- School of Nursing, Chengdu Medical College, Chengdu, Sichuan, China
Background: Continuous glucose monitoring (CGM) has revolutionized diabetes management, but a comprehensive analysis of its clinical implementation is lacking. This study aims to explore CGM in diabetes practice over the past decade using bibliometric analysis. It will identify trends, research focal points, and provide a framework for future investigations.
Materials and methods: The Web of Science Core Collection (WOSCC) was utilized to acquire literature pertaining to the employment of continuous glucose monitoring (CGM) in diabetes that was published between the years 2012 and 2022, and to conduct a comprehensive analysis of the associated citation data. To achieve bibliometric visualization and analysis of the collated data, the bibliography package in the Rstudio(v.4.2.2), Citespace 6.2.R4, and VOS viewer were employed.
Results: A total of 3024 eligible publications were extracted from 91 countries, with the United States being the leading country in terms of the number of issued articles. Furthermore, the annual publication rate has shown a gradual increase during the past decade. Among the various journals in this field, DIABETES TECHNOLOGY & THERAPEUTICS was identified as the most highly cited one. Keyword clustering analysis of the extracted publications indicates that the research hotspots in the past decade have primarily focused on “continuous glucose monitoring”, “glycemic variability”, “type 1 diabetes”, “hypoglycemia”, and “glycemic control”. Moreover, the analysis of keyword emergence reveals that “Time In Range” and “Young Adult” represent the current research frontiers for the years 2012-2022.
Conclusion: The concept of Time in Range (TIR) has garnered considerable attention as a significant area of inquiry and an emerging research trend in the clinical practice of Continuous Glucose Monitoring (CGM) for Diabetes Mellitus. Moreover, recent investigations have demonstrated a growing focus on young adults with type 1 diabetes as the research population of interest. In the foreseeable future, research endeavors will persist in the pursuit of improving glycemic management among young adults through the utilization of continuous glucose monitoring (CGM) technology, while also delving into the examination of the Time in Range metric via supplementary clinical investigations.
1 Introduction
Diabetes, a chronic non-communicable disease, ranks third after cardiovascular and cerebrovascular diseases and tumors in posing a serious risk to human health. With the accelerating pace of urbanization, lifestyle modifications, and the aging of the population, the prevalence of diabetes is escalating rapidly. According to the International Diabetes Federation (IDF), the global prevalence of diabetes among individuals aged 20 to 79 years is estimated to be 10.5% (536.6 million people) in 2021, and this figure is projected to reach 12.2% (783.2 million people) by 2045. The global health expenditure associated with diabetes is also projected to rise continuously (1, 2). Blood glucose serves as the fundamental source of energy in the body and plays a pivotal role in maintaining normal physiological functions. Abnormal fluctuations in blood glucose levels are closely linked to the onset and progression of numerous diseases, such as diabetes mellitus and hypoglycemia. Consequently, blood glucose monitoring has emerged as a crucial and indispensable tool in clinical management. Moreover, with the growing focus on health, an increasing number of individuals are becoming aware of their blood glucose levels and adopting corresponding measures to safeguard their well-being. Continuous glucose monitoring (CGM) is a non-invasive technique that enables the continuous monitoring of the concentration of glucose in subcutaneous interstitial fluid through the use of a glucose sensor. This technology facilitates the recording of the trend and characteristics of blood glucose fluctuations in real time (3, 4). Scanning CGMs, in particular, can provide continuous glucose monitoring for up to 14 days, with the sensor measuring glucose levels every minute and storing readings every 15 minutes. Scanning allows for the presentation of continuous and reliable information on blood glucose fluctuations throughout the day. The CGM system is factory-calibrated, eliminating the need for frequent finger-stick blood calibrations during use. This feature reduces the discomfort of blood collection, promotes patient compliance and initiative in blood glucose monitoring (5), and facilitates ease of operation. With the proliferation of continuous glucose monitoring (CGM) in clinical practice, it has emerged as a widely utilized tool for ambulatory glucose monitoring, facilitating the monitoring of blood glucose levels and the identification of uncontrolled hyperglycemia, hypoglycemia, and fluctuations in blood glucose (6). Consequently, prospective clinical studies have increasingly adopted CGM devices to gather data and evaluate the blood glucose profiles of study participants, in conjunction with HbA1c findings, in order to further assess the efficacy of therapeutic interventions on HbA1c (7). Given the expanding evidence supporting the efficacy of CGM in diabetes treatment and its rising demand in primary care, it is imperative to attend to its clinical use for diabetes (8). In light of these contextual factors, this research delves comprehensively into the clinical practice of Continuous Glucose Monitoring (CGM) within the domain of diabetes. This includes investigating its impact on glycemic control, the utilization of CGM-related metrics, remote monitoring and telemedicine applications, artificial pancreas(closed-loop systems), as well as integration with insulin pump mechanisms, among other facets (9).The vast quantity of research-related literature currently being produced presents a challenge for traditional literature analysis in obtaining comprehensive and pertinent information. Bibliometric analysis, however, enables both quantitative and qualitative information contained within journal articles to be analyzed (10). This approach has been proven effective in identifying emerging topics and research frontiers across a wide range of disciplines (11, 12). Accordingly, in this study, we employ scientific bibliometric analysis to systematically examine published works, with the aim of revealing annual publication outputs, identifying leading countries, regions, journals, and institutions, and evaluating research impact. We further report on the research impact of countries, regions, institutions, and journals through analysis of keywords and co-cited literature. Finally, we explore current research hotspots and future trends in the use of CGM in clinical practice for diabetes.
2 Materials and methods
2.1 Extraction of citation data
On the 1st of August 2023, a comprehensive search was conducted on the Web of Science Core Collection (WOSCC) to retrieve all citations published from the 1st of January 2012 to the 31st of December 2022. The search was executed using the following formula: TS=(“Continuous blood glucose monitoring” OR “Continuous glucose monitoring” OR “Implantable CGM system” OR CGM OR FGM OR “Flash glucose monitoring” OR “Ambulatory glucose monitoring” OR “Continuous glucose sensors” OR “Real-time glucose monitoring” OR rtCGM OR “Subcutaneous glucose monitoring” OR “Continuous glucose measurement” OR “Continuous glycemic monitoring” OR “Continuous glucose sensing” OR “Continuous glucose meters”) AND TS=(Diabetes* OR “Diabetes mellitus*”), while limiting document types to “Article” or “Review Article”. Articles and reviews written in English were considered, while meeting abstracts, early access articles, editorial material, letters, collections, proceeding papers, news items, book chapters, hardware reviews, and withdrawn publications were excluded. The initial screening process yielded a total of 3680 original English articles, comprising 3207 articles and 473 reviews, which were deemed to be potential candidates for inclusion in the study.
To ensure the precision and caliber of the acquired data, a dual review process was undertaken by two researchers, Laixi Kong and Maoting Guo, who independently scrutinized the abstracts and keywords of literature to obtain the most pertinent articles. The objective of this study was to investigate the clinical practice of CGM in diabetes; thus, these clinical practice encompass various aspects: improvements in blood glucose control following CGM use, the utilization and interpretation of CGM-related metrics, remote monitoring and telemedicine, artificial pancreas (closed-loop systems), and the integration of CGM with multiple insulin pump systems. Exclusion criteria encompass topics such as technical design in CGM sensors, sensor material research, and unrelated reviews. Following manual screening, a total of 3024 papers were deemed suitable for inclusion in this study. From each publication, the title, publication year, country or region, institution, author, journal, references, author and keywords were methodically extracted. Further details pertaining to the literature extraction process are presented in Figure 1.
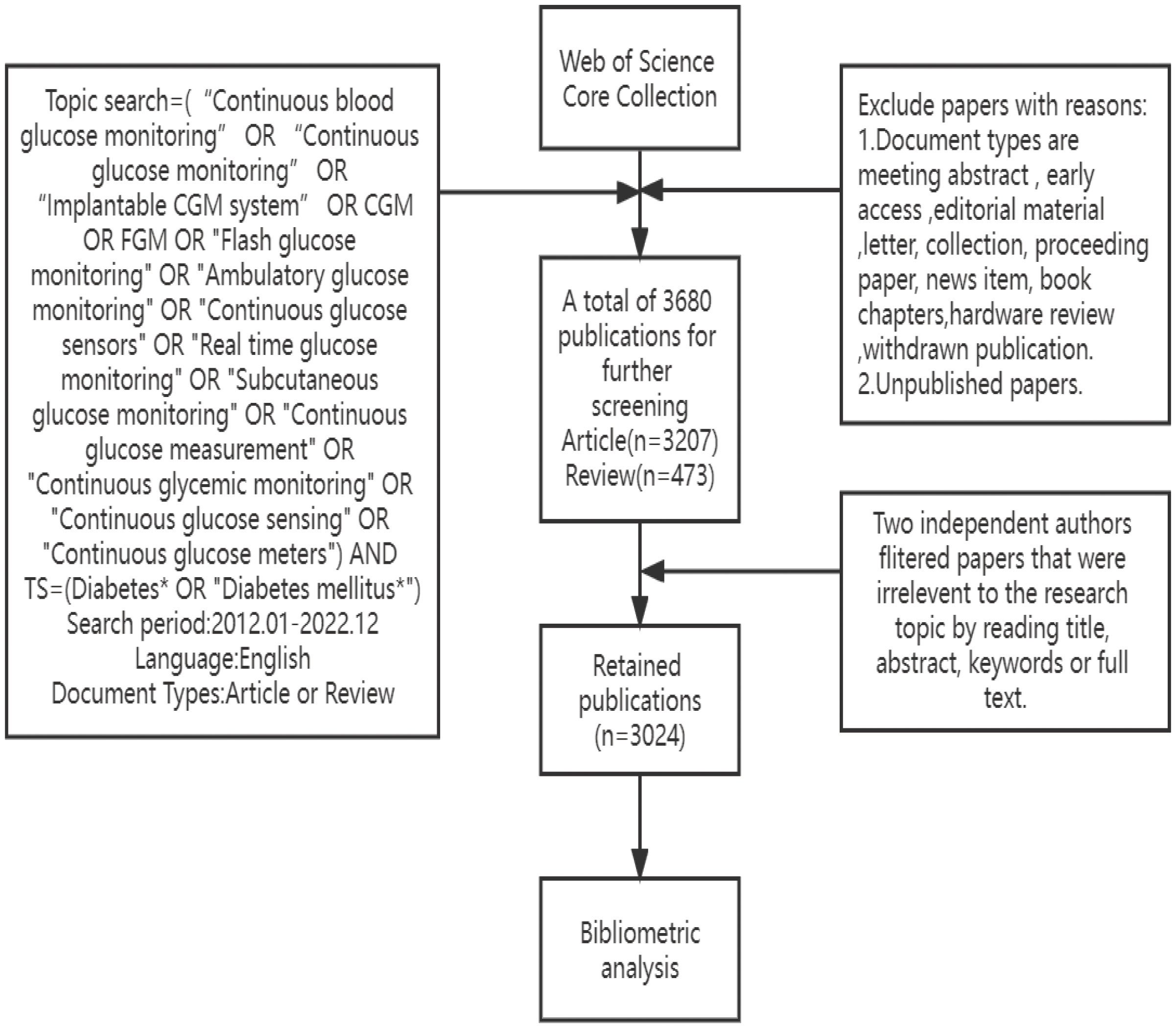
Figure 1 The framework diagram illustrates the comprehensive screening methodology employed in the evaluation of research literature pertinent to the clinical practice of Continuous Glucose Monitoring (CGM) in Diabetes Mellitus from 2012-2022.
2.2 Statistical analysis
Initially, a basic statistical analysis of the dataset was conducted utilizing Rstudio (v.4.2.2). The “bibliometrix” format was employed to store the data, and the “biblishiny” package was utilized to extract a range of features related to the research literature between 2012 to 2022 (13). These features, including Main Information, Most Relevant Authors, Author’s Production Over Time, Most Global Cited Documents, served for quantitative analysis. Subsequently, CiteSpace 6.1.R6 was utilized to cluster the keywords of institutions present in the literature, perform dual map overlay analysis of journals, unveil keyword clustering analyses within the text, identify the strongest cited bursts, and construct co-cited references timeline maps of publications. In addition, VOS viewer was employed to identify the collaborative networks of countries and institutions, evaluate the keywords pertaining to the subject, and visualize the post-analysis of the results.
3 Results
3.1 Publications
In this review, a comprehensive analysis of 3024 literature sources was conducted, and the resulting search data was used to plot the trends in studies related to the application of continuous glucose monitoring (CGM) to clinical practice in diabetes using R studio. As illustrated in Figure 2, the analysis revealed a consistent annual increase in the volume of research articles on this subject from 2012 to 2019, followed by a sharp rise in the number of publications from 2019 to 2021, suggesting a heightened interest in research pertaining to clinical practice of CGM in diabetes during this period. Notably, 2021 recorded the highest output of 555 articles. Furthermore, a linear trend line of annual publications was developed to gain further insights into the output trend, resulting in the equation Y=44.745X+6.4364, where Y represents the annual publications and X denotes the year. This model exhibits a coefficient of determination (R²) of 0.8819. Figure 3 presents an overview of the analyzed articles, encompassing a total of 46243 references and an average publication year of 4.37. Moreover, each article garnered an average of 22.88 citations, while the annual publication growth rate was 15.83%.
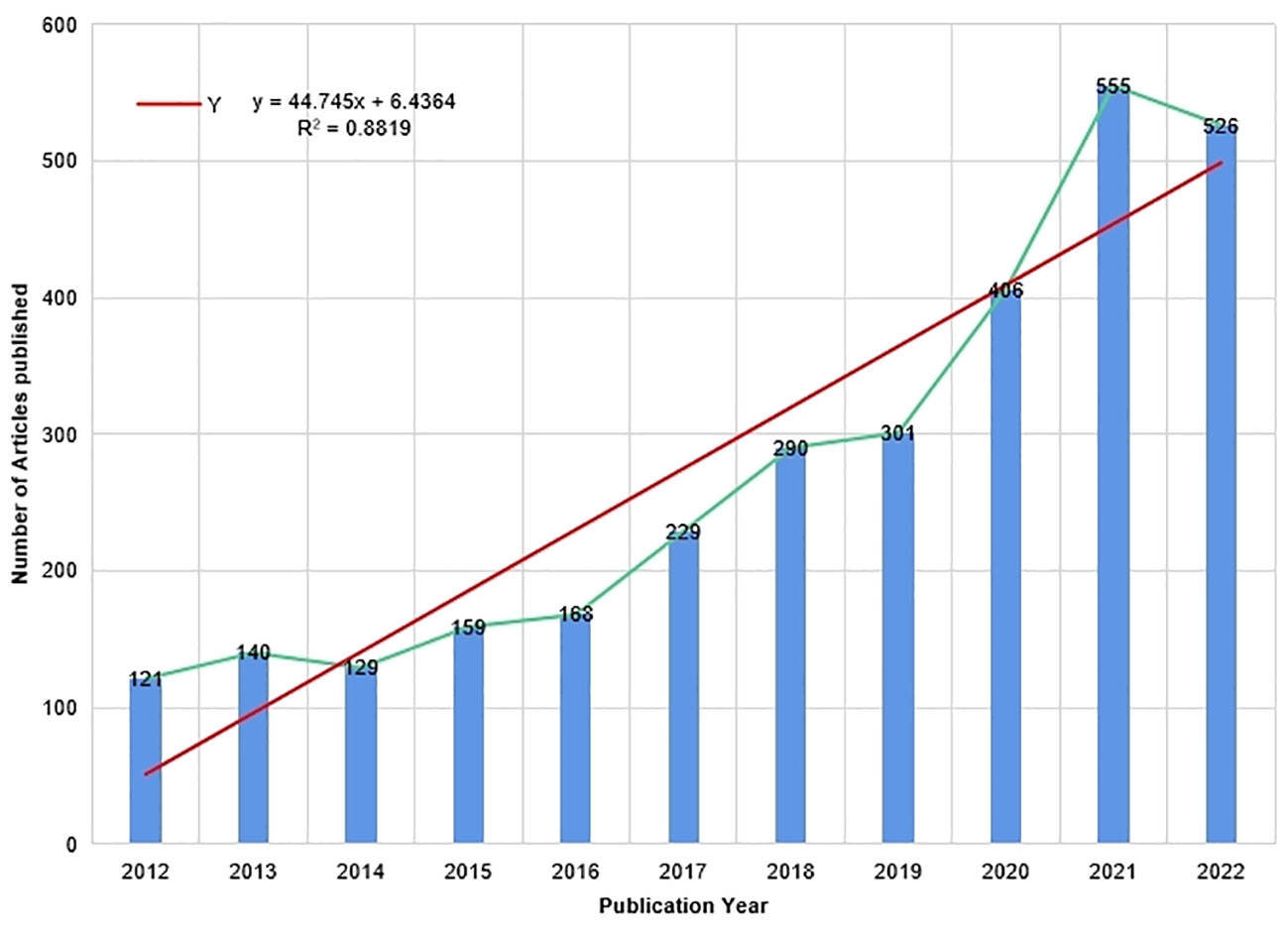
Figure 2 Trends in the Number of Publications on the Clinical Practice of CGM in Diabetes Mellitus from 2012 to 2022.
3.2 Countries and regions
A total of 91 countries have conducted studies on the topic at hand. The Figure 4A indicated that the United States had the highest number of articles published (1029), followed by the United Kingdom (331) and China (264). The top 10 countries in terms of output were summarized in the Table 1, with the United States exhibiting the highest centrality (0.17), H-index (86), and Citations Per Papers (32.00), surpassing other countries by a significant margin. Although China and Japan ranked high, their H-index and centrality were comparatively lower than those of other countries. The international cooperation relationship of each country was visualized using the CiteSpace, as shown in Figure 4B, where nodes represented countries and node size reflected the amount of national issuance. The purple portion of the circle represented centrality, with the United States positioned at the center, indicating frequent cooperation with other countries. Furthermore, the circle of the United States was the largest, indicative of the most influential issuance in the region.
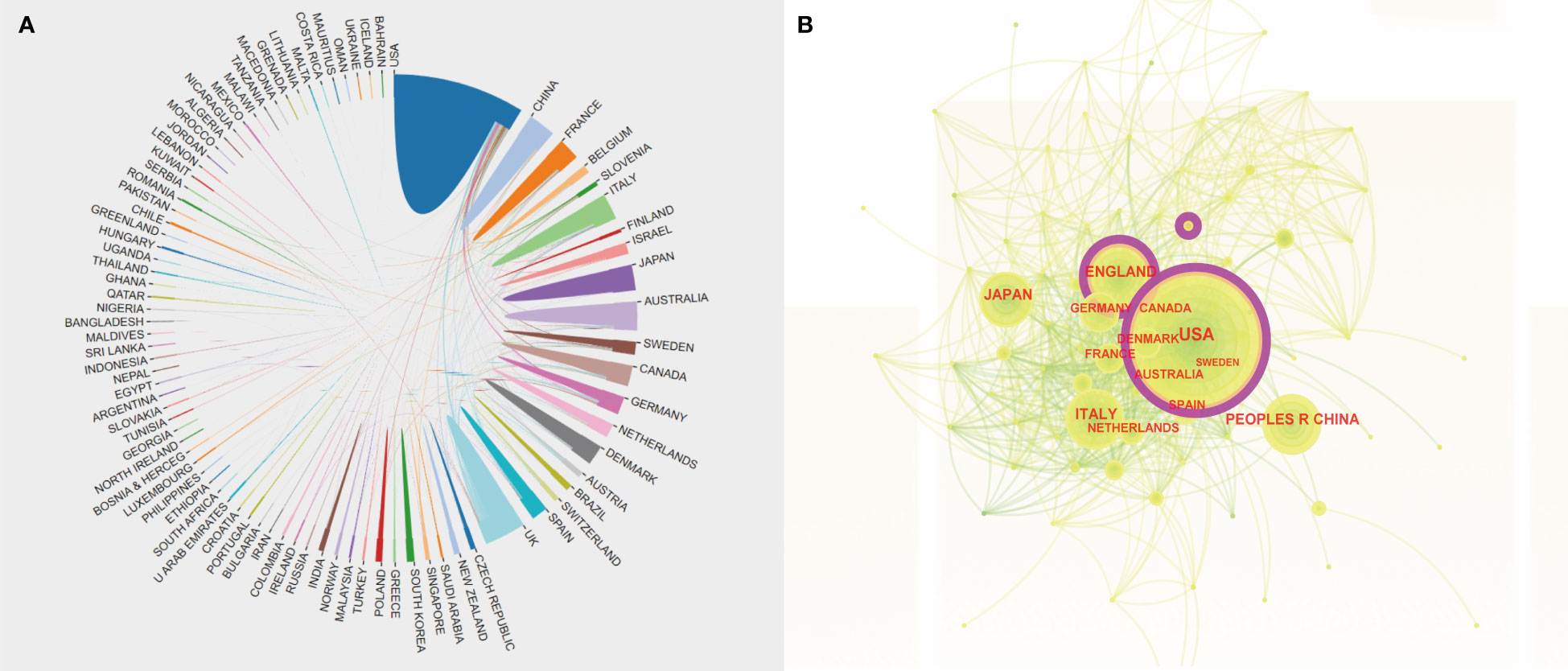
Figure 4 (A) An Analysis of International Cooperation Between Diverse Countries. The correlation among distinct color blocks signifies the bilateral collaborative association between the two countries. (B) Cooperation of Countries or Regions that Contributed to Publications on the Clinical Practice of CGM in Diabetes Mellitus from 2012 to 2022. The size of the purple ring area serves as an indicator of the scope of influence of the regional articles and is equivalent to their centrality.
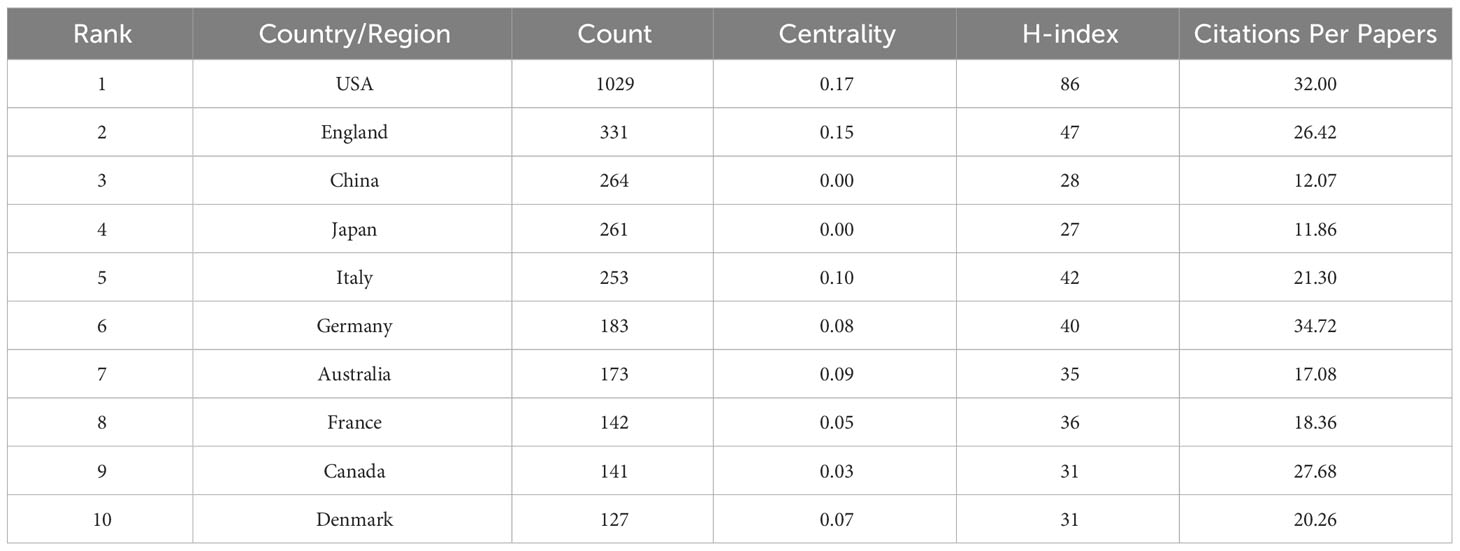
Table 1 Top 10 countries or regions with publications on clinical practice of CGM in diabetes mellitus from 2012 to 2022.
3.3 Institutions
Table 2 illustrates the top 10 institutions with the highest literature output, where in HARVARD UNIVERSITY, UNIVERSITY OF COLORADO SYSTEM, and UNIVERSITY OF COLORADO ANSCHUTZ MEDICALCAMPUS emerged as the top three institutions with the highest number of published articles (151, 144, and 132, respectively). Notably, HARVARD UNIVERSITY exhibited a significantly higher H-Index compared to other two institutions, indicating its dominant influence in publishing articles. Seven out of the top 10 institutions were affiliated with the United States.
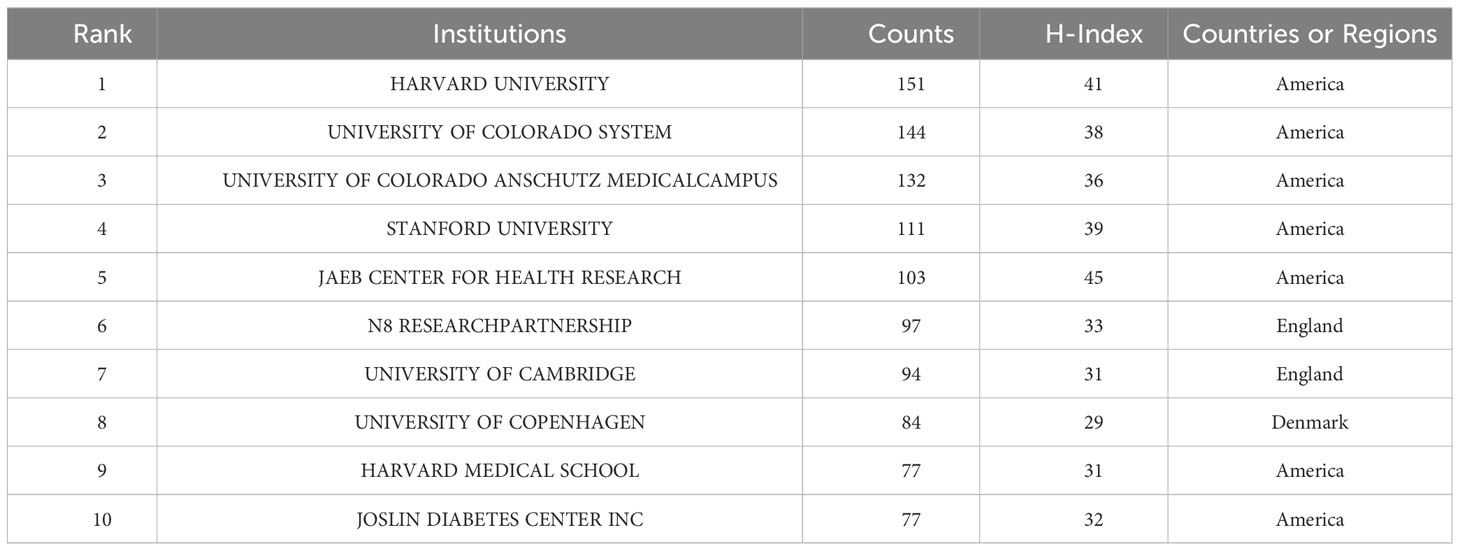
Table 2 The top 10 institutions with publications on clinical practice of CGM in diabetes mellitus from 2012-2022.
The collaborative relationships between institutions were disclosed through the use of CiteSpace, as depicted in Figure 5A. The connecting line between each of the two labels in Figure 5B shows that the institutions in the same country cooperate closely.
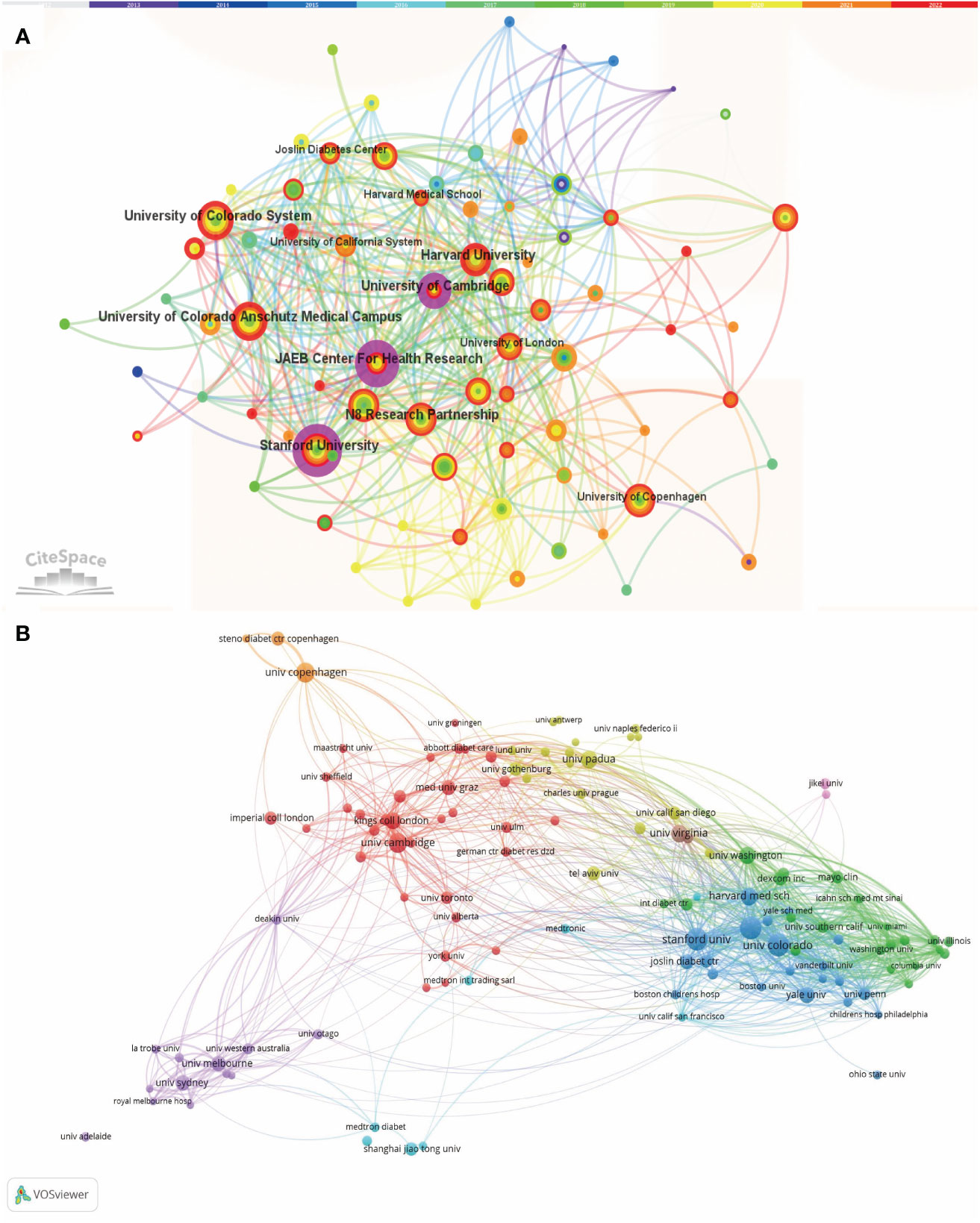
Figure 5 (A) Collaborative Network Analysis by CiteSpace Amongst Institutions Pertaining to the Clinical Practice of CGM in Diabetes Mellitus from 2012 to 2022. Each node with colorful annual rings represents an institution, and the size of each node represents its relative quantity of research output. (B) The overlay visualization map of Institution co-authorship analysis conducted by VOSviewer.
3.4 Analysis of authors
Figure 6A presents the roster of the ten most pertinent authors within this research domain, with particular emphasis on the 61 articles affiliated with Roy W. Beck. A nuanced comprehension of the potency of influence and the yearly evolution of publications among these ten authors over the past decade is facilitated by Figure 6B. Evidently, Roy W. Beck sustains a conspicuously high echelon of scientific impact within this research sphere (14). Remarkably, it is salient that seven studies associated with him have ascended to constitute the upper echelon of the ten most frequently cited articles within this field (15–21).
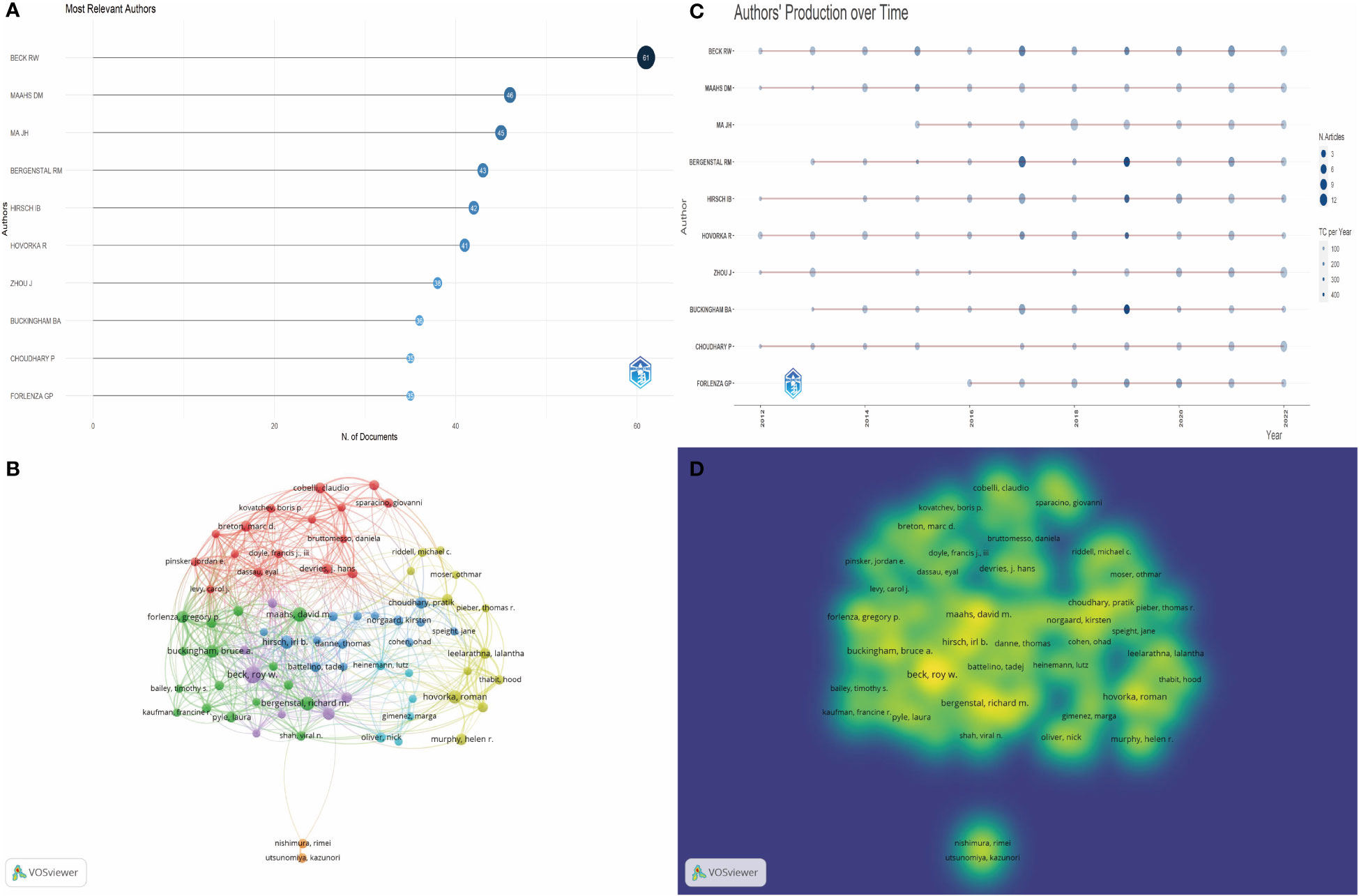
Figure 6 (A) Top 10 most relevant authors in this field. (B) The visual depiction of author co-citation analysis using VOSviewer showcases a graphical representation where each author is symbolized as a distinct node. The size of these nodes is proportionate to the total number of citations received. Connections between nodes signify instances of co-citation, implying a connection between the authors' works. The proximity between nodes reflects their degree of association, with shorter distances denoting a stronger relationship. Nodes with closer proximity are grouped together using matching colors, indicating their membership in a shared cluster. (C) Top 10 authors’ production over time. The circle size represents the number of documents (N. Documents), and the shade of the color signifies the total number of citations (TC). (D) The visualization of author collaboration patterns produced by VOSviewer depicts clusters denoting groups of authors with significant collaborative ties. Authors closely linked in terms of cooperation are represented within a shared cluster, visually distinguished by a common color.
In light of these dynamics, collaborative networks of research materialize as instrumental conduits for researchers to augment the breadth of their investigative pursuits or to conjoin forces with cohorts engaged in cognate inquiries. Accordingly, a judicious author threshold of 107 was established. Employing VOSviewer, we proceeded to visualize the extant panorama and gradation of author interplay within this domain, with the ensuing outcomes being expounded in Figures 6C, D.
3.5 Journals
Upon analyzing the literature’s cited and citing journals, it was possible to determine the influential journals in the field. Table 3 illustrated the top ten cited and citing journals, with DIABETES TECHNOLOGY & THERAPEUTICS holding the highest rank as the first citing journal, followed by DIABETES CARE and DIABETES RESEARCH AND CLINICAL PRACTICE. Among the cited journals, DIABETES CARE held the top spot, followed by DIABETES TECHNOLOGY & THERAPEUTICS and J DIABETES SCI TECHNOL. In 2022, DIABETES CARE held the highest impact factor among the citing journals, with a score of 17.152, followed by DIABETES TECHNOLOGY & THERAPEUTICS with a score of 7.337.
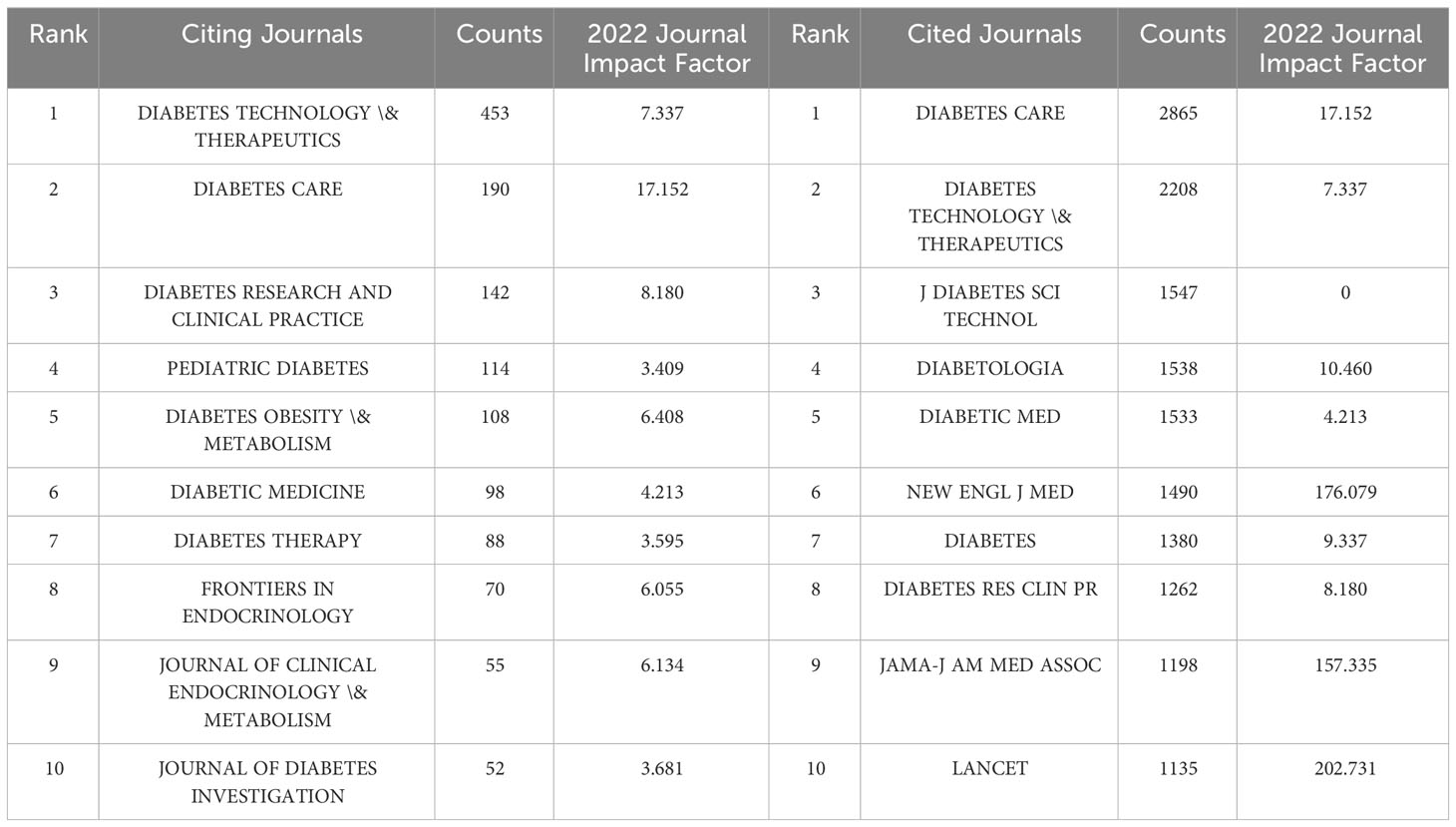
Table 3 The Top 10 citing and cited journals of publications on the clinical practice of CGM in diabetes mellitus from 2012 to 2022.
Furthermore, in plotting the Dual map overlay using CiteSpace, the journals that contributed to publications on the clinical practice of CGM in Diabetes Mellitus were analyzed. The resulting map in Figure 7 was divided into two halves, with the left side representing the research area of the cited journals and the right side depicting the research area of the citing journals. The colored curves between the nodes on the left and right halves illustrated the relationship between the highly active research areas of the two journals. The examination of the graph indicated the presence of two discernible green curves, which implied that publications pertaining to medicine, medical and clinical domains have a higher likelihood of being referenced by journals that focus on molecular, biological, and genetic areas, as well as health, nursing, and medical fields.
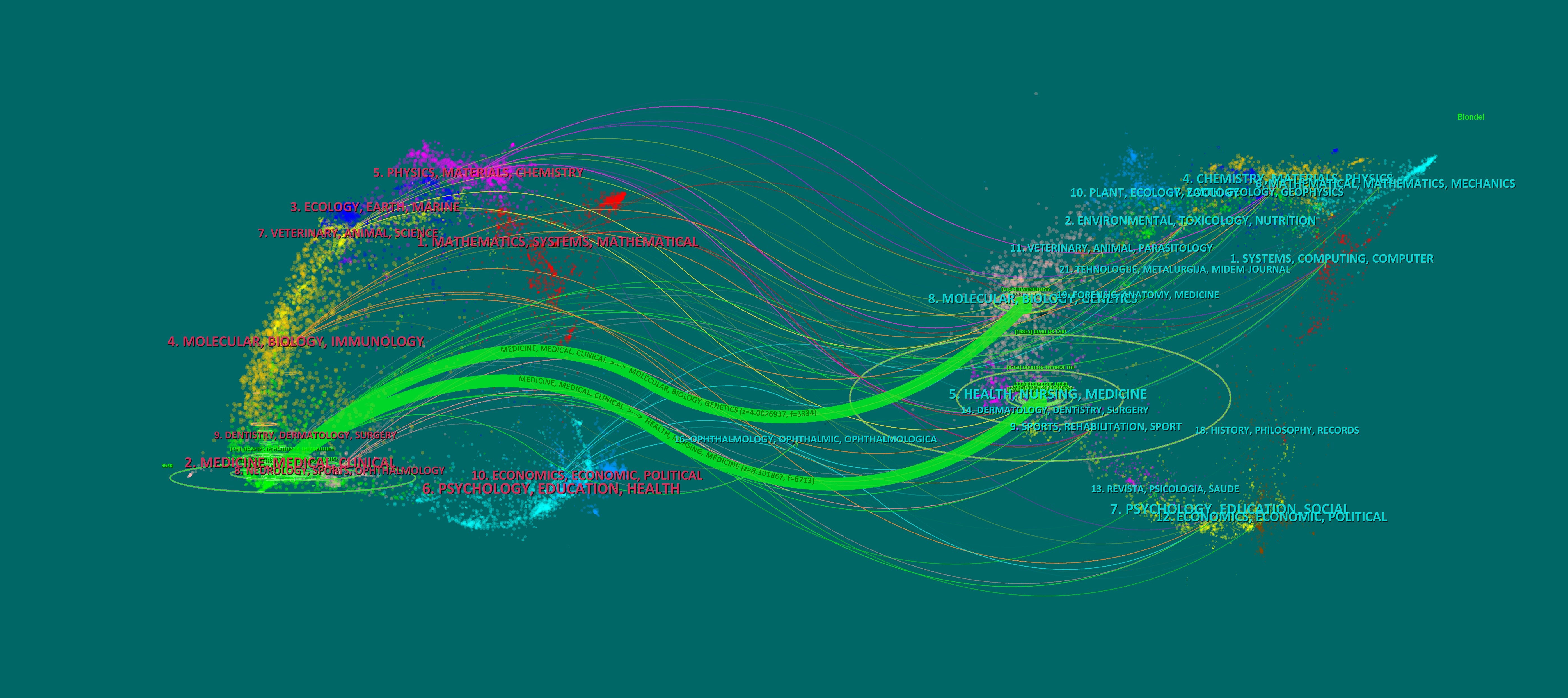
Figure 7 The Dual-map Overlay of Journals on the Clinical Practice of CGM in Diabetes Mellitus. The green path at the top suggests that research literature from MEDICINE, MEDICAL, CLINICAL area may be utilized to support the results and findings in the field of MOLECULES, BIOLOGY, GENETICS research. The findings from research literature in the MEDCINE, MEDICAL, CLINICAL area may be utilized to support the results from research conducted in the HEALTH, NURSING, MEDICINE area.
3.6 Keywords
In this study, we utilized VOSviewer to visualize the 100 high-frequency keywords in the literature pertaining to the topic of interest. A threshold of 41 occurrences was set for the selection of these keywords. The resulting visualization in Figure 8A demonstrated that darker color blocks corresponded to higher frequency of occurrence of the respective keyword in the literature. Furthermore, proximity of a color block to the center of the yellow block indicated higher citation frequency and cited frequency. The top ten hot keywords, as ranked by frequency, were presented in Table 4. Notably, “Type 1 diabetes”, “Glycemic control”, “Hypoglycemia” were among the top keywords, suggesting their significance as hot topics in this research field over the past decade. And Figure 8B illustrates the chronological depiction of keyword clustering analysis, offering a timeline perspective. The diagram portrays various clusters denoted by distinctively colored horizontal lines on the right side, each corresponding to a collection of keywords. The nodes positioned along these horizontal lines symbolize individual keywords. Notably, the spatial arrangement of these nodes along the horizontal axis signifies the inaugural appearance year within the scholarly literature for the associated keyword, thereby constituting a comprehensive temporal representation of the keyword cluster’s evolutionary progression. The cluster “0# glycemic variability” is the largest, Next is “#1 artificial pancreas”, “#2 physical activity”, “#3 type 2 diabetes”, “#4 flash glucose monitoring” and “#diabetes mellitus”
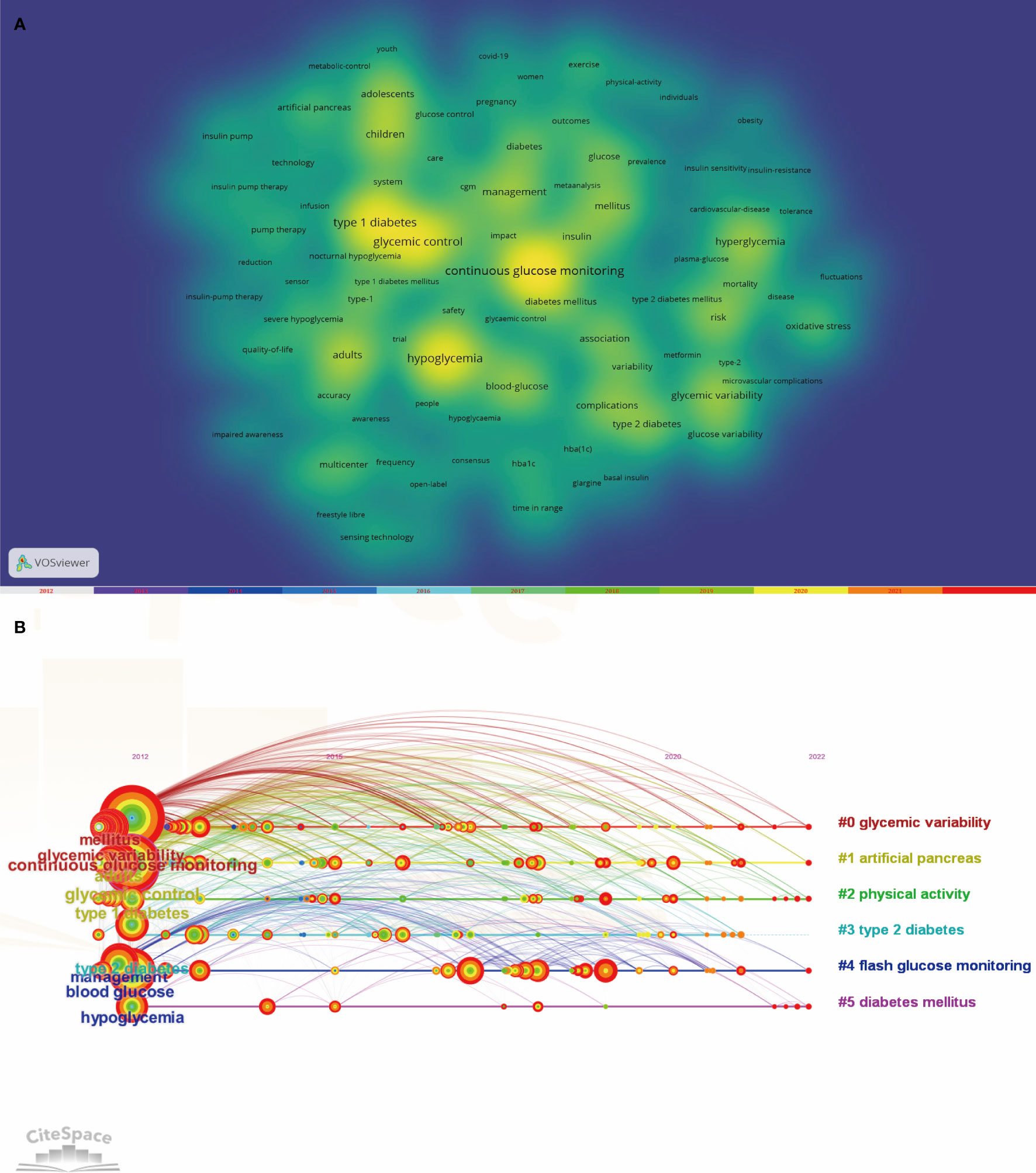
Figure 8 (A) Co-occurrence Keywords Network and Density Visualization on the Clinical Practice of CGM in Diabetes Mellitus from 2012-2022. (B) CiteSpace visualization timeline view of keywords clustering analysis related to the clinical practice of CGM in diabetes.
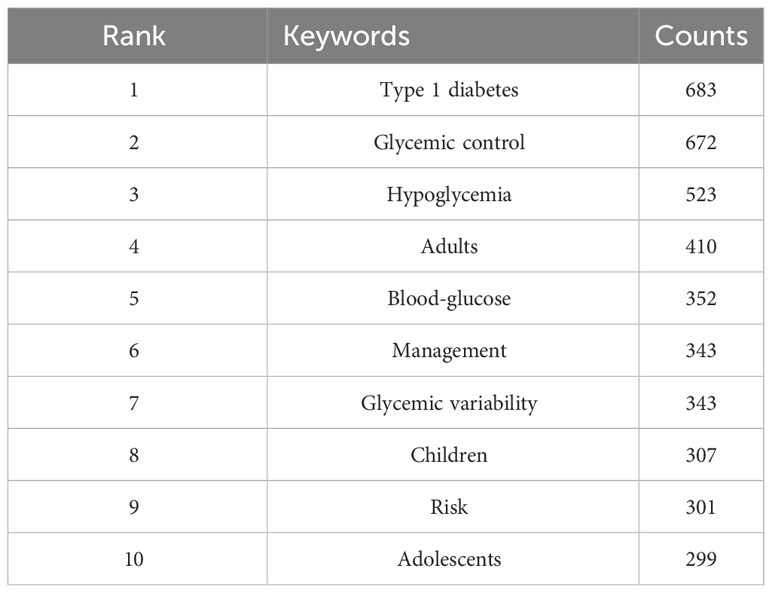
Table 4 Top 10 keywords related to the clinical practice of CGM in diabetes mellitus from 2012-2022.
The CiteSpace is capable of identifying keywords that experience significant changes in frequency during a specific time period, commonly known as emergent words. Keywords that exhibit a delayed emergence and extended duration are indicative of the most recent research trends in a given field, enabling a temporal review of research hotspots and the projection of future trends. The default configuration of CiteSpace was substituted with the ensuing modes: “Year Per Slice” set to 1, “Top N%” set to 30.0%, and “Minimum Duration” set to 1. After conducting an analysis on the keywords with citation bursts, we determined that the 8 strongest burst keywords should be displayed as illustrated in Figure 9. During the period spanning from January 2012 to December 2022, the ensuing keywords surfaced as outcomes: fluctuation (2012-2018), plasma glucose (2012-2016), hyperglycemia (2013-2016), cardiovascular disease (2013-2016), reduction (2014-2017), intensive treatment (2018-2020), time in range (2020-2022), and young adults (2020-2022). In the preceding two years, the keywords “Time in Range” and “Young Adult” have surfaced and persisted throughout 2020 and beyond. Of the two, “Time in Range” has exhibited the most intense outbreak with a value of 20.47, indicating that it currently represents the primary research focus and potentially marks a pivotal juncture with notable implications for future inquiry.
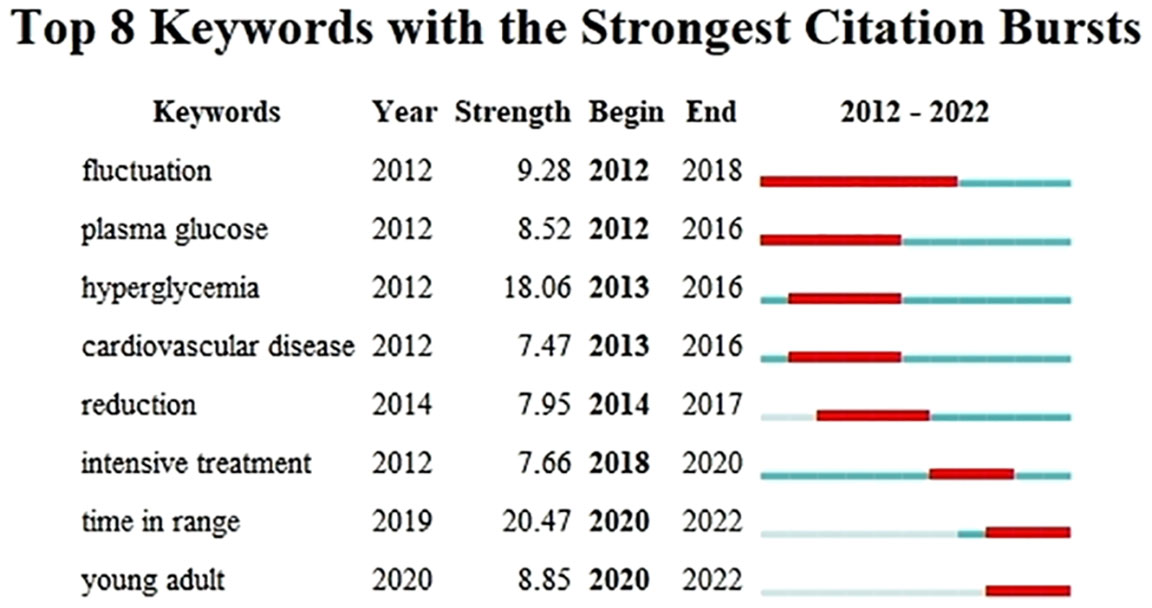
Figure 9 Keywords with the Strongest Citation Bursts for Publications on the Clinical Practice of CGM in Diabetes Mellitus Diabetic from 2012- 2022.
3.7 Co-cited references
A timeline map of co-cited references was constructed using CiteSpace with the aim of comprehending the principal research topics and their progression within the field. The outcomes of the keyword clustering analysis of the references were exhibited on the right-hand side of Figure 10, with “#flash glucose monitoring” comprising the most significant cluster. On the left-hand side, the citation relationship among each reference was presented over time, wherein larger nodes signified more frequent citations, and node color indicated the time when the reference was cited. The top 10 most frequently cited references were enumerated in Table 5.
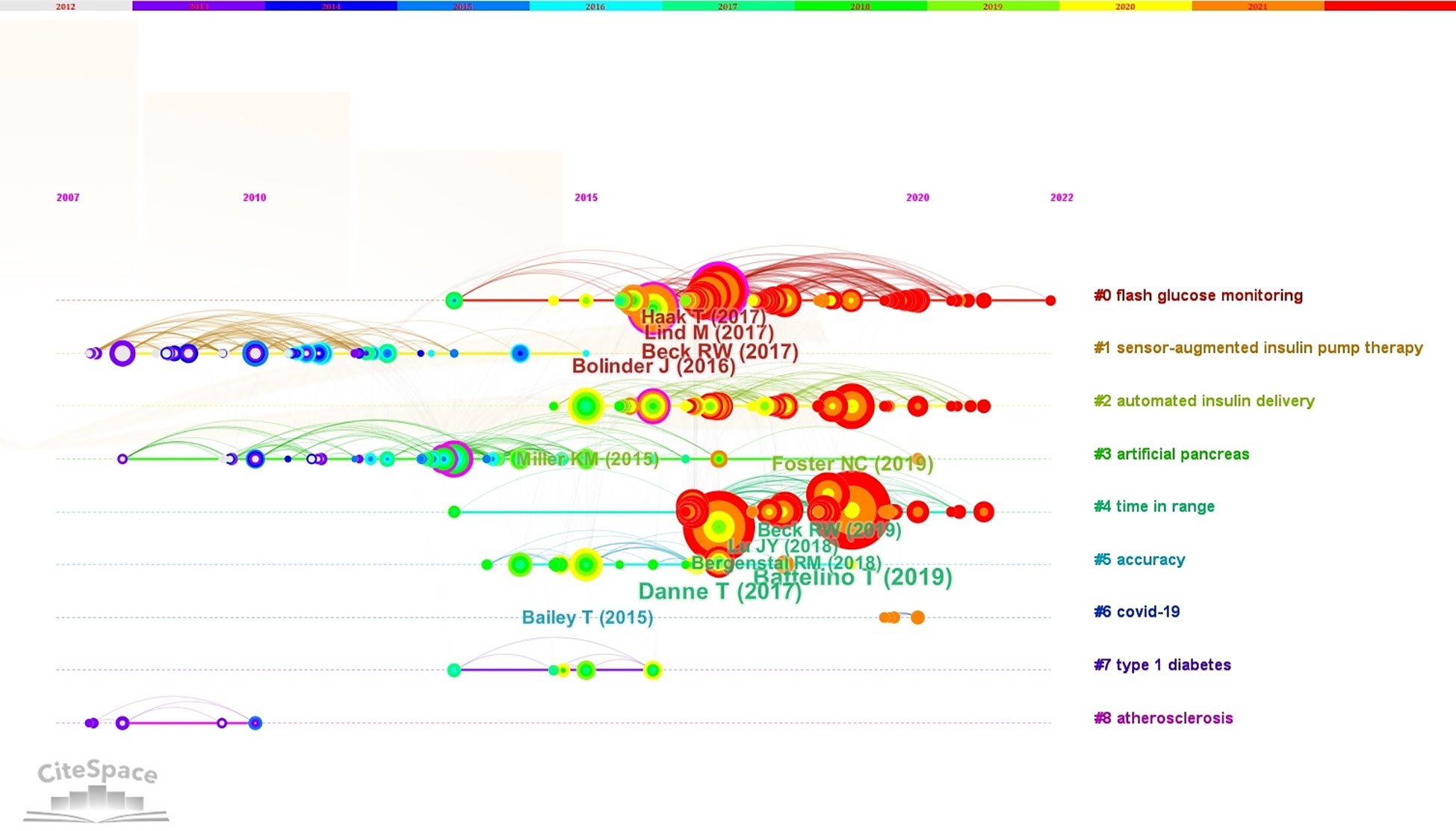
Figure 10 The Timeline View of Keyword Clustering Analysis Related to the Clinical Practice of CGM in Diabetes Mellitus was Visualized Using CiteSpace. The clusters formed by the keywords were represented by different colored horizontal lines, with labels on the right. The nodes positioned on these horizontal lines depicted the keywords themselves, while the position of the nodes on the horizontal lines indicated the year of the literature in which the keywords first appeared, thus forming a timeline representing the evolution of the keyword clusters.
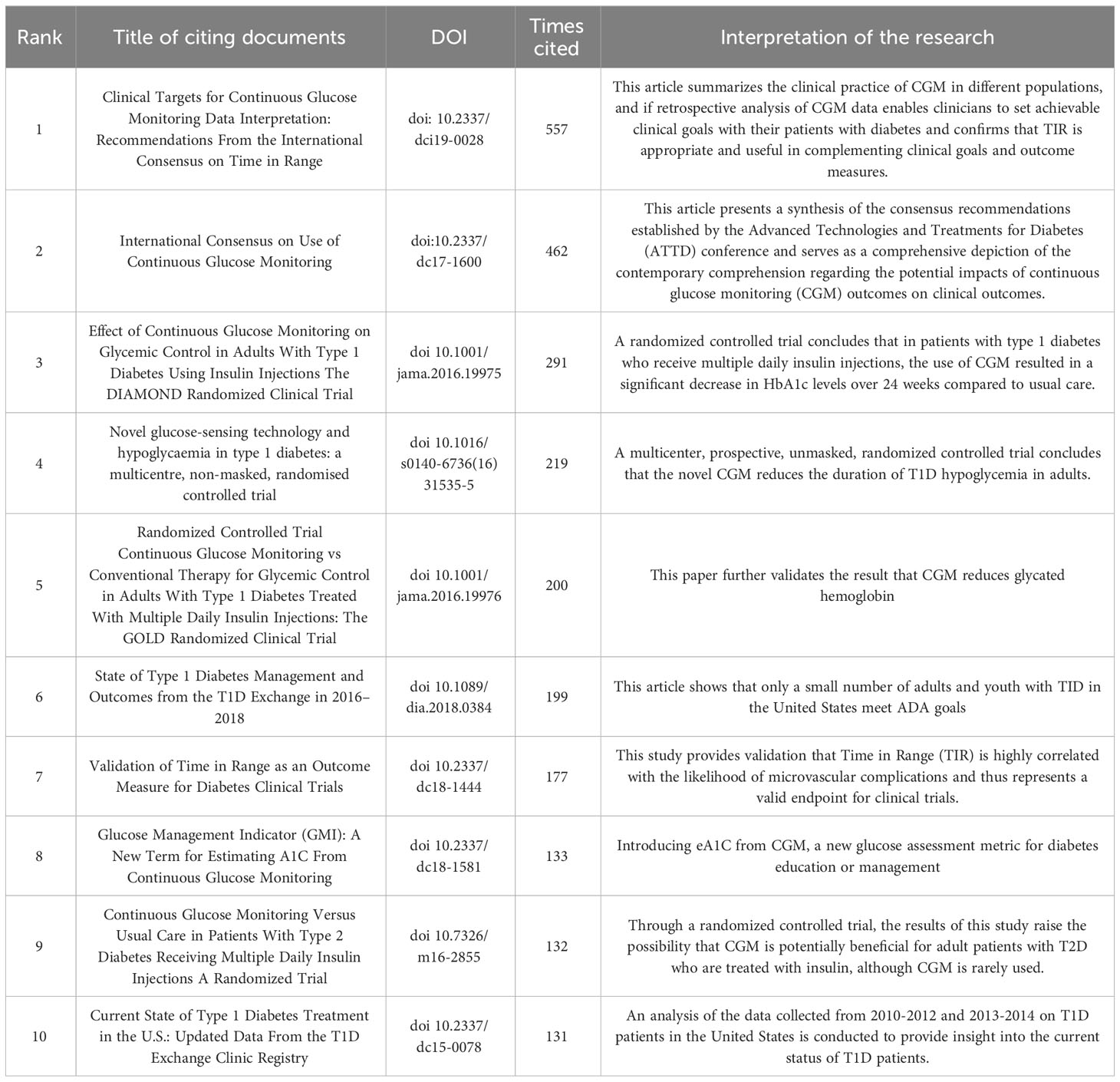
Table 5 The Top 10 references of publications on the clinical practice of CGM in diabetes mellitus from 2012 to 2022.
4 Discussion
4.1 General information
In brief, the annual production of scholarly articles in this field exhibited an upward trend overall, with a significant and substantial surge observed in 2020, likely due to the outbreak of the COVID-19 pandemic. The pandemic-induced public health measures have altered people’s lifestyles, potentially impacting the glycemic control of individuals with diabetes by limiting physical activity to some extent (22). Furthermore, the use of glucocorticoid therapy may exacerbate hyperglycemia once severe infections such as COVID-19 pneumonia have developed. Consequently, a study has suggested that a system that integrates telemedicine and continuous glucose monitoring (CGM) can effectively manage blood glucose levels and prevent adverse outcomes (23, 24). As a result, CGM has gained increasing adoption in clinical settings, with a peak in related research output in 2021. In the analysis of countries within a particular research area, the United States emerged as the leading contributor in terms of number of publications, centrality, H-index, and citations. This suggested that the United States possessed a greater level of influence within this research area and engages in frequent collaborations with other countries. Based on the aforementioned analysis, it is recommended that research teams hailing from Asian countries seek to augment their international influence by engaging in heightened cooperation with their counterparts in European and American nations. The dual map overlay depicted in Figure 7 reveals a wide range of subject areas covered by cited and citing journals, indicating untapped potential for further exploration within this research area. By employing a clustering analysis approach and examining the emergence of keywords and references, we have been able to identify research hotspots between 2012 and 2022 and forecast future trends in this research area. Notably, the most cited reference is a review authored by Tadej Battelino, Thomas Danne et al. This international consensus validated the feasibility of using the TIR index as a clinical endpoint and outcome measure to supplement HbA1c in various relevant populations, and the target thresholds outlined in the article serve as a valuable framework and point of reference for the clinical application of CGM (15).
4.2 Research hotspots
The fundamental essence of an academic field can be encapsulated by its keywords, and through visual analysis of these keywords, one can discern the prevailing research trends and trajectories (25). Based on the high-frequency keywords extracted and the keyword clustering timeline mapping generated by CiteSpace, the primary research areas in this field during the past decade can be identified. These areas include continuous glucose monitoring (CGM), glycemic variability, type 1 diabetes, and hypoglycemia. Significantly, the other three hot keywords were all generated based on CGM.
In the realm of diabetes management, the advent of continuous glucose monitoring (CGM) technology has bestowed unprecedented prospects for the monitoring and regulation of patient glycemic levels. Traditional intermittent approaches to glucose monitoring have progressively exhibited their inherent limitations, rendering the comprehensive capture of blood glucose fluctuations throughout a patient’s diurnal existence a challenging endeavor. In contrast, the real-time and uninterrupted monitoring attribute intrinsic to CGM technology introduces a novel instrument for therapeutic guidance, both for medical practitioners and their patients. The study of glycemic variability has emerged as a pivotal domain of investigation in contemporary times, and concomitant with the evolution of CGM technology, its integration within clinical practice has experienced a notable escalation in recent years (26, 27). Recent findings have indicated a correlation between elevated glycemic variability (GV) and the advancement and escalation of vascular complications in diabetic patients, heightened susceptibility to hypoglycemic episodes, as well as a decline in the quality of life (QOL) for affected individuals (28–30). The metrics encompassing Glycemic Variability are presently acknowledged as a significant gauge of glycemic management (31). This underscores the imperative of delving into the systematic examination of continuous glucose monitoring (CGM) data, and underscores the criticality of adeptly harnessing and interpreting CGM data to effectively serve its role within the realm of clinical practice.
The assessment of transient glycemic fluctuations is frequently derived from continuous glucose monitoring standard deviation (CGM.SD), a readily computable metric commonly employed to quantify short-term glycemic variability. However, it is worth noting that CGM.SD is influenced by the prevailing mean glucose levels, thereby rendering it susceptible to this parameter. Conversely, the coefficient of variation (CV), derived from both the standard deviation and mean glucose, serves to ameliorate this inherent limitation by compensating for the aforementioned sensitivity to mean glucose levels (15). Furthermore, within clinical settings, diverse indices such as the mean amplitude of glycemic excursion (MAGE), J-index, low blood glucose index/high blood glucose index, average daily risk range, and mean of daily differences (MODD) are employed to evaluate distinct facets of glycemic fluctuations in patients (32–36). Additionally, the Time in Range (TIR) parameter, denoting the proportion of time during which blood glucose levels remain within specified glycemic thresholds, while not strictly categorized as a glycemic variability metric, assumes significance as a supplementary clinical target and an outcome measure for HbA1c assessment across a spectrum of diabetes mellitus presentations, as established by international consensus (15). Consensus opinions have also established a link between TIR and the risk of diabetic complications, such as the close association between TIR and the risk of microvascular complications (19), as well as the good correlation between TIR and HbA1c. Additional research has further substantiated the notion that HbA1c inadequately captures data pertaining to the fluctuation of blood glucose levels or the duration of time spent within the hypoglycemic or hyperglycemic spectrum. Consequently, the Time in Range (TIR) metric is presently being embraced as a favored metric for prognosticating the susceptibility to diabetic complications, delineating outcomes in clinical investigations, and evaluating glycemic management in patient cohorts (37, 38).
As the keyword emergence shown in Figure 9, the emerging keywords for 2020 to 2022 are “Time in Range” and “Young Adult”. Research conducted in the realm of clinical practice concerning Continuous Glucose Monitoring (CGM) throughout this timeframe has been predominantly centered around acquiring more precise and up-to-the-minute data pertaining to glycemic regulation. Additionally, investigations have been dedicated to the viability of employing Time in Range (TIR) as a quantifiable parameter, alongside examinations concentrated on the demographic of young adults. Envisioning the future, the burgeoning prominence of TIR as a measurable criterion is anticipated to persistently mirror the evolving methodology within the domain of diabetes management. Progressions in technological innovations coupled with an increasingly profound comprehension of the intricacies inherent in diabetes are poised to propel these transformations forward, culminating in a sustained emphasis on ameliorating long-term prognosis and enhancing the quality of life for individuals afflicted with diabetes.
Although Continuous Glucose Monitoring (CGM) can furnish real-time insights into blood glucose levels and trends, alongside retrospective analyses of glycemic regulation patterns and glycemic metrics over specific temporal intervals (4, 39), their assimilation into clinical practice falls short of reaching optimal levels (40). The suboptimal adoption can be attributed, in significant part, to the dearth of software possessing the capacity for relatively straightforward and standardized statistical and graphical depiction, as well as interpretation, of glycemic data, thereby engendering uncertainty and reluctance among clinicians towards integrating CGM into their professional milieu (41, 42). Consequently, to surmount these obstacles and harness the full potential of continuous glucose monitoring (CGM) data within clinical settings, a method christened as the “ambulatory glucose profile” (AGP) was devised. The AGP is a tool utilized for assessing short-term glycemic variability indices in diabetic patients. By analyzing CGM data, it generates charts depicting median, interquartile range (IQR), and other statistical values, thereby providing a comprehensive evaluation of intra-day and inter-day glycemic fluctuations for patients (43, 44). A methodical examination of AGP reports proves to be a valuable and pragmatic approach, enabling real-time and comprehensive assessment of glycemic control and the effectiveness of any therapeutic modifications (45, 46). Through meticulous scrutiny of AGP charts, clinical practitioners can attain enhanced comprehension of patients’ glycemic patterns, identify potential issues, and discern opportunities for refining treatment regimens. Thorough AGP analysis aids in pinpointing pivotal factors for achieving optimal glycemic control, thereby furnishing robust support for formulating appropriate therapeutic adjustments and further integrating CGM data into routine clinical practice (47, 48). With the introduction of the AGP approach, clinicians are better equipped to expound upon and communicate glycemic data, collaboratively establish personalized treatment objectives with patients, and monitor their progress throughout the course of treatment. This endeavor fosters closer doctor-patient relationships, heightens patients’ awareness of glycemic control, and ultimately augments the efficacy of diabetes management. In addition, there are a number of Software Packages and Tools that support comprehensive analysis of CGM data (49).
Ultimately, through a bibliometric analysis of this research domain, we can discern with clarity that the focal point of clinical practice research transcends the mere analysis of various metrics and has surpassed conventional data analysis. As depicted by the keyword clustering analysis in Figure 8, investigations are progressively expanding into the application of cutting-edge technologies such as artificial pancreas, machine learning, and artificial intelligence. These studies not only furnish diabetic patients with more advanced therapeutic modalities but also usher in novel possibilities for technological innovation and advancement within the realm of medicine. Looking ahead, we can anticipate witnessing further breakthroughs in these domains, heralding a positive impact on the well-being and quality of life for individuals afflicted by diabetes.
5 Conclusion
The current clinical practice of continuous glucose monitoring (CGM) holds great promise. Since its introduction in the United States in 1999 (50), the accuracy of CGM systems has steadily improved, facilitating better daily management of diabetes. The present state of glycemic management in diabetic patients is deemed precarious, as it falls short of the established standards set forth by the World Health Organization. Nevertheless, the burgeoning utilization of continuous glucose monitoring (CGM) has garnered significant interest and is anticipated to be comprehensively explored in the realm of research.
6 Limitations
The present study exhibits certain potential limitations that should be acknowledged. Firstly, the pertinent articles were exclusively obtained from a solitary database, WOSCC, which may have resulted in a biased sample, particularly in comparison to other databases, such as Scopus and PubMed. Secondly, some studies that could have provided valuable insights to the study are ongoing and hence not yet included. Thirdly, researcher bias is a possibility, as the screening process for literature necessitates the artificial exclusion of articles that do not bear relevance to the study. Fourthly, the study solely focused on the clinical practice of CGM in diabetes and did not encompass the technological advancements of CGM sensors, which may have caused the omission of certain potentially beneficial articles.
Data availability statement
The raw data supporting the conclusions of this article will be made available by the authors, without undue reservation.
Author contributions
LK, BD, and MG contributed to conception and design of the study. LY and ZX organized the database. BD performed the statistical analysis. LK and BD wrote the first draft of the manuscript. MG, MC, XW, MZ, HT, and QW wrote sections of the manuscript. All authors contributed to the article and approved the submitted version.
Funding
This study was supported by the 2022 Clinical Medicine Research Center for Geriatric Diseases Open Topics (Grant numbers [2022 LHFSSYB-10] and [2022 LHNBSYB-05]).
Acknowledgments
The authors would like to thank any researcher who contributed to this study.
Conflict of interest
The authors declare that the research was conducted in the absence of any commercial or financial relationships that could be construed as a potential conflict of interest.
Publisher’s note
All claims expressed in this article are solely those of the authors and do not necessarily represent those of their affiliated organizations, or those of the publisher, the editors and the reviewers. Any product that may be evaluated in this article, or claim that may be made by its manufacturer, is not guaranteed or endorsed by the publisher.
References
1. Ogle GD, James S, Dabelea D, Pihoker C, Svennson J, Maniam J, et al. Global estimates of incidence of type 1 diabetes in children and adolescents: Results from the International Diabetes Federation Atlas, 10th edition. Diabetes Res Clin Pract (2022) 183:109083. doi: 10.1016/j.diabres.2021.109083
2. Ogurtsova K, Da RFJ, Huang Y, Linnenkamp U, Guariguata L, Cho NH, et al. IDF Diabetes Atlas: Global estimates for the prevalence of diabetes for 2015 and 2040. Diabetes Res Clin Pract (2017) 128:40–50. doi: 10.1016/j.diabres.2017.03.024
3. Ajjan RA. How can we realize the clinical benefits of continuous glucose monitoring? Diabetes Technol Ther (2017) 19:S27–36. doi: 10.1089/dia.2017.0021
4. Peters AL. Integration of diabetes technology in clinical practice. Endocrinol Metab Clin North Am (2020) 49:69–77. doi: 10.1016/j.ecl.2019.10.002
5. Garg SK, Akturk HK. Flash glucose monitoring: the future is here. Diabetes Technol Ther (2017) 19:1–03. doi: 10.1089/dia.2017.0098
6. Riveline JP, Schaepelynck P, Chaillous L, Renard E, Sola-Gazagnes A, Penfornis A, et al. Assessment of patient-led or physician-driven continuous glucose monitoring in patients with poorly controlled type 1 diabetes using basal-bolus insulin regimens: a 1-year multicenter study. Diabetes Care (2012) 35:965–71. doi: 10.2337/dc11-2021
7. Battelino T, Alexander CM, Amiel SA, Arreaza-Rubin G, Beck RW, Bergenstal RM, et al. Continuous glucose monitoring and metrics for clinical trials: an international consensus statement. Lancet Diabetes Endocrinol (2023) 11:42–57. doi: 10.1016/S2213-8587(22)00319-9
8. Hirsch IB, Miller E. Integrating continuous glucose monitoring into clinical practices and patients' lives. Diabetes Technol Ther (2021) 23:S72–80. doi: 10.1089/dia.2021.0233
9. Aleppo G, Webb K. Continuous glucose monitoring integration in clinical practice: A stepped guide to data review and interpretation. J Diabetes Sci Technol (2019) 13:664–73. doi: 10.1177/1932296818813581
10. Guler AT, Waaijer CJ, Palmblad M. Scientific workflows for bibliometrics. Scientometrics (2016) 107:385–98. doi: 10.1007/s11192-016-1885-6
11. Wu H, Cheng K, Guo Q, Yang W, Tong L, Wang Y, et al. Mapping knowledge structure and themes trends of osteoporosis in rheumatoid arthritis: a bibliometric analysis. Front Med (Lausanne) (2021) 8:787228. doi: 10.3389/fmed.2021.787228
12. Cheng K, Guo Q, Yang W, Wang Y, Sun Z, Wu H. Mapping knowledge landscapes and emerging trends of the links between bone metabolism and diabetes mellitus: a bibliometric analysis from 2000 to 2021. Front Public Health (2022) 10:918483. doi: 10.3389/fpubh.2022.918483
13. Wu W, Xie Y, Liu X, Gu Y, Zhang Y, Tu X, et al. Analysis of scientific collaboration networks among authors, institutions, and countries studying adolescent myopia prevention and control: a review article. Iran J Public Health (2019) 48:621–31. doi: 10.18502/ijph.v48i4.983
14. Battelino T, Danne T, Bergenstal RM, Amiel SA, Beck R, Biester T, et al. Clinical targets for continuous glucose monitoring data interpretation: recommendations from the international consensus on time in range. Diabetes Care (2019) 42:1593–603. doi: 10.2337/dci19-0028
15. Danne T, Nimri R, Battelino T, Bergenstal RM, Close KL, DeVries JH, et al. International consensus on use of continuous glucose monitoring. Diabetes Care (2017) 40:1631–40. doi: 10.2337/dc17-1600
16. Beck RW, Riddlesworth T, Ruedy K, Ahmann A, Bergenstal R, Haller S, et al. Effect of continuous glucose monitoring on glycemic control in adults with type 1 diabetes using insulin injections: the DIAMOND randomized clinical trial. Jama (2017) 317:371–78. doi: 10.1001/jama.2016.19975
17. Foster NC, Beck RW, Miller KM, Clements MA, Rickels MR, DiMeglio LA, et al. State of type 1 diabetes management and outcomes from the T1D exchange in 2016-2018. Diabetes Technol Ther (2019) 21:66–72. doi: 10.1089/dia.2018.0384
18. Beck RW, Bergenstal RM, Riddlesworth TD, Kollman C, Li Z, Brown AS, et al. Validation of time in range as an outcome measure for diabetes clinical trials. Diabetes Care (2019) 42:400–05. doi: 10.2337/dc18-1444
19. Beck RW, Riddlesworth TD, Ruedy K, Ahmann A, Haller S, Kruger D, et al. Continuous glucose monitoring versus usual care in patients with type 2 diabetes receiving multiple daily insulin injections: a randomized trial. Ann Intern Med (2017) 167:365–74. doi: 10.7326/M16-2855
20. Miller KM, Foster NC, Beck RW, Bergenstal RM, DuBose SN, DiMeglio LA, et al. Current state of type 1 diabetes treatment in the U.S.: updated data from the T1D Exchange clinic registry. Diabetes Care (2015) 38:971–78. doi: 10.2337/dc15-0078
21. Swaich H, Advani A. Sustained improvement in glycemic control in emerging adults with type 1 diabetes 2 years after the start of the COVID-19 pandemic. Diabetes Ther (2023) 14:153–65. doi: 10.1007/s13300-022-01346-5
22. Romeo GR, Shaikh YA, Middelbeek R. Post-graduate medical education in the time of COVID-19: Not a remotely simple task. Front Endocrinol (Lausanne) (2022) 13:980505. doi: 10.3389/fendo.2022.980505
23. Kodani N, Ohsugi M. The patient-centered diabetes management during the COVID-19 pandemic. Glob Health Med (2022) 4:210–15. doi: 10.35772/ghm.2022.01031
24. Zhang W, Zhang S, Dong C, Guo S, Jia W, Jiang Y, et al. A bibliometric analysis of RNA methylation in diabetes mellitus and its complications from 2002 to 2022. Front Endocrinol (Lausanne) (2022) 13:997034. doi: 10.3389/fendo.2022.997034
25. Zhou Z, Sun B, Huang S, Zhu C, Bian M. Glycemic variability: adverse clinical outcomes and how to improve it? Cardiovasc Diabetol (2020) 19:102. doi: 10.1186/s12933-020-01085-6
26. Psoma O, Makris M, Tselepis A, Tsimihodimos V. Short-term glycemic variability and its association with macrovascular and microvascular complications in patients with diabetes. J Diabetes Sci Technol (2022), 550756024. doi: 10.1177/19322968221146808
27. Rodbard D. Interpretation of continuous glucose monitoring data: glycemic variability and quality of glycemic control. Diabetes Technol Ther (2009) 11 Suppl 1:S55–67. doi: 10.1089/dia.2008.0132
28. Penckofer S, Quinn L, Byrn M, Ferrans C, Miller M, Strange P. Does glycemic variability impact mood and quality of life? Diabetes Technol Ther (2012) 14:303–10. doi: 10.1089/dia.2011.0191
29. Ceriello A. The post-prandial state and cardiovascular disease: relevance to diabetes mellitus. Diabetes Metab Res Rev (2000) 16:125–32. doi: 10.1002/(sici)1520-7560(200003/04)16:2<125::aid-dmrr90>3.0.co;2-4
30. Chen Y, Jia T, Yan X, Dai L. Blood glucose fluctuations in patients with coronary heart disease and diabetes mellitus correlates with heart rate variability: A retrospective analysis of 210 cases. Niger J Clin Pract (2020) 23:1194–200. doi: 10.4103/njcp.njcp_529_19
31. Service FJ, Molnar GD, Rosevear JW, Ackerman E, Gatewood LC, Taylor WF. Mean amplitude of glycemic excursions, a measure of diabetic instability. Diabetes (1970) 19:644–55. doi: 10.2337/diab.19.9.644
32. Kovatchev B. Glycemic variability: risk factors, assessment, and control. J Diabetes Sci Technol (2019) 13:627–35. doi: 10.1177/1932296819826111
33. Wojcicki JM. "J"-index. A new proposition of the assessment of current glucose control in diabetic patients. Horm Metab Res (1995) 27:41–2. doi: 10.1055/s-2007-979906
34. Kovatchev BP, Cox DJ, Gonder-Frederick LA, Young-Hyman D, Schlundt D, Clarke W. Assessment of risk for severe hypoglycemia among adults with IDDM: validation of the low blood glucose index. Diabetes Care (1998) 21:1870–75. doi: 10.2337/diacare.21.11.1870
35. Kovatchev BP, Otto E, Cox D, Gonder-Frederick L, Clarke W. Evaluation of a new measure of blood glucose variability in diabetes. Diabetes Care (2006) 29:2433–38. doi: 10.2337/dc06-1085
36. Vigersky RA, McMahon C. The relationship of hemoglobin A1C to time-in-range in patients with diabetes. Diabetes Technol Ther (2019) 21:81–5. doi: 10.1089/dia.2018.0310
37. Yapanis M, James S, Craig ME, O'Neal D, Ekinci EI. Complications of diabetes and metrics of glycemic management derived from continuous glucose monitoring. J Clin Endocrinol Metab (2022) 107:e2221–36. doi: 10.1210/clinem/dgac034
38. Bergenstal RM, Ahmann AJ, Bailey T, Beck RW, Bissen J, Buckingham B, et al. Recommendations for standardizing glucose reporting and analysis to optimize clinical decision making in diabetes: the ambulatory glucose profile. J Diabetes Sci Technol (2013) 7:562–78. doi: 10.1177/193229681300700234
39. Beck RW, Tamborlane WV, Bergenstal RM, Miller KM, DuBose SN, Hall CA. The T1D Exchange clinic registry. J Clin Endocrinol Metab (2012) 97:4383–89. doi: 10.1210/jc.2012-1561
40. Benhalima K, Mathieu C. The role of blood glucose monitoring in non-insulin treated type 2 diabetes: what is the evidence? Prim Care Diabetes (2012) 6:179–85. doi: 10.1016/j.pcd.2012.05.001
41. Beck RW, Bergenstal RM. Beyond A1C-standardization of continuous glucose monitoring reporting: why it is needed and how it continues to evolve. Diabetes Spectr (2021) 34:102–08. doi: 10.2337/ds20-0090
42. Carlson AL, Mullen DM, Bergenstal RM. Clinical use of continuous glucose monitoring in adults with type 2 diabetes. Diabetes Technol Ther (2017) 19:S4–11. doi: 10.1089/dia.2017.0024
43. Peters AL. The evidence base for continuous glucose monitoring. In: Role of continuous glucose monitoring in diabetes treatment. Arlington (VA): American Diabetes Association (2018) 3–07. doi: 10.2337/db20181-3
44. Rodbard D. Clinical interpretation of indices of quality of glycemic control and glycemic variability. Postgrad Med (2011) 123:107–18. doi: 10.3810/pgm.2011.07.2310
45. Forlenza GP, Pyle LL, Maahs DM, Dunn TC. Ambulatory glucose profile analysis of the juvenile diabetes research foundation continuous glucose monitoring dataset-Applications to the pediatric diabetes population. Pediatr Diabetes (2017) 18:622–28. doi: 10.1111/pedi.12474
46. Czupryniak L, Dzida G, Fichna P, Jarosz-Chobot P, Gumprecht J, Klupa T, et al. Ambulatory glucose profile (AGP) report in daily care of patients with diabetes: practical tips and recommendations. Diabetes Ther (2022) 13:811–21. doi: 10.1007/s13300-022-01229-9
47. Bergenstal RM, Ahmann AJ, Bailey T, Beck RW, Bissen J, Buckingham B, et al. Recommendations for standardizing glucose reporting and analysis to optimize clinical decision making in diabetes: the Ambulatory Glucose Profile (AGP). Diabetes Technol Ther (2013) 15:198–211. doi: 10.1089/dia.2013.0051
48. Piersanti A, Giurato F, Gobl C, Burattini L, Tura A, Morettini M. Software packages and tools for the analysis of continuous glucose monitoring data. Diabetes Technol Ther (2023) 25:69–85. doi: 10.1089/dia.2022.0237
49. Clarke SF, Foster JR. A history of blood glucose meters and their role in self-monitoring of diabetes mellitus. Br J BioMed Sci (2012) 69:83–93. doi: 10.1080/09674845.2012.12002443
Keywords: diabetes mellitus, continuous glucose monitoring, bibliometric analysis, Citespace, VOSviewer
Citation: Kong L, Deng B, Guo M, Chen M, Wang X, Zhang M, Tang H, Wang Q, Yang L and Xiong Z (2023) A systematic bibliometric analysis on the clinical practice of CGM in diabetes mellitus from 2012 to 2022. Front. Endocrinol. 14:1229494. doi: 10.3389/fendo.2023.1229494
Received: 26 May 2023; Accepted: 06 September 2023;
Published: 22 September 2023.
Edited by:
Maurizio Delvecchio, Giovanni XXIII Children’s Hospital, ItalyReviewed by:
Micaela Morettini, Marche Polytechnic University, ItalyHongwei Jiang, The First Affiliated Hospital of Henan University of Science and Technology, China
Pranay Goel, Indian Institute of Science Education and Research, Pune, India
Copyright © 2023 Kong, Deng, Guo, Chen, Wang, Zhang, Tang, Wang, Yang and Xiong. This is an open-access article distributed under the terms of the Creative Commons Attribution License (CC BY). The use, distribution or reproduction in other forums is permitted, provided the original author(s) and the copyright owner(s) are credited and that the original publication in this journal is cited, in accordance with accepted academic practice. No use, distribution or reproduction is permitted which does not comply with these terms.
*Correspondence: Zhenzhen Xiong, eHp6NjIzMDg2MzFAMTYzLmNvbQ==; Liwei Yang, eWFuZ2xsd2VpQDE2My5jb20=
†These authors have contributed equally to this work and share first authorship