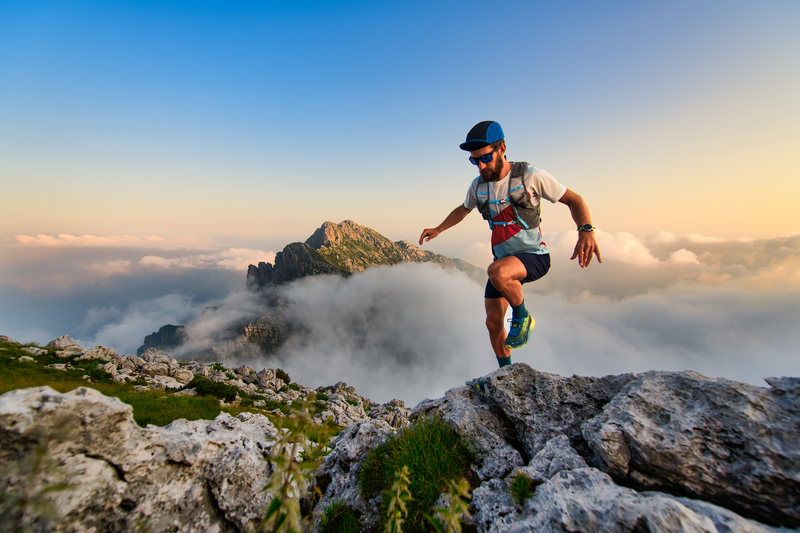
94% of researchers rate our articles as excellent or good
Learn more about the work of our research integrity team to safeguard the quality of each article we publish.
Find out more
ORIGINAL RESEARCH article
Front. Endocrinol. , 14 August 2023
Sec. Clinical Diabetes
Volume 14 - 2023 | https://doi.org/10.3389/fendo.2023.1224889
Objective: The purpose of this study was to determine the relation between the lipid accumulation product index (LAPI) and diabetic kidney disease (DKD) in patients with type 2 diabetes mellitus (T2DM).
Methods: Herein, 931 patients were enrolled and their data were collected. Then the interrelation between LAPI and DKD was assessed using multivariate logistic regression analyses (LRAs) and by a restricted cubic spline (RCS).
Results: In total, 931 participants (352 females and 579 males) aged 55 years on average were included in the study. After adjusting for several confounders, the odds ratio for DKD was increased evidently in the third LAPI tertile compared with that in the first LAPI tertile. In addition, the RCS revealed a positive interrelation between LAPI and DKD. In the subgroup analyses, age, sex, hyperlipidemia, hypertension, and HbA1c did not significantly interact with LAPI.
Conclusions: LAPI was higher in the DKD group than in the no-DKD group, and LAPI is positively linked with DKD, which may have potential value to diagnose DKD in clinical practice.
In 2021, about 10.5% of the global population aged 20–79 years suffered from diabetes. This percentage is expected to rise to approximately 12.2% in 2045 (1). The proportion of diabetes patients with type 2 diabetes mellitus (T2DM) is estimated to be 90% (2). Diabetes can lead to disability and mortality, which largely result from diabetic kidney disease (DKD) (3). DKD has become a major cause of end-stage renal disease (ESRD), and the incidence of DKD increases as the global prevalence of diabetes rises (1). Of all ESRD cases worldwide, 30–50% are estimated to be caused by DKD (4). Moreover, DKD increases the risk of cardiovascular and cerebrovascular diseases (4). Early surveillance and treatment of DKD are critical for reducing the burden on the healthcare system. Therefore, an effective tool for screening patients at a high risk of DKD is necessary.
Accumulating evidence has revealed that abdominal obesity (AO) is related to diabetes and its complications (5, 6). Moreover, it has been unveiled that relative to the total amount of adipose tissue, the distribution of adipose tissue is more important in the progression of vascular complications (7, 8). Some examinations, such as computed tomography and magnetic resonance imaging, can precisely detect abdominal adiposity. However, these examinations cannot be widely used because of their high cost and inconvenience. Therefore, indicators for AO, such as the body mass index (BMI), waist circumference (WC), waist-to-hip ratio (WHR) (9), waist-to-height ratio (WHtR) (10), the lipid accumulation product index (LAPI) (11), the visceral adiposity index (VAI) (12), and the Chinese visceral adiposity index (CVAI) (13), have been employed to assess abdominal adiposity. LAPI is a new marker of central lipid accumulation based on serum triglycerides and waist circumference, which was proposed to assess the lipid overaccumulation (11). Previous study indicated that LAPI had the ability to predict the risk of metabolic syndrome and T2DM (14, 15), cardiovascular events (16), non-alcoholic fatty liver disease (17). Moreover, LAPI may be an accurate marker of insulin resistance (18). However, little is known about the interrelation between LAPI and DKD.
Therefore, we conducted a cross-sectional study to decipher the relation between LAPI and DKD.
This study was a cross-sectional study. This study was approved by the Ethics Committee of the hospital. Informed consent was provided by all subjects before participating.
The participants between April 2017 and September 2021 were obtained from the electronic medical database of the National Metabolic Management Center (MMC) of Shanghai General Hospital (Songjiang District), which was established to conveniently and precisely diagnose and treat metabolic diseases (19). The inclusion criteria were as follows: patients met the World Health Organization’s 1999 criteria for diagnosis of T2DM (20) and patients were aged >18 years. The exclusion criteria were as follows: pregnancy, malignant tumor, chronic nephritis, and missing data. After screening, a total of 931 individuals were enrolled in this study.
Data including name, sex, age, educational level, coexisting diseases, and medical therapy were collected. A variety of anthropometric measurements were taken, including WC, hip circumference (HC), height, weight, blood pressure (BP), and heart rate. WC and HC were evaluated by trained staff in accordance with standard protocols. Biochemical data were collected from the MMC, including leukocyte counts, hemoglobin levels, and high-sensitivity C-reactive protein (hs-CRP) levels.
A diagnosis of hypertension was made when systolic BP (SBP) ≥ 140 mmHg and/or diastolic BP (DBP) ≥ 90 mmHg during repeated examinations (21), patients used antihypertensive drugs, or patients had a history of hypertension. A diagnosis of hyperlipidemia was made when total cholesterol (TC) ≥ 5.2 mM, triglycerides (TG) ≥1.7 mM, patients used lipid-lowering medications, or patients had a prior history of hyperlipidemia. The definition of current smokers was those who were smoking cigarettes currently, and the definition of current drinkers was those who drank alcohol currently. Educational level was with two categories of under high school and high school or above.
BMI was calculated as per the following formulas: BMI = weight [kg]/height [m2]. The formulas to calculate LAPI was previously published (12, 22): males: LAPI = [WC(cm)−65] × TG(mmol/L); females: LAPI = [WC(cm)−58] × TG(mmol/L). Estimated glomerular filtration rate (eGFR) scores were computed with reference to a previous study (23). We calculated the albumin (ALB)-to-creatinine ratio (ACR) based on the following formula: ACR = ALB/creatinine. The diagnosis of DKD was made in accord with a previous study (24): ACR was higher than 30 mg/g or eGFR<60 mL/min per 1.73 m2.
Numbers or medians (interquartile range) are used to present data. Continuous variables with skewed distributions were parsed by use of the Mann–Whitney U test, and an analysis of categorical variables was conducted for pairwise comparison using the χ2 test. P (two-sided) < 0.05 was indicative of statistical significance. SPSS 13.0 and R-4.1.3 were employed for statistical processing.
LAPI was divided into tertiles; the first tertile of LAPI was the lowest tertile, and the third tertile was the highest tertile. How LAPI is linked with DKD was parsed via multivariate logistic regression analyses (LRAs). Model 1 was adjusted for sex and age, Model 2 was further adjusted for DBP, SBP, educational level, and current drinking status, Model 3 was further adjusted for the duration of diabetes and hypertension, and Model 4 was further adjusted for fasting plasma glucose (FPG), leukocyte, ALB, hs-CRP, and glycated hemoglobin (HbA1c) levels. The interrelation between LAPI and DKD was examined by use of a restricted cubic spline (RCS) with four knots at the 5th, 35th, 65th, and 95th percentiles.
Additionally, by multivariate LRAs, we examined the association of LAPI with DKD based on age, sex, hyperlipidemia, hypertension, and HbA1c in different subgroups and computed the odds ratio (OR) and 95% confidence interval (CI). Moreover, we evaluated how the aforementioned subgroup variables interact with LAPI.
In total, 931 participants (352 females and 579 males) aged 55 years on average were enrolled. Of these participants, 327 had DKD and 604 did not. The two groups displayed significant differences in clinical data such as age, sex, educational level, hypertension, current drinker, SBP, DBP, height, BMI, WC, duration of diabetes, FPG, HbA1c, hemoglobin, leukocytes, gamma-glutamyl transferase, ALB, TG, TC, hs-CRP, ACR, and eGFR. Clinical data are illustrated in Table 1.
As demonstrated by multivariate LRAs, after adjusting for several confounding factors, elevated LAPI was associated with a higher likelihood of DKD (Table 2). Subsequent to the adjustment of the multivariate regression model for age, sex, DBP, SBP, educational level, current drinker, duration of diabetes, hypertension, FPG, HbA1c, leukocytes, ALB, and hs-CRP, higher OR values for DKD were found in the third LAPI tertile than in the first LAPI tertile (P < 0.001), and the OR (95% CI) for DKD was 2.135 (1.438–3.171) in the third tertile of LAPI compared to that in the first tertile. Additionally, RCS was performed to identify the relationship of LAPI with DKD. Consistently, the RCS curve demonstrated a positive relationship between LAPI and DKD in the study participants (Figure 1).
Table 2 Relations of lipid accumulation product index with diabetic kidney disease in patients with type 2 diabetes mellitus.
Figure 1 Association of lipid accumulation product index on a continuous scale with diabetic kidney disease in study participants. The solid line represents the odds ratio and the shad area represents the 95% confidence interval. Model was adjusted for age, diastolic blood pressure, systolic blood pressure, educational level, current drinker, duration of diabetes, hypertension, fasting plasma glucose, glycated hemoglobin, leukocyte, albumin and high-sensitivity C-reactive protein. OR, odds ratio; CI, confidence interval; RCS, restricted cubic spline; LAPI, lipid accumulation product index.
To further investigate the association of LAPI with DKD in different populations, the participants were grouped by age (<65 or ≥65 years), hyperlipidemia (N or Y), sex (female or male), HbA1c (<7% or ≥7%), and hypertension (N or Y). In most categories, LAPI was positively associated with DKD according to the subgroup analyses. Age, sex, hyperlipidemia, hypertension, and HbA1c did not significantly interact with LAPI (Figure 2).
Figure 2 Subgroup analyses of associations between lipid accumulation product index and diabetic kidney disease. Model was adjusted for age, sex, diastolic blood pressure, systolic blood pressure, educational level, current drinker, duration of diabetes, hypertension, fasting plasma glucose, glycated hemoglobin, leukocyte, albumin and high-sensitivity C-reactive protein. Subgroup variable was excluded from the model. OR, odds ratio; CI, confidence interval; HbA1c, glycated hemoglobin.
In conclusion, LAPI was higher in the DKD group than in the no-DKD group, and a positive relationship between LAPI and DKD was found after adjusting several potential confounders. Therefore, LAPI may have potential value to diagnose DKD in T2DM patients in clinical practice.
AO has been shown to be associated with a high risk of DKD in T2DM (25–27). A population-based cohort study has reported longitudinal associations of VAI and CVAI with DKD and suggested that VAI is independently related to the incidence of DKD (28). LAPI was calculated using TG and WC, which are novel indicators of central lipid accumulation (11). Accumulating evidence indicates that patients with diabetes display a higher LAPI level (14, 29). One study reported that the ability of single LAPI to predict CKD was better than that of VAI (30). Another study unveiled that several indicators pertaining to obesity, including BMI, WHR, WHtR, LAPI, and VAI, show relevance to kidney disease in late-stage T2DM patients (31). Similar results were obtained in the present study, we found that LAPI, one indicator for AO, was positively associated with DKD, which provides new scientific evidence for further study.
A previous study indicated that plasma TG levels are associated with reduced eGFR in new-onset cases of T2DM (32), and a high TG level contributes to DKD progression (33). These results are consistent with our conclusion that LAPI is positively associated with DKD. It is well acknowledged that oxidative stress and inflammation are common causes of DKD (34). Lipids accumulated in the kidney may induce oxidative stress or promote the release of proinflammatory cytokines, resulting in glomerular damage, glomerulosclerosis, and interstitial fibrosis (35, 36). Dysfunction of lipid metabolism in DKD could lead to podocyte damage, thus promoting DKD progression, which results in the disruption of mitochondrial function and lipid metabolism (37).
LAPI is recognized as a new indicator of accumulated visceral adipose tissue (38). Several studies reported that LAPI can be used to evaluate the risk of metabolic syndrome, diabetes, and cardiovascular disease (11, 14, 39). A higher LAPI in patients with T2DM was associated with increased oxidative stress, insulin resistance, and inflammation (40). The use of LAPI to assess visceral adipose tissue is characterized by higher lipolytic and proinflammatory activity (41). LAPI can be calculated easily, quickly and cheaply, which makes it suitable for use in clinical practice and in a large population (42). Our study revealed that LAPI was associated with DKD, so LAPI may be a useful marker of DKD in T2DM patients.
The strengths of the current study deserved to be mentioned. The study found the dose-response relationship between LAPI and DKD, and LAPI was evidently linked with DKD, which may be a potential index to identify DKD in clinical practice. There were a number of limitations to this study. First, it was cross-sectional study; longitudinal associations between LAPI and DKD need to be examined in future studies. Second, this study had a small sample size. In order to verify these results, further studies with larger samples are needed. Third, the insulin resistance index and the effects of lipid-lowering drugs were not analyzed in our study. Finally, further investigations of different ethnic groups are essential to examine the association between LAPI and DKD.
In conclusion, LAPI was significantly and positively associated with DKD in T2DM patients. Therefore, LAPI may be a potential marker for identifying DKD in T2DM. And longitudinal associations between LAPI and DKD need to be examined in further study.
The original contributions presented in the study are included in the article/supplementary material. Further inquiries can be directed to the corresponding authors.
The studies involving humans were approved by the Ethics Committee of Shanghai General Hospital, Shanghai Jiao Tong University School of Medicine. The studies were conducted in accordance with the local legislation and institutional requirements. The participants provided their written informed consent to participate in this study.
NF and YP designed this study. MT and SY collected and analyzed the study data. All authors wrote and reviewed the manuscript of the study. All authors approved the final manuscript.
This study was supported by the National Natural Science Foundation of China (81870596, 82170827, 82170829, 82200639), the Natural Science Foundation of Shanghai (21ZR1451200, 22ZR1450100), clinical research plan of SHDC (SHDC2020CR1016B) and Science and Technology Research Project in Songjiang District (22SJKGGG17).
The authors declare that the research was conducted in the absence of any commercial or financial relationships that could be construed as a potential conflict of interest.
All claims expressed in this article are solely those of the authors and do not necessarily represent those of their affiliated organizations, or those of the publisher, the editors and the reviewers. Any product that may be evaluated in this article, or claim that may be made by its manufacturer, is not guaranteed or endorsed by the publisher.
LAPI, lipid accumulation product index; DKD, diabetic kidney disease; T2DM, type 2 diabetes mellitus; LRAs, logistic regression analyses; RCS, restricted cubic spline; AO, abdominal obesity; BMI, body mass index; WC, waist circumference; WHR, waist-to-hip ratio; WHtR, waist-to-height ratio; VAI, visceral adiposity index; CVAI, Chinese visceral adiposity index; MMC, Metabolic Management Center; HC, hip circumference; BP, blood pressure; hs-CRP, high-sensitivity C-reactive protein; SBP, systolic blood pressure; DBP, diastolic blood pressure; TC, total cholesterol; TG, triglycerides; eGFR, Estimated glomerular filtration rate; ALB, albumin; ACR, albumin-to-creatinine ratio; FPG, fasting plasma glucose; HbA1c, glycated hemoglobin; OR, odds ratio; CI, confidence interval.
1. Sun H, Saeedi P, Karuranga S, Pinkepank M, Ogurtsova K, Duncan BB, et al. Idf diabetes atlas: global, regional and country-level diabetes prevalence estimates for 2021 and projections for 2045. Diabetes Res Clin Pract (2022) 183:109119. doi: 10.1016/j.diabres.2021.109119
2. Saeedi P, Petersohn I, Salpea P, Malanda B, Karuranga S, Unwin N, et al. Global and regional diabetes prevalence estimates for 2019 and projections for 2030 and 2045: results from the international diabetes federation diabetes atlas, 9(Th) edition. Diabetes Res Clin Pract (2019) 157:107843. doi: 10.1016/j.diabres.2019.107843
3. DeFronzo RA, Reeves WB, Awad AS. Pathophysiology of diabetic kidney disease: impact of sglt2 inhibitors. Nat Rev Nephrol (2021) 17(5):319–34. doi: 10.1038/s41581-021-00393-8
4. Ruiz-Ortega M, Rodrigues-Diez RR, Lavoz C, Rayego-Mateos S. Special issue "Diabetic nephropathy: diagnosis, prevention and treatment". J Clin Med (2020) 9(3). doi: 10.3390/jcm9030813
5. Salehinia F, Abdi H, Hadaegh F, Serahati S, Valizadeh M, Azizi F, et al. Abdominal obesity phenotypes and incident diabetes over 12 years of follow-up: the tehran lipid and glucose study. Diabetes Res Clin Pract (2018) 144:17–24. doi: 10.1016/j.diabres.2018.07.021
6. Zhou R, Li F, Chen G, Fu Q, Gu S, Wu X. Associations between general and abdominal obesity and incident diabetic neuropathy in participants with type 2 diabetes mellitus. J Diabetes (2021) 13(1):33–42. doi: 10.1111/1753-0407.13075
7. Man RE, Sabanayagam C, Chiang PP, Li LJ, Noonan JE, Wang JJ, et al. Differential association of generalized and abdominal obesity with diabetic retinopathy in asian patients with type 2 diabetes. JAMA Ophthalmol (2016) 134(3):251–7. doi: 10.1001/jamaophthalmol.2015.5103
8. Lee SW, Son JY, Kim JM, Hwang SS, Han JS, Heo NJ. Body fat distribution is more predictive of all-cause mortality than overall adiposity. Diabetes Obes Metab (2018) 20(1):141–7. doi: 10.1111/dom.13050
9. Yousuf M. Waist-to-Hip Circumference Ratio (Whr) as an Index of Obesity. Ann Saudi Med (1996) 16(1):93–4. doi: 10.5144/0256-4947.1996.93b
10. Yoo EG. Waist-to-Height Ratio as a Screening Tool for Obesity and Cardiometabolic Risk. Korean J Pediatr (2016) 59(11):425–31. doi: 10.3345/kjp.2016.59.11.425
11. Kahn HS. The "Lipid accumulation product" Performs better than the body mass index for recognizing cardiovascular risk: A population-based comparison. BMC Cardiovasc Disord (2005) 5:26. doi: 10.1186/1471-2261-5-26
12. Amato MC, Giordano C, Galia M, Criscimanna A, Vitabile S, Midiri M, et al. Visceral adiposity index: A reliable indicator of visceral fat function associated with cardiometabolic risk. Diabetes Care (2010) 33(4):920–2. doi: 10.2337/dc09-1825
13. Han M, Qin P, Li Q, Qie R, Liu L, Zhao Y, et al. Chinese visceral adiposity index: A reliable indicator of visceral fat function associated with risk of type 2 diabetes. Diabetes/Metabolism Res Rev (2021) 37(2):e3370. doi: 10.1002/dmrr.3370
14. Wakabayashi I, Daimon T. A strong association between lipid accumulation product and diabetes mellitus in Japanese women and men. J Atheroscl Thromb (2014) 21(3):282–8. doi: 10.5551/jat.20628
15. Xiang S, Hua F, Chen L, Tang Y, Jiang X, Liu Z. Lipid accumulation product is related to metabolic syndrome in women with polycystic ovary syndrome. Exp Clin Endocrinol Diabetes Off Journal German Soc Endocrinol [and] German Diabetes Assoc (2013) 121(2):115–8. doi: 10.1055/s-0032-1333261
16. Hosseinpanah F, Barzin M, Mirbolouk M, Abtahi H, Cheraghi L, Azizi F. Lipid accumulation product and incident cardiovascular events in a normal weight population: tehran lipid and glucose study. Eur J Prev Cardiol (2016) 23(2):187–93. doi: 10.1177/2047487314558771
17. Dai H, Wang W, Chen R, Chen Z, Lu Y, Yuan H. Lipid accumulation product is a powerful tool to predict non-alcoholic fatty liver disease in chinese adults. Nutr Metab (2017) 14:49. doi: 10.1186/s12986-017-0206-2
18. Ji B, Qu H, Wang H, Wei H, Deng H. Association between the visceral adiposity index and homeostatic model assessment of insulin resistance in participants with normal waist circumference. Angiology (2017) 68(8):716–21. doi: 10.1177/0003319716682120
19. Zhang Y, Wang W, Ning G. Metabolic management center: an innovation project for the management of metabolic diseases and complications in China. J Diabetes (2019) 11(1):11–3. doi: 10.1111/1753-0407.12847
20. Gabir MM, Hanson RL, Dabelea D, Imperatore G, Roumain J, Bennett PH, et al. The 1997 american diabetes association and 1999 world health organization criteria for hyperglycemia in the diagnosis and prediction of diabetes. Diabetes Care (2000) 23(8):1108–12. doi: 10.2337/diacare.23.8.1108
21. Unger T, Borghi C, Charchar F, Khan NA, Poulter NR, Prabhakaran D, et al. International society of hypertension global hypertension practice guidelines. Hypertension (Dallas Tex 1979) (2020) 75(6):1334–57. doi: 10.1161/hypertensionaha.120.15026
22. Wan H, Wang Y, Xiang Q, Fang S, Chen Y, Chen C, et al. Associations between abdominal obesity indices and diabetic complications: chinese visceral adiposity index and neck circumference. Cardiovasc Diabetol (2020) 19(1):118. doi: 10.1186/s12933-020-01095-4
23. Levey AS, Stevens LA, Schmid CH, Zhang YL, Castro AF 3rd, Feldman HI, et al. A new equation to estimate glomerular filtration rate. Ann Intern Med (2009) 150(9):604–12. doi: 10.7326/0003-4819-150-9-200905050-00006
24. American Diabetes A. 11. Microvascular complications and foot care: standards of medical care in diabetes-2021. Diabetes Care (2021) 44(Suppl 1):S151–S67. doi: 10.2337/dc21-S011
25. Pinto-Sietsma SJ, Navis G, Janssen WM, de Zeeuw D, Gans RO, de Jong PE. A central body fat distribution is related to renal function impairment, even in lean subjects. Am J Kidney Dis (2003) 41(4):733–41. doi: 10.1016/s0272-6386(03)00020-9
26. Hanai K, Babazono T, Nyumura I, Toya K, Ohta M, Bouchi R, et al. Involvement of visceral fat in the pathogenesis of albuminuria in patients with type 2 diabetes with early stage of nephropathy. Clin Exp Nephrol (2010) 14(2):132–6. doi: 10.1007/s10157-009-0245-8
27. Zhao Q, Yi X, Wang Z. Meta-analysis of the relationship between abdominal obesity and diabetic kidney disease in type 2 diabetic patients. Obes Facts (2021) 14(4):338–45. doi: 10.1159/000516391
28. Wu Z, Yu S, Kang X, Liu Y, Xu Z, Li Z, et al. Association of visceral adiposity index with incident nephropathy and retinopathy: A cohort study in the diabetic population. Cardiovasc Diabetol (2022) 21(1):32. doi: 10.1186/s12933-022-01464-1
29. Yan G, Li F, Elia C, Zhao Y, Wang J, Chen Z, et al. Association of lipid accumulation product trajectories with 5-year incidence of type 2 diabetes in chinese adults: A cohort study. Nutr Metab (2019) 16:72. doi: 10.1186/s12986-019-0399-7
30. Chen T, Wang X, Wang X, Chen H, Xiao H, Tang H, et al. Comparison of novel metabolic indices in estimation of chronic kidney diseases in a southern chinese population. Diabetes Metab Syndrome Obes Targets Ther (2020) 13:4919–27. doi: 10.2147/dmso.s286565
31. Ou YL, Lee MY, Lin IT, Wen WL, Hsu WH, Chen SC. Obesity-related indices are associated with albuminuria and advanced kidney disease in type 2 diabetes mellitus. Ren Fail (2021) 43(1):1250–8. doi: 10.1080/0886022x.2021.1969247
32. Wang C, Wang L, Liang K, Yan F, Hou X, Liu F, et al. Poor control of plasma triglycerides is associated with early decline of estimated glomerular filtration rates in new-onset type 2 diabetes in China: results from a 3-year follow-up study. J Diabetes Res (2020) 2020:3613041. doi: 10.1155/2020/3613041
33. Gong L, Wang C, Ning G, Wang W, Chen G, Wan Q, et al. High concentrations of triglycerides are associated with diabetic kidney disease in new-onset type 2 diabetes in China: findings from the China cardiometabolic disease and cancer cohort (4c) study. Diabetes Obes Metab (2021) 23(11):2551–60. doi: 10.1111/dom.14502
34. Forbes JM, Cooper ME. Mechanisms of diabetic complications. Physiol Rev (2013) 93(1):137–88. doi: 10.1152/physrev.00045.2011
35. Abrass CK. Cellular lipid metabolism and the role of lipids in progressive renal disease. Am J Nephrol (2004) 24(1):46–53. doi: 10.1159/000075925
36. Abrass CK. Lipid metabolism and renal disease. Contrib Nephrol (2006) 151:106–21. doi: 10.1159/000095323
37. Ducasa GM, Mitrofanova A, Fornoni A. Crosstalk between lipids and mitochondria in diabetic kidney disease. Curr Diabetes Rep (2019) 19(12):144. doi: 10.1007/s11892-019-1263-x
38. Brahimaj A, Rivadeneira F, Muka T, Sijbrands EJG, Franco OH, Dehghan A, et al. Novel metabolic indices and incident type 2 diabetes among women and men: the rotterdam study. Diabetologia (2019) 62(9):1581–90. doi: 10.1007/s00125-019-4921-2
39. Taverna MJ, Martínez-Larrad MT, Frechtel GD, Serrano-Ríos M. Lipid accumulation product: A powerful marker of metabolic syndrome in healthy population. Eur J Endocrinol (2011) 164(4):559–67. doi: 10.1530/eje-10-1039
40. MirmIran P, Bahadoran Z, Azizi F. Lipid accumulation product is associated with insulin resistance, lipid peroxidation, and systemic inflammation in type 2 diabetic patients. Endocrinol Metab (Seoul Korea) (2014) 29(4):443–9. doi: 10.3803/EnM.2014.29.4.443
41. Rotter I, Rył A, Szylińska A, Pawlukowska W, Lubkowska A, Laszczyńska M. Lipid accumulation product (Lap) as an index of metabolic and hormonal disorders in aging men. Exp Clin Endocrinol Diabetes Off journal German Soc Endocrinol [and] German Diabetes Assoc (2017) 125(3):176–82. doi: 10.1055/s-0042-116071
Keywords: lipid accumulation product, abdominal obesity, restricted cubic spline, type 2 diabetes mellitus, diabetic kidney disease
Citation: Tang M, Yao S, Cao H, Wei X, Zhen Q, Tan Y, Liu F, Wang Y, Peng Y and Fan N (2023) Interrelation between the lipid accumulation product index and diabetic kidney disease in patients with type 2 diabetes mellitus. Front. Endocrinol. 14:1224889. doi: 10.3389/fendo.2023.1224889
Received: 18 May 2023; Accepted: 31 July 2023;
Published: 14 August 2023.
Edited by:
Habib Yaribeygi, Semnan University of Medical Sciences, IranReviewed by:
Ningjian Wang, Shanghai Jiao Tong University, ChinaCopyright © 2023 Tang, Yao, Cao, Wei, Zhen, Tan, Liu, Wang, Peng and Fan. This is an open-access article distributed under the terms of the Creative Commons Attribution License (CC BY). The use, distribution or reproduction in other forums is permitted, provided the original author(s) and the copyright owner(s) are credited and that the original publication in this journal is cited, in accordance with accepted academic practice. No use, distribution or reproduction is permitted which does not comply with these terms.
*Correspondence: Nengguang Fan, Zm5nd2xwQDE2My5jb20=; Yongde Peng, eW9uZ2RlcGVuZzA5MDhAMTI2LmNvbQ==
†These authors have contributed equally to this work
Disclaimer: All claims expressed in this article are solely those of the authors and do not necessarily represent those of their affiliated organizations, or those of the publisher, the editors and the reviewers. Any product that may be evaluated in this article or claim that may be made by its manufacturer is not guaranteed or endorsed by the publisher.
Research integrity at Frontiers
Learn more about the work of our research integrity team to safeguard the quality of each article we publish.