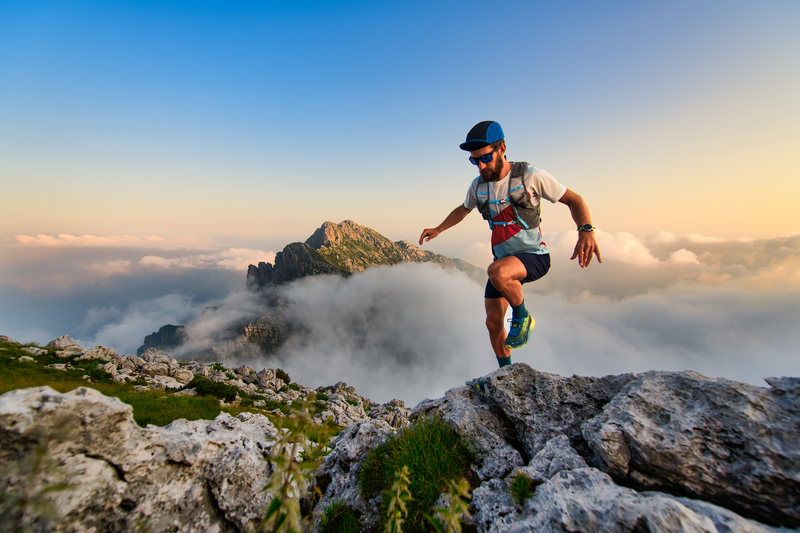
94% of researchers rate our articles as excellent or good
Learn more about the work of our research integrity team to safeguard the quality of each article we publish.
Find out more
ORIGINAL RESEARCH article
Front. Endocrinol. , 12 October 2023
Sec. Gut Endocrinology
Volume 14 - 2023 | https://doi.org/10.3389/fendo.2023.1225742
This article is part of the Research Topic Gut-Brain-Liver Axis at a Glance: the Role of the Gut Microbiota View all 6 articles
Background: The gut-liver axis indicates a close relationship between the gastrointestinal microbiome (GM) and primary biliary cholangitis (PBC). However, the causality of this relationship remains unknown. This study investigates the causal relationship between the GM and PBC using a bidirectional, two-sample Mendelian randomization (MR) analysis.
Methods: Genome-wide association data for GM and PBC were obtained from public databases. The inverse-variance weighted method was the primary method used for MR analysis. Sensitivity analyses were conducted to assess the stability of the MR results. A reverse MR analysis was performed to investigate the possibility of reverse causality.
Results: Three bacterial taxa were found to be causally related to PBC. Class Coriobacteriia (odds ratio (OR) = 2.18, 95% confidence interval (CI): 1.295-3.661, P< 0.05) and order Coriobacteriales (OR = 2.18, 95% CI: 1.295-3.661, P<0.05) were associated with a higher risk of PBC. Class Deltaproteobacteria (OR = 0.52, 95% CI: 0.362–0.742, P< 0.05) had a protective effect on PBC. There was no evidence of reverse causality between PBC and the identified bacterial taxa.
Conclusion: Previously unrecognized taxa that may be involved in the pathogenesis of PBC were identified in this study, confirming the causality between the GM and PBC. These results provide novel microbial targets for the prevention and treatment of PBC.
Primary biliary cholangitis (PBC) is a type of autoimmune liver disease with the clinical features of a high titer of anti-mitochondrial antibody (AMA) in the serum, elevated biliary enzymes, and specific bile duct pathology (1). Anatomically, there is bidirectional crosstalk between the intestine and liver, suggesting that various intestinal-derived products (such as nutrients, bile acids, and bacterial metabolites) are transported to the liver through the portal vein and that the liver secretes bile and antibodies into the intestine. This bidirectional relationship is termed the gut-liver axis, which is the theoretical basis for the relationships between diseases and the gastrointestinal microbiome (GM) (2).
Gastrointestinal barrier dysfunction and alterations in the gut microbial composition are commonly observed in patients with chronic liver diseases. Substances produced by the GM, such as bacterial components and microbial metabolites, accumulate in the liver through the gut-liver axis (3, 4). Specifically, small molecular motifs derived from the GM, such as lipopolysaccharide, are detected by innate immune receptors such as Toll-like receptors. This recognition triggers innate immune responses that ultimately lead to changes in the liver immune microenvironment, resulting in chronic inflammation and, in some cases, progression to hepatocellular carcinoma (5, 6). Microbial metabolites, including secondary bile acids, short-chain fatty acids, and ethanol, are produced through fermentation of intestinal contents by the GM (7). These metabolites have various effects on the liver, including direct toxic effects, pro-inflammatory or anti-inflammatory immune responses, and the maintenance of intestinal epithelial cell stability via the gut-liver axis (8–11).
Changes to the GM are observed in patients with PBC. Compared with healthy controls, the fecal microbial α-diversity is decreased in patients with PBC (12, 13). Several studies have reported depletion of potential probiotics and enrichment of opportunistic pathogenic bacteria within the GM of patients with PBC (12, 14, 15). Disturbance of the GM is a pivotal factor in the progression of PBC. However, previous studies report both consistent and conflicting results as they are case-control observational studies. Although these studies controlled for the effects of age and sex as much as possible, the GM is susceptible to environmental, dietary pattern, and lifestyle changes. The influence of confounding factors (16) renders these factors difficult to control. In addition, due to the bidirectional relationship of the gut-liver axis, it is unclear whether an altered GM triggers PBC or is a reflection of disease status (17).
Mendelian randomization (MR) is a new technique used to explore causal associations using genetics. Genetic variants are used to construct instrumental variables (IVs) representing exposures, which are then used to estimate the causal association between exposures and an outcome (18). Due to the random allocation of genotypes from parents to offspring, the association between genetic variants and outcomes remains unaffected by common confounding factors, establishing reasonable causality (19).
In this study, two-sample MR analyses were conducted to assess the potential causal relationships between bacterial taxa (used as exposures) and PBC (used as the outcome) using genome-wide association study (GWAS) summary statistics from the MiBioGen and GWAS Catalog public databases. The reverse causal relationships between each identified bacterial taxon and PBC were also investigated.
A two-sample MR analysis satisfies three assumptions (20): the IVs chosen from the datasets are related to the exposure; there is no association between the IVs and confounders of the exposure-outcome relationship; and the IVs are linked to the outcome through exposures rather than any other way (Figure 1). Single-nucleotide polymorphisms (SNPs) of each bacterial taxon were screened using the MiBioGen database to be used as IVs (21, 22). According to the previous publication (22), once the quality control-filtered merged reads are processed, all cohorts can use the standardized 16S processing pipeline available at the GitHub repository (23). The cohorts used in this study are detailed in the Supplementary Materials of the previous publication (22). The overall proportion of proton pump inhibitor and antibiotic usage was less than 10% in this study. Most of the fecal samples were gender- and age-matched, and sample quality control, such as removing ethnic outliers and sex mismatches, was performed prior to the GWAS analysis. Outcome data were obtained from the latest genome-wide meta-analysis of PBC (24). Each data source included in the analyses is detailed in Table 1.
Figure 1 Three assumptions of two-sample Mendelian randomization (MR) analysis and the workflow of this study. Concisely, each bacterial taxon (at phylum, class, order, family, and genus) served as an exposure, and PBC served as the outcome. Quality control was taken to ensure that the selected IVs were reliable and accurate in determining a causal link between GM and PBC.
SNPs with a less stringent cutoff of P < 1×10−5 were considered significantly related to GM and chosen as the candidate IVs. This strategy increased the number of SNPs available for subsequent analyses (25–27). The linkage disequilibrium (LD) between the candidate IVs were calculated using the 1000 Genomes Project European sample data as the reference panel (LD correlation coefficient set to R2< 0.001 and clumping window size = 10,000 kb). Then, the PhenoScanner V2 (28, 29) was used to search the candidate IVs for confounders to avoid horizontal pleiotropy. IVs that correlated with risk factors for PBC were excluded. The F-statistic was used to identify any IV bias, and IVs with an F-statistic< 10 were eliminated (30, 31) by applying formula used in a previous study (Appendices Equations A, B) (31, 32). Last, the exposure and outcome of the SNPs were harmonized to confirm that the effect alleles of the SNPs on the exposure matched with the identical effect alleles on the outcome. Unmatched SNPs were removed from the analyses. Palindromic and ambiguous SNPs were inferred during the harmonization process and also removed.
MR-PRESSO (NbDistribution = 10000) and MR-Egger regression tests were used to detect potential horizontal pleiotropy effects. The MR-PRESSO outlier test assessed the pleiotropic significance of individual SNPs, whereas the MR-PRESSO global test provided an overall p value for horizontal pleiotropy. The SNPs were sorted based on their MR-PRESSO outlier test p values in ascending order and eliminated individually. Each time an SNP was removed, the MR-PRESSO global test was performed for the remaining SNPs. This procedure was repeated until the global test p value was no longer statistically significant (P > 0.05). All pleiotropic SNPs were removed prior to the MR analysis.
MR results based on fewer than three shared SNPs were excluded. The common methods used for causal inference were the inverse-variance weighted (IVW) method (the main approach for causal detection in two-sample MR analysis without horizontal pleiotropy (33)), weighted median (WM) method (34), MR-Egger regression method (20), simple mode (35), and weighted mode (36). The IVW method is complimented by the other methods, which expands the range of confidence intervals (37). A previously-reported multiple testing significance threshold at each feature level (phylum, class, order, family, and genus), defined as P< 0.05/n (where n is the effective number of independent bacterial taxa at the corresponding taxonomic level), was used (38). Sensitivity analyses were performed to assess the robustness of the findings. A leave-one-out analysis was conducted to determine if any single SNP drove the significant results (39).
The heterogeneity of the IVs was measured using Cochran’s IVW Q statistics. A Q value higher than the number of instruments minus one suggests the presence of invalid instruments and heterogeneity. A Q statistic p value< 0.05 indicates heterogeneity (40, 41).
A reverse MR analysis was conducted to investigate the causal influence of PBC on each identified bacterial taxon that was identified as significant in the previous analyses. PBC was used as the exposure (the p value for SNPs significantly related to PBC was set to 5×10–8), and each identified causal bacterial taxon was set as the outcome. The reverse MR analysis was conducted using the same steps as the quality control of the IVs, MR analysis, and sensitivity analysis.
All statistical analyses were performed using R software (42) (version 4.1.3). The R packages used for the statistical analysis were TwoSampleMR (35, 39) (version 0.5.6) and MR-PRESSO (43) (version 1.0).
A total of 14570 SNPs among 211 taxa were identified, including 937, 1583,1642, 2567, and 7841 at the phylum, class, order, family, and genus levels, respectively (Table S1). After clumping, 2212 SNPs remained as candidate IVs. The PhenoScanner V2 identified only one candidate IV (Table S2) that was associated with smoking, a risk factor for PBC (1). The F-statistics of the IVs were all > 10 (Table S3), suggesting no indication of weak instrument bias. A total of 711 IVs remained after harmonizing the exposure and outcome data (Table S4). Three pleiotropic SNPs were removed via the MR-PRESSO outlier test (Table S5).
The MR results were retained if they were based on three or more shared SNPs. A total of 149 independent bacterial taxa remained after the MR analysis, including 88 at the genus level (P threshold of 5.68×10−4), 27 at the family level (P threshold of 1.85×10-3), 14 at the order level (P threshold of 3.57×10-3), 12 at the class level (P threshold of 4.17×10-3), and eight at the phylum level (P threshold of 6.25×10-3) (Table S6).
Causal relationships between PBC and three bacterial taxa, class Coriobacteriia, order Coriobacteriales, and class Deltaproteobacteria, were identified using the IVW and WM methods (P< 0.05) (Table 2, Figures 2, 3). The p values of the IVW method were inferior to the corresponding modified thresholds. The results of the IVW method indicate that the class Coriobacteriia (odds ratio (OR) = 2.18, 95% confidence interval (CI): 1.295-3.661, P = 3.33×10-3) and order Coriobacteriales (OR = 2.18, 95% CI: 1.295-3.661, P = 3.33×10-3) are associated with a higher risk of PBC and that the class Deltaproteobacteria (OR = 0.52, 95% CI: 0.362–0.742, P = 3.25×10−4) has a protective effect on PBC. The MR-Egger regression, simple mode, and weighted mode methods yielded similar causal estimates for the magnitude and direction.
Figure 2 Scatter plots for the causal association between bacterial taxa and primary biliary cholangitis. Single nucleotide polymorphisms (SNPs) were used to assess the impact of each bacterial taxon on primary biliary cholangitis (PBC) by five MR methods (A–C). The dots represent the effect size (β) of each SNP on each bacterial taxon (x-axis) and PBC (y-axis), and the grey crosses represent the standard errors. Regression slopes show the estimated causal effect of each bacterial taxon on PBC. The light blue, dark blue, light green, dark green, and red regression lines represent the inverse variance weighted method, MR-Egger regression, simple mode, weighted median method, and weighted mode, respectively.
Figure 3 The circular heat map of two-sample Mendelian randomization (MR) analysis. The first track represented the p-value of the MR analysis. From external to internal, it represented different methods, including the inverse variance weighted method, MR-Egger regression, Simple mode, Weighted median, and weighted mode method. The color gradient tended towards red to indicate smaller p-values. The block marked with ‘black stars’ signified that the taxon reached the modified significance threshold at a specific taxonomy. The second track represented the β value. Red blocks indicated harmful factors for PBC, with darker shades indicating a higher risk of illness. Green blocks represented protective factors against PBC, with darker shades indicating a lower risk of illness.
The MR-Egger intercept indicated no horizontal pleiotropy in the identified taxa (P > 0.05). The MR-PRESSO analysis revealed no outliers among the IVs. Additionally, the Cochrane Q statistics indicated no noticeable heterogeneity (P > 0.05) (Table S7). The leave-one-out analysis demonstrated that no individual SNP significantly affected the correlation between each identified bacterial taxon and PBC (Figure 4).
Figure 4 Leave-one-out analysis for identified bacterial taxa on primary biliary cholangitis. The sensitivity of the causal effect of different single nucleotide polymorphisms (SNPs) of each taxon on primary biliary cholangitis was analyzed through leave-one-out analysis in (A–C). The error bar depicts the 95% confidence interval using the inverse variance weighted method.
No reverse causal association between PBC and the identified bacteria were identified (Table S8).
Researchers have reported that the GM contributes to PBC via molecular mimicry, translocation of gut bacteria to the liver through the damaged intestinal epithelium, movement of immune cells from the intestine to the liver, and abnormal bile acid metabolism (44, 45). Molecular mimicry is a common mechanism by which foreign substances, such as the GM, cause autoimmunity in the body. This occurs when proteins or peptides from the gut microbiota resemble self-peptides, which may activate T or B cells that attack host cells in vulnerable individuals (46). AMA is an autoantibody specific for PBC that targets lipoic acid on 2-oxo-acid dehydrogenase complexes within the inner mitochondrial membrane. Increases in the number of autoreactive clusters of CD4+or CD8+ pyruvate dehydrogenase complex (PDC-E2)-specific T cells are observed in the liver (1). There is growing evidence that microbial mimics can induce AMA expression. Bacterial sequences from Escherichia coli and Sphingomonas can react with AMA in the serum of patients with PBC (47, 48), and their ability to induce autoimmune cholangitis has been verified in vivo (49, 50). No causal relationship between Escherichia coli or Sphingomonas and PBC was identified in this study, which may be due to the limited number of taxa in the MiBioGen database; therefore, the corresponding IVs could not be screened for the MR analyses.
In this study, the class Coriobacteriia and its lower taxonomic rank order Coriobacteriales of the phylum Actinobacteria (51) were associated with a higher risk of developing PBC. Members of this category are anaerobic organisms that survive in various ecological environments and do not produce spores. They may either be strict or facultative in their anaerobic requirements (52–55). Limited research regarding their influence on human diseases and the relationships between the class Coriobacteriia, order Coriobacteriales, and PBC has been conducted. Yi et al. reported that long-term exposure to nitrogen dioxide significantly increases gamma-glutamyl transpeptidase and glutamic-pyruvic transaminase levels in patients with schizophrenia and that Coriobacteriales intestinal bacteria mediates this effect by 13.98% and 49.56%, respectively (56). The results of this previous study suggest that Coriobacteriales may be an intermediary in the mechanism by which smoking or environmental exposure initiates PBC (1). Another study reported that Coriobacteriaceae and Coriobacteriales are significantly enriched in the urethral secretions of patients with chronic prostatitis (57), a specific type of urinary tract infection. In addition, several extensive case-control cohort studies have reported that urinary tract infections are related to PBC (1), which is supported by the genetic results of the current study. Approximately 75-95% of patients with PBC suffer from hyperlipidemia due to various complex procedures associated with biliary cholestasis (58, 59). Coriobacteriia were elevated in the guts of females with low high-density lipoprotein cholesterol (60). Interestingly, genera in the Coriobacteriia class were lower in patients with familial hypercholesterolemia who had used statins for more than 12 months than in healthy control patients (61). Patients with PBC and metabolic syndrome are at a higher risk of cardiovascular events (62). However, further experimental verification is required to determine whether Coriobacteria can be targeted to improve the lipid metabolism abnormalities in patients with PBC.
In this study, the class Deltaproteobacteria was found to have a defensive role in preventing PBC. Deltaproteobacteria is a gram-negative class of Proteobacteria involved in the carbon and sulfur cycles (63). The lower taxonomic levels of the class Deltaproteobacteria, order Desulfovibrionales, and family Desulfovibrionaceae tended to have a protective effect on PBC, though the threshold of the modified p-value was not met in this study (Figure 3, Table S6). In contrast to the current findings, previous studies suggested that Deltaproteobacteria and its descendants are potentially pathogenic gut bacteria (64–70). Desulfovibrionaceae is capable of producing hydrogen sulfide (H2S) (71). Excessive H2S in the focal intestinal tract may reduce disulfide bonds in the mucous layer, breaking down the mucous barrier and exposing epithelial cells to bacteria and toxins, which may lead to intestinal inflammation (72). In contrast, low levels of endogenous or exogenous H2S directly stabilize the mucus layers, preventing microbial biofilms from attaching to the epithelium, which stops the release of harmful pathogenic microorganisms and assists in resolving tissue damage and inflammation (72). In addition, metabolic H2S has been reported to improve insulin resistance in mice with non-alcoholic fatty liver disease via the AKT signaling pathway (73), suggesting that an overabundance of Deltaproteobacteria and their descendants colonizing the human gut may disrupt the balance of the local microbiota and become pathogenic. However, the safety zone for the abundance of these taxa is not predictable. Therefore, Deltaproteobacteria and their descendants may be protective or harmful. The necessary equilibrium of these taxa in the human gut requires further investigation.
Organisms that are causally related to PBC (12, 14, 74), such as Streptococcus, Enterococcus, or Veillonella, were not identified as potential biomarkers in this study. The alterations in the GM associated with PBC may be an effect rather than a cause, indicating that the disease state of PBC (bile stasis and immune dysregulation of the gut-liver axis) affects the composition of the GM.
The efficacies of treatment options for PBC are limited (1, 75). Microbiome modulation therapies, such as the use of antibiotics, supplementation with probiotics, and fecal microbiota transplantation (FMT), have been used to treat liver diseases, including recurrent encephalopathy, non-alcoholic fatty liver disease, liver cancer, and cholestatic liver diseases (76–79). Although no clinical trials have specifically focused on PBC, promising results have been obtained regarding the safety of FMT in primary sclerosing cholangitis (PSC), another cholestatic liver disease. A previous study demonstrated improvements in the alkaline phosphatase levels and enrichment of specific bacterial strains in patients with PSC (80). Therefore, the identification of bacteria that could be protective or potentially harmful against PBC may be a therapeutic intervention for the regulation of the GM.
This study has advantages and limitations. It is the first bi-directional, two-sample MR study to explore the causal relationship between the GM and PBC, with strict conditions for screening the IVs. This study provides genetic evidence regarding the gut-liver axis and identifies three bacterial taxa associated with PBC that have not been previously studied. However, the number of microbiota taxa in the database was limited, resulting in a lack of IVs for the MR analysis. In addition, it is unclear whether there are overlapping samples in the GWAS data of GM and PBC, which may lead to bias. Further experimental and clinical validation is necessary to confirm these findings. Last, the GWAS samples of the MiBioGen database were mainly of European ancestry; therefore, these results are limited to patients of European descent.
In conclusion, this study identified a causal relationships between the GM and PBC. The class Coriobacteriia and order Coriobacteriales may be intermediate factors in inducing PBC and participating in disease progression, whereas Deltaproteobacteria may play a protective role. However, it is essential to note that the findings of this study have limitations, and further validation through experiments and clinical studies is required to confirm these observations.
The original contributions presented in the study are included in the article/Supplementary Material. Further inquiries can be directed to the corresponding author.
Each cohort obtained ethical approval and consent to participate in accordance with their local regulations and institute requirements (mentioned in previous studies). The current study remained within the limits of the ethical committee’s original permission. The studies were conducted in accordance with the local legislation and institutional requirements. The human samples used in this study were acquired from gifted from another research group. Written informed consent for participation was not required from the participants or the participants’ legal guardians/next of kin in accordance with the national legislation and institutional requirements.
XL and XY contributed to the study design. XL performed data collecting, computations, and manuscript writing. XY and XL contributed to the article and approved the submitted version.
This work was supported by Beijing Natural Science Foundation (L222085). The founders had no role in study design, data collection or manuscript preparation.
We wish to express our gratitude to the participants and researchers of the GWAS catalog. We also thank the MiBioGen consortium for providing the GWAS data of intestinal flora. In addition, we want to acknowledge BioRender(www.biorender.com) for their assistance in Figure 1. We would like to thank Editage (www.editage.cn) for English language editing.
The authors declare that the research was conducted in the absence of any commercial or financial relationships that could be construed as a potential conflict of interest.
All claims expressed in this article are solely those of the authors and do not necessarily represent those of their affiliated organizations, or those of the publisher, the editors and the reviewers. Any product that may be evaluated in this article, or claim that may be made by its manufacturer, is not guaranteed or endorsed by the publisher.
The Supplementary Material for this article can be found online at: https://www.frontiersin.org/articles/10.3389/fendo.2023.1225742/full#supplementary-material
1. Lindor KD, Bowlus CL, Boyer J, Levy C, Mayo M. Primary biliary cholangitis: 2018 practice guidance from the american association for the study of liver diseases. Hepatology (2019) 69(1):394–419. doi: 10.1002/hep.30145
2. Pabst O, Hornef MW, Schaap FG, Cerovic V, Clavel T, Bruns T. Gut-liver axis: barriers and functional circuits. Nat Rev Gastroenterol Hepatol (2023) 20(7):447–61. doi: 10.1038/s41575-023-00771-6
3. Di Ciaula A, Baj J, Garruti G, Celano G, De Angelis M, Wang HH, et al. Liver steatosis, gut-liver axis, microbiome and environmental factors. A never-ending bidirectional cross-talk. J Clin Med (2020) 9(8):2648. doi: 10.3390/jcm9082648
4. Gudan A, Jamioł-Milc D, Hawryłkowicz V, Skonieczna-Żydecka K, Stachowska E. The prevalence of small intestinal bacterial overgrowth in patients with non-alcoholic liver diseases: nafld, nash, fibrosis, cirrhosis-a systematic review, meta-analysis and meta-regression. Nutrients (2022) 14(24):5261. doi: 10.3390/nu14245261
5. Thaiss CA, Zmora N, Levy M, Elinav E. The microbiome and innate immunity. Nature (2016) 535(7610):65–74. doi: 10.1038/nature18847
6. Carpino G, Del Ben M, Pastori D, Carnevale R, Baratta F, Overi D, et al. Increased liver localization of lipopolysaccharides in human and experimental nafld. Hepatology (2020) 72(2):470–85. doi: 10.1002/hep.31056
7. Chen J, Thomsen M, Vitetta L. Interaction of gut microbiota with dysregulation of bile acids in the pathogenesis of nonalcoholic fatty liver disease and potential therapeutic implications of probiotics. J Cell Biochem (2019) 120(3):2713–20. doi: 10.1002/jcb.27635
8. Allen K, Jaeschke H, Copple BL. Bile acids induce inflammatory genes in hepatocytes: A novel mechanism of inflammation during obstructive cholestasis. Am J Pathol (2011) 178(1):175–86. doi: 10.1016/j.ajpath.2010.11.026
9. Manley S, Ni HM, Kong B, Apte U, Guo G, Ding WX. Suppression of autophagic flux by bile acids in hepatocytes. Toxicol Sci (2014) 137(2):478–90. doi: 10.1093/toxsci/kft246
10. Zhu L, Baker SS, Gill C, Liu W, Alkhouri R, Baker RD, et al. Characterization of gut microbiomes in nonalcoholic steatohepatitis (Nash) patients: A connection between endogenous alcohol and nash. Hepatology (2013) 57(2):601–9. doi: 10.1002/hep.26093
11. Chen J, Vitetta L. The role of butyrate in attenuating pathobiont-induced hyperinflammation. Immune Netw (2020) 20(2):e15. doi: 10.4110/in.2020.20.e15
12. Tang R, Wei Y, Li Y, Chen W, Chen H, Wang Q, et al. Gut microbial profile is altered in primary biliary cholangitis and partially restored after udca therapy. Gut (2018) 67(3):534–41. doi: 10.1136/gutjnl-2016-313332
13. Liwinski T, Casar C, Ruehlemann MC, Bang C, Sebode M, Hohenester S, et al. A disease-specific decline of the relative abundance of bifidobacterium in patients with autoimmune hepatitis. Aliment Pharmacol Ther (2020) 51(12):1417–28. doi: 10.1111/apt.15754
14. Lv LX, Fang DQ, Shi D, Chen DY, Yan R, Zhu YX, et al. Alterations and correlations of the gut microbiome, metabolism and immunity in patients with primary biliary cirrhosis. Environ Microbiol (2016) 18(7):2272–86. doi: 10.1111/1462-2920.13401
15. Furukawa M, Moriya K, Nakayama J, Inoue T, Momoda R, Kawaratani H, et al. Gut dysbiosis associated with clinical prognosis of patients with primary biliary cholangitis. Hepatol Res (2020) 50(7):840–52. doi: 10.1111/hepr.13509
16. Rinninella E, Raoul P, Cintoni M, Franceschi F, Miggiano GAD, Gasbarrini A, et al. What is the healthy gut microbiota composition? A changing ecosystem across age, environment, diet, and diseases. Microorganisms (2019) 7(1):14. doi: 10.3390/microorganisms7010014
17. Terziroli Beretta-Piccoli B, Mieli-Vergani G, Vergani D, Vierling JM, Adams D, Alpini G, et al. The challenges of primary biliary cholangitis: what is new and what needs to be done. J Autoimmun (2019) 105:102328. doi: 10.1016/j.jaut.2019.102328
18. Greenland S. An introduction to instrumental variables for epidemiologists. Int J Epidemiol (2000) 29(4):722–9. doi: 10.1093/ije/29.4.722
19. Burgess S, Thompson SG. Mendelian Randomization: Methods for Causal Inference Using Genetic Variants. New York: CRC Press (2021).
20. Bowden J, Davey Smith G, Burgess S. Mendelian randomization with invalid instruments: effect estimation and bias detection through egger regression. Int J Epidemiol (2015) 44(2):512–25. doi: 10.1093/ije/dyv080
21. Wang J, Kurilshikov A, Radjabzadeh D, Turpin W, Croitoru K, Bonder MJ, et al. Meta-analysis of human genome-microbiome association studies: the mibiogen consortium initiative. Microbiome (2018) 6(1):101. doi: 10.1186/s40168-018-0479-3
22. Kurilshikov A, Medina-Gomez C, Bacigalupe R, Radjabzadeh D, Wang J, Demirkan A, et al. Large-scale association analyses identify host factors influencing human gut microbiome composition. Nat Genet (2021) 53(2):156–65. doi: 10.1038/s41588-020-00763-1
23. Kurilshikov A. Mibiogen Miqtl Pipeline: Github (2018). Available at: https://github.com/alexa-kur/miQTL_cookbook.
24. Cordell HJ, Fryett JJ, Ueno K, Darlay R, Aiba Y, Hitomi Y, et al. An international genome-wide meta-analysis of primary biliary cholangitis: novel risk loci and candidate drugs. J Hepatol (2021) 75(3):572–81. doi: 10.1016/j.jhep.2021.04.055
25. Sanna S, van Zuydam NR, Mahajan A, Kurilshikov A, Vich Vila A, Võsa U, et al. Causal relationships among the gut microbiome, short-chain fatty acids and metabolic diseases. Nat Genet (2019) 51(4):600–5. doi: 10.1038/s41588-019-0350-x
26. Cao J, Wang N, Luo Y, Ma C, Chen Z, Chenzhao C, et al. A cause–effect relationship between graves’ Disease and the gut microbiome contributes to the thyroid–gut axis: A bidirectional two-sample mendelian randomization study. Front Immunol (2023) 14:977587. doi: 10.3389/fimmu.2023.977587
27. Li P, Wang H, Guo L, Gou X, Chen G, Lin D, et al. Association between gut microbiota and preeclampsia-eclampsia: A two-sample mendelian randomization study. BMC Med (2022) 20(1):443. doi: 10.1186/s12916-022-02657-x
28. Kamat MA, Blackshaw JA, Young R, Surendran P, Burgess S, Danesh J, et al. Phenoscanner V2: an expanded tool for searching human genotype–phenotype associations. Bioinformatics (2019) 35(22):4851–3. doi: 10.1093/bioinformatics/btz469
29. Staley JR, Blackshaw J, Kamat MA, Ellis S, Surendran P, Sun BB, et al. Phenoscanner: A database of human genotype–phenotype associations. Bioinformatics (2016) 32(20):3207–9. doi: 10.1093/bioinformatics/btw373
30. Lawlor DA, Harbord RM, Sterne JA, Timpson N, Davey Smith G. Mendelian randomization: using genes as instruments for making causal inferences in epidemiology. Stat Med (2008) 27(8):1133–63. doi: 10.1002/sim.3034
31. Burgess S, Thompson SG. Avoiding bias from weak instruments in mendelian randomization studies. Int J Epidemiol (2011) 40(3):755–64. doi: 10.1093/ije/dyr036
32. Shim H, Chasman DI, Smith JD, Mora S, Ridker PM, Nickerson DA, et al. A multivariate genome-wide association analysis of 10 ldl subfractions, and their response to statin treatment, in 1868 caucasians. PloS One (2015) 10(4):e0120758. doi: 10.1371/journal.pone.0120758
33. Burgess S, Butterworth A, Thompson SG. Mendelian randomization analysis with multiple genetic variants using summarized data. Genet Epidemiol (2013) 37(7):658–65. doi: 10.1002/gepi.21758
34. Bowden J, Davey Smith G, Haycock PC, Burgess S. Consistent estimation in mendelian randomization with some invalid instruments using a weighted median estimator. Genet Epidemiol (2016) 40(4):304–14. doi: 10.1002/gepi.21965
35. Hemani G, Zheng J, Elsworth B, Wade KH, Haberland V, Baird D, et al. The mr-base platform supports systematic causal inference across the human phenome. Elife (2018) 7:e34408. doi: 10.7554/eLife.34408
36. Hartwig FP, Davey Smith G, Bowden J. Robust inference in summary data mendelian randomization via the zero modal pleiotropy assumption. Int J Epidemiol (2017) 46(6):1985–98. doi: 10.1093/ije/dyx102
37. Slob EAW, Burgess S. A comparison of robust mendelian randomization methods using summary data. Genet Epidemiol (2020) 44(4):313–29. doi: 10.1002/gepi.22295
38. Long Y, Tang L, Zhou Y, Zhao S, Zhu H. Causal relationship between gut microbiota and cancers: A two-sample mendelian randomisation study. BMC Med (2023) 21(1):66. doi: 10.1186/s12916-023-02761-6
39. Hemani G, Tilling K, Davey Smith G. Orienting the causal relationship between imprecisely measured traits using gwas summary data. PloS Genet (2017) 13(11):e1007081. doi: 10.1371/journal.pgen.1007081
40. Greco MF, Minelli C, Sheehan NA, Thompson JR. Detecting pleiotropy in mendelian randomisation studies with summary data and a continuous outcome. Stat Med (2015) 34(21):2926–40. doi: 10.1002/sim.6522
41. Bowden J, Del Greco MF, Minelli C, Zhao Q, Lawlor DA, Sheehan NA, et al. Improving the accuracy of two-sample summary-data mendelian randomization: moving beyond the nome assumption. Int J Epidemiol (2019) 48(3):728–42. doi: 10.1093/ije/dyy258
42. Team RC. R: A Language and Environment for Statistical Computing: R Foundation for Statistical Computing (2018). Available at: https://www.R-project.org/.
43. Verbanck M, Chen C-Y, Neale B, Do R. Detection of widespread horizontal pleiotropy in causal relationships inferred from mendelian randomization between complex traits and diseases. Nat Genet (2018) 50(5):693–8. doi: 10.1038/s41588-018-0099-7
44. Wang R, Tang R, Li B, Ma X, Schnabl B, Tilg H. Gut microbiome, liver immunology, and liver diseases. Cell Mol Immunol (2021) 18(1):4–17. doi: 10.1038/s41423-020-00592-6
45. Li Y, Tang R, Leung PSC, Gershwin ME, Ma X. Bile acids and intestinal microbiota in autoimmune cholestatic liver diseases. Autoimmun Rev (2017) 16(9):885–96. doi: 10.1016/j.autrev.2017.07.002
46. Rojas M, Restrepo-Jiménez P, Monsalve DM, Pacheco Y, Acosta-Ampudia Y, Ramírez-Santana C, et al. Molecular mimicry and autoimmunity. J Autoimmun (2018) 95:100–23. doi: 10.1016/j.jaut.2018.10.012
47. Bogdanos DP, Baum H, Grasso A, Okamoto M, Butler P, Ma Y, et al. Microbial mimics are major targets of crossreactivity with human pyruvate dehydrogenase in primary biliary cirrhosis. J Hepatol (2004) 40(1):31–9. doi: 10.1016/s0168-8278(03)00501-4
48. Selmi C, Balkwill DL, Invernizzi P, Ansari AA, Coppel RL, Podda M, et al. Patients with primary biliary cirrhosis react against a ubiquitous xenobiotic-metabolizing bacterium. Hepatology (2003) 38(5):1250–7. doi: 10.1053/jhep.2003.50446
49. Mattner J, Savage PB, Leung P, Oertelt SS, Wang V, Trivedi O, et al. Liver autoimmunity triggered by microbial activation of natural killer T cells. Cell Host Microbe (2008) 3(5):304–15. doi: 10.1016/j.chom.2008.03.009
50. Wang JJ, Yang GX, Zhang WC, Lu L, Tsuneyama K, Kronenberg M, et al. Escherichia coli infection induces autoimmune cholangitis and anti-mitochondrial antibodies in non-obese diabetic (Nod).B6 (Idd10/idd18) mice. Clin Exp Immunol (2014) 175(2):192–201. doi: 10.1111/cei.12224
51. Ludwig W, Euzéby J, Schumann P, Busse H-J, Trujillo ME, Kämpfer P, et al. Road map of the phylum actinobacteria. In: Bergey’s Manual® of Systematic Bacteriology. New York: Springer New York, NY (2012). p. 1–28.
52. Würdemann D, Tindall BJ, Pukall R, Lünsdorf H, Strömpl C, Namuth T, et al. Gordonibacter pamelaeae gen. Nov., sp. Nov., a new member of the coriobacteriaceae isolated from a patient with crohn’s disease, and reclassification of eggerthella hongkongensis lau et al. 2006 as paraeggerthella hongkongensis gen. Nov., comb. Nov. Int J Syst Evol Microbiol (2009) 59(Pt 6):1405–15. doi: 10.1099/ijs.0.005900-0
53. Stackebrandt E, Zeytun A, Lapidus A, Nolan M, Lucas S, Hammon N, et al. Complete genome sequence of coriobacterium glomerans type strain (Pw2(T)) from the midgut of pyrrhocoris apterus L. (Red soldier bug). Stand Genomic Sci (2013) 8(1):15–25. doi: 10.4056/sigs.3507020
54. Mavrommatis K, Pukall R, Rohde C, Chen F, Sims D, Brettin T, et al. Complete genome sequence of cryptobacterium curtum type strain (12-3). Stand Genomic Sci (2009) 1(2):93–100. doi: 10.4056/sigs.12260
55. Gupta RS, Chen WJ, Adeolu M, Chai Y. Molecular signatures for the class coriobacteriia and its different clades; proposal for division of the class coriobacteriia into the emended order coriobacteriales, containing the emended family coriobacteriaceae and atopobiaceae fam. Nov., and eggerthellales ord. Nov., containing the family eggerthellaceae fam. Nov. Int J Syst Evol Microbiol (2013) 63(Pt 9):3379–97. doi: 10.1099/ijs.0.048371-0
56. Yi W, Ji Y, Gao H, Pan R, Wei Q, Cheng J, et al. Does the gut microbiome partially mediate the impact of air pollutants exposure on liver function? Evidence based on schizophrenia patients. Environ pollut (2021) 291:118135. doi: 10.1016/j.envpol.2021.118135
57. Wu Y, Tan MB, Jiang HY, Lu XD, Li XW, Yang FS. [Microorganisms in the urethral secretions of chronic prostatitis patients: A genomic analysis]. Zhonghua Nan Ke Xue (2021) 27(2):114–23.
58. Sorokin A, Brown JL, Thompson PD. Primary biliary cirrhosis, hyperlipidemia, and atherosclerotic risk: A systematic review. Atherosclerosis (2007) 194(2):293–9. doi: 10.1016/j.atherosclerosis.2006.11.036
59. Carey EJ, Ali AH, Lindor KD. Primary biliary cirrhosis. Lancet (2015) 386(10003):1565–75. doi: 10.1016/s0140-6736(15)00154-3
60. Guo L, Wang YY, Wang JH, Zhao HP, Yu Y, Wang GD, et al. Associations of gut microbiota with dyslipidemia based on sex differences in subjects from northwestern China. World J Gastroenterol (2022) 28(27):3455–75. doi: 10.3748/wjg.v28.i27.3455
61. Storm-Larsen C, Hande LN, Kummen M, Thunhaug H, Vestad B, Hansen SH, et al. Reduced gut microbial diversity in familial hypercholesterolemia with no effect of omega-3 polyunsaturated fatty acids intervention - a pilot trial. Scand J Clin Lab Invest (2022) 82(5):363–70. doi: 10.1080/00365513.2022.2102540
62. Floreani A, Cazzagon N, Franceschet I, Canesso F, Salmaso L, Baldo V. Metabolic syndrome associated with primary biliary cirrhosis. J Clin Gastroenterol (2015) 49(1):57–60. doi: 10.1097/mcg.0000000000000029
63. Waite DW, ChuvoChina M, Pelikan C, Parks DH, Yilmaz P, Wagner M, et al. Proposal to reclassify the proteobacterial classes deltaproteobacteria and oligoflexia, and the phylum thermodesulfobacteria into four phyla reflecting major functional capabilities. Int J Syst Evol Microbiol (2020) 70(11):5972–6016. doi: 10.1099/ijsem.0.004213
64. Campbell AG, Campbell JH, Schwientek P, Woyke T, Sczyrba A, Allman S, et al. Multiple single-cell genomes provide insight into functions of uncultured deltaproteobacteria in the human oral cavity. PloS One (2013) 8(3):e59361. doi: 10.1371/journal.pone.0059361
65. Amado PPP, Kawamoto D, Albuquerque-Souza E, Franco DC, Saraiva L, Casarin RCV, et al. Oral and fecal microbiome in molar-incisor pattern periodontitis. Front Cell Infect Microbiol (2020) 10:583761. doi: 10.3389/fcimb.2020.583761
66. Wei B, Wang Y, Xiang S, Jiang Y, Chen R, Hu N. Alterations of gut microbiome in patients with type 2 diabetes mellitus who had undergone cholecystectomy. Am J Physiol Endocrinol Metab (2021) 320(1):E113–e21. doi: 10.1152/ajpendo.00471.2020
67. Natividad JM, Lamas B, Pham HP, Michel ML, Rainteau D, Bridonneau C, et al. Bilophila wadsworthia aggravates high fat diet induced metabolic dysfunctions in mice. Nat Commun (2018) 9(1):2802. doi: 10.1038/s41467-018-05249-7
68. Coutinho C, Coutinho-Silva R, Zinkevich V, Pearce CB, Ojcius DM, Beech I. Sulphate-reducing bacteria from ulcerative colitis patients induce apoptosis of gastrointestinal epithelial cells. Microb Pathog (2017) 112:126–34. doi: 10.1016/j.micpath.2017.09.054
69. Lin YC, Lin HF, Wu CC, Chen CL, Ni YH. Pathogenic effects of desulfovibrio in the gut on fatty liver in diet-induced obese mice and children with obesity. J Gastroenterol (2022) 57(11):913–25. doi: 10.1007/s00535-022-01909-0
70. Murros KE. Hydrogen sulfide produced by gut bacteria may induce parkinson’s disease. Cells (2022) 11(6):978. doi: 10.3390/cells11060978
71. Karnachuk OV, Rusanov II, Panova IA, Grigoriev MA, Zyusman VS, Latygolets EA, et al. Microbial sulfate reduction by desulfovibrio is an important source of hydrogen sulfide from a large swine finishing facility. Sci Rep (2021) 11(1):10720. doi: 10.1038/s41598-021-90256-w
72. Buret AG, Allain T, Motta JP, Wallace JL. Effects of hydrogen sulfide on the microbiome: from toxicity to therapy. Antioxid Redox Signal (2022) 36(4-6):211–9. doi: 10.1089/ars.2021.0004
73. Chen L, Gao Y, Zhao Y, Yang G, Wang C, Zhao Z, et al. Chondroitin sulfate stimulates the secretion of H(2)S by desulfovibrio to improve insulin sensitivity in nafld mice. Int J Biol Macromol (2022) 213:631–8. doi: 10.1016/j.ijbiomac.2022.05.195
74. Chen W, Wei Y, Xiong A, Li Y, Guan H, Wang Q, et al. Comprehensive analysis of serum and fecal bile acid profiles and interaction with gut microbiota in primary biliary cholangitis. Clin Rev Allergy Immunol (2020) 58(1):25–38. doi: 10.1007/s12016-019-08731-2
75. Lindor KD, Bowlus CL, Boyer J, Levy C, Mayo M. Primary biliary cholangitis: 2021 practice guidance update from the american association for the study of liver diseases. Hepatology (2022) 75(4):1012–3. doi: 10.1002/hep.32117
76. Del Barrio M, Lavín L, Santos-Laso Á, Arias-Loste MT, Odriozola A, Rodriguez-Duque JC, et al. Faecal microbiota transplantation, paving the way to treat non-alcoholic fatty liver disease. Int J Mol Sci (2023) 24(7):6123. doi: 10.3390/ijms24076123
77. Suk KT, Koh H. New perspective on fecal microbiota transplantation in liver diseases. J Gastroenterol Hepatol (2022) 37(1):24–33. doi: 10.1111/jgh.15729
78. Yan X, Bai L, Qi P, Lv J, Song X, Zhang L. Potential effects of regulating intestinal flora on immunotherapy for liver cancer. Int J Mol Sci (2023) 24(14):11387. doi: 10.3390/ijms241411387
79. Gerussi A, D’Amato D, Cristoferi L, O’Donnell SE, Carbone M, Invernizzi P. Multiple therapeutic targets in rare cholestatic liver diseases: time to redefine treatment strategies. Ann Hepatol (2020) 19(1):5–16. doi: 10.1016/j.aohep.2019.09.009
80. Allegretti JR, Kassam Z, Carrellas M, Mullish BH, Marchesi JR, Pechlivanis A, et al. Fecal microbiota transplantation in patients with primary sclerosing cholangitis: A pilot clinical trial. Off J Am Coll Gastroenterol | ACG (2019) 114(7):1071–9. doi: 10.14309/ajg.0000000000000115
stands for the exposure variance defined by each IV, means effect allele frequency, and refer to the estimated effect and standard error of SNPs on specific gut microbiome respectively, and is the sample size.
Keywords: gastrointestinal microbiome, primary biliary cholangitis, Mendelian randomization, genetic predisposition, autoimmune liver disease
Citation: Luo X and You X (2023) Genetic predisposition of the gastrointestinal microbiome and primary biliary cholangitis: a bi-directional, two-sample Mendelian randomization analysis. Front. Endocrinol. 14:1225742. doi: 10.3389/fendo.2023.1225742
Received: 19 May 2023; Accepted: 29 September 2023;
Published: 12 October 2023.
Edited by:
Raffaella Maria Gadaleta, University of Bari Aldo Moro, ItalyReviewed by:
Bruno Fosso, University of Bari Aldo Moro, ItalyCopyright © 2023 Luo and You. This is an open-access article distributed under the terms of the Creative Commons Attribution License (CC BY). The use, distribution or reproduction in other forums is permitted, provided the original author(s) and the copyright owner(s) are credited and that the original publication in this journal is cited, in accordance with accepted academic practice. No use, distribution or reproduction is permitted which does not comply with these terms.
*Correspondence: Xin You, eW91eGluQHB1bWNoLmNu
Disclaimer: All claims expressed in this article are solely those of the authors and do not necessarily represent those of their affiliated organizations, or those of the publisher, the editors and the reviewers. Any product that may be evaluated in this article or claim that may be made by its manufacturer is not guaranteed or endorsed by the publisher.
Research integrity at Frontiers
Learn more about the work of our research integrity team to safeguard the quality of each article we publish.