepsis: an epidemiological study. Crit Care (2009) 13(1):R18. doi: 10.1186/cc7717
22. Johnson AEW, Bulgarelli L, Shen L, Gayles A, Shammout A, Horng S, et al. MIMIC-IV, a freely accessible electronic health record dataset. Sci Data (2023) 10(1):1. doi: 10.1038/s41597-023-01945-2
23. Wei X, Min Y, Yu J, Wang Q, Wang H, Li S, et al. The value of admission serological indicators for predicting 28-day mortality in intensive care patients with acute heart failure: construction and validation of a nomogram. Front Cardiovasc Med (2021) 8:741351. doi: 10.3389/fcvm.2021.741351
24. Zhou S, Zeng Z, Wei H, Sha T, An S. Early combination of albumin with crystalloids administration might be beneficial for the survival of septic patients: a retrospective analysis from MIMIC-IV database. Ann Intensive Care (2021) 11(1):42. doi: 10.1186/s13613-021-00830-8
25. Shu T, Huang J, Deng J, Chen H, Zhang Y, Duan M, et al. Development and assessment of scoring model for ICU stay and mortality prediction after emergency admissions in ischemic heart disease: a retrospective study of MIMIC-IV databases. Intern Emerg Med (2023) 18(2):487–97. doi: 10.1007/s11739-023-03199-7
26. Peng S, Huang J, Liu X, Deng J, Sun C, Tang J, et al. Interpretable machine learning for 28-day all-cause in-hospital mortality prediction in critically ill patients with heart failure combined with hypertension: A retrospective cohort study based on medical information mart for intensive care database-IV and eICU databases. Front Cardiovasc Med (2022) 9:994359. doi: 10.3389/fcvm.2022.994359
27. Benchimol EI, Smeeth L, Guttmann A, Harron K, Moher D, Petersen I, et al. The REporting of studies Conducted using Observational Routinely-collected health Data (RECORD) statement. PloS Med (2015) 12(10):e1001885. doi: 10.1371/journal.pmed.1001885
28. Iasonos A, Schrag D, Raj GV, Panageas KS. How to build and interpret a nomogram for cancer prognosis. J Clin Oncol (2008) 26(8):1364–70. doi: 10.1200/JCO.2007.12.9791
29. Long J, Wang M, Li W, Cheng J, Yuan M, Zhong M, et al. The risk assessment tool for intensive care unit readmission: A systematic review and meta-analysis. Intensive Crit Care Nurs (2023) 76:103378. doi: 10.1016/j.iccn.2022.103378
30. Balachandran VP, Gonen M, Smith JJ, DeMatteo RP. Nomograms in oncology: more than meets the eye. Lancet Oncol (2015) 16(4):e173–80. doi: 10.1016/S1470-2045(14)71116-7
31. Berkelmans GFN, Read SH, Gudbjörnsdottir S, Wild SH, Franzen S, van der Graaf Y, et al. Population median imputation was noninferior to complex approaches for imputing missing values in cardiovascular prediction models in clinical practice. J Clin Epidemiol (2022) 145:70–80. doi: 10.1016/j.jclinepi.2022.01.011
32. Tibshirani R. The lasso method for variable selection in the Cox model. Stat Med (1997) 16(4):385–95. doi: 10.1002/(SICI)1097-0258(19970228)16:4<385::AID-SIM380>3.0.CO;2-3
33. Harrell FE Jr., Lee KL, Mark DB. Multivariable prognostic models: issues in developing models, evaluating assumptions and adequacy, and measuring and reducing errors. Stat Med (1996) 15(4):361–87. doi: 10.1002/(SICI)1097-0258(19960229)15:4<361::AID-SIM168>3.0.CO;2-4
34. Jiang L, Wang Z, Wang L, Liu Y, Chen D, Zhang D, et al. Predictive value of the serum anion gap for 28-day in-hospital all-cause mortality in sepsis patients with acute kidney injury: a retrospective analysis of the MIMIC-IV database. Ann Trans Med (2022) 10(24):1373. doi: 10.21037/atm-22-5916
35. Xu W, Huo J, Cheng G, Fu J, Huang X, Feng J, et al. Association between different concentrations of human serum albumin and 28-day mortality in intensive care patients with sepsis: A propensity score matching analysis. Front Pharmacol (2022) 13:1037893. doi: 10.3389/fphar.2022.1037893
36. Qi J, Xie Q, Li Z, Sun C. A nomogram to better predict the in-hospital mortality of trauma patients with sepsis in the intensive care unit. Int J Clin Pract (2022) 2022:4134138. doi: 10.1155/2022/4134138
37. Firzli TR, Sathappan S, Antwi-Amoabeng D, Beutler BD, Ulanja MB, Madhani-Lovely F. Association between histamine 2 receptor antagonists and sepsis outcomes in ICU patients: a retrospective analysis using the MIMI-IV database. J Anesth Analg Crit Care (2023) 3(1):3. doi: 10.1186/s44158-023-00089-4
38. Kjær MN, Meyhoff TS, Sivapalan P, Granholm A, Hjortrup PB, Madsen MB, et al. Long-term effects of restriction of intravenous fluid in adult ICU patients with septic shock. Intensive Care Med (2023) 49(7):820–30. doi: 10.1007/s00134-023-07114-8
39. Zampieri FG, Bagshaw SM, Semler MW. Fluid therapy for critically ill adults with sepsis: A review. JAMA (2023) 329(22):1967–80. doi: 10.1001/jama.2023.7560
40. Ma Z, Krishnamurthy M, Modi V, Allen D, Shirani J. Impact of cardiac troponin release and fluid resuscitation on outcomes of patients with sepsis. Int J Cardiol (2023) 2023:131144. doi: 10.1016/j.ijcard.2023.131144
41. Sivapalan P, Ellekjaer KL, Jessen MK, Meyhoff TS, Cronhjort M, Hjortrup PB, et al. Lower vs higher fluid volumes in adult patients with sepsis: an updated systematic review with meta-analysis and trial sequential analysis. Chest (2023) S0012-3692(23)00637-2. doi: 10.1016/j.chest.2023.04.036
42. Geerlings SE, Hoepelman AI. Immune dysfunction in patients with diabetes mellitus (DM). FEMS Immunol Med Microbiol (1999) 26(3-4):259–65. doi: 10.1111/j.1574-695X.1999.tb01397.x
43. Balintescu A, Lind M, Andersson Franko M, Oldner A, Cronhjort M, Eliasson B, et al. Glycaemic control and sepsis risk in adults with type 1 diabetes. Diabetes Obes Metab (2023) 25(7):1942–9. doi: 10.1111/dom.15060
44. Guo F, Shen H. Glycosylated hemoglobin as a predictor of sepsis and all-cause mortality in trauma patients. Infection Drug resistance (2021) 14:2517–26. doi: 10.2147/IDR.S307868
45. Iba T, Levy JH. Derangement of the endothelial glycocalyx in sepsis. J Thromb haemostasis: JTH (2019) 17(2):283–94. doi: 10.1111/jth.14371
46. Colbay G, Cetin M, Colbay M, Berker D, Guler S. Type 2 diabetes affects sleep quality by disrupting the respiratory function. J Diabetes (2015) 7(5):664–71. doi: 10.1111/1753-0407.12225
47. Wu CP, Huang KL, Peng CK, Lan CC. Acute hyperglycemia aggravates lung injury via activation of the SGK1-NKCC1 pathway. Int J Mol Sci (2020) 21(13):4803. doi: 10.3390/ijms21134803
48. Sanz-Cánovas J, López-Sampalo A, Cobos-Palacios L, Ricci M, Hernández-Negrín H, Mancebo-Sevilla JJ, et al. Management of type 2 diabetes mellitus in elderly patients with frailty and/or sarcopenia. Int J Environ Res Public Health (2022) 19(14):8677. doi: 10.3390/ijerph19148677
49. Migdal A, Yarandi SS, Smiley D, Umpierrez GE. Update on diabetes in the elderly and in nursing home residents. J Am Med Directors Assoc (2011) 12(9):627–32.e2. doi: 10.1016/j.jamda.2011.02.010
50. Bansal N, Dhaliwal R, Weinstock RS. Management of diabetes in the elderly. Med Clinics North America (2015) 99(2):351–77. doi: 10.1016/j.mcna.2014.11.008
51. Kingren MS, Starr ME, Saito H. Divergent sepsis pathophysiology in older adults. Antioxidants Redox Signaling (2021) 35(16):1358–75. doi: 10.1089/ars.2021.0056
52. Luo M, Chen Y, Cheng Y, Li N, Qing H. Association between hematocrit and the 30-day mortality of patients with sepsis: A retrospective analysis based on the large-scale clinical database MIMIC-IV. PloS One (2022) 17(3):e0265758. doi: 10.1371/journal.pone.0265758
53. Zhang L, Huang T, Xu F, Li S, Zheng S, Lyu J, et al. Prediction of prognosis in elderly patients with sepsis based on machine learning (random survival forest). BMC Emergency Med (2022) 22(1):26. doi: 10.1186/s12873-022-00582-z
54. Hu J, Lv C, Hu X, Liu J. Effect of hypoproteinemia on the mortality of sepsis patients in the ICU: a retrospective cohort study. Sci Rep (2021) 11(1):24379. doi: 10.1038/s41598-021-03865-w
55. Han D, Zhang L, Zheng S, Xu F, Li C, Yang R, et al. Prognostic value of blood urea nitrogen/creatinine ratio for septic shock: an analysis of the MIMIC-III clinical database. BioMed Res Int (2021) 2021:5595042. doi: 10.1155/2021/5595042
56. Gong Y, Li D, Cheng B, Ying B, Wang B. Increased neutrophil percentage-to-albumin ratio is associated with all-cause mortality in patients with severe sepsis or septic shock. Epidemiol infection (2020) 148:e87. doi: 10.1017/S0950268820000771
57. Etzrodt V, Idowu TO, Schenk H, Seeliger B, Prasse A, Thamm K, et al. Role of endothelial microRNA 155 on capillary leakage in systemic inflammation. Crit Care (2021) 25(1):76. doi: 10.1186/s13054-021-03500-0
58. Yanagita I, Fujihara Y, Iwaya C, Kitajima Y, Tajima M, Honda M, et al. Low serum albumin, aspartate aminotransferase, and body mass are risk factors for frailty in elderly people with diabetes-a cross-sectional study. BMC geriatrics (2020) 20(1):200. doi: 10.1186/s12877-020-01601-z
59. Kellum JA. Fluid resuscitation and hyperchloremic acidosis in experimental sepsis: improved short-term survival and acid-base balance with Hextend compared with saline. Crit Care Med (2002) 30(2):300–5. doi: 10.1097/00003246-200202000-00006
60. Achanti A, Szerlip HM. Acid-base disorders in the critically ill patient. Clin J Am Soc Nephrology: CJASN (2023) 18(1):102–12. doi: 10.2215/CJN.04500422
61. Bakker J, Postelnicu R, Mukherjee V. Lactate: where are we now? Crit Care Clin (2020) 36(1):115–24. doi: 10.1016/j.ccc.2019.08.009
62. Liu Z, Meng Z, Li Y, Zhao J, Wu S, Gou S, et al. Prognostic accuracy of the serum lactate level, the SOFA score and the qSOFA score for mortality among adults with Sepsis. Scand J Trauma Resusc Emerg Med (2019) 27(1):51. doi: 10.1186/s13049-019-0609-3
63. Morgan TJ, Scott PH, Anstey CM, Bowling FG. Hyperlactatemia in diabetic ketoacidosis is common and can be prolonged: lactate time-series from 25 intensive care admissions. J Clin Monit Comput (2021) 35(4):757–64. doi: 10.1007/s10877-020-00532-9
64. Masharani U, Strycker LA, Lazar AA, Wu K, Brooks GA. Hyperlactatemia in diabetic ketoacidosis. Diabetes Med (2022) 39(4):e14723. doi: 10.1111/dme.14723
65. Cox K, Cocchi MN, Salciccioli JD, Carney E, Howell M, Donnino MW. Prevalence and significance of lactic acidosis in diabetic ketoacidosis. J Crit Care (2012) 27(2):132–7. doi: 10.1016/j.jcrc.2011.07.071
66. Vieira IH, Petrova M, Moura JP. Does the same hyperlactatemia cut-off in the context of acute diseases hold the same meaning in diabetes mellitus? Cureus (2022) 14(5):e25163. doi: 10.7759/cureus.25163
67. Arayici S, Şimşek GK, Canpolat FE, Oncel MY, Uras N, Oguz SS. Can base excess be used for prediction to early diagnosis of neonatal sepsis in preterm newborns? Mediterr J Hematol Infect Dis (2019) 11(1):e2019014. doi: 10.4084/mjhid.2019.014
68. Seo MH, Choa M, You JS, Lee HS, Hong JH, Park YS, et al. Hypoalbuminemia, low base excess values, and tachypnea predict 28-day mortality in severe sepsis and septic shock patients in the emergency department. Yonsei Med J (2016) 57(6):1361–9. doi: 10.3349/ymj.2016.57.6.1361
69. Gong F, Zhou Q, Gui C, Huang S, Qin Z. The relationship between the serum anion gap and all-cause mortality in acute pancreatitis: an analysis of the MIMIC-III database. Int J Gen Med (2021) 14:531–8. doi: 10.2147/IJGM.S293340
70. Jhou HJ, Chen PH, Yang LY, Chang SH, Lee CH. Plasma anion gap and risk of in-hospital mortality in patients with acute ischemic stroke: analysis from the MIMIC-IV database. J personalized Med (2021) 11(10):1004. doi: 10.3390/jpm11101004
71. Wang H, Liu C, Xu H, Zhang Y, Gao P, Geng S, et al. The Association between Serum Anion Gap and All-Cause Mortality in Cerebral Infarction Patients after Treatment with rtPA: A Retrospective Analysis. Dis Markers (2022) 2022:1931818. doi: 10.1155/2022/1931818
72. Abramowitz MK, Hostetter TH, Melamed ML. Lower serum bicarbonate and a higher anion gap are associated with lower cardiorespiratory fitness in young adults. Kidney Int (2012) 81(10):1033–42. doi: 10.1038/ki.2011.479
73. Farwell WR, Taylor EN. Serum bicarbonate, anion gap and insulin resistance in the National Health and Nutrition Examination Survey. Diabetic medicine: J Br Diabetic Assoc (2008) 25(7):798–804. doi: 10.1111/j.1464-5491.2008.02471.x
74. Liu J, Bai C, Li B, Shan A, Shi F, Yao C, et al. Mortality prediction using a novel combination of biomarkers in the first day of sepsis in intensive care units. Sci Rep (2021) 11(1):1275. doi: 10.1038/s41598-020-79843-5
75. Levi M, van der Poll T. Coagulation and sepsis. Thromb Res (2017) 149:38–44. doi: 10.1016/j.thromres.2016.11.007
76. Iba T, Levy JH. Sepsis-induced coagulopathy and disseminated intravascular coagulation. Anesthesiology (2020) 132(5):1238–45. doi: 10.1097/ALN.0000000000003122
77. Siegler JE, Marcaccio C, Nawalinski K, Quattrone F, Sandsmark DK, Maloney-Wilensky E, et al. Elevated red cell distribution width is associated with cerebral infarction in aneurysmal subarachnoid hemorrhage. Neurocritical Care (2017) 26(1):26–33. doi: 10.1007/s12028-016-0306-2
78. Abul Y, Ozsu S, Korkmaz A, Bulbul Y, Orem A, Ozlu T. Red cell distribution width: a new predictor for chronic thromboembolic pulmonary hypertension after pulmonary embolism. Chronic Respir Dis (2014) 11(2):73–81. doi: 10.1177/1479972314525057
79. Xanthopoulos A, Giamouzis G, Melidonis A, Kitai T, Paraskevopoulou E, Paraskevopoulou P, et al. Red blood cell distribution width as a prognostic marker in patients with heart failure and diabetes mellitus. Cardiovasc Diabetol (2017) 16(1):81. doi: 10.1186/s12933-017-0563-1
80. Ai L, Mu S, Hu Y. Prognostic role of RDW in hematological malignancies: a systematic review and meta-analysis. Cancer Cell Int (2018) 18:61. doi: 10.1186/s12935-018-0558-3
81. Epstein D, Nasser R, Mashiach T, Azzam ZS, Berger G. Increased red cell distribution width: A novel predictor of adverse outcome in patients hospitalized due to acute exacerbation of chronic obstructive pulmonary disease. Respir Med (2018) 136:1–7. doi: 10.1016/j.rmed.2018.01.011
82. Nada AM. Red cell distribution width in type 2 diabetic patients. Diabetes Metab syndrome obesity: Targets Ther (2015) 8:525–33. doi: 10.2147/DMSO.S85318
83. Wu H, Liao B, Cao T, Ji T, Huang J, Ma K. Diagnostic value of RDW for the prediction of mortality in adult sepsis patients: A systematic review and meta-analysis. Front Immunol (2022) 13:997853. doi: 10.3389/fimmu.2022.997853
84. Thomas-Rüddel DO, Hoffmann P, Schwarzkopf D, Scheer C, Bach F, Komann M, et al. Fever and hypothermia represent two populations of sepsis patients and are associated with outside temperature. Crit Care (2021) 25(1):368. doi: 10.1186/s13054-021-03776-2
Keywords: sepsis, diabetes mellitus, glycosylated hemoglobin, intensive care unit, all-cause mortality
Citation: Yang C, Jiang Y, Zhang C, Min Y and Huang X (2023) The predictive values of admission characteristics for 28-day all-cause mortality in septic patients with diabetes mellitus: a study from the MIMIC database. Front. Endocrinol. 14:1237866. doi: 10.3389/fendo.2023.1237866
Received: 10 June 2023; Accepted: 14 July 2023;
Published: 01 August 2023.
Edited by:
Sen Li, Beijing University of Chinese Medicine, ChinaReviewed by:
Gisha Mohan, Centinela Hospital Medical Center, United StatesVasilios E. Papaioannou, Democritus University of Thrace, Greece
Copyright © 2023 Yang, Jiang, Zhang, Min and Huang. This is an open-access article distributed under the terms of the Creative Commons Attribution License (CC BY). The use, distribution or reproduction in other forums is permitted, provided the original author(s) and the copyright owner(s) are credited and that the original publication in this journal is cited, in accordance with accepted academic practice. No use, distribution or reproduction is permitted which does not comply with these terms.
*Correspondence: Xin Huang, bXVzaHVpMTU2QDE2My5jb20=