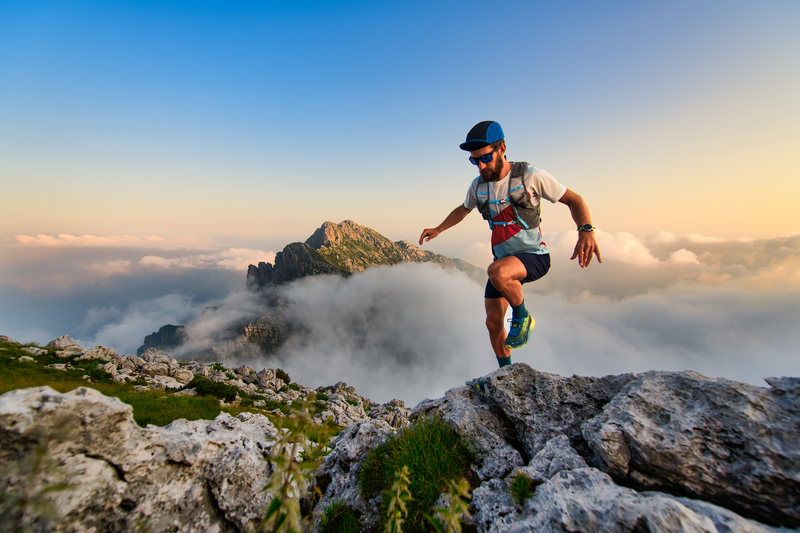
94% of researchers rate our articles as excellent or good
Learn more about the work of our research integrity team to safeguard the quality of each article we publish.
Find out more
ORIGINAL RESEARCH article
Front. Endocrinol. , 22 November 2023
Sec. Systems Endocrinology
Volume 14 - 2023 | https://doi.org/10.3389/fendo.2023.1165178
This article is part of the Research Topic Machine Learning-Assisted Diagnosis and Treatment of Endocrine-Related Diseases View all 13 articles
Objective: Acute ischemic stroke (AIS) brings an increasingly heavier economic burden nowadays. Prolonged length of stay (LOS) is a vital factor in healthcare expenditures. The aim of this study was to predict prolonged LOS in AIS patients based on an interpretable machine learning algorithm.
Methods: We enrolled AIS patients in our hospital from August 2017 to July 2019, and divided them into the “prolonged LOS” group and the “no prolonged LOS” group. Prolonged LOS was defined as hospitalization for more than 7 days. The least absolute shrinkage and selection operator (LASSO) regression was applied to reduce the dimensionality of the data. We compared the predictive capacity of extended LOS in eight different machine learning algorithms. SHapley Additive exPlanations (SHAP) values were used to interpret the outcome, and the most optimal model was assessed by discrimination, calibration, and clinical utility.
Results: Prolonged LOS developed in 149 (22.0%) of the 677 eligible patients. In eight machine learning algorithms, prolonged LOS was best predicted by the Gaussian naive Bayes (GNB) model, which had a striking area under the curve (AUC) of 0.878 ± 0.007 in the training set and 0.857 ± 0.039 in the validation set. The variables sorted by the gap values showed that the strongest predictors were pneumonia, dysphagia, thrombectomy, and stroke severity. High net benefits were observed at 0%–76% threshold probabilities, while good agreement was found between the observed and predicted probabilities.
Conclusions: The model using the GNB algorithm proved excellent for predicting prolonged LOS in AIS patients. This simple model of prolonged hospitalization could help adjust policies and better utilize resources.
With acute ischemic stroke (AIS) being the first leading cause of disability and the second leading cause of mortality worldwide, economic burden remains a prominent issue in clinical practice (1). Length of stay (LOS) is a vital factor of overwhelmed healthcare cost expenditures. Pellico-Lopez et al. (2) found that 15.8% of the total cost of stroke cases depended on the cost of prolonged stay. Reducing unnecessary hospital stays is important to relieve insurance stress, especially under the policy of diagnosis-related groups (DRGs) payment. Therefore, it is essential that the risk model of prolonged LOS be analyzed to relieve economic burden and optimize the discharge plan for patients with AIS.
The average LOS following stroke onset varied according to time and country. In the United States, the LOS for stroke hospitalizations decreased from 2004 to 2018, according to the data survey of 8 million stroke patients (unadjusted: 6.3 days in 2004 vs. 5.6 days in 2018; adjusted: 7.6 days in 2004 vs. 5.4 days in 2018) (3). A post-hoc analysis (4) based on information from multiple sources in China found that the median and IQR of LOS for AIS was 10.0 (7.0–13.0) days. Hao et al. (5) found that malnutrition estimated by the CONUT score on admission could increase LOS in elderly AIS patients. Moreover, Neale et al. (6) found that stroke patients receiving an early supported discharge model of care spent fewer days in hospital and incurred less cost. In addition, the mode of treatment could also be related to the LOS after a stroke. Intravenous tissue plasminogen activator (IV-tPA) was associated with an increase in LOS in stroke patients treated with endovascular treatment within 4.5 h (7).
Only a few articles have currently established risk models for predicting the length of hospital stay in stroke patients. Koton et al. (8) evaluated the performance of the prolonged length of stay (PLOS) score in the cohort of stroke, and concluded that the PLOS score could be clinically useful in different healthcare systems. However, they only included patients from 2002 to 2007, and the treatments for stroke have developed dramatically in recent years. Nowadays, artificial intelligence is able to deduce from voluminous datasets and to incorporate nonlinear interactions among a large set of predictors (9–11). For machine learning predicting prolonged LOS in AIS, Kurtz et al. (12) accurately predicted the LOS of patients admitted to the ICU with stroke through machine learning methods, but they did not include stroke-specific data, such as the National Institutes of Health Stroke Scale (NIHSS) score or neuroimaging findings. Yang et al. (13) found that the artificial neural network model achieved adequate discriminative power for predicting prolonged LOS after AIS and identified crucial factors associated with a prolonged hospital stay. However, they did not include pneumonia or another important onset symptom of stroke, which proved to be strong influencing factors of LOS in AIS patients.
As a result, we set out to gather extensive stroke-specific data and create a scientific risk model based on an interpretable machine learning algorithm to predict prolonged hospital LOS in AIS patients. This simple model of prolonged hospitalization could help adjust policies and better utilize resources.
This study continuously enrolled AIS patients who were admitted to the Department of Neurology at the Second Affiliated Hospital of Xuzhou Medical University between August 2017 and July 2019 (Figure 1). The inclusion criteria were as follows: (1) age ≥ 18 years; (2) a diagnosis of AIS (14, 15)and within 24 h of onset (16, 17). The exclusion criteria were as follows: (1) patients who needed to be transferred from one department (or hospital) to another; (2) patients who had in-hospital strokes; (3) patients who had transient ischemic attack; and (4) patients who were unable to extract complete data. This flowchart indicated that our hospital managed about a total of 1,354 patients from August 2017 and July 2019, of whom 745 (55%) AIS participants had complete data (Figure 1). Of these 745 patients, 68 patients were those who needed to be transferred from one department (or hospital) to another/those who had in-hospital strokes, leaving a final cohort of 677 patients. Retrospective review of medical health records for this study was approved by our Institutional Review Board. Owing to the retrospective nature of this study, written informed consent was waived (Number: 2020081603). Moreover, the Transparent Reporting of a Multivariable Prediction Model for Individual Prognosis or Diagnosis (TRIPOD) statements were followed for all data analysis and reporting (18).
Figure 1 Flowchart of inclusion and exclusion of study patients. This flowchart indicated that our hospital managed about total 1,354 patients from August 2017 and July 2019, of which 745 (55%) AIS participants had complete data (Figure 1). Of these 745 patients, 68 patients were those who needed to be transferred from one department (or hospital) to another/those who had in-hospital strokes, leaving a final cohort of 677 patients. Abbreviation: LOS, length of stay.
The primary outcome was the prediction of a prolonged LOS for AIS patients, which was defined as more than 7 days of hospitalization. The LOS was measured from the admission day to the death or discharge day. This definition was similar to previous studies on LOS in stroke patients (8, 19, 20). The main clinical data included the following categories: baseline demographics, clinical features, and laboratory data. For baseline demographics, systolic blood pressure (SBP) and diastolic blood pressure (DBP) were tested on the right hand and extracted from the nursing record sheet on admission. For clinical features, stroke severity was divided into “mild” (NIHSS score < 8) and “moderate to severe” (NIHSS score ≥9), which was similar to previous clinical trials (21–23). Sato et al. (22) found that the optimal cutoff score of the baseline NIHSS for the favorable outcome was 8 for patients with anterior circulation stroke (sensitivity, 80%; specificity, 82%). The pneumonia in our study referred to those with development of pneumonia within 72 h after hospitalization (24). We diagnosed pneumonia by the CDC criteria because it was the most commonly used (25). The dysphagia was defined as abnormal swallowing physiology of the upper aerodigestive tract and as detected from clinician testing including screening, clinical bedside, or instrumental tests (26). The thrombolysis, thrombectomy, antiplatelets, anticoagulation, statins, and proton pump inhibitors were also collected from medical records. Treatment methods for AIS were followed by the 2019 American Heart Association/American Stroke Association (AHA/ASA) guideline (27). For laboratory data, they were extracted from blood test results on admission.
Continuous data were presented as median and interquartile range (IQR), and the Mann–Whitney U-test was used for statistical comparison between two groups. Categorical data were described as proportions, and the chi-squared or Fisher’s exact test was used for comparison between two groups. The least absolute shrinkage and selection operator (LASSO) regression was applied to reduce the dimensionality of the data. In total, we utilized eight different machine learning algorithms, including the extreme gradient boosting (XGB) classifier, logistic regression, the light gradient boosting machine (LGBM) classifier, the AdaBoost classifier, Gaussian naive Bayes (GNB), complement naive Bayes (Complement NB), the multilayered perceptron (MLP) classifier, and the support vector (SVC) classifier. The hyperparameter settings for eight different machine learning algorithms used in our study are listed in Supplementary Table 1. For the XGB classifier, learning rate was set as 0.001, and the reg lambda was 0.01. Max depth and min child weight were set as 2. The area under the receiver operating characteristic (ROC) curve of the model was calculated by 10 bootstrapping resamples. For each bootstrap resample, the validation set (135 cases) accounted for 20% of the total sample, and the training set (542 cases) accounted for 80% of the total sample. After selecting the best model classifiers for this dataset, we exploited SHapley Additive exPlanations (SHAP) values to interpret the outcomes of the classifiers, which was a unified approach that connected cooperative game theory with local explanations to explain the output of any machine learning model. In addition, the decision curve analysis (DCA) was applied to present the net benefits at various threshold probabilities. A calibration plot was used to investigate the degree of agreement between two groups.
A total of 677 patients remained for evaluation of the machine learning algorithms to predict prolonged LOS in AIS patients, among whom prolonged LOS was detected in 22.0% (n = 149). The average of LOS in all 677 participants was 10.78 ± 4.69 days. The baseline and clinical characteristics between the two groups are compared in Table 1. Longer LOS was linked to elevated levels of brain natriuretic peptide (BNP), S100-β, and neuron-specific enolase (NSE). Moreover, the prolonged LOS group was more likely to suffer from dysphagia, pneumonia, and a moderate-to-severe stroke. As for treatment, the prolonged LOS group had more frequent use of thrombolysis, thrombectomy, anticoagulation, and proton pump inhibitors (PPIs). Then, least absolute shrinkage and selection operator (LASSO) regression was used to reduce the number of factors with an optimal λ of 0.002. The candidate characteristics were narrowed down to the following 28 features with nonzero coefficients: age, gender, diastolic blood pressure, anterior or posterior stroke, side of hemisphere, stroke lesion, single or multiple lesions, cholesterol, triglyceride, low-density lipoprotein (LDL), glycosylated hemoglobin (HbA1c), homocysteine (HCY), uric acid (UA), myoglobin (MB), and fibrinogen. The coefficients of characteristics selected by LASSO regression are illustrated in Figure 2.
Table 1 The baseline and clinical characteristics in prolonged LOS patients and no prolonged LOS patients.
Figure 2 The coefficients of characteristics selected by LASSO regression. LASSO, least absolute shrinkage and selection operator; SAP, stroke-associated pneumonia; SS, stroke severity; PPI, proton pump inhibitor; NSE, neuron-specific enolase; BNP, b-type natriuretic peptide; FIB, fibrinogen; MB, myoglobin; UA, uric acid; HCY, homocysteine; HbA1c, glycosylated hemoglobin; LDL, low-density lipoprotein; NOS, number of stroke lesions; SOS, site of stroke lesion; SOH, side of hemisphere; SD, stroke distribution; DBP, diastolic blood pressure.
As shown in Table 2, the GNB model with all characteristics had a striking AUROC of 0.878 ± 0.007 in the training set and 0.857 ± 0.039 in the validation set, while the other seven representative models had the highest AUROC of 0.875 ± 0.014 in the training set and 0.837 ± 0.031 in the validation set. For the GNB model, the sensitivities were 0.818 (training sets) and 0.804 (validation sets), while the specificities were 0.814 (training sets) and 0.816 (validation sets). The cross-reference between the full names and abbreviations in our manuscript is shown in Supplementary Table 2. The forest plot of each AUROC of eight models is depicted in Figure 3. Figures 4A, B present the comparison of AUROC between the GNB model and the other seven models, respectively, in the training and validation sets. The learning curve of the GNB model is displayed in Figure 5. Obviously, the GNB model significantly outperformed the other seven models in both the training and validation sets. Despite the narrow gap, De Long’s test showed that the difference between the GNB and XGB model remained significant (p = 0.04).
Figure 3 The forest plot of the each AUROC of eight models. AUROC, area under the receiver operating characteristic curve; XGB, extreme gradient boosting; LGBM, light gradient boosting machine; GNB, Gaussian naive Bayes; CNB, complement naive Bayes; MLP, multilayered perceptron; SVM, support vector machine.
Figure 4 The comparison of AUROC between the GNB model and the other seven models. (A) The comparison of AUROC between the GNB model and the other seven models in the training sets. (B) The comparison of AUROC between the GNB model and the other seven models in the validation sets. ROC, area under the receiver operating characteristic curve; XGB, extreme gradient boosting; LGBM, light gradient boosting machine; GNB, Gaussian naive Bayes; CNB, complement naive Bayes; MLP, multilayered perceptron; SVM, support vector machine.
The SHAP values for the GNB model and the importance of the variables sorted by the gap values are shown in Figures 6A, B. Red bars indicated an increase in the probability of prolonged LOS, whereas blue bars demonstrated a decrease in the probability of prolonged LOS for AIS patients. As Figure 6B shows, pneumonia, dysphagia, thrombectomy, and stroke severity all substantially increased the probability of prolonged LOS. In addition, we performed a decision curve analysis (Figure 7A) and a calibration plot (Figure 7B) to illustrate the performance of the GNB model. High net benefits could be observed in 0%–76% threshold probabilities, while good agreement could be found between the observed and predicted probabilities of prolonged LOS.
Figure 6 The SHAP values for the GNB model and the importance ranking of the variables. (A) The SHAP values for the GNB model. (B) The importance of the variables sorted by the gap values. SHAP, SHapley Additive exPlanations; GNB, Gaussian naive Bayes; SAP, stroke-associated pneumonia; SS, stroke severity; PPI, proton pump inhibitor; MB, myoglobin; NSE, neuron-specific enolase; BNP, b-type natriuretic peptide; FIB, fibrinogen; SOS, site of stroke lesion; HbA1c, glycosylated hemoglobin; DBP, diastolic blood pressure; LDL, low-density lipoprotein; NOS, number of stroke lesions.
Figure 7 The decision curve analysis and calibration plot to illustrate the performance of the GNB model. (A) The decision curve analysis for the GNB model. (B) Calibration plot for the GNB model. GNB, Gaussian naive Bayes.
This study generated a simple clinical risk model that can be used to determine patients at increased risk of prolonged LOS. Our risk model had a promising AUC of 0.878 and 0.857 in the training and validation sets, respectively. The main outcomes of the current study were that pneumonia, dysphagia, thrombectomy, and stroke severity were the strongest clinical parameters for prolonged LOS following AIS after recursive feature elimination. Moreover, the artificial intelligence algorithms developed by these parameters showed excellent model performance on discrimination, calibration, and decision curve analysis. The strengths of our clinical risk score included the use of simple demographic and common biochemical parameters, and we collected enough candidate variables to develop this model. To our knowledge, this is the first study to predict prolonged LOS for common AIS patients based on an interpretable machine learning algorithm. The difference from previous studies was that we developed an integrated machine learning model with high performance, which could help adjust the policies to better utilize resources, especially under the DRG payment policy and the increasingly serious aging problem in the global world.
Su et al. (28) included 129,444 patients with AIS and found that the inpatient cost was $1,020 ($742–$1,545) in China. In an attempt to decrease patients’ risk of prolonged LOS following AIS, previous retrospective studies have identified some factors. Many studies define prolonged LOS as more than 7 days (8, 19, 20). However, when it comes to patients with severe strokes or those admitted to an intensive care unit, some studies define it as more than 30 days (12, 29). Common factors affecting stroke hospitalization duration included quality of care, hospital-acquired infection, stroke severity and type, level of consciousness, history of heart failure and atrial fibrillation, and receiving reperfusion therapy (19, 29–33). Interestingly, during adolescence, low stress resilience, underweight, and higher systolic blood pressure were associated with longer hospital stays in AIS, with adjusted relative hazard ratios of 1.46, 1.41, and 1.01, respectively (34), whereas these prior studies did not show the weight of each parameter on the probability of prolonged LOS. An interpretable machine learning algorithm has the ability to analyze big datasets with high accuracy through automated analysis of non-linear relationships between numerous variables (35). Machine learning algorithms apply various statistical methods from past experience to select useful patterns in large and complex datasets, which involves extreme gradient boosting (XGB) classifier, GNB, SVC classifier, and so on (36). Raizada et al. (37) concluded the advantages and limitations of different algorithms and found that GNB produced results that were statistically robust and were replicates across two independent datasets. An additional advantage of GNB classifiers was that GNB produced an accuracy similar to more sophisticated classifiers but with a substantial gain in speed (38). Therefore, we selected the GNB model from eight different machine learning algorithms that showed excellent performance in predicting prolonged LOS in AIS patients.
In this study, pneumonia, dysphagia, thrombectomy, and stroke severity were the leading clinical parameters in our interpretable machine learning algorithm. Pneumonia is an early complication of stroke and usually leads to prolonged LOS. The prevalence of pneumonia in patients with dysphagia after stroke was reported to range from 7% to 33%, and the prevalence of dysphagia has been reported as between 28% and 65% (39, 40). Aspiration without a cough, known as “silent aspiration,” further increased the incidence of pneumonia to 54% (40). A systematic review of stroke-associated pneumonia reported that the overall incidence of pneumonia ranged from 0% to 23.6% (41), which was a little lower than the incidence in our study. In our study, the incidence of pneumonia in all participants is 24.37%. It may be because of the varied definitions and diagnosis criteria of stroke-associated pneumonia. The Centers for Disease Control and Prevention (CDC) criteria (25), the PISCES SAP diagnostic criteria (42), and the combination of the clinical symptoms and auxiliary examination results criteria were all used to diagnose stroke-associated pneumonia in previous studies (41). In our study, we diagnosed pneumonia by the CDC criteria because it was the most commonly used, using clinical (lung auscultation and percussion, presence of fever, and purulent tracheal secretion), microbiological (tracheal specimens and blood cultures), and chest radiography findings. For dysphagia, the incidence in all participants was 22.45%, while in the “prolonged LOS group”, it was 59.73%, and in the “no prolonged LOS group”, it was 11.93% (Table 1). The incidence of dysphagia varied greatly between studies (ranged from 20% to 80%), depending on the definition of dysphagia, which can range from failing a dysphagia screen, to prescribed diet modifications, to measures of physiology on an instrumented swallowing study (26, 41, 43). Ogawa et al. (40) found that patients who underwent a flexible endoscopic evaluation of swallowing and received optimal nutritional intervention were more likely to have a shorter hospital stay (p = 0.005). The complications of dysphagia include the consequences of modifications to dietary intake: compromised nutrition and hydration, prolonged LOS, and reduced quality of life. As a result, the optimal treatments and measures for dysphagia should be performed. Many studies have investigated a variety of interventions, including therapist-delivered, behavioral, acupuncture, and electrical or magnetic stimulation to treat dysphagia (39). As for stroke severity, it was the most consistent factor among the factors contributing to LOS in AIS patients, and those who received reperfusion therapy were more likely to have prolonged LOS, which was similar to the previous study (29). Patients with more severe strokes may require more intensive medical care, including medication treatment and rehabilitation. Thrombectomy is a procedure used to remove a blood clot from a blood vessel, and is typically used in the treatment of acute ischemic stroke. While thrombectomy can be effective in reducing the severity of stroke and improving patient outcomes, it is also a relatively invasive procedure that can carry some risks and complications. As a result, patients who undergo thrombectomy may require longer hospital stays than those who do not. In summary, both thrombectomy and stroke severity are independent risk factors for prolonged LOS following AIS.
Our study has several limitations. First, its retrospective study design and only including patients from one single tertiary central hospital may limit the generalizability of the machine learning algorithm in clinical practice. Second, owing to the availability of the data, we were not able to consider more detailed factors, such as specific steps of reperfusion therapy, infarction or penumbra volume, and the collateral circulation status. More valuable and dynamic predictors could improve the performance. Third, some special reasons that might affect hospitalization time, such as economic stress or medical disputes, were not analyzed. Fourth, the sample size and certain bias limited the predictive ability of the model. We just internally validated our interpretable machine learning algorithms by bootstrap resample and multi-center large-sample studies are warranted to verify this conclusion in the future.
We developed a model for predicting the prolonged LOS for AIS patients using the GNB algorithm. This model included 20 potential clinical factors and performed well in terms of discrimination, calibration, and clinical utility, but it needs to be validated in larger multicenter cohorts. In this model, pneumonia, dysphagia, thrombectomy, and stroke severity might be strong predictors of prolonged LOS. We explained these main variables and analyzed the effects of their changing trends on prolonged LOS. Timely prevention and intervention for complications, as well as high quality standard of care, may be prospects worthy of clinicians’ promising efforts.
The raw data supporting the conclusions of this article will be made available by the authors, without undue reservation.
The studies involving human participants were reviewed and approved by Ethics Committee of the Second Affiliated Hospital of Xuzhou Medical University. The patients/participants provided their written informed consent to participate in this study.
WL, LR, and HW completed the study design. KW and WL performed the study, and collected and analyzed the data. QJ and WL drafted the manuscript. LR, XW, KW, and HL provided the expert consultations and suggestions. MG, RG, CX, and CY conceived the study, participated in its design and coordination, and helped to embellish language. All authors contributed to the article and approved the submitted version.
This study was supported by: Scientific Research Project of Jiangsu Health Committee (No.H2019054), the Xuzhou Science and Technology Planning Project (No. KC21220) and Science and Technology Development Fund of Affiliated Hospital of Xuzhou Medical University (No.XYFY202250), Shaanxi Provincial Health and Health Research Fund Project (2022E006).
The authors declare that the research was conducted in the absence of any commercial or financial relationships that could be construed as a potential conflict of interest.
All claims expressed in this article are solely those of the authors and do not necessarily represent those of their affiliated organizations, or those of the publisher, the editors and the reviewers. Any product that may be evaluated in this article, or claim that may be made by its manufacturer, is not guaranteed or endorsed by the publisher.
The Supplementary Material for this article can be found online at: https://www.frontiersin.org/articles/10.3389/fendo.2023.1165178/full#supplementary-material
Supplementary Table 1 | The hyperparameter settings for eight different machine learning algorithms. Abbreviations: XGB, extreme gradient boosting; LGBM, light gradient boosting machine; GNB, Gaussian naive Bayes; CNB, complement naive Bayes; MLP, multilayered perceptron; SVM, support vector machine.
Supplementary Table 2 | The cross-reference between the full names and abbreviations in our manuscript.
1. Tsao CW, Aday AW, Almarzooq ZI, Alonso A, Beaton AZ, Bittencourt MS, et al. Heart disease and stroke statistics-2022 update: a report from the American heart association. Circulation. (2022) 145(8):e153–639. doi: 10.1161/CIR.0000000000001052
2. Pellico-Lopez A, Fernandez-Feito A, Cantarero D, Herrero-Montes M, Cayón-de Las Cuevas J, Parás-Bravo P, et al. Cost of stay and characteristics of patients with stroke and delayed discharge for non-clinical reasons. Sci Rep Jun 27 (2022) 12(1):10854. doi: 10.1038/s41598-022-14502-5
3. Salah HM, Minhas AMK, Khan MS, Khan SU, Ambrosy AP, Blumer V, et al. Trends in hospitalizations for heart failure, acute myocardial infarction, and stroke in the united states from 2004 to 2018. Am Heart J (2022) 243:103–9. doi: 10.1016/j.ahj.2021.09.009
4. Wang YJ, Li ZX, Gu HQ, Zhai Y, Zhou Q, Jiang Y, et al. China Stroke statistics: an update on the 2019 report from the national center for healthcare quality management in neurological diseases, China national clinical research center for neurological diseases, the Chinese stroke association, national center for chronic and non-communicable disease control and prevention, Chinese center for disease control and prevention and institute for global neuroscience and stroke collaborations. Stroke Vasc Neurol (2022) 7(5):415–50. doi: 10.1136/svn-2021-001374
5. Hao R, Qi X, Xia X, Wang L, Li X. Malnutrition on admission increases the in-hospital mortality and length of stay in elder adults with acute ischemic stroke. J Clin Lab Anal (2022) 36(1):e24132. doi: 10.1002/jcla.24132
6. Neale S, Leach K, Steinfort S, Hitch D. Costs and length of stay associated with early supported discharge for moderate and severe stroke survivors. J Stroke Cerebrovasc Dis (2020) 29(8):104996. doi: 10.1016/j.jstrokecerebrovasdis.2020.104996
7. Hassan AE, Ringheanu VM, Preston L, Tekle W, Qureshi AI. IV tPA is associated with increase in rates of intracerebral hemorrhage and length of stay in patients with acute stroke treated with endovascular treatment within 4.5 hours: should we bypass IV tPA in large vessel occlusion? J neurointerv Surg (2021) 13(2):114–8. doi: 10.1136/neurintsurg-2020-016045
8. Koton S, Luengo-Fernandez R, Mehta Z, Rothwell PM. Independent validation of the prolonged length of stay score. Neuroepidemiology. (2010) 35(4):263–6. doi: 10.1159/000320241
9. Hey T, Butler K, Jackson S, Thiyagalingam J. Machine learning and big scientific data. Philos Trans A Math Phys Eng Sci (2020) 378(2166):20190054. doi: 10.1098/rsta.2019.0054
10. Zhang W, Bao Z, Jiang S, He J. An artificial neural network-based algorithm for evaluation of fatigue crack propagation considering nonlinear damage accumulation. Materials (Basel). (2016) 9(6):484. doi: 10.3390/ma9060483
11. Kulkarni H, Thangam M, Amin AP. Artificial neural network-based prediction of prolonged length of stay and need for post-acute care in acute coronary syndrome patients undergoing percutaneous coronary intervention. Eur J Clin Invest. (2021) 51(3):e13406. doi: 10.1111/eci.13406
12. Kurtz P, Peres IT, Soares M, Salluh JIF, Bozza FA. Hospital length of stay and 30-day mortality prediction in stroke: a machine learning analysis of 17,000 ICU admissions in Brazil. Neurocrit Care (2022) 37(Suppl 2):313–21. doi: 10.1007/s12028-022-01486-3
13. Yang CC, Bamodu OA, Chan L, Chen JH, Hong CT, Huang YT, et al. Risk factor identification and prediction models for prolonged length of stay in hospital after acute ischemic stroke using artificial neural networks. Front Neurol (2023) 14:1085178. doi: 10.3389/fneur.2023.1085178
15. Powers WJ, Rabinstein AA, Ackerson T, Adeoye OM, Bambakidis NC, Becker K, et al. Guidelines for the early management of patients with acute ischemic stroke: 2019 update to the 2018 guidelines for the early management of acute ischemic stroke: a guideline for healthcare professionals from the American heart Association/American stroke association. Stroke. (2019) 50(12):e344–418. doi: 10.1161/STR.0000000000000211
16. Zivin JA, Sehra R, Shoshoo A, Albers GW, Bornstein NM, Dahlof B, et al. NeuroThera(R) efficacy and safety trial-3 (NEST-3): a double-blind, randomized, sham-controlled, parallel group, multicenter, pivotal study to assess the safety and efficacy of transcranial laser therapy with the NeuroThera(R) laser system for the treatment of acute ischemic stroke within 24 h of stroke onset. Int J Stroke. (2014) 9(7):950–5. doi: 10.1111/j.1747-4949.2012.00896.x
17. Renner CJ, Kasner SE, Bath PM, Bahouth MN, Committee VAS. Stroke outcome related to initial volume status and diuretic use. J Am Heart Assoc (2022) 11(24):e026903. doi: 10.1161/JAHA.122.026903
18. Collins GS, Reitsma JB, Altman DG, Moons KG. Transparent reporting of a multivariable prediction model for individual prognosis or diagnosis (TRIPOD). Ann Intern Med (2015) 162(10):735–6. doi: 10.7326/L15-5093-2
19. Koton S, Bornstein NM, Tsabari R, Tanne D, Investigators N. Derivation and validation of the prolonged length of stay score in acute stroke patients. Neurology. (2010) 74(19):1511–6. doi: 10.1212/WNL.0b013e3181dd4dc5
20. Saposnik G, Webster F, O'Callaghan C, Hachinski V. Optimizing discharge planning: clinical predictors of longer stay after recombinant tissue plasminogen activator for acute stroke. Stroke. (2005) 36(1):147–50. doi: 10.1161/01.STR.0000150492.12838.66
21. Yaghi S, Harik SI, Hinduja A, Bianchi N, Johnson DM, Keyrouz SG. Post t-PA transfer to hub improves outcome of moderate to severe ischemic stroke patients. J Telemed Telecare. (2015) 21(7):396–9. doi: 10.1177/1357633X15577531
22. Sato S, Toyoda K, Uehara T, Toratani N, Yokota C, Moriwaki H, et al. Baseline NIH stroke scale score predicting outcome in anterior and posterior circulation strokes. Neurology (2008) 70(24 Pt 2):2371–7. doi: 10.1212/01.wnl.0000304346.14354.0b
23. de Celis-Ruiz E, Fuentes B, Alonso de Lecinana M, Gutiérrez-Fernández M, Borobia AM, Gutiérrez-Zúñiga R, et al. Final results of allogeneic adipose tissue-derived mesenchymal stem cells in acute ischemic stroke (AMASCIS): a phase II, randomized, double-blind, placebo-controlled, single-center, pilot clinical trial. Cell Transplant. (2022) 31:9636897221083863. doi: 10.1177/09636897221083863
24. Maeshima S, Osawa A, Hayashi T, Tanahashi N. Elderly age, bilateral lesions, and severe neurological deficit are correlated with stroke-associated pneumonia. J Stroke Cerebrovasc Dis (2014) 23(3):484–9. doi: 10.1016/j.jstrokecerebrovasdis.2013.04.004
25. Garner JS, Jarvis WR, Emori TG, Horan TC, Hughes JM. CDC Definitions for nosocomial infections, 1988. Am J Infect Control. (1988) 16(3):128–40. doi: 10.1016/0196-6553(88)90053-3
26. Martino R, Foley N, Bhogal S, Diamant N, Speechley M, Teasell R. Dysphagia after stroke: incidence, diagnosis, and pulmonary complications. Stroke. (2005) 36(12):2756–63. doi: 10.1161/01.STR.0000190056.76543.eb
27. Warner JJ, Harrington RA, Sacco RL, Elkind MSV. Guidelines for the early management of patients with acute ischemic stroke: 2019 update to the 2018 guidelines for the early management of acute ischemic stroke. Stroke. (2019) 50(12):3331–2. doi: 10.1161/STROKEAHA.119.027708
28. Su M, Pan D, Zhao Y, Chen C, Wang X, Lu W, et al. The direct and indirect effects of length of hospital stay on the costs of inpatients with stroke in ningxia, China, between 2015 and 2020: a retrospective study using quantile regression and structural equation models. Front Public Health (2022) 10:881273. doi: 10.3389/fpubh.2022.881273
29. Lin KH, Lin HJ, Yeh PS. Determinants of prolonged length of hospital stay in patients with severe acute ischemic stroke. J Clin Med (2022) 11(12):3457. doi: 10.3390/jcm11123457
30. Svendsen ML, Ehlers LH, Andersen G, Johnsen SP. Quality of care and length of hospital stay among patients with stroke. Med Care (2009) 47(5):575–82. doi: 10.1097/MLR.0b013e318195f852
31. Borghans I, Hekkert KD, den Ouden L, Cihangir S, Vesseur J, Kool RB, et al. Unexpectedly long hospital stays as an indicator of risk of unsafe care: an exploratory study. BMJ Open (2014) 4(6):e004773. doi: 10.1136/bmjopen-2013-004773
32. Beckers V, De Smedt A, Van Hooff RJ, De Raedt S, Van Dyck R, Putman K, et al. Prediction of hospitalization duration for acute stroke in Belgium. Acta Neurol Belg. (2012) 112(1):19–25. doi: 10.1007/s13760-012-0026-0
33. Spratt N, Wang Y, Levi C, Ng K, Evans M, Fisher J. A prospective study of predictors of prolonged hospital stay and disability after stroke. J Clin Neurosci (2003) 10(6):665–9. doi: 10.1016/j.jocn.2002.12.001
34. Bergh C, Udumyan R, Appelros P, Fall K, Montgomery S. Determinants in adolescence of stroke-related hospital stay duration in men: a national cohort study. Stroke. (2016) 47(9):2416–8. doi: 10.1161/STROKEAHA.116.014265
35. Helm JM, Swiergosz AM, Haeberle HS, Karnuta JM, Schaffer JL, Krebs VE, et al. Machine learning and artificial intelligence: definitions, applications, and future directions. Curr Rev Musculoskelet Med (2020) 13(1):69–76. doi: 10.1007/s12178-020-09600-8
36. Uddin S, Khan A, Hossain ME, Moni MA. Comparing different supervised machine learning algorithms for disease prediction. BMC Med Inform Decis Mak. (2019) 19(1):281. doi: 10.1186/s12911-019-1004-8
37. Raizada RD, Lee YS. Smoothness without smoothing: why Gaussian naive bayes is not naive for multi-subject searchlight studies. PloS One (2013) 8(7):e69566. doi: 10.1371/journal.pone.0069566
38. Ontivero-Ortega M, Lage-Castellanos A, Valente G, Goebel R, Valdes-Sosa M. Fast Gaussian naive bayes for searchlight classification analysis. Neuroimage. (2017) 163:471–9. doi: 10.1016/j.neuroimage.2017.09.001
39. Cohen DL, Roffe C, Beavan J, Blackett B, Fairfield CA, Hamdy S, et al. Post-stroke dysphagia: a review and design considerations for future trials. Int J Stroke. (2016) 11(4):399–411. doi: 10.1177/1747493016639057
40. Ogawa Y, Inagawa M, Kimura M, Iida T, Hirai A, Yoshida T, et al. Nutritional intervention after an early assessment by a flexible endoscopic evaluation of swallowing is associated with a shorter hospital stay for patients with acute cerebral infarction: a retrospective study. Asia Pac J Clin Nutr (2021) 30(2):199–205. doi: 10.6133/apjcn.202106_30(2).0003
41. Eltringham SA, Kilner K, Gee M, Sage K, Bray BD, Pownall S, et al. Impact of dysphagia assessment and management on risk of stroke-associated pneumonia: a systematic review. Cerebrovasc Dis (2018) 46(3-4):99–107. doi: 10.1159/000492730
42. Smith CJ, Kishore AK, Vail A, Chamorro A, Garau J, Hopkins SJ, et al. Diagnosis of stroke-associated pneumonia: recommendations from the pneumonia in stroke consensus group. Stroke. (2015) 46(8):2335–40. doi: 10.1161/STROKEAHA.115.009617
Keywords: prolonged hospital stay, stroke, machine learning, prediction model, SHAP (SHapley Additive exPlanations)
Citation: Wang K, Jiang Q, Gao M, Wei X, Xu C, Yin C, Liu H, Gu R, Wang H, Li W and Rong L (2023) A clinical prediction model based on interpretable machine learning algorithms for prolonged hospital stay in acute ischemic stroke patients: a real-world study. Front. Endocrinol. 14:1165178. doi: 10.3389/fendo.2023.1165178
Received: 13 February 2023; Accepted: 21 April 2023;
Published: 22 November 2023.
Edited by:
Prem P. Kushwaha, Case Western Reserve University, United StatesReviewed by:
Jianbo Chang, Peking Union Medical College and Chinese Academy of Medical Sciences, ChinaCopyright © 2023 Wang, Jiang, Gao, Wei, Xu, Yin, Liu, Gu, Wang, Li and Rong. This is an open-access article distributed under the terms of the Creative Commons Attribution License (CC BY). The use, distribution or reproduction in other forums is permitted, provided the original author(s) and the copyright owner(s) are credited and that the original publication in this journal is cited, in accordance with accepted academic practice. No use, distribution or reproduction is permitted which does not comply with these terms.
*Correspondence: Wenle Li, ZHJsZWUwOTEwQDE2My5jb20=; Liangqun Rong, cm9uZ2xpYW5ncXVuQDE2My5jb20=; Haosheng Wang, RHJfaGFvc2hlbmdAMTYzLmNvbQ==
†These authors have contributed equally to this work
‡ORCID: Wenle Li, orcid.org/0000-0002-2933-646X
Disclaimer: All claims expressed in this article are solely those of the authors and do not necessarily represent those of their affiliated organizations, or those of the publisher, the editors and the reviewers. Any product that may be evaluated in this article or claim that may be made by its manufacturer is not guaranteed or endorsed by the publisher.
Research integrity at Frontiers
Learn more about the work of our research integrity team to safeguard the quality of each article we publish.