- 1Medical Department III - Endocrinology, Nephrology, Rheumatology, University of Leipzig Medical Center, Leipzig, Germany
- 2Helmholtz Institute for Metabolic, Obesity and Vascular Research (HI-MAG) of the Helmholtz Center Munich at the University of Leipzig and University Hospital Leipzig, Leipzig, Germany
- 3Deutsches Zentrum für Diabetesforschung e.V., Neuherberg, Germany
Over the past 50 years, the number of overweight/obese people increased significantly, making obesity a global public health challenge. Apart from rare monogenic forms, obesity is a multifactorial disease, most likely resulting from a concerted interaction of genetic, epigenetic and environmental factors. Although recent studies opened new avenues in elucidating the complex genetics behind obesity, the biological mechanisms contributing to individual’s risk to become obese are not yet fully understood. Non-genetic factors such as eating behaviour or physical activity are strong contributing factors for the onset of obesity. These factors may interact with genetic predispositions most likely via epigenetic mechanisms. Epigenome-wide association studies or methylome-wide association studies are measuring DNA methylation at single CpGs across thousands of genes and capture associations to obesity phenotypes such as BMI. However, they only represent a snapshot in the complex biological network and cannot distinguish between causes and consequences. Intervention studies are therefore a suitable method to control for confounding factors and to avoid possible sources of bias. In particular, intervention studies documenting changes in obesity-associated epigenetic markers during lifestyle driven weight loss, make an important contribution to a better understanding of epigenetic reprogramming in obesity. To investigate the impact of lifestyle in obesity state specific DNA methylation, especially concerning the development of new strategies for prevention and individual therapy, we reviewed 19 most recent human intervention studies. In summary, this review highlights the huge potential of targeted interventions to alter disease-associated epigenetic patterns. However, there is an urgent need for further robust and larger studies to identify the specific DNA methylation biomarkers which influence obesity.
Introduction
Obesity has reached pandemic proportions in the last 50 years and represents a worldwide health challenge (1). People who are overweight or obese have a higher risk for numerous cardiometabolic diseases such as type 2 diabetes (T2D), dyslipidemia, stroke and hypertension (1). Beside some rare monogenic forms (2), obesity is a multifactorial disease driven by an interaction of polygenic predispositions and the exposure to obesogenic environmental factors. Various diets with different levels of macronutrients have been tested and compared to define successful weight loss strategies (3), however, responses to dietary changes are extremely individual. That is why personalized nutritional recommendations are becoming more and more important to meet individual needs. Lifestyle interventions such as increasing energy expenditure through intensive physical activity and lowering energy intake through various diets are important set points to control the disease. However, long-term success is often very individual and weight regain quite common (4). In order, to better understand this highly complex interplay, research into genetic and lifestyle-related factors that influence human metabolism, most likely mediated by epigenetic regulations, is inevitable. Future findings might contribute to better understanding of the high variability in individual's response to specific nutritional and physical activity interventions and may improve long-term therapy by personalisation (5).
Although epigenetics and its involvement in metabolic diseases still represents a young research field, it has significantly advanced during the last two decades. Epigenetic modifications include DNA methylation, histone modifications, and non-coding RNAs which are regulating cell-specific gene expression, cell differentiation, parental imprinting, X chromosome inactivation, and genomic stability and structure (6). DNA methylation is the most commonly studied modification and describes the methylation of the carbon 5 position within cytosine bases, resulting in 5-methylcytosine. This occurs predominantly at cytosines within CG dinucleotides ("CpG" sites) in mammalian genomes and is associated with gene silencing when occurring at gene promoters and enhancers (7). Epigenetic modifications are mediating between environmental and genetic factors - environmental changes can lead to epigenetic regulations, which in turn can affect gene activity (8). There are two different starting points to analyse epigenetic modifications in regard to obesity. On the one hand, epigenetic alterations might be causal for the development of obesity by inducing inappropriate expression or silencing of obesity-associated genes and regulatory sequences, leading to metabolic imbalances (9). On the other hand, epigenetic changes can also arise as a consequence of obesity and predispose obesity-associated co-morbidities such as T2D (10) or cancer (11, 12).
Epigenome-wide association studies (EWAS) represent a powerful tool for investigating associations between epigenetic markers and the obesity state defined by BMI or parameters of fat distribution, aiming to understand the molecular mechanisms underlying the disease risk (13). EWAS or methylome-wide association studies (MWAS) measure DNA methylations at single CpGs or regions containing CpGs across thousands of genes using genome-wide approaches based on techniques such as Illumina arrays or whole genome bisulfite sequencing and analyse potential associations with disease phenotypes. However, they only represent a snapshot in complex biological networks and cannot distinguish between causes and consequences. Intervention studies are therefore a suitable method to control confounding factors and avoid possible sources of bias. In particular, intervention studies which document changes in obesity-associated epigenetic markers during weight loss can make an important contribution to this research field. Peripheral blood is the most commonly used biospecimen for these intervention studies (14). This is due to its accessibility through a minimally invasive procedure and due to the fact that blood is often the only available source for a biomaterial. Additionally, some studies suggest that specific DNA methylation changes in blood may reflect pathological conditions in target organs that are not accessible by biopsy (15). However, while DNA methylation profiles in blood can summarize information about systemic exposures or diseases, they cannot specifically assess cells from a single organ or tissue (16). Studies use different techniques to control for the effect of cell heterogeneity and thus possible confusion (17, 18). These include various deconvolution techniques (19), which provide a framework for estimating the relative proportions of blood cell types. Of note, there are studies, which identified an epigenetic signature potentially mirroring the epigenetic regulation of obesity-related adipose tissue dysfunction. These studies provide a DNA methylation map in circulating leukocytes reflecting subcutaneous adipose tissue methylation pattern by comparing both tissues in patients with obesity vs normal-weight individuals (20–22).
Although corresponding research is advancing very fast, aiming to identify new markers allowing the development of new therapy options in obesity, the last systematic review was performed by Aronica et al. in 2017. The authors summarised candidate-based and genome-wide approaches analysing DNA methylation at baseline and at the endpoint of a weight loss intervention in overweight/obese subjects who were free of comorbidities such as hereditary diseases or cancer (8). They identified 25 longitudinal intervention studies over eight years (from 2008 to 2016), which examined either pre- vs. post-interventional (diet and/or exercise/ metabolic surgery interventions) DNA methylation changes or DNA methylation differences between patients with high and low response to the intervention. Back in 2017 the majority of studies were candidate gene approaches (N=16), while only nine used genome-wide data sets analysing either differentially methylated single CpG positions (DMPs) or differentially methylated regions (DMRs).
However, since 2017 numerous new studies in this field came up with improved interventional and analytical approaches, further highlighting lifestyle habits as therapeutic options and strongly contributing to our understanding of the mechanistic role of DNA methylation in obesity. In the present review, we focus on human interventional studies, which aim to reduce body weight in individuals with overweight or obesity and which are based on the main lifestyle parameters: diet and physical activity.
This review discusses the contribution of candidate-based and genome-wide DNA methylation studies in regard to lifestyle treatments, including a special focus on methylation age and in-utero studies. We discuss recent achievements in this young field of research and point out strengths and possible weaknesses. We finally wish to draw attention to the potential of this field for the development of new strategies for prevention and individualised therapies.
Methods
Screening and inclusion/exclusion criteria
We performed a PubMed search (dated 05.07.2023) as described in Figure 1, for studies published during the last five years (2018-2023) using the following mash terms: “lifestyle intervention and methylation” and “weight loss intervention and methylation”. We focused on longitudinal studies measuring either specific candidate loci, global or genome-wide DNA methylation at baseline and after finishing an individual lifestyle intervention designed to lose weight and/or improve general health conditions in subjects with overweight or obesity. Cross-sectional studies, case-control studies, longitudinal studies without intervention, interventional studies conducted in vitro only and in animals or subjects without overweight or obesity were excluded. We further excluded subjects undergoing pharmacotherapies, weight loss surgeries and those suffering from severe disease stages such as cancer. On the other hand, we included studies comparing responder vs. non- responder to respective lifestyle interventions. Furthermore, we focused exclusively on DNA methylation, excluding other epigenetic mechanisms such as histone modifications and noncoding RNAs.
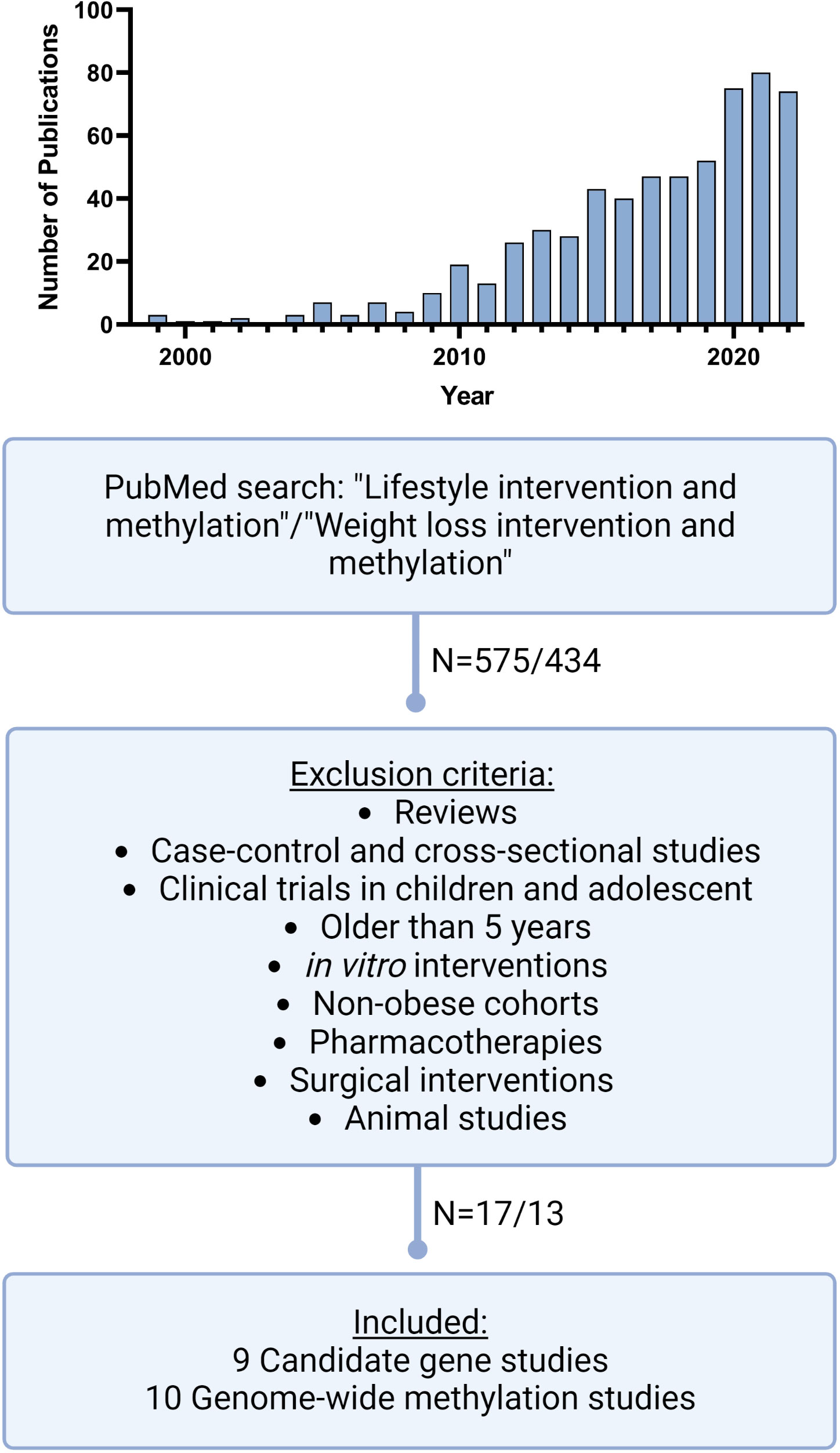
Figure 1 Literature search workflow. Schematic presentation of our literature search on PubMed including mash terms, exclusion criteria and publication numbers. In addition, the top figure shows the distribution of the numbers of publications during the recent years, with a sharp increase of publications matching our mash terms since 2010 but also the most significant increase in the last 3 years. (Created with Biorender.com).
Results
Current state of research
Our structured PubMed search revealed an eminent increase of studies during the last 5 years, dealing with DNA methylation under weight loss conditions. In the years before 2009, the number of publications was still in the single digits and rose sharply to 80 publications in 2021 (Figure 1). We identified nine new candidate gene and ten new genome-wide studies, which were performed between 2018 and 2023. All candidate gene approaches analysed changes in DNA methylation before and after an individual lifestyle intervention, while the identified ten genome-wide studies (Table 1) assessed either DNA methylation before and after weight loss (N=7) or compared responder vs. non-responder (N=3) after different lifestyle interventions. The majority of them used array-based platforms, most commonly Infinium 850k or 450k arrays (Illumina), to interrogate methylation changes on single site resolution. The 850k array examines the methylation status of 853,307 CpG sites, adding 413,745 CpG sites to the 450k (33). Six of the genome-wide studies also performed gene expression analysis (25, 26, 28, 29, 31, 32, 34).
DNA methylation associated with weight loss
Three of the reviewed genome-wide intervention studies aimed to identify DNA methylation biomarkers for response to weight loss. This was accomplished by comparing baseline and endpoint methylation differences between high and low responders to an individual intervention. The individual response to the weight loss intervention was defined differently within the three studies. In the study published by Keller et al., the responder group lost at least 10 % of their initial body weight, whereas in the study by Samblas et al., subjects were defined as high responders already with a weight reduction of > 8 % (23, 25). In the third study, the change in BMI was used to classify responders (lost 4.55 ± 0.91 BMI units (kg/m2)) and non-responders (lost 1.95 ± 0.73 kg/m2) rather than mean weight loss (24). Two studies intervened with different low-calorie diets (24, 25), while the other study used a Mediterranean diet combined with physical activity (23). Study intervention times ranged from four months to 18 months and the number of participants varied from 47 to 201 (Table 1). Among them two studies identified DMRs (23, 24) whereas the third only reported DMPs (25). The identified DMRs and DMPs from the three studies retained their significance even after correction for multiple testing. Among the identified DMRs five were significantly hypermethylated (CRISP2, SLC6A12, SLFN12, AURKC, PON3) and four significantly hypomethylated (LRRC27, RNF39, LINC00539, NTSR1) in responders compared to non-responders (23, 24). DNA methylation analysis by Salas-Pérez et al., not only identified one DMR at PON3, but also 63 CpG sites that were differentially methylated between these two groups (24). The third study revealed four genes, CD44, ITPR1, MTSS1 and FBXW5 by overlapping identified DMPs with differentially regulated transcripts (25). We were able to find other overlaps in genes between the three studies, but these did not withstand multiple testing. Although no overlap in differentially methylated genes could be observed between the three newly published studies in regard to weight loss response, such studies indicate that methylation differences at multiple genomic sites could serve as prognostic biomarkers to predict successful weight loss therapy. However, validation is still essential in order to delineate the real potential of DNA methylation pattern in predicting individual’s response to a specific lifestyle therapy.
The remaining seven studies measured DNA methylation changes in response to weight loss. Most of the studies intervened with a low-calorie diet, and some studies also combined this change in diet with physical activity (31, 32). One study even compared three different dietary interventions as addition to physical activity and thereby investigated the beneficial effect of dietary polyphenols (26). Personalised weight loss programs have also been used, including T2D-specific social support programs, aiming to reduce risk factors associated with diabetes complications (28). Study intervention times ranged from six weeks to two years and the number of participants varied from eight to 672 (Table 1). Most of these studies used Benjamini Hochberg to correct their results for multiple testing with a false discovery rate (FDR) < 0.05 to detect changes in DMRs and DMPs. All studies examined DNA methylation in blood samples except for the study by Bollepalli et al., which examined subcutaneous adipose tissue (SAT) (31). Numerous DMRs with different functions have been identified. Those DMRs were annotated to genes mainly involved in e.g. immune, metabolic, and cardiometabolic signalling pathways, protein metabolism, and the development of muscles, organs, and skeletal systems. In addition, the study by Crujeiras et al. provided evidence of obesity-related methylome remodeling after dietary interventions, finding similar levels of methylation in treated obese patients as in normal-weight individuals. In fact, in the same study the most representative genes ZNF533 and FGFRL1, which were differentially methylated after dietary weight loss treatment, were previously identified as an epigenetic signature of adipose tissue associated with obesity, which is reflected in blood leukocytes (22). The study by Hoffmann et al. demonstrated many DMRs induced by the three different dietary interventions and further showed that a Mediterranean diet enriched in plant-based polyphenols has the highest capacity to regulate individual’s blood epigenome (26). This was the so far largest long-term RCT, analyzing lifestyle effects on human DNA methylation pattern. Thus, we performed an intersection analysis comparing all identified unique genes from the other six genome-wide approaches that showed statistically significant (FDR < 0.05) weight loss-related changes in DNA methylation with the results of Hoffmann et al. (26). The majority of the 2097 genes identified in the seven studies focusing on genome-wide methylation changes were unique (N=2051), however 47 genes were reported by at least two intervention studies and one gene, the Mitotic Arrest Deficient 1 Like 1 (MAD1L1) was even identified by three independent studies (26, 28, 29). This gene is part of the mitotic spindle-assembly checkpoint and thus plays a major role for cell cycle control with potential involvement in cancer development (35). Furthermore, other known candidate genes for obesity and T2D such as TCF7L2 locus, which was previously reported by Aronica et al. (8), were now validated again by two other lifestyle studies (26, 28).
While further intersecting those unique genes with the studies associating DNA methylation with weight loss response, we found 518 genes reported by both study types. Among them five genes (BCAS4, MYH15, SH3PXD2A, VIPR2 and WDPCP) were even reported by two weight loss response studies. Among them the WD repeat containing planar cell polarity effector (WDPCP) gene (24, 31) was identified by Salas-Perez et al. reporting one DMP in WDPCP, which was hypomethylated (~5%) in responders compared to non-responders of the weight loss intervention (24). This goes in line with the results of Bollepalli et al., who found an upregulation of the WDPCP gene expression in the heavier co-twins (31).
Epigenetic regulation of physiological candidates
Our search revealed only nine new candidate gene studies in the last five years. All studies compared DNA methylation at baseline and after finishing an individual lifestyle intervention for one or several candidate genes. The studies employed different methods including pyrosequencing, although array-based platforms have been dominating in the last years. Therefore, it is not surprising that candidate gene analyses were frequently based on previously generated and available genome-wide datasets. The candidate gene analyses were performed in interventional studies based on diet or a combination of diet and physical activity. Only the study by Willmer et al. intervened solely with physical activity (36). The candidate gene studies also varied greatly in the number of participants from 18 to 811 subjects, and the duration of the intervention from three weeks to two years (Table 2).
These studies analysed the methylation status of several genes related to immune response (NFATC2IP & FKBP5) (36, 38), mitochondrial function (CPT1A) (37) diabetes and ageing (TXNIP & CAV1) (39, 40), circadian rhythm (CLOCK, CRY2 & PER2) (41) as well as obesity related genes (e.g. FTO) (42). These genes were chosen based on previous studies because they were, among other things, associated with metabolic control, glucose homeostasis or obesity.
Because most studies examined different genes, reproducibility between the different studies is lacking, although genes such as FTO, PER2 and CLOCK were already reviewed by Aronica et al. (43, 44). The Fat Mass and Obesity-associated (FTO) gene was the first gene to be associated with body fat, obesity and BMI (45, 46). A study from 2017 reported that hypomethylation of the FTO non-promoter region is an early marker of T2D (47) and another study showed that hypomethylation induces overeating, fat accumulation, and obesity (48). The study we found suggests that resistance training increased DNA methylation in the FTO 5’UTR region due to weight loss and thereby may repress FTO mRNA expression (42). Thus, the epigenetic regulation of FTO might also play a role in obesity development, but further replication studies with larger sample sizes as well as functional analyses are warranted.
Chronobiological misalignment and disrupted physiological rhythms has been repeatedly linked to obesity (49, 50) and genes previously shown to be associated with the regulation of circadian rhythm such as CLOCK are likely to play a crucial role (51). A study by Milagro et al. from 2012 showed that the baseline methylation levels of CLOCK and PER2 are correlated with the magnitude of weight loss (43). A recent study by Rigamonti et al. showed that a short-term body weight reduction program induced a significant CLOCK hypermethylation together with a significant PER2 hypomethylation, suggesting that the body weight reduction program might result in beneficial cardiometabolic effects as well as in epigenetic remodelling of specific CLOCK genes. However, due to the experimental design, this study could not disclose whether epigenetic changes in CLOCK genes are cause or consequence of global cardiometabolic improvements (41).
Weight loss driven improvement of methylation age
With increasing age, the risk of mental and physical impairment and the development of diseases such as cancer, neurodegeneration, T2D and cardiovascular disease (52, 53) is also rising. Epigenetic changes can be induced by multiple factors such as genetics (54), environment (55) and lifestyle (56). In addition to molecular and cellular characteristics, epigenetic changes are affected by ageing but can also influence the ageing process itself (57). Out of more than 20 million methylation sites in the human genome, there are several thousand where the methylation levels correlate closely with age (58). Methylation age acceleration, defined as the difference between biological age (age of cells and tissue based on physiological evidence) and chronological age (actual age in years), is used to study the links between epigenetic ageing and disease development (59) and it has been implicated not only in obesity (60), but also in physical fitness (61) and stress (62). Cross-sectional studies have already provided evidence of a beneficial effect of a healthy lifestyle on several biological indicators of ageing such as epigenetic clock, telomere length and transcriptomic age (63, 64). However, little is known about the interaction between epigenetic ageing and lifestyle factors, including diet, alcohol abuse and physical activity. Therefore, longitudinal and interventional studies are needed to address and accurately quantify the benefit of lifestyle improvements on ageing. Thus, intervention studies can help to identify factors that have a positive influence on epigenetic age and thus contribute to improving general health.
So far, two intervention studies that examined changes in epigenetic age through weight loss and a healthier lifestyle in overweight and obese patients have been reported since 2018 (Table 3). One study linked physical functioning to biological age in older adults (65) whereas the other showed that lifestyle-based weight loss interventions can indeed reduce DNA methylation age (mAge) (66). Furthermore, Yaskolka Meir et al. demonstrated associations between mAge acceleration and parameters of body fat distribution and glycaemic control (66). Thus, DNA methylation-based biological measures of ageing might allow to stratify the individual health status and to predict risk of premature cardiometabolic diseases (66). It is thereby possible to investigate positive effects of lifestyle improvements on age-related epigenetic mechanisms (67). Yet, the results could be influenced by other important factors, and so, it is still elusive whether weight loss was the main reason for the positive biological effect (66). However, both studies were able to show that the epigenetic age is strongly correlated with health and could therefore be considered as an important biomarker for outcome predictions in human intervention studies.
Weight loss driven methylation remodelling during pregnancy
Maternal overweight and obesity is associated with the risk of overweight and obesity in children (68). Underlying mechanisms which contribute to the increased susceptibility for overweight and obesity and associated health consequences in children are not yet fully understood (69–72). Furthermore, overweight and obesity of the mother, weight gain during pregnancy, prenatal nutrition and physical activity have a direct impact on the health of the foetus (68). Those intrauterine disturbances can lead to epigenetic remodelling (6, 73) and further influence health outcomes in the offspring (74). In line, previous studies have shown the involvement of dietary micronutrients, after birth and during early life, in altering gene expression and thereby influencing health and disease later in life (75, 76). Moreover, low birth weight is a risk factor for developing non-insulin dependent diabetes mellitus (NIDDM) and cardiovascular disease (CVD) later in life (77, 78) and the delivery (caesarean section or vaginal delivery) plays an important role in the formation of the microbiome (79–82). The development of the child’s microbiome is determined by proper nutrition and the transition from breastfeeding to solid food (79). The microbiome normally returns to a state of equilibrium following stress such as a change in diet, short-term antibiotic treatment, or acute invasion by a pathogenic bacterium (83). However, short-term modulations of the gut microbiome can disrupt normal metabolite production (84) and this can lead to changes in host gene expression, which in turn could trigger longer-lasting effects in the host. For example, germ-free mice, a method used to determine whether the microbiome plays a causal role in regulating gene expression, showed lower genome-wide DNA methylation in colonic tissue compared to control animals (85). However, global DNA methylation increased dramatically in the germ-free mice after a fecal transplant.
Our systematic review highlighted five studies in the last five years which examined lifestyle interventions in pregnant women with obesity or overweight. They demonstrated clear associations between DNA methylation changes and health benefits of the mother and the child, such as a protective effect against the development of gestational diabetes (86), a beneficial effect on improving glycaemic control (87) and a reduced risk of the new born for obesity and obesity-related disorders later in life (88). These five studies reported genome-wide analyses following interventions with diet or a combination of diet and physical activity during pregnancy. Numerous genes were identified showing significantly altered DNA methylation after diet or physical activity interventions, such as DISC1, GBX2, HERC2, HUWE1 (89), LGR6 (90), RNF214, PCSK7, SYN3, JARID2, POLR2C (91). Nevertheless, one study showed no effect of the intervention neither on DNA methylation nor on the maternal BMI in early pregnancy (92). Of note, two of the identified genes, JARID2 and LGR2 have been reported in the above mentioned studies comparing responders and non-responders (23–25). It needs to be noted that all studies measured DNA methylation in cord blood samples which might not be a reliable indicator of the DNA methylation in the infant. In addition, umbilical cord blood contains different cell types, which may be present in different proportions in different samples, possibly distorting the effects of interest (93). Therefore, all analyses are adjusted for estimated cell type proportions and the true cell type proportions in the samples remain unknown. Nevertheless, since drawing cord blood is non-invasive and blood can be obtained in large quantities, it is often used for DNA methylation studies in pregnant women (94). Newly reviewed studies, as well as previous work, suggest that a healthy lifestyle during pregnancy is immensely important to reduce the risk of childhood obesity and the serious diseases associated with it, though the results are not consistent and challenging to compare as each study identified various differently methylated sites. Often these are single CpG sites located in different regions of the genome and with an unknown association to overweight, obesity or growth (95, 96). Furthermore, explicit attempts to replicate the findings from other studies have so far not been successful (96–98). Given the scarceness and the heterogeneous character of the available studies, there is an urgent need to perform large-scale analyses elucidating epigenetic foetal markers that are influenced by parental lifestyle (99).
Discussion
We conducted a systematic literature review on DNA methylation analysis in individuals with overweight or obesity who underwent a weight loss intervention program driven by lifestyle modifications. In addition, we also looked for studies analysing DNA methylation pattern as a marker for weight loss response. We focused on DNA methylation only since it is by far the most extensively studied epigenetic modification and can be used to quantify allele specific epigenetic changes on a single nucleotide resolution (8). In contrast, there are no human intervention studies examining histone modifications and only a few studies in mice which characterised histone modifications by lifestyle interventions (100, 101). However, when it comes to DNA methylation, it must be noted that many of the genome-wide approaches are still array based and thus only analyse around 1.5 - 2.8 % of all CpG sites in the human genome. Although sequencing-based methods with higher coverage have recently became more accessible and cost-effective, a large part of the human epigenome remains unexplored especially in regard to lifestyle interventions and obesity. Numerous new DNA methylation profiling methods such as reduced representation bisulfite sequencing, methylation capture bisulfite sequencing and advanced microarrays have been established over the past decades, expanding our understanding of DNA methylation in diseases such as obesity. Consequently, advances in DNA methylation have shifted the challenge from big data generation to data analysis (102).
The majority of epigenetic studies related to obesity are cross-sectional and lack measurements and potential adjustments for relevant lifestyle factors. Therefore, we exclusively chose human intervention studies in our review, as these overcome some biases, such as inferring false associations or those not driven by lifestyle factors. As mentioned above, we also excluded surgical intervention studies, since they represent major metabolic remodelling, including shortage of supply and are thus less comparable to standard lifestyle therapies based on diet and physical training (30, 103). Furthermore, patients eligible for a surgical intervention are characterised with higher degree of obesity, which is linked to a more frequent prescription of drugs targeting the associated comorbidities. In addition, also other factors affecting DNA methylation levels in post-surgical care, such as the prescription of vitamin/mineral supplements to prevent nutritional deficiencies, would compromise comparisons between surgical and dietary interventions (30, 103). We found 19 human intervention studies in our literature search which were published during the last five years, of which nine were candidate gene based and ten were genome-wide approaches. Among the genome-wide studies, three studies compared responders and non-responders to an individual lifestyle intervention and seven the effect of the intervention on DNA methylation changes.
Genome-wide DNA methylation changes
Compared with candidate gene approaches, the number of genome-wide analyses has increased greatly in the last five years (Figure 1), necessitating this updated discussion and bringing hypothesis-free studies more into focus (8).
Among them three studies showed baseline methylation levels at multiple genomic sites to be associated with individuals’ propensity to lose weight on specific lifestyle treatments. Although no overlap in response related methylation marks could be found between the three interventions, partially driven by differences in type of intervention, its duration, sample size and analytical approaches, they clearly suggest using blood DNA methylation marks as prognostic markers. Among the identified genes CD44 represents an interesting candidate since its hepatic mRNA expression was previously described to be enriched in morbidly obese individuals (104) and is associated with the development of adipose tissue inflammation and insulin resistance (105). Thus, CD44 renders a biomarker for insulin resistance and a possible therapeutic target for T2D treatment (106). In line with a previous work, showing that CD44 methylation can contribute to weight loss prediction (107) a recent work from the same lab showed that CD44 had higher expression and lower levels of DNA methylation in low responders compared to high responders through a low-calorie diet intervention. CD44 is involved in amplifying the inflammatory processes in obese individuals, and increased expression of CD44 prior to a calorie-restricted diet intervention may impair its effectiveness in terms of a successful weight loss (25). In addition to CD44, genes such as ATP10A, AQP9, and HIPK3 have previously been discussed by Aronica et al. in regard to weight loss response and were also identified in the latest studies comparing responders and non-responders to an individual calorie restriction intervention (23, 107, 108). However, they did not withstand corrections for multiple testing, as has also been shown for a number of genes in the three responder/non-responder studies (including RNF39, VIPR2, IGHMBP2, SH3PXD2A, WDPCP, VWDE, SYNJ2, VWDE, BCAS4, MYH15, PCDHGA4, KCNG2, FRMD4A, STK32C and LGR6). Although these genes may still be of considerable interest in the respective research field, unless validated in further studies, they should be seen with caution.
By intersecting the results of all studies analysing genome-wide methylation changes based on lifestyle interventions we identified 47 genes which were affected in two independent studies and even one gene which was reported by three studies – MAD1L1. Although the role of this cell cycle and tumor suppression relevant gene for obesity was not yet investigated, one recent study showed differently blood methylation of MAD1L1 in infants of obese mothers (109). Furthermore, DNA methylation of this genes in visceral adipose tissue may contribute to the discrimination of patients with and without colorectal cancer (110). In addition, two new studies reported DNA methylation changes on TCF7L2 which was previously reported by Aronica et al., and is a well-known candidate gene for T2D as identified by GWAS (111). The genetic risk variant was reported to alter mRNA expression levels and decrease insulin secretion in the pancreatic ß-cells (112). In line, the methylation status of TCF7L2 is altered in T2D islets (110) and was previously shown to be modified in adipose tissue under physical activity training (113).
Finally, we aligned those 47 candidates with those genes being potentially involved in weight loss response. Thereby, we could identify 518 genes. Among the five genes which were reported several times among all the studies was WDPCP, which is shown to be involved in ciliogenesis and collective cell movement during embryogenesis (114). To date, there are two human intervention studies, which described altered DNA methylation or gene expression in WDPCP (24, 31). The study by Salas-Perez et al. identified one DMP in the WDPCP body, which was hypomethylated (~5%) in responders compared to non-responders of the intervention. In line with this, Bollipalli et al. found a downregulation of the WDPCP mRNA expression in SAT of patients who successfully lost weight one year after intervention, but no corresponding change in DNA methylation for WDPCP was found in this study (31). However, Keller et al. also found a change in DNA methylation of WDPCP, but this did not survive multiple testing (23). Both studies included healthy participants with overweight and intervened with a hypocaloric diet. However, the study by Bollepalli et al. additionally included physical activity and the duration of the intervention varied from four months to one year. In contrast, the gene was not previously reported by Aronica et al.
Candidate gene methylation changes
In comparison to the genome-wide studies, no overlaps could be found between the candidate-gene studies. However, there are some overlaps with previous studies reported by Aronica et al. This includes the genes CLOCK, PER2 and FTO. Furthermore, overlaps between the genes TXNIP (39), CAV1 (40), FTO (42) and CRY (41) were recently identified in regard to weight loss response (23), as CpG sites among those genes were included in a weight loss prediction model.
Nevertheless, the lack of reproducible results even within comparable diet regimes can be explained by differences in studies regarding factors like gender distributions, ethnicity, BMI, sample size or duration of intervention. For example, the number of participants varied from 8 to 672 in the genome-wide studies and between 18 and 811 in candidate gene approaches. Furthermore, presence of comorbidities has been differently handled and reported, and specific medications were poorly addressed within downstream analyses.
However, weight loss is a dynamic process, and the underlying physiological and potential epigenetic modifications are under permanent change. Thus, the duration of the intervention plays a major role for the results. Within the here reviewed publications intervention length ranged from two weeks to two years. Due to the large differences, DNA methylation was assessed at different stages of metabolic remodelling. This was further supported by the wide range of weight loss reported in the different studies. Furthermore, weight loss success was defined either by actual weight loss in kg, by reduction in BMI or by specific health improvements. This makes comparing weight loss success between the studies highly challenging (if not impossible) and not allowing to conduct a comprehensive meta-analysis.
Analysed tissue
Whole blood is the most commonly used biological material in genetic and epigenetic studies as it is easy to draw and often the only available source. Studies included in this review also predominantly used blood samples. However, blood is a mixture of different cell types with different methylation patterns. Deconvolution techniques, such as constrained projection/quadratic programming (CP/QP) (19) have been further developed in recent years with the goal to improve the accuracy of the cell composition estimates and to overcome potential technical differences between the platforms (115, 116). This has been exemplarily shown in the study by Salas et al, where the available reference library for the deconvolution of blood cell fractions using the EPIC array was expanded (14). Although statistically correction for cell type composition appears essential for this kind of data (117, 118), this was only done in four of 14 studies. Nevertheless, blood DNA methylation could serve as a biomarker for weight-change despite it may mirror target tissue methylation changes only partly, which clearly warrants similar analyses in metabolic relevant target tissues such as adipose tissue. Although Aronica et al. reviewed two interventional studies in AT, since 2017 only a few studies used AT to examine changes in DNA methylation associated with weight loss. Some of these studies were able to show that DNA methylation in adipose tissue changed significantly after diet and exercise intervention (8, 31, 34, 113, 119). However, adipose tissue also consists of several cell types and only 20-40 % of these are adipocytes. The rest are fibroblasts, preadipocytes, stem cells and immune cells and during weight loss, this cell composition changes, which may also affect DNA methylation (120). In summary, further studies aiming to measure the capacity of lifestyle changes on epigenetic remodelling in AT are required.
DNA methylation age
DNA methylation age, a newly established epigenetic measure, and thus not previously discussed, has received increasing attention in the last five years. Although DNA methylation is considered rather a stable epigenetic mark, the genome gains and loses methylation stochastically, whereas ageing seems to be associated with this epigenetic remodelling. Recent studies showed that higher diet quality is inversely associated with methylation-based measures of biological age and that a healthier lifestyle may play a major role in reducing biological age (121, 122). In line with this, weight loss interventions seem to reduce methylation age and the mAge acceleration which correspond to an improved metabolic and physical phenotype (Figure 2) (65, 66).
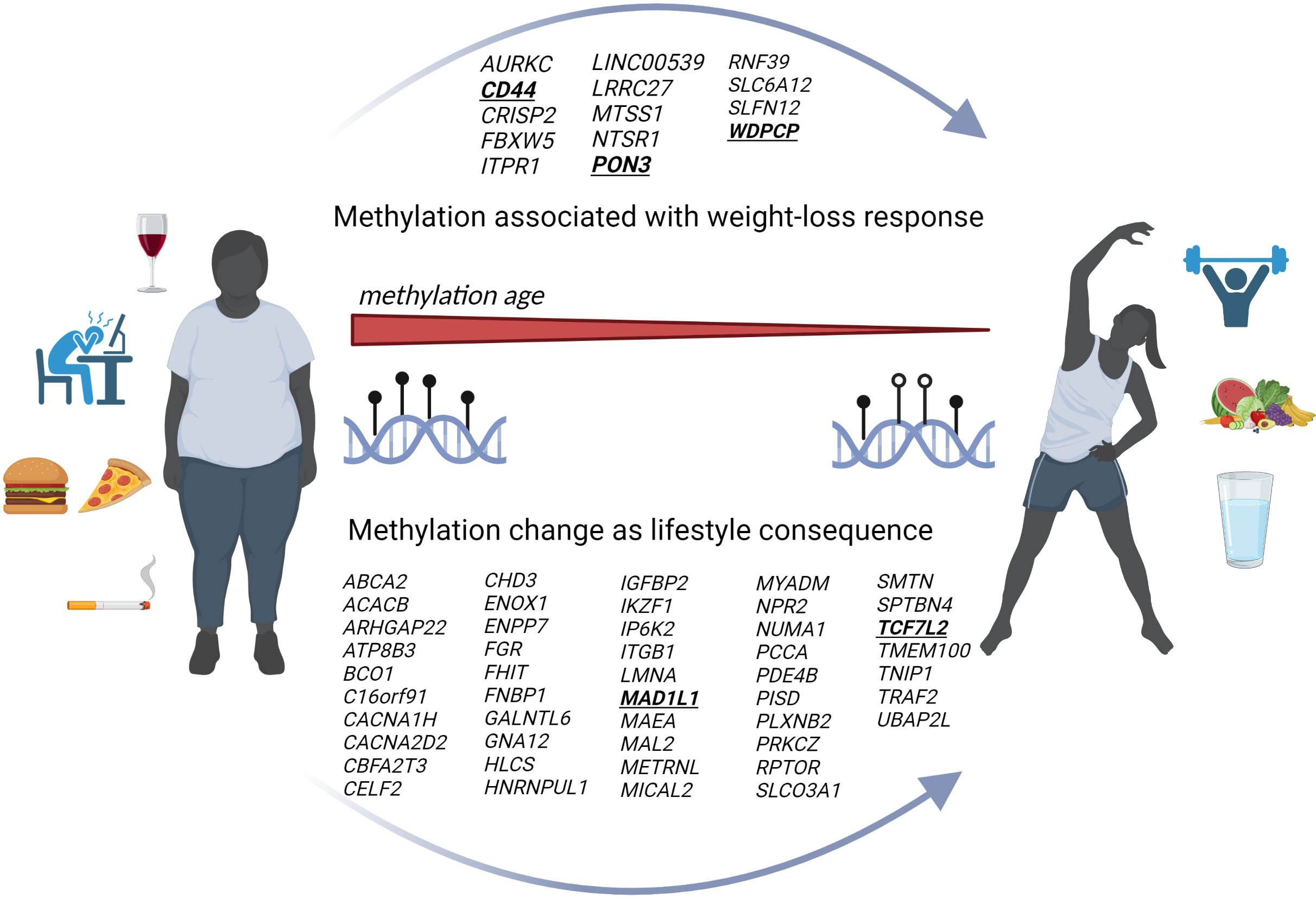
Figure 2 Genome-wide DNA methylation and lifestyle mediated weight loss. Scheme summarizes our results based on genome-wide DNA methylation during the previous five years. The figure indicates a reduced mAge which is associated with an improved lifestyle and weight loss. The upper arrow within the figure represents newly identified genes being associated with a weight loss success whereas the lower arrow shows all at least twice reported genes with changes on the methylome based on lifestyle modifications and weight loss. (Created with Biorender.com).
Smoking as important lifestyle contributor
Although known to be strongly associated with blood methylome profiles (123–132), smoking is another important cofactor that is mostly underreported in epigenetic studies related to obesity (133, 134). The well-established association of DNA methylation levels in AHRR (aryl hydrocarbon repressor repressor) and F2RL3 (coagulation factor II receptor‐like 3) genes with smoking (126, 130–132) appears particularly interesting in this context, since these two candidates were also identified in a study comparing blood methylome of subjects living a very healthy vs. a very unhealthy lifestyle based on a combined score derived from diet, physical activity, smoking and alcohol consumption (122). This further supports smoking to be a prominent lifestyle factor, potentially masking smaller effects derived from other components such as diet or physical activity (Figure 2). Unfortunately, most of the intervention studies have ignored the impact of smoking on DNA methylation so far, which is a clear limitation and could partially explain the lack of reproducibility in reported studies.
In-utero reprogramming
It is well-acknowledged that epigenetic changes could be passed onto the following generation (6, 89–91, 135) (Figure 2). To date, there is only limited research proving that transgenerational epigenetic inheritance exists in humans (6) and, moreover, explicit attempts to replicate the results of other studies have so far failed (96–98). The fact that epigenetic changes are more dynamic and reversible poses another challenge. However, further studies are needed to develop strategies for preventing obese phenotypes in the offspring, trying to control epigenetic (re)programming during early pregnancy and breastfeeding (136, 137). Early pregnancy appears to be the most vulnerable and important phase and, interestingly, cord blood CpG site methylation of the offspring seems to be more frequently altered in underweight (N=1621) than in obese mothers (N=28) (95). According to the study by Sharp et al., paternal BMI appeared to be of less important, then weight gain during pregnancy (95). However, we identified five genome-wide analyses that intervened with diet or a combination of diet and physical activity during pregnancy. Various genes demonstrated significantly altered DNA methylation after diet or physical activity interventions, such as DISC1, GBX2, HERC2, HUWE1 (89), LGR6 (90), SYN3, JARID2, POLR2C (91). Since JARID2 and LGR2 were also found in intervention studies aiming to identify DNA methylation biomarkers for response to weight loss, those for example might represent interesting candidates for further functional analyses. However, numerous further studies are needed to elucidate specific epigenetic fetal markers that are influenced by parental lifestyle (Table 4).
Future therapy options
Exploring causal epigenetic mechanisms in obesity could lead to novel treatments (138). There are already several pharmacological agents that affect DNA methylation and histone modifications, including DNMT inhibitors (e.g. Aza) and histone deacetylase inhibitors (HDACi, e.g. VPA and TSA). In addition, epigenetic drugs have already been tested for other diseases. This includes leukaemia, where Aza, BET and IDH1/2 inhibitors have been used in clinical trials (6, 113, 139, 140). Furthermore, some drugs such as metformin and statins, which are used to treat T2DM or lipid dysregulation in obesity, may affect epigenetic mechanisms (141–144). However, lifestyle interventions, including physical activity and a healthy diet, have been shown to be more effective than metformin in reducing the incidence of T2D in people at high risk (145), whereby lifestyle interventions continue to play an important role in elucidating epigenetic mechanisms and thus contributing to treatment options for obesity (Figure 2). On the other hand, DNA methylation marks could improve the success of weight loss therapies in the context of precision nutrition.
Limitations
Due to the numerous elaborated limitations and factors influencing DNA methylation profiles, the results of the reviewed studies can only be assigned to biological mechanisms with caution. First, knowledge of large, sophisticated epigenome-based risk scores for nutritional interventions, as has already been done for genotype-based interventions (146–150), is essential. The lack of replication does not allow a definitive conclusion on genotype-diet interactions in weight loss, thus requiring further studies with larger sample sizes (151). Because research on longitudinal epigenetic changes is still a quite underrepresented area, most data come from observational studies only, and data on histone modifications and chromatin remodelling tend to be lacking (152). In addition, compared to a stable genotype which can alter individual's physiology and behaviour without active intervention (153), this review indicates that epigenetic modification are not only cause but mostly consequence of a lifestyle intervention, complicating the development of bioinformatics tools for personalized nutrition.
On the other hand, the gut microbiome has previously been reported to interact with the host gene expression and play causal roles in the development of several diseases, including obesity (154, 155) and diabetes (156). The composition of the microbiome changes rapidly up to the age of three, increases until around the age of 40 and then remains fairly stable (157–159). However, short-term modulations of the gut microbiome can disrupt normal metabolite production (84) and this can lead to changes in host gene expression, which in turn could trigger longer-lasting effects in the host. In addition, it has already been shown that the human microbiome can also influence epigenetic modifications such as DNA methylation and histone acetylation (85, 160). It is possible that the response to specific diet and exercise interventions is influenced by the microbiome, which could explain the high variability in individual response. However, increasing number of individual nutritional studies are now incorporating microbiome data obtained from stool samples by 16S RNA sequencing, and thus accelerating information about the interaction of the microbiome and host gene expression (152). As mentioned, another limitation is that the studies analyzed mostly blood samples and further studies examining lifestyle effects on epigenetic remodelling in metabolic target organs such as adipose tissue, liver and muscle are inevitable. In regard to both, the limitation of target tissue access and the ability of blood methylome mirroring target tissue changes, circulating cell-free DNA reveals a high potential to full this gap and serve as a direct proxy of e.g. AT specific methylation changes. Circulating cfDNAs are short double stranded DNA fragments with low concentrations in blood, urine and other fluids (161) which are able to maintain characteristics of the tissue of origin such as methylation, nucleosome confirmations and mutations (162, 163), making cfDNA to biomarker for disease detection (164, 165). However, although recent findings clearly indicate that cfDNA might also be involved in disease progression driven by obesity-related tissue degeneration (166, 167) (including insulin resistance (168)), it is not yet commonly used as a biomarker in metabolic diseases. Altogether, a subsequent meta-analysis based on identical bioinformatic data processing would be required to analyse and summarise the results of the included studies. However, meta-analyses are rarely represented in this research field, which might be driven through the high diversity between the different intervention studies and heterogeneity of the study cohorts. To overcome this problem, all studies would have to meet a certain standard in order to be comparable. These standards include high sample sizes with equal gender distribution, long-term study durations, and random assignment to intervention groups, i.e. randomised controlled trials.
Conclusion
As demonstrated in the present review, the analysis of DNA methylation in a longitudinal design is very recent and the number of studies identified is excessively low to be able to draw relevant conclusions. Nevertheless, although the reviewed genome-wide studies are still lacking reproducibility, a number of candidate genes have been successfully validated. Recent studies on DNA methylation as weight loss predictor and mAge in relation to obesity state, further indicate its role and potential as an important biomarker for the improvement of obesity stratification and treatment.
Author contributions
All authors listed have made a substantial, direct, and intellectual contribution to the work and approved it for publication.
Funding
SA obtained a Pre-Doc award from the University of Leipzig.
Conflict of interest
The authors declare that the research was conducted in the absence of any commercial or financial relationships that could be construed as a potential conflict of interest.
Publisher’s note
All claims expressed in this article are solely those of the authors and do not necessarily represent those of their affiliated organizations, or those of the publisher, the editors and the reviewers. Any product that may be evaluated in this article, or claim that may be made by its manufacturer, is not guaranteed or endorsed by the publisher.
Glossary
References
1. Blüher M. Obesity: global epidemiology and pathogenesis. Nat Rev Endocrinol (2019) 15:288–98. doi: 10.1038/s41574-019-0176-8
2. Kaur Y, de SRJ, WT G, Meyre D. A systematic review of genetic syndromes with obesity. Obes Rev (2017) 18:603–34. doi: 10.1111/obr.12531
3. Seid H, Rosenbaum M. Low carbohydrate and low-fat diets: what we don't know and why we should know it. Nutrients (2019) 11(11):2749. doi: 10.3390/nu11112749
4. Bonora E, DeFronzo RA. Diabetes complications, comorbidities and related disorders Vol. 1. . Cham: Springer (2018).
5. Samblas M, Milagro FI, Martínez A. DNA methylation markers in obesity, metabolic syndrome, and weight loss. Epigenetics (2019) 14:421–44. doi: 10.1080/15592294.2019.1595297
6. Ling C, Rönn T. Epigenetics in human obesity and type 2 diabetes. Cell Metab (2019) 29:1028–44. doi: 10.1016/j.cmet.2019.03.009
7. Jones PA. Functions of DNA methylation: islands, start sites, gene bodies and beyond. Nature reviews. Genetics (2012) 13:484–92. doi: 10.1038/nrg3230
8. Aronica L, Levine AJ, Brennan K, Mi J, Gardner C, Haile RW, et al. A systematic review of studies of DNA methylation in the context of a weight loss intervention. Epigenomics (2017) 9:769–87. doi: 10.2217/epi-2016-0182
9. Drummond EM, Gibney ER. Epigenetic regulation in obesity. Curr Opin Clin Nutr Metab Care (2013) 16:392–7. doi: 10.1097/MCO.0b013e3283620f45
10. Wahl S, Drong A, Lehne B, Loh M, Scott WR, Kunze S, et al. Epigenome-wide association study of body mass index, and the adverse outcomes of adiposity. Nature (2017) 541:81–6. doi: 10.1038/nature20784
11. Barrero MJ, Cejas P, Long HW, Ramirez de Molina A. Nutritional epigenetics in cancer. Adv Nutr (Bethesda Md.) (2022) 13:1748–61. doi: 10.1093/advances/nmac039
12. Chakravarti R, Lenka SK, Gautam A, Singh R, RavichandIran V, Roy S, et al. A review on CRISPR-mediated epigenome editing: A future directive for therapeutic management of cancer. Curr Drug Targets (2022) 23:836–53. doi: 10.2174/1389450123666220117105531
13. Rakyan VK, Down TA, Balding DJ, Beck S. Epigenome-wide association studies for common human diseases. Nat Rev Genet (2011) 12:529–41. doi: 10.1038/nrg3000
14. Salas LA, Koestler DC, Butler RA, Hansen HM, Wiencke JK, Kelsey KT, et al. An optimized library for reference-based deconvolution of whole-blood biospecimens assayed using the Illumina HumanMethylationEPIC BeadArray. Genome Biol (2018) 19:64. doi: 10.1186/s13059-018-1448-7
15. Levenson VV. DNA methylation as a universal biomarker. Expert Rev Mol diagnostics (2010) 10:481–8. doi: 10.1586/erm.10.17
16. Houseman EA, Kim S, Kelsey KT, Wiencke JK. DNA methylation in whole blood: uses and challenges. Curr Environ Health Rep (2015) 2:145–54. doi: 10.1007/s40572-015-0050-3
17. Titus AJ, Gallimore RM, Salas LA, Christensen BC. Cell-type deconvolution from DNA methylation: a review of recent applications. Hum Mol Genet (2017) 26:R216–24. doi: 10.1093/hmg/ddx275
18. Teschendorff AE, Yang Z, Wong A, Pipinikas CP, Jiao Y, Jones A, et al. Correlation of smoking-associated DNA methylation changes in buccal cells with DNA methylation changes in epithelial cancer. JAMA Oncol (2015) 1:476–85. doi: 10.1001/jamaoncol.2015.1053
19. Houseman EA, AccOmando WP, Koestler DC, Christensen BC, Marsit CJ, Nelson HH, et al. DNA methylation arrays as surrogate measures of cell mixture distribution. BMC Bioinf (2012) 13:86. doi: 10.1186/1471-2105-13-86
20. Dick KJ, Nelson CP, Tsaprouni L, Sandling JK, Aïssi D, Wahl S, et al. DNA methylation and body-mass index: a genome-wide analysis. Lancet (London England) (2014) 383:1990–8. doi: 10.1016/S0140-6736(13)62674-4
21. Rönn T, Volkov P, Gillberg L, Kokosar M, Perfilyev A, Jacobsen AL, et al. Impact of age, BMI and HbA1c levels on the genome-wide DNA methylation and mRNA expression patterns in human adipose tissue and identification of epigenetic biomarkers in blood. Hum Mol Genet (2015) 24:3792–813. doi: 10.1093/hmg/ddv124
22. Crujeiras AB, Diaz-Lagares A, Sandoval J, Milagro FI, Navas-Carretero S, Carreira MC, et al. DNA methylation map in circulating leukocytes mirrors subcutaneous adipose tissue methylation pattern: a genome-wide analysis from non-obese and obese patients. Sci Rep (2017) 7:41903. doi: 10.1038/srep41903
23. Keller M, Yaskolka Meir A, Bernhart SH, Gepner Y, Shelef I, Schwarzfuchs D, et al. DNA methylation signature in blood mirrors successful weight-loss during lifestyle interventions: the CENTRAL trial. Genome Med (2020) 12:97. doi: 10.1186/s13073-020-00794-7
24. Salas-Pérez F, Cuevas-Sierra A, Cuervo M, Goni L, Milagro FI, Martínez JA, et al. Differentially methylated regions (DMRs) in PON3 gene between responders and non-responders to a weight loss dietary intervention: a new tool for precision management of obesity. Epigenetics (2022) 17:81–92. doi: 10.1080/15592294.2021.1873629
25. Samblas M, Mansego ML, Zulet MA, Milagro FI, Martinez JA. An integrated transcriptomic and epigenomic analysis identifies CD44 gene as a potential biomarker for weight loss within an energy-restricted program. Eur J Nutr (2019) 58:1971–80. doi: 10.1007/s00394-018-1750-x
26. Hoffmann A, Meir AY, Hagemann T, Czechowski P, Müller L, Engelmann B, et al. A polyphenol-rich green Mediterranean diet enhances epigenetic regulatory potential: the DIRECT PLUS randomized controlled trial. Metabolism: Clin Exp (2023) 145:155594. doi: 10.1016/j.metabol.2023.155594
27. Kou M, Li X, Shao X, Grundberg E, Wang X, Ma H, et al. DNA methylation of birthweight-blood pressure genes and changes of blood pressure in response to weight-loss diets in the POUNDS lost trial. Hypertension (2023) 80:1223–30. doi: 10.1161/HYPERTENSIONAHA.123.20864
28. Dye CK, Corley MJ, Ing C, Lum-Jones A, Li D, Mau, et al. Shifts in the immunoepigenomic landscape of monocytes in response to a diabetes-specific social support intervention: a pilot study among Native Hawaiian adults with diabetes. Clin Epigenet (2022) 14:91. doi: 10.1186/s13148-022-01307-6
29. Crujeiras AB, Izquierdo AG, Primo D, Milagro FI, Sajoux I, Jácome A, et al. Epigenetic landscape in blood leukocytes following ketosis and weight loss induced by a very low calorie ketogenic diet (VLCKD) in patients with obesity. Clin Nutr (Edinburgh Scotland) (2021) 40:3959–72. doi: 10.1016/j.clnu.2021.05.010
30. Nicoletti CF, Nonino CB, de Oliveira BA, Pinhel MA, Mansego ML, Milagro FI, et al. DNA methylation and hydroxymethylation levels in relation to two weight loss strategies: energy-restricted diet or bariatric surgery. Obes Surg (2016) 26:603–11. doi: 10.1007/s11695-015-1802-8
31. Bollepalli S, Kaye S, Heinonen S, Kaprio J, Rissanen A, Virtanen KA, et al. Subcutaneous adipose tissue gene expression and DNA methylation respond to both short- and long-term weight loss. Int J Obes (2005) (2018) 42:412–23. doi: 10.1038/ijo.2017.245
32. Hibler E, Huang L, Andrade J, Spring B. Impact of a diet and activity health promotion intervention on regional patterns of DNA methylation. Clin Epigenet (2019) 11:133. doi: 10.1186/s13148-019-0707-0
33. Moran S, Arribas C, Esteller M. Validation of a DNA methylation microarray for 850,000 CpG sites of the human genome enriched in enhancer sequences. Epigenomics (2016) 8:389–99. doi: 10.2217/epi.15.114
34. Izquierdo AG, Carreira MC, Boughanem H, Moreno-Navarrete JM, Nicoletti CF, Oliver P, et al. Adipose tissue and blood leukocytes ACE2 DNA methylation in obesity and after weight loss. Eur J Clin Invest (2022) 52:e13685. doi: 10.1111/eci.13685
35. Cahill DP, Lengauer C, Yu J, Riggins GJ, Willson JK, Markowitz SD, et al. Mutations of mitotic checkpoint genes in human cancers. Nature (1998) 392:300–3. doi: 10.1038/32688
36. Willmer T, Oosthuizen A, Dias S, Mendham AE, Goedecke JH, Pheiffer C. A pilot investigation of genetic and epigenetic variation of FKBP5 and response to exercise intervention in African women with obesity. Sci Rep (2022) 12:11771. doi: 10.1038/s41598-022-15678-6
37. Li X, Shao X, Xue Q, Kou M, Champagne CM, Koseva BS, et al. DNA methylation near CPT1A and changes in triglyceride-rich lipoproteins in response to weight-loss diet interventions. J Clin Endocrinol Metab (2023) 108(8):e542–9. doi: 10.1210/clinem/dgad086
38. Sun D, Heianza Y, Li X, Shang X, Smith SR, Bray GA, et al. Genetic, epigenetic and transcriptional variations at NFATC2IP locus with weight loss in response to diet interventions: The POUNDS Lost Trial. Diabetes Obes Metab (2018) 20:2298–303. doi: 10.1111/dom.13333
39. Li X, Shao X, Bazzano LA, Xue Q, Koseva BS, Grundberg E, et al. Blood DNA methylation at TXNIP and glycemic changes in response to weight-loss diet interventions: the POUNDS lost trial. Int J Obes (2022) 46:1122–7. doi: 10.1038/s41366-022-01084-5
40. Fachim HA, Siddals K, Malipatil N, Donn RP, Moreno GY, Dalton CF, et al. Lifestyle intervention in individuals with impaired glucose regulation affects Caveolin-1 expression and DNA methylation. Adipocyte (2020) 9:96–107. doi: 10.1080/21623945.2020.1732513
41. Rigamonti AE, Bollati V, Favero C, Albetti B, Caroli D, de CA, et al. Changes in DNA methylation of clock genes in obese adolescents after a short-term body weight reduction program: A possible metabolic and endocrine chrono-resynchronization. Int J Environ Res Public Health (2022) 19(23):15492. doi: 10.3390/ijerph192315492
42. Nishida H, Onishi K, Kurose S, Tsutsumi H, Miyauchi T, Takao N, et al. Changes in body composition and FTO whole blood DNA methylation among Japanese women: A randomized clinical trial of weight-loss program. Diabetes Metab syndrome Obes Targets Ther (2020) 13:2157–67. doi: 10.2147/DMSO.S248769
43. Milagro FI, Gómez-Abellán P, Campión J, Martínez JA, Ordovás JM, Garaulet M. CLOCK. PER2 and BMAL1 DNA methylation: association with obesity and metabolic syndrome characteristics and monounsaturated fat intake. Chronobiol Int (2012) 29:1180–94. doi: 10.3109/07420528.2012.719967
44. Reiter RJ, Tan D-X, Korkmaz A, Ma S. Obesity and metabolic syndrome: association with chronodisruption, sleep deprivation, and melatonin suppression. Ann Med (2012) 44:564–77. doi: 10.3109/07853890.2011.586365
45. McCaffery JM, Papandonatos GD, Peter I, Huggins GS, Raynor HA, Delahanty LM, et al. Obesity susceptibility loci and dietary intake in the Look AHEAD Trial. Am J Clin Nutr (2012) 95:1477–86. doi: 10.3945/ajcn.111.026955
46. Yang Q, Xiao T, Guo J, Su Z. Complex relationship between obesity and the fat mass and obesity locus. Int J Biol Sci (2017) 13:615–29. doi: 10.7150/ijbs.17051
47. van Otterdijk SD, Binder AM, Szarc Vel Szic K, Schwald J, Michels KB. DNA methylation of candidate genes in peripheral blood from patients with type 2 diabetes or the metabolic syndrome. PloS One (2017) 12:e0180955. doi: 10.1371/journal.pone.0180955
48. Church C, Moir L, McMurray F, Girard C, Banks GT, Teboul L, et al. Overexpression of Fto leads to increased food intake and results in obesity. Nat Genet (2010) 42:1086–92. doi: 10.1038/ng.713
49. Li M-D, Li C-M, Wang Z. The role of circadian clocks in metabolic disease. Yale J Biol Med (2012) 85:387–401.
50. Qian J, Caputo R, Morris CJ, Wang W, Scheer FA. 0041 Circadian misalignment increases the desire for food intake in chronic shift workers. Sleep (2018) 41:A17–7. doi: 10.1093/sleep/zsy061.040
51. Zimmet P, Alberti KG, Stern N, Bilu C, El-Osta A, Einat H, et al. The Circadian Syndrome: is the Metabolic Syndrome and much more! J Internal Med (2019) 286:181–91. doi: 10.1111/joim.12924
52. Jin K, Simpkins JW, Ji X, Leis M, Stambler I. The critical need to promote research of aging and aging-related diseases to improve health and longevity of the elderly population. Aging Dis (2015) 6:1–5. doi: 10.14336/AD.2014.1210
53. Sen P, Shah PP, Nativio R, Berger SL. Epigenetic mechanisms of longevity and aging. Cell (2016) 166:822–39. doi: 10.1016/j.cell.2016.07.050
54. van Eijk KR, de JS, MP B, Langeveld T, Colas F, Veldink JH, et al. Genetic analysis of DNA methylation and gene expression levels in whole blood of healthy human subjects. BMC Genomics (2012) 13:636. doi: 10.1186/1471-2164-13-636
55. Tammen SA, Friso S, Choi S-W. Epigenetics: the link between nature and nurture. Mol aspects Med (2013) 34:753–64. doi: 10.1016/j.mam.2012.07.018
56. Arpón A, Riezu-Boj JI, Milagro FI, Marti A, Razquin C, Martínez-González MA, et al. Adherence to Mediterranean diet is associated with methylation changes in inflammation-related genes in peripheral blood cells. J Physiol Biochem (2016) 73:445–55. doi: 10.1007/s13105-017-0552-6
57. López-Otín C, Blasco MA, Partridge L, Serrano M, Kroemer G. The hallmarks of aging. Cell (2013) 153:1194–217. doi: 10.1016/j.cell.2013.05.039
58. Horvath S, Raj K. DNA methylation-based biomarkers and the epigenetic clock theory of ageing. Nat Rev Genet (2018) 19:371–84. doi: 10.1038/s41576-018-0004-3
59. Nannini DR, Joyce BT, Zheng Y, Gao T, Liu L, Yoon G, et al. Epigenetic age acceleration and metabolic syndrome in the coronary artery risk development in young adults study. Clin Epigenet (2019) 11:160. doi: 10.1186/s13148-019-0767-1
60. Nevalainen T, Kananen L, Marttila S, Jylhävä J, Mononen N, Kähönen M, et al. Obesity accelerates epigenetic aging in middle-aged but not in elderly individuals. Clin Epigenet (2017) 9:20. doi: 10.1186/s13148-016-0301-7
61. Marioni RE, Shah S, McRae AF, Ritchie SJ, Muniz-Terrera G, Harris SE, et al. The epigenetic clock is correlated with physical and cognitive fitness in the Lothian Birth Cohort 1936. Int J Epidemiol (2015) 44:1388–96. doi: 10.1093/ije/dyu277
62. Zannas AS, Arloth J, Carrillo-Roa T, Iurato S, Röh S, Ressler KJ, et al. Lifetime stress accelerates epigenetic aging in an urban, African American cohort: relevance of glucocorticoid signaling. Genome Biol (2015) 16:266. doi: 10.1186/s13059-015-0828-5
63. Quach A, Levine ME, Tanaka T, Lu AT, Chen BH, Ferrucci L, et al. Epigenetic clock analysis of diet, exercise, education, and lifestyle factors. Aging (2017) 9:419–46. doi: 10.18632/aging.101168
64. Jylhävä J, Pedersen NL, Hägg S. Biological age predictors. EBioMedicine (2017) 21:29–36. doi: 10.1016/j.ebiom.2017.03.046
65. Petersen CL, Christensen BC, Batsis JA. Weight management intervention identifies association of decreased DNA methylation age with improved functional age measures in older adults with obesity. Clin Epigenet (2021) 13:46. doi: 10.1186/s13148-021-01031-7
66. Yaskolka Meir A, Keller M, Bernhart SH, Rinott E, Tsaban G, Zelicha H, et al. Lifestyle weight-loss intervention may attenuate methylation aging: the CENTRAL MRI randomized controlled trial. Clin Epigenet (2021) 13:48. doi: 10.1186/s13148-021-01038-0
67. Lu AT, Quach A, Wilson JG, Reiner AP, Aviv A, Raj K, et al. DNA methylation GrimAge strongly predicts lifespan and healthspan. Aging (2019) 11:303–27. doi: 10.18632/aging.101684
68. Michael I. Goran. In: Childhood obesity: Causes, consequences, and intervention approaches, vol. 1. . Boca Raton: CRC Press (2016).
69. Gluckman PD, Hanson MA. Developmental and epigenetic pathways to obesity: an evolutionary-developmental perspective. Int J Obes (2008) 32(Suppl 7):S62–71. doi: 10.1038/ijo.2008.240
70. Berglind D, Müller P, Willmer M, Sinha I, Tynelius P, Näslund E, et al. Differential methylation in inflammation and type 2 diabetes genes in siblings born before and after maternal bariatric surgery. Obes (Silver Spring Md.) (2016) 24:250–61. doi: 10.1002/oby.21340
71. Relton CL, Groom A, St Pourcain B, Sayers AE, Swan DC, Embleton ND, et al. DNA methylation patterns in cord blood DNA and body size in childhood. PloS One (2012) 7:e31821. doi: 10.1371/journal.pone.0031821
72. Desai M, Jellyman JK, Ross MG. Epigenomics, gestational programming and risk of metabolic syndrome. Int J Obes (2015) 39:633–41. doi: 10.1038/ijo.2015.13
73. Morales E, Groom A, Lawlor DA, Relton CL. DNA methylation signatures in cord blood associated with maternal gestational weight gain: results from the ALSPAC cohort. BMC Res Notes (2014) 7:278. doi: 10.1186/1756-0500-7-278
74. Juvinao-Quintero DL, Marioni RE, Ochoa-Rosales C, Russ TC, Deary IJ, van Meurs JB, et al. DNA methylation of blood cells is associated with prevalent type 2 diabetes in a meta-analysis of four European cohorts. Clin Epigenet (2021) 13:40. doi: 10.1186/s13148-021-01027-3
75. Gabory A, Attig L, Junien C. Developmental programming and epigenetics. Am J Clin Nutr (2011) 94:1943S–52S. doi: 10.3945/ajcn.110.000927
76. Bekdash RA. Early life nutrition and mental health: the role of DNA methylation. Nutrients (2021) 13. doi: 10.3390/nu13093111
77. Vaag A, Poulsen P. Twins in metabolic and diabetes research: what do they tell us? Curr Opin Clin Nutr Metab Care (2007) 10:591–6. doi: 10.1097/MCO.0b013e3282ab9ea6
78. Bergvall N, Iliadou A, Johansson S, de Faire U, Kramer MS, Pawitan Y, et al. Genetic and shared environmental factors do not confound the association between birth weight and hypertension: a study among Swedish twins. Circulation (2007) 115:2931–8. doi: 10.1161/CIRCULATIONAHA.106.674812
79. Bäckhed F, Roswall J, Peng Y, Feng Q, Jia H, Kovatcheva-Datchary P, et al. Dynamics and stabilization of the human gut microbiome during the first year of life. Cell Host Microbe (2015) 17:690–703. doi: 10.1016/j.chom.2015.04.004
80. Dominguez-Bello MG, Costello EK, Contreras M, Magris M, Hidalgo G, Fierer N, et al. Delivery mode shapes the acquisition and structure of the initial microbiota across multiple body habitats in newborns. Proc Natl Acad Sci United States America (2010) 107:11971–5. doi: 10.1073/pnas.1002601107
81. Montoya-Williams D, Lemas DJ, Spiryda L, Patel K, O'nO C, Neu J, et al. The neonatal microbiome and its partial role in mediating the association between birth by cesarean section and adverse pediatric outcomes. Neonatology (2018) 114:103–11. doi: 10.1159/000487102
82. Papathoma E, Triga M, Fouzas S, Dimitriou G. Cesarean section delivery and development of food allergy and atopic dermatitis in early childhood. Pediatr Allergy Immunol (2016) 27:419–24. doi: 10.1111/pai.12552
83. Bäckhed F, Fraser CM, Ringel Y, Sanders ME, Sartor RB, Sherman PM, et al. Defining a healthy human gut microbiome: current concepts, future directions, and clinical applications. Cell Host Microbe (2012) 12:611–22. doi: 10.1016/j.chom.2012.10.012
84. Yoon MY, Yoon SS. Disruption of the gut ecosystem by antibiotics. Yonsei Med J (2018) 59:4–12. doi: 10.3349/ymj.2018.59.1.4
85. Yu D-H, Gadkari M, Zhou Q, Yu S, Gao N, Guan Y, et al. Postnatal epigenetic regulation of intestinal stem cells requires DNA methylation and is guided by the microbiome. Genome Biol (2015) 16:211. doi: 10.1186/s13059-015-0763-5
86. Hopkins SA, Artal R. The role of exercise in reducing the risks of gestational diabetes mellitus. Women's Health (London England) (2013) 9:569–81. doi: 10.2217/whe.13.52
87. Harrison AL, Shields N, Taylor NF, Frawley HC. Exercise improves glycaemic control in women diagnosed with gestational diabetes mellitus: a systematic review. J physiotherapy (2016) 62:188–96. doi: 10.1016/j.jphys.2016.08.003
88. Gaillard R, Steegers E, Duijts L, Felix J, Hofman A, Franco O, et al. Childhood cardiometabolic outcomes of maternal obesity during pregnancy: the generation R study. Hypertension (2014) 63(4):683–91. doi: 10.1161/HYPERTENSIONAHA.113.02671
89. Jönsson J, Renault KM, García-Calzón S, Perfilyev A, Estampador AC, Nørgaard K, et al. Lifestyle intervention in pregnant women with obesity impacts cord blood DNA methylation, which associates with body composition in the offspring. Diabetes (2021) 70:854–66. doi: 10.2337/db20-0487
90. Antoun E, Kitaba NT, Titcombe P, Dalrymple KV, Garratt ES, Barton SJ, et al. Maternal dysglycaemia, changes in the infant's epigenome modified with a diet and physical activity intervention in pregnancy: Secondary analysis of a randomised control trial. PloS Med (2020) 17:e1003229. doi: 10.1371/journal.pmed.1003229
91. Lecorguillé M, McAuliffe FM, Twomey PJ, Viljoen K, Mehegan J, Kelleher CC, et al. Maternal glycaemic and insulinemic status and newborn DNA methylation: findings in women with overweight and obesity. J Clin Endocrinol Metab (2022) 108:85–98. doi: 10.1210/clinem/dgac553
92. Louise J, Deussen AR, Koletzko B, Owens J, Saffery R, Dodd JM. Effect of an antenatal diet and lifestyle intervention and maternal BMI on cord blood DNA methylation in infants of overweight and obese women: The LIMIT Randomised Controlled Trial. PloS One (2022) 17:e0269723. doi: 10.1371/journal.pone.0269723
93. Jaffe AE, Irizarry RA. Accounting for cellular heterogeneity is critical in epigenome-wide association studies. Genome Biol (2014) 15:R31. doi: 10.1186/gb-2014-15-2-r31
94. Hoyo C, Fortner K, Murtha AP, Schildkraut JM, Soubry A, Demark-Wahnefried W, et al. Association of cord blood methylation fractions at imprinted insulin-like growth factor 2 (IGF2), plasma IGF2, and birth weight. Cancer causes control CCC (2012) 23:635–45. doi: 10.1007/s10552-012-9932-y
95. Sharp GC, Lawlor DA, Richmond RC, Fraser A, Simpkin A, Suderman M, et al. Maternal pre-pregnancy BMI and gestational weight gain, offspring DNA methylation and later offspring adiposity: findings from the Avon Longitudinal Study of Parents and Children. Int J Epidemiol (2015) 44:1288–304. doi: 10.1093/ije/dyv042
96. Sharp GC, Salas LA, Monnereau C, Allard C, Yousefi P, Everson TM, et al. Maternal BMI at the start of pregnancy and offspring epigenome-wide DNA methylation: findings from the pregnancy and childhood epigenetics (PACE) consortium. Hum Mol Genet (2017) 26:4067–85. doi: 10.1093/hmg/ddx290
97. Soubry A, Murphy SK, Wang F, Huang Z, Vidal AC, Fuemmeler BF, et al. Newborns of obese parents have altered DNA methylation patterns at imprinted genes. Int J Obes (2015) 39:650–7. doi: 10.1038/ijo.2013.193
98. Martin CL, Jima D, Sharp GC, McCullough LE, Park SS, Gowdy KM, et al. Maternal pre-pregnancy obesity, offspring cord blood DNA methylation, and offspring cardiometabolic health in early childhood: an epigenome-wide association study. Epigenetics (2019) 14:325–40. doi: 10.1080/15592294.2019.1581594
99. Rasmussen L, Knorr S, Antoniussen CS, Bruun JM, Ovesen PG, Fuglsang J, et al. The impact of lifestyle, diet and physical activity on epigenetic changes in the offspring-A systematic review. Nutrients (2021) 13. doi: 10.3390/nu13082821
100. Nasser S, Solé T, Vega N, Thomas T, Balcerczyk A, Strigini M, et al. Ketogenic diet administration to mice after a high-fat-diet regimen promotes weight loss, glycemic norMalization and induces adaptations of ketogenic pathways in liver and kidney. Mol Metab (2022) 65:101578. doi: 10.1016/j.molmet.2022.101578
101. Leung A, Trac C, Du J, Natarajan R, Schones DE. Persistent chromatin modifications induced by high fat diet. J Biol Chem (2016) 291:10446–55. doi: 10.1074/jbc.M115.711028
102. Li S, Tollefsbol TO. DNA methylation methods: Global DNA methylation and methylomic analyses. Methods (San Diego Calif) (2021) 187:28–43. doi: 10.1016/j.ymeth.2020.10.002
103. Kirchner H, Nylen C, Laber S, Barrès R, Yan J, Krook A, et al. Altered promoter methylation of PDK4, IL1 B, IL6, and TNF after Roux-en Y gastric bypass. Surg Obes related Dis (2014) 10:671–8. doi: 10.1016/j.soard.2013.12.019
104. Bertola A, Deveaux V, Bonnafous S, Rousseau D, Anty R, Wakkach A, et al. Elevated expression of osteopontin may be related to adipose tissue macrophage accumulation and liver steatosis in morbid obesity. Diabetes (2009) 58:125–33. doi: 10.2337/db08-0400
105. Liu LF, Kodama K, Wei K, Tolentino LL, Choi O, Engleman EG, et al. The receptor CD44 is associated with systemic insulin resistance and proinflammatory macrophages in human adipose tissue. Diabetologia (2015) 58:1579–86. doi: 10.1007/s00125-015-3603-y
106. Kodama K, Horikoshi M, Toda K, Yamada S, Hara K, Irie J, et al. Expression-based genome-wide association study links the receptor CD44 in adipose tissue with type 2 diabetes. Proc Natl Acad Sci United States America (2012) 109:7049–54. doi: 10.1073/pnas.1114513109
107. Milagro FI, Campión J, Cordero P, Goyenechea E, Gómez-Uriz AM, Abete I, et al. A dual epigenomic approach for the search of obesity biomarkers: DNA methylation in relation to diet-induced weight loss. FASEB J (2011) 25:1378–89. doi: 10.1096/fj.10-170365
108. Moleres A, Campión J, Milagro FI, Marcos A, Campoy C, Garagorri JM, et al. Differential DNA methylation patterns between high and low responders to a weight loss intervention in overweight or obese adolescents: the EVASYON study. FASEB J (2013) 27:2504–12. doi: 10.1096/fj.12-215566
109. Sasaki A, Murphy KE, Briollais L, McGowan PO, Matthews SG. DNA methylation profiles in the blood of newborn term infants born to mothers with obesity. PloS One (2022) 17:e0267946. doi: 10.1371/journal.pone.0267946
110. Izquierdo AG, Boughanem H, Diaz-Lagares A, Arranz-Salas I, Esteller M, Tinahones FJ, et al. DNA methylome in visceral adipose tissue can discriminate patients with and without colorectal cancer. Epigenetics (2022) 17:665–76. doi: 10.1080/15592294.2021.1950991
111. Mahajan A, Taliun D, Thurner M, Robertson NR, Torres JM, Rayner NW, et al. Fine-mapping type 2 diabetes loci to single-variant resolution using high-density imputation and islet-specific epigenome maps. Nat Genet (2018) 50:1505–13. doi: 10.1038/s41588-018-0241-6
112. Lyssenko V, Lupi R, Marchetti P, Del Guerra S, Orho-Melander M, Almgren P, et al. Mechanisms by which common variants in the TCF7L2 gene increase risk of type 2 diabetes. J Clin Invest (2007) 117:2155–63. doi: 10.1172/JCI30706
113. Rönn T, Volkov P, Davegårdh C, Dayeh T, Hall E, Olsson AH, et al. A six months exercise intervention influences the genome-wide DNA methylation pattern in human adipose tissue. PloS Genet (2013) 9:e1003572. doi: 10.1371/journal.pgen.1003572
114. Kim SK, Shindo A, Park TJ, Oh EC, Ghosh S, Gray RS, et al. Planar cell polarity acts through septins to control collective cell movement and ciliogenesis. Sci (New York N.Y.) (2010) 329:1337–40. doi: 10.1126/science.1191184
115. Zhou W, Laird PW, Shen H. Comprehensive characterization, annotation and innovative use of Infinium DNA methylation BeadChip probes. Nucleic Acids Res (2017) 45:e22. doi: 10.1093/nar/gkw967
116. Logue MW, Smith AK, Wolf EJ, Maniates H, Stone A, Schichman SA, et al. The correlation of methylation levels measured using Illumina 450K and EPIC BeadChips in blood samples. Epigenomics (2017) 9:1363–71. doi: 10.2217/epi-2017-0078
117. Heijmans BT, Mill J. Commentary: The seven plagues of epigenetic epidemiology. Int J Epidemiol (2012) 41:74–8. doi: 10.1093/ije/dyr225
118. Koestler DC, Christensen B, Karagas MR, Marsit CJ, Langevin SM, Kelsey KT, et al. Blood-based profiles of DNA methylation predict the underlying distribution of cell types: a validation analysis. Epigenetics (2013) 8:816–26. doi: 10.4161/epi.25430
119. Bouchard L, Rabasa-Lhoret R, Faraj M, Lavoie M-E, Mill J, Pérusse L, et al. Differential epigenomic and transcriptomic responses in subcutaneous adipose tissue between low and high responders to caloric restriction. Am J Clin Nutr (2010) 91:309–20. doi: 10.3945/ajcn.2009.28085
120. Birney E, Smith GD, Greally JM. Epigenome-wide association studies and the interpretation of disease -omics. PloS Genet (2016) 12:e1006105. doi: 10.1371/journal.pgen.1006105
121. Kresovich JK, Park Y-MM, Keller JA, Sandler DP, Taylor JA. Healthy eating patterns and epigenetic measures of biological age. Am J Clin Nutr (2022) 115:171–9. doi: 10.1093/ajcn/nqab307
122. Klemp I, Hoffmann A, Müller L, Hagemann T, Horn K, Rohde-Zimmermann K, et al. DNA methylation patterns reflect individual's lifestyle independent of obesity. Clin Trans Med (2022) 12:e851. doi: 10.1002/ctm2.851
123. Elliott HR, Tillin T, McArdle WL, Ho K, Duggirala A, Frayling TM, et al. Differences in smoking associated DNA methylation patterns in South Asians and Europeans. Clin Epigenet (2014) 6:4. doi: 10.1186/1868-7083-6-4
124. Tsaprouni LG, Yang T-P, Bell J, Dick KJ, Kanoni S, Nisbet J, et al. Cigarette smoking reduces DNA methylation levels at multiple genomic loci but the effect is partially reversible upon cessation. Epigenetics (2014) 9:1382–96. doi: 10.4161/15592294.2014.969637
125. Joehanes R, Just AC, Marioni RE, Pilling LC, Reynolds LM, Mandaviya PR, et al. Epigenetic signatures of cigarette smoking. Circulation Cardiovasc Genet (2016) 9:436–47. doi: 10.1161/CIRCGENETICS.116.001506
126. Ambatipudi S, Cuenin C, Hernandez-Vargas H, Ghantous A, Le Calvez-Kelm F, Kaaks R, et al. Tobacco smoking-associated genome-wide DNA methylation changes in the EPIC study. Epigenomics (2016) 8:599–618. doi: 10.2217/epi-2016-0001
127. Gao X, Thomsen H, Zhang Y, Breitling LP, Brenner H. The impact of methylation quantitative trait loci (mQTLs) on active smoking-related DNA methylation changes. Clin Epigenet (2017) 9:87. doi: 10.1186/s13148-017-0387-6
128. Zeilinger S, Kühnel B, Klopp N, Baurecht H, Kleinschmidt A, Gieger C, et al. Tobacco smoking leads to extensive genome-wide changes in DNA methylation. PloS One (2013) 8:e63812. doi: 10.1371/journal.pone.0063812
129. Shenker NS, Ueland PM, Polidoro S, van Veldhoven K, Ricceri F, Brown R, et al. DNA methylation as a long-term biomarker of exposure to tobacco smoke. Epidemiol (Cambridge Mass) (2013) 24:712–6. doi: 10.1097/EDE.0b013e31829d5cb3
130. Philibert R, Hollenbeck N, Andersen E, McElroy S, Wilson S, Vercande K, et al. Reversion of AHRR demethylation is a quantitative biomarker of smoking cessation. Front Psychiatry (2016) 7:55. doi: 10.3389/fpsyt.2016.00055
131. Philibert R, Hollenbeck N, Andersen E, Osborn T, Gerrard M, Gibbons FX, et al. A quantitative epigenetic approach for the assessment of cigarette consumption. Front Psychol (2015) 6:656. doi: 10.3389/fpsyg.2015.00656
132. Zhang Y, Yang R, Burwinkel B, Breitling LP, Brenner H. F2RL3 methylation as a biomarker of current and lifetime smoking exposures. Environ Health Perspect (2014) 122:131–7. doi: 10.1289/ehp.1306937
133. Gupta R, van Dongen J, Fu Y, Abdellaoui A, Tyndale RF, Velagapudi V, et al. Epigenome-wide association study of serum cotinine in current smokers reveals novel genetically driven loci. Clin Epigenet (2019) 11:1. doi: 10.1186/s13148-018-0606-9
134. Loukola A, Buchwald J, Gupta R, Palviainen T, Hällfors J, Tikkanen E, et al. A genome-wide association study of a biomarker of nicotine metabolism. PloS Genet (2015) 11:e1005498. doi: 10.1371/journal.pgen.1005498
135. Geraghty AA, Sexton-Oates A, O'Brien EC, Alberdi G, Fransquet P, Saffery R, et al. A low glycaemic index diet in pregnancy induces DNA methylation variation in blood of newborns: results from the ROLO randomised controlled trial. Nutrients (2018) 10. doi: 10.3390/nu10040455
136. Cordero P, Milagro FI, Campion J, Martinez JA. Supplementation with methyl donors during lactation to high-fat-sucrose-fed dams protects offspring against liver fat accumulation when consuming an obesogenic diet. J Dev origins Health Dis (2014) 5:385–95. doi: 10.1017/S204017441400035X
137. Pauwels S, Ghosh M, Duca RC, Bekaert B, Freson K, Huybrechts I, et al. Maternal intake of methyl-group donors affects DNA methylation of metabolic genes in infants. Clin Epigenet (2017) 9:16. doi: 10.1186/s13148-017-0321-y
138. Liu XS, Wu H, Ji X, Stelzer Y, Wu X, Czauderna S, et al. Editing DNA methylation in the mamMalian genome. Cell (2016) 167:233–247.e17. doi: 10.1016/j.cell.2016.08.056
139. Lenoir O, Flosseau K, Ma FX, Blondeau B, Mai A, Bassel-Duby R, et al. Specific control of pancreatic endocrine β- and δ-cell mass by class IIa histone deacetylases HDAC4, HDAC5, and HDAC9. Diabetes (2011) 60:2861–71. doi: 10.2337/db11-0440
140. Mihaylova MM, Vasquez DS, Ravnskjaer K, Denechaud P-D, Yu RT, Alvarez JG, et al. Class IIa histone deacetylases are hormone-activated regulators of FOXO and mamMalian glucose homeostasis. Cell (2011) 145:607–21. doi: 10.1016/j.cell.2011.03.043
141. Schrader S, Perfilyev A, Martinell M, García-Calzón S, Ling C. Statin therapy is associated with epigenetic modifications in individuals with Type 2 diabetes. Epigenomics (2021) 13:919–25. doi: 10.2217/epi-2020-0442
142. Ochoa-Rosales C, Portilla-Fernandez E, Nano J, Wilson R, Lehne B, Mishra PP, et al. Epigenetic link between statin therapy and type 2 diabetes. Diabetes Care (2020) 43:875–84. doi: 10.2337/dc19-1828
143. Allen SC, Mamotte CD. Pleiotropic and adverse effects of statins-do epigenetics play a role? J Pharmacol Exp Ther (2017) 362:319–26. doi: 10.1124/jpet.117.242081
144. Bridgeman SC, Ellison GC, Melton PE, Newsholme P, Mamotte CD. Epigenetic effects of metformin: From molecular mechanisms to clinical implications. Diabetes Obes Metab (2018) 20:1553–62. doi: 10.1111/dom.13262
145. Knowler WC, Barrett-Connor E, Fowler SE, Hamman RF, Lachin JM, Walker EA, et al. Reduction in the incidence of type 2 diabetes with lifestyle intervention or metformin. New Engl J Med (2002) 346:393–403. doi: 10.1056/NEJMoa012512
146. Roke K, Walton K, Klingel SL, Harnett A, Subedi S, Haines J, et al. Evaluating changes in omega-3 fatty acid intake after receiving personal FADS1 genetic information: A randomized nutrigenetic intervention. Nutrients (2017) 9. doi: 10.3390/nu9030240
147. Gardner CD, Trepanowski JF, Del Gobbo LC, Hauser ME, Rigdon J, Ioannidis JP, et al. Effect of low-fat vs low-carbohydrate diet on 12-month weight loss in overweight adults and the association with genotype pattern or insulin secretion: the DIETFITS randomized clinical trial. JAMA (2018) 319:667–79. doi: 10.1001/jama.2018.0245
148. Celis-Morales C, Livingstone KM, Marsaux CF, Macready AL, Fallaize R, O'Donovan CB, et al. Effect of personalized nutrition on health-related behaviour change: evidence from the Food4Me European randomized controlled trial. Int J Epidemiol (2017) 46:578–88. doi: 10.1093/ije/dyw186
149. Grau K, Cauchi S, Holst C, Astrup A, Martinez JA, Saris WH, et al. TCF7L2 rs7903146-macronutrient interaction in obese individuals' responses to a 10-wk randomized hypoenergetic diet. Am J Clin Nutr (2010) 91:472–9. doi: 10.3945/ajcn.2009.27947
150. Larsen TM, Dalskov S-M, van Baak M, Jebb SA, Papadaki A, Pfeiffer AF, et al. Diets with high or low protein content and glycemic index for weight-loss maintenance. New Engl J Med (2010) 363:2102–13. doi: 10.1056/nejmoa1007137
151. Bayer S, Winkler V, Hauner H, Holzapfel C. Associations between genotype-diet interactions and weight loss-A systematic review. Nutrients (2020) 12. doi: 10.3390/nu12092891
152. Holzapfel C, Waldenberger M, Lorkowski S, Daniel H. Genetics and epigenetics in personalized nutrition: evidence, expectations, and experiences. Mol Nutr Food Res (2022) 66:e2200077. doi: 10.1002/mnfr.202200077
153. Turnwald BP, Goyer JP, Boles DZ, Silder A, Delp SL, Crum AJ. Learning one's genetic risk changes physiology independent of actual genetic risk. Nat Hum Behav (2019) 3:48–56. doi: 10.1038/s41562-018-0483-4
154. Ridaura VK, Faith JJ, Rey FE, Cheng J, Duncan AE, Kau AL, et al. Gut microbiota from twins discordant for obesity modulate metabolism in mice. Sci (New York N.Y.) (2013) 341:1241214. doi: 10.1126/science.1241214
155. Turnbaugh PJ, Ley RE, Mahowald MA, Magrini V, Mardis ER, Gordon JI. An obesity-associated gut microbiome with increased capacity for energy harvest. Nature (2006) 444:1027–31. doi: 10.1038/nature05414
156. Wen L, Ley RE, Volchkov PY, Stranges PB, Avanesyan L, Stonebraker AC, et al. Innate immunity and intestinal microbiota in the development of Type 1 diabetes. Nature (2008) 455:1109–13. doi: 10.1038/nature07336
157. de La Cuesta-Zuluaga J, Kelley ST, Chen Y, Escobar JS, Mueller NT, Ley RE, et al. Age- and sex-dependent patterns of gut microbial diversity in human adults. mSystems (2019) 4(4):e00261–19. doi: 10.1128/msystems.00261-19
158. Yadav D, Ghosh TS, Mande SS. Global investigation of composition and interaction networks in gut microbiomes of individuals belonging to diverse geographies and age-groups. Gut Pathog (2016) 8:17. doi: 10.1186/s13099-016-0099-z
159. Yatsunenko T, Rey FE, Manary MJ, Trehan I, Dominguez-Bello MG, Contreras M, et al. Human gut microbiome viewed across age and geography. Nature (2012) 486:222–7. doi: 10.1038/nature11053
160. Krautkramer KA, Kreznar JH, ROmano KA, Vivas EI, Barrett-Wilt GA, Rabaglia ME, et al. Diet-microbiota interactions mediate global epigenetic programming in multiple host tissues. Mol Cell (2016) 64:982–92. doi: 10.1016/j.molcel.2016.10.025
161. Chan AK, Chiu RW, Lo YM. Cell-free nucleic acids in plasma, serum and urine: a new tool in molecular diagnosis. Ann Clin Biochem (2003) 40:122–30. doi: 10.1258/000456303763046030
162. Snyder MW, Kircher M, Hill AJ, Daza RM, Shendure J. Cell-free DNA comprises an in vivo nucleosome footprint that informs its tissues-of-origin. Cell (2016) 164:57–68. doi: 10.1016/j.cell.2015.11.050
163. Erger F, Nörling D, Borchert D, Leenen E, Habbig S, Wiesener MS, et al. cfNOMe - A single assay for comprehensive epigenetic analyses of cell-free DNA. Genome Med (2020) 12:54. doi: 10.1186/s13073-020-00750-5
164. Che H, Villela D, Dimitriadou E, Melotte C, Brison N, Neofytou M, et al. Noninvasive prenatal diagnosis by genome-wide haplotyping of cell-free plasma DNA. Genet Med (2020) 22:962–73. doi: 10.1038/s41436-019-0748-y
165. Schwarzenbach H, Hoon DS, Pantel K. Cell-free nucleic acids as biomarkers in cancer patients. Nature reviews. Cancer (2011) 11:426–37. doi: 10.1038/nrc3066
166. Nishimoto S, Fukuda D, Higashikuni Y, Tanaka K, Hirata Y, Murata C, et al. Obesity-induced DNA released from adipocytes stimulates chronic adipose tissue inflammation and insulin resistance. Sci Adv (2016) 2:e1501332. doi: 10.1126/sciadv.1501332
167. Haghiac M, Vora NL, Basu S, Johnson KL, Presley L, Bianchi DW, et al. Increased death of adipose cells, a path to release cell-free DNA into systemic circulation of obese women. Obes (Silver Spring Md.) (2012) 20:2213–9. doi: 10.1038/oby.2012.138
Keywords: overweight, obesity, weight loss, lifestyle, intervention, DNA-methylation, epigenetics
Citation: Aurich S, Müller L, Kovacs P and Keller M (2023) Implication of DNA methylation during lifestyle mediated weight loss. Front. Endocrinol. 14:1181002. doi: 10.3389/fendo.2023.1181002
Received: 06 March 2023; Accepted: 18 July 2023;
Published: 08 August 2023.
Edited by:
Bruno Ramos-Molina, Biomedical Research Institute of Murcia (IMIB), SpainReviewed by:
Neil A. Youngson, University of New South Wales, AustraliaAna B. Crujeiras, Complejo Hospitalario Universitario de Santiago, Spain
Copyright © 2023 Aurich, Müller, Kovacs and Keller. This is an open-access article distributed under the terms of the Creative Commons Attribution License (CC BY). The use, distribution or reproduction in other forums is permitted, provided the original author(s) and the copyright owner(s) are credited and that the original publication in this journal is cited, in accordance with accepted academic practice. No use, distribution or reproduction is permitted which does not comply with these terms.
*Correspondence: Maria Keller, maria.keller@helmholtz-munich.de