- 1Department of Biochemistry and Molecular Biology, Indiana University School of Medicine, Indianapolis, IN, United States
- 2Indiana University Melvin and Bren Simon Comprehensive Cancer Center, Indiana University School of Medicine, Indianapolis, IN, United States
- 3Department of Obstetrics and Gynecology, Indiana University School of Medicine, Indianapolis, IN, United States
- 4Mayo Clinic Graduate School of Biomedical Sciences, Mayo Clinic College of Medicine and Science, Rochester, MN, United States
- 5Department of Anatomy, Cell Biology, and Physiology, Indiana University School of Medicine, Indianapolis, IN, United States
- 6Department of Medical and Molecular Genetics, Indiana University School of Medicine, Indianapolis, IN, United States
- 7Center for Computational Biology and Bioinformatics, Indiana University School of Medicine, Indianapolis, IN, United States
- 8Center for Genomics and Bioinformatics, Indiana University, Bloomington, IN, United States
- 9Department of Medicine, Baylor College of Medicine, Houston, TX, United States
Introduction: Endometriosis, a benign inflammatory disease whereby endometrial-like tissue grows outside the uterus, is a risk factor for endometriosis-associated ovarian cancers. In particular, ovarian endometriomas, cystic lesions of deeply invasive endometriosis, are considered the precursor lesion for ovarian clear-cell carcinoma (OCCC).
Methods: To explore this transcriptomic landscape, OCCC from women with pathology-proven concurrent endometriosis (n = 4) were compared to benign endometriomas (n = 4) by bulk RNA and small-RNA sequencing.
Results: Analysis of protein-coding genes identified 2449 upregulated and 3131 downregulated protein-coding genes (DESeq2, P< 0.05, log2 fold-change > |1|) in OCCC with concurrent endometriosis compared to endometriomas. Gene set enrichment analysis showed upregulation of pathways involved in cell cycle regulation and DNA replication and downregulation of pathways involved in cytokine receptor signaling and matrisome. Comparison of pathway activation scores between the clinical samples and publicly-available datasets for OCCC cell lines revealed significant molecular similarities between OCCC with concurrent endometriosis and OVTOKO, OVISE, RMG1, OVMANA, TOV21G, IGROV1, and JHOC5 cell lines. Analysis of miRNAs revealed 64 upregulated and 61 downregulated mature miRNA molecules (DESeq2, P< 0.05, log2 fold-change > |1|). MiR-10a-5p represented over 21% of the miRNA molecules in OCCC with endometriosis and was significantly upregulated (NGS: log2fold change = 4.37, P = 2.43e-18; QPCR: 8.1-fold change, P< 0.05). Correlation between miR-10a expression level in OCCC cell lines and IC50 (50% inhibitory concentration) of carboplatin in vitro revealed a positive correlation (R2 = 0.93). MiR-10a overexpression in vitro resulted in a significant decrease in proliferation (n = 6; P< 0.05) compared to transfection with a non-targeting control miRNA. Similarly, the cell-cycle analysis revealed a significant shift in cells from S and G2 to G1 (n = 6; P< 0.0001). Bioinformatic analysis predicted that miR-10a-5p target genes that were downregulated in OCCC with endometriosis were involved in receptor signaling pathways, proliferation, and cell cycle progression. MiR-10a overexpression in vitro was correlated with decreased expression of predicted miR-10a target genes critical for proliferation, cell-cycle regulation, and cell survival including [SERPINE1 (3-fold downregulated; P< 0.05), CDK6 (2.4-fold downregulated; P< 0.05), and RAP2A (2-3-fold downregulated; P< 0.05)].
Discussion: These studies in OCCC suggest that miR-10a-5p is an impactful, potentially oncogenic molecule, which warrants further studies.
Introduction
Previous studies have shown that each histotype of epithelial ovarian carcinoma, including high-grade serous, endometrioid, and clear-cell carcinomas, are transcriptomically distinct (1, 2). Large-scale molecular analyses of high-grade serous ovarian carcinomas showed unique classifications of tumors based on integrating multi-platform profiling (3). Molecular profiling of the endometriosis-associated ovarian carcinomas, including ovarian endometrioid and clear-cell carcinomas, showed frequent loss-of-function mutations in ARID1A (4–6). Previous work showed a unique transcriptomic profile in endometrioid ovarian carcinoma from women with concurrent endometriosis compared to those without concurrent endometriosis, supporting the role of the endometriotic microenvironment in ovarian cancer development (7, 8).
Endometriosis, a benign, chronic inflammatory condition where endometrial-like tissue grows outside the uterus, is a significant and potentially modifiable risk factor for ovarian cancer development (9, 10). Women with any amount or anatomical location of endometriosis have an increased risk of developing ovarian endometrioid (3.1-fold) or clear-cell (5.1-fold) carcinoma (11, 12). Specifically, women with ovarian endometriomas or deep infiltrating endometriotic lesions of the ovary are at even higher risk for developing ovarian endometrioid (4.7-fold) or clear-cell (10.1-fold) carcinoma (11). OCCC is a rare histological subtype composing 5-25% of ovarian malignancies, with the wide variation thought to be due to the subjective evaluation of histologic features and country-specific differences in prevalence (13–15).
Unlike the large sample size high-grade serous transcriptomic profiling studies focusing only on high-grade serous histology, transcriptomic profiling of OCCC is more limited in sample size or primarily used to show differences between transcriptomic profiles of different histological types of epithelial ovarian cancers (3, 16–21). Significantly, up to 50% of OCCCs are associated with endometriosis (22, 23). However, most transcriptomic studies of primary tumors classified as OCCC do not characterize samples as coming from women with concurrent endometriosis, pathology-proven endometriosis, or even a history of endometriosis. Only recently was a large sample size, OCCC-focused, multi-platform study performed that characterized samples as from women with a history of endometriosis (16). In this study, samples from women with a history of endometriosis were more likely to have loss-of-function mutations in ARID1A. In contrast, those with p53 mutations were likely to have poorer outcomes (16). While the tumors transcriptomically clustered into two groups – a traditional OCCC group and an aggressive p53-mutant high-grade serous-like group (16), the study did not discern particular transcriptomic contributions in samples from women with a history of endometriosis. To fill this gap, we focused our transcriptomic profiles on OCCC with pathology-proven concurrent endometriosis using both bulk RNA and miRNA sequencing.
MicroRNAs (miRNAs) are single-stranded, 22-24 nucleotide RNA molecules that function through an eight nucleotide seed sequence to modulate gene networks (24). MiRNAs are dysregulated in malignant and benign gynecological diseases and play impactful, functional roles in endometriomas and OCCC cell lines (24–27). For example, previous work has shown that endometriomas have distinct miRNA profiles and specific miRNAs, including miR-29c, play critical roles in uterine dysfunction (26). As another example, next-generation sequencing of OCCC cell lines showed that miR-100 played a critical role in rapamycin resistance in vitro (27). These limited studies suggest that miRNA molecules play essential roles. However, miRNA profiles in OCCC with concurrent endometriosis have not been examined. As a multi-platform approach, we integrated small RNA sequencing for miRNAs on matched samples of OCCC from women with pathology-confirmed concurrent endometriosis. From our list of dysregulated miRNAs, miR-10a was chosen to explore potential cellular and molecular associations in OCCC cell lines.
Materials and methods
Institutional review board approval for collection of human tissues and metadata
The expedited protocol to obtain and use tissues for this study was reviewed and approved by the Institutional Review Board (IRB) at Indiana University (#1812764043). The participants provided written informed consent to participate in this study. De-identified flash-frozen specimens, surgical pathology reports, and demographic data were obtained from tissue banks or previous studies (26). Tissue banks included the NRG Oncology Biospecimen Bank (NRG BB) and the Biospecimen Collection and Banking Core (BC2) at the Indiana University Melvin and Bren Simon Comprehensive Cancer Center (IUSCCC). Supplementary Table S1 lists the metadata and associated experiments for each de-identified human tissue sample.
OCCC with concurrent endometriosis and OCCC without endometriosis were pure clear-cell histology samples collected from adnexal masses. Tumors with mixed histology (i.e., clear-cell with endometrioid or clear-cell with serous) were not included in these studies. Inclusion criteria for OCCC with concurrent endometriosis samples were defined as the explicit mention of endometriosis on the surgical pathology report. Per banking protocols, ovarian cancer samples were taken away from obvious pathologies such as necrotic tissue or endometriosis. The malignant samples were 50-90% malignant cells (Supplementary Table S1). Endometrioma cyst wall tissues were collected as described previously (26).
Next-generation sequencing studies
Total RNA was isolated from 50-100 mg of fresh frozen tissue using the mirVana miRNA Isolation Kit with phenol (Thermo Fisher Scientific, Waltham, MA). RNA was treated with the Turbo DNA-free Kit (Thermo Fisher Scientific). RNA quality control was assessed using a 2100 Bioanalyzer (Agilent Technologies, Palo Alto, CA) at the Center for Medical Genomics at Indiana University School of Medicine (Indianapolis, IN). High-quality RNA samples were sent to the Center for Genomics and Bioinformatics at Indiana University (Bloomington, IN). Poly-A RNA libraries were constructed using mRNA Stranded TruSeq protocol (Illumina, San Diego, CA). Small RNA library construction was performed using the TruSeq Small RNA kit (Illumina). Purified libraries were visualized and quantified using a TapeStation HSD1000 (Agilent Technologies).
For poly-A bulk RNA sequencing analysis, NextSeq reads were trimmed using fastp (version 0.23.2) with parameters “-l 17 -g -p” (28). The resulting reads were mapped against GRCh38 using HISAT2 version 2.2.1 with default parameters (29). HISAT uses Bowtie2, based on the Burrows-Wheeler transform algorithm, for sequence alignment and allows for mapping across exon junctions (30). Read counts for each gene were created using featureCounts from the Subread package version 2.0.3 with the parameters “-O -M –primary –largestOverlap -s 2” and Gencode v42 as the annotation (31–33). For small RNA sequencing analysis, NextSeq reads were trimmed using fastp (version 0.23.2) with parameters “-l 17 -g -p” (28). MiRDeep2 version 2.0.0.8 was used to map the resulting reads against GRCh38 and miRBase version 22 as a reference to detect known, mature miRNA sequences (34–36). MiRDeep2 uses Bowtie to perform mapping of the reads and includes tools for the identification and quantification of miRNAs (37). Bowtie version 1.3.0 was the version of Bowtie installed. Differential expression analysis for bulk RNA and miRNA was performed using the DESeq2 package (version 1.36.0) in R/Bioconductor (R version 4.2.0) (38). Transcriptomic data have been deposited into the Gene Expression Omnibus (GSE230956). Figures from poly-A bulk RNA and small RNA sequencing analysis were created using R (version 4.2.0) and R libraries: ggplot2, complex heatmap, and ggrepel.
Quantitative PCR for mRNA and miRNA expression
Total RNA was extracted from 50-100 mg of fresh frozen tissue or cultured cells using the miRNeasy Mini Kit (Qiagen, Hilden Germany) following the manufacturer’s protocol with on-column RNase-Free DNase Set (Qiagen) or previously extracted DNase-treated RNA using the mirVana kit described above. A NanoDrop ND-1000 (Thermo Fisher Scientific) was used for the determination of RNA quantity and purity. For mRNA expression experiments, 1000 ng of DNase-treated RNA was reverse transcribed in a 20 µL reaction using 50 units MultiScribe Reverse Transcriptase (Thermo Fisher Scientific), 1X reverse transcriptase Buffer (Thermo Fisher Scientific), 0.5 mM deoxynucleotide triphosphate (Thermo Fisher Scientific), 6 units RNase Inhibitor (Thermo Fisher Scientific), and 2.5 µM random hexamers (Thermo Fisher Scientific) on a 2720 Thermo Cycler (Thermo Fisher Scientific): 10 minutes at 25°C, 30 minutes at 48°C, and 5 minutes at 95°C. Samples were diluted 1:5 for qPCR. QPCR was performed using 2 μL of diluted cDNA using either SYBR Green PCR Master Mix (Thermo Fisher Scientific) with previously published primers (39) or custom-designed primers (Supplementary Table S2) in a reaction volume of 10 μl. Only custom-designed primer pairs specific for the gene of interest, intron-spanning, with a primer efficiency of 80-110%, lacking primer-dimers, and R2 >0.95 were used (40, 41). mRNA experiments were normalized to the human β-actin (ACTB) (39). For miRNA expression experiments, total RNA (25 ng) was reverse transcribed using the TaqMan MicroRNA Reverse Transcription Kit (Thermo Fisher Scientific) in a reaction volume of 15 μl. Mature miRNA expression was performed using TaqMan mature microRNA assays on undiluted cDNA. U6 snRNA was used for normalization (26, 42). Supplementary Table S2 lists the TaqMan assays used.
Both mRNA and miRNA assays were run on a QuantStudio 3 Real-Time PCR Instrument (Thermo Fisher Scientific) with reaction conditions as follows: 2 minutes at 50°C, 10 minutes at 95°C, followed by 40 cycles of 15 seconds at 95°C (denaturation), and 1 minute at 60°C (annealing/extension). All SYBR Green assays ran dissociation curves to detect primer dimers. Each sample was analyzed in duplicate. Expression fold change calculations utilized the 2-ΔΔCT method (43). Data were plotted as mean ± SEM, and statistical analyses were performed with GraphPad Prism (Dotmatics, Boston, MA). P< 0.05 was considered statistically significant. Power analyses were performed using G*Power (version 3.1.9.7) (44, 45). Post-hoc analysis of A2780 and A2780CR5 miR-10a expression, with a type I error set at 0.05, found that we had greater than 95% power to detect a three-fold change with effect size d= 9.6 with a sample size of two in each group using a two-tailed t-test. For tissue miR-10a expression, due to greater variability, a total sample size of 24 was calculated to achieve greater than 80% power to detect a 0.7 effect size f and a type I error set at 0.05 using a one-way ANOVA.
Tissue-cell line transcriptomics data comparison analyses
To compare tissue and cell line collected from different studies, we computed the pathway activity scores (PAS) of an extensive collection of canonical biological pathways for each sample and utilized the PAS to assess the similarity between samples. We assume that the cell lines and tissue samples of high similarity should have a similar profile in more similarly activated cellular pathways. Noting that cell line samples do not have the biological characteristics of tumor microenvironments, we excluded stromal genes and related pathways from the PAS analysis. Specifically, canonical gene sets were downloaded from MSigDB version 6 c2, containing 1329 gene sets (46–48). Cancer Cell Line Encyclopedia (CCLE) cell line RNA-seq gene expression data were downloaded from the Broad Institute (49). The housekeeping genes and immune and stromal cell marker genes derived from our previous analysis were excluded (50, 51). Pathway activity scores (PAS) were assigned using the following function for each sample and pathway. For a given gene expression profile x1×N of N genes and a pathway P as a set of genes, denote y1×N as the sorted x1×N in the decreasing order and ig as the rank of gene g in y, the pathway activity score of P on x, denoted as PAS(x,P,K) is computed by
where K is the hyperparameter in this study. Here, K is set as 3000. Here, the PAS can be viewed as a normalized and weighted sum of the rank of the pathway genes whose expression is within the top K=3000 rank. PAS is computed for each pathway and each sample. Then Pearson Correlation Coefficients of the PAS of all pathways were computed between samples and used as their molecular similarity measure.
Ovarian cancer cell lines
ES-2 (52, 53), TOV-21G (54), and IGROV-1 (55) were obtained from the American Type Culture Collection (ATCC, Manassas, VA). SKOV3ip1 (56) was obtained from the Cytogenetics and Cell Authentication Core at the University of Texas M.D. Anderson Cancer Center (Houston, TX, USA). OVISE (57), OVAS (58), and OVTOKO (57) were generously obtained from Dr. Hiroshi Minaguchi (Yokohama City University, Yokohama, Japan). KK (59) was generously obtained from Dr. Yoshihiro Kikuchi (National Defense Medical College, Tokorozawa, Japan). SMOV-2 (60) was generously obtained from Dr. Tomohiro Iida (St. Marianna University, Kawasaki, Japan). A2780 (61) and A2780CR5 (62) were provided by Dr. Kenneth P. Nephew (Indiana University, Bloomington, IN, USA). SKOV3 (63) was generously obtained from Dr. Salvatore Condello (Indiana University School of Medicine, Indianapolis, IN, USA). RMG-I (64) was generously obtained from Dr. Samuel C. Mok (The University of Texas MD Anderson Cancer Center, Houston, TX, USA). Cell line authentication was confirmed using a short tandem repeat (STR) marker profile (IDEXX BioAnalytics, Westbrook, ME) within six months of experiments and tested for mycoplasma contamination monthly (MycoAlert Plus Mycoplasma Detection Kit, Lonza, Switzerland).
KK, OVISE, OVTOKO, IGROV-1, RMG-I, A2780, A2780CR5, and SKOV3 were maintained in RPMI 1640 (Thermo Fisher Scientific). OVAS was maintained in DMEM/F12 (Thermo Fisher Scientific). TOV-21G was maintained in a 1:1 ratio of Medium 199 to MCBD 105 (Sigma-Aldrich, St. Louis, MO). ES-2 was grown in McCoy’s (Thermo Fisher Scientific). All cell lines were supplemented with 1% penicillin and streptomycin (Thermo Fisher Scientific) and 10% fetal bovine serum (Atlanta Biologicals, Minneapolis, MN) except for TOV-21G, which was supplemented with 15%. All cells were cultured in a humidified incubator at 37°C with 5% carbon dioxide. Supplementary Table S3 lists the published common genetic mutations, drug responses, and the experimental uses for each of the cell lines used in this manuscript.
Carboplatin cytotoxicity assays
Carboplatin cytotoxicity assays were performed using the CellTiter 96 AQueous One Solution Cell Proliferation Assay (MTS) (Promega, Madison, WI). Cells were plated 1x103 cells per 96-well. After 24 hours, cells were treated with ten increasing (5-200 µM) doses of carboplatin [cis-diammine (1,1-cyclobutane-dicarboxylate) platinum, (C2358, Sigma)] diluted in tissue culture grade water (Thermo Fisher Scientific) in triplicate. Following 72-hours of carboplatin treatment, absorbance was read on a Synergy H1 Hybrid Reader (BioTek, Winooski, VT), background absorbance was subtracted, and data were presented as normalized to vehicle control. GraphPad Prism version 9.3.0 (Dotmatics) was used to calculate an IC50 (50% inhibitory concentration). GraphPad Prism (Dotmatics) was used to calculate the correlation between IC50 and miR-10a-5p expression. With a type I error set at 0.05, we will have 90% power to detect a correlation of 0.85 with a total sample size of 8. Figures were created using GraphPad Prism (Dotmatics).
miRNA target prediction
Putative miRNA:mRNA pairs were facilitated using SigTerms (65) with input from TargetScan (66–68), miRDB (69, 70), and miRTarBase (71). Putative target genes were further curated for potential as impactful miR-10a-5p targets using hand annotation.
MiR-10a-5p mimic transfection of human OCCC cell lines
Each cell line underwent optimization of transfection conditions using the siGLO Red Transfection Indicator (Horizon Discovery, Cambridge, United Kingdom) to determine the optimum amount of lipid transfection reagent, miRNA mimic concentration, and initial cell density. Cells were seeded at a density of 2-3x105 cells per well of a 6-well plate. After 24 hours, cells were transfected using Lipofectamine RNAiMAX Transfection Reagent (Thermo Fisher Scientific) with 100 nM hsa-miR-10a-5p mimic (mirVana miRNA mimic, Assay ID MC10787) or 100 nM negative control (mirVana miRNA mimic, Negative Control #1, catalog #4464058).
Transfected cells were used simultaneously for four different endpoints: confirmation of miR-10a overexpression, cellular proliferation, cell cycle analysis, and associated putative target gene expression by qPCR. To confirm miR-10a overexpression and associated putative target gene expression, cells were lysed at 24 hours after transfection for RNA isolation. To evaluate the effects of miR-10a overexpression on proliferation, 24 hours after transfection, cells were seeded into a 96-well plate at a density of 1000 cells/well. Cellular proliferation was measured using CellTiter 96 AQueous One Solution Cell Proliferation Assay (MTS) (Promega) in triplicate at 24-hour intervals. Absorbance was read with the Synergy H1 Hybrid Reader (BioTek). Proliferation was plotted as the percent of viable cells as a function of time using GraphPad Prism (Dotmatics). To assess the effects of miR-10a overexpression on the cell cycle, 24 hours post-transfection cells were fixed using 66% ethanol (Decon Laboratories Inc., King of Prussia, PA) and stained with Propidium Iodide (PI) according to the manufacturer’s protocol (Thermo Fisher Scientific, F#10797). Stained cells were analyzed using BD LSRFortessa (BD Biosciences, Franklin Lakes, NJ), and cell cycle analysis was performed with ModFit LT4.1 (Verity Software House, Topsham, ME). A two-tailed Student’s t-test was performed using GraphPad Prism (Dotmatics). Figures were created using GraphPad Prism (Dotmatics).
Results
OCCC samples with concurrent endometriosis had unique molecular characteristics
While nearly half of all women with OCCC have endometriosis (22, 23), transcriptomic profiling studies have not examined OCCC samples from women with pathology-proven endometriosis. Only one transcriptomic study contained OCCC samples from women with a history of endometriosis (16), but the samples were not defined as pathology-proven nor were their transcriptomic profiles analyzed independently. Here, we focused on OCCC samples from women with pathologically-confirmed endometriosis. Table 1 summarizes the clinical and pathological characteristics. OCCC with concurrent endometriosis was defined as having endometriosis at the time of staging surgery, listed on the pathology report. Women with OCCC were significantly older (median 53 years; range 39-79 years, P< 0.0001) than women with benign endometriomas (median 30.5 years; range 21-48 years). Women with OCCC and concurrent endometriosis did not differ in age (median 51 years; range 45-72 years, P = 0.15) from those without concurrent endometriosis (median 56.5 years; range 39-79). Using the Federation Internationale de Gynécologie et d’Obstétrique (FIGO) ovarian cancer staging system implemented in 2014 (72), there was no difference in the stage between the women with and without concurrent endometriosis (Fisher’s exact test = 1, P > 0.1). Thus, the OCCC samples were clinically similar except for concurrent endometriosis.
Poly-A bulk RNA and small RNA sequencing were performed on RNA isolated from specimens (n = 8), including endometrioma (n = 4) and OCCC with concurrent endometriosis (n = 4). Endometrioma samples were full cyst wall thickness samples taken from areas without gross pathology such as necrosis or abscess, with pathology-proven endometriosis without atypia and no evidence of ovarian cancer. OCCC samples were taken from adnexal masses, and samples were taken from areas free from gross endometriosis, necrosis, or abscess.
Poly-A bulk sequencing revealed over 437 million mapped reads (mean: 54,646,311 ± 6,117,236 mapped reads per clinical sample). There was no difference between endometrioma and OCCC with concurrent endometriosis samples regarding overall alignment rate, as all eight bulk RNA samples had greater than 97% of mapped reads aligned. To categorize mapped reads into RNA categories or feature counts, HISAT analysis was used (Supplementary Table S4). More reads were assigned in endometrioma (145,847,035 reads) than OCCC with concurrent endometriosis (124,361,541 reads, Student’s t-test, P< 0.01). OCCC with concurrent endometriosis had more reads assigned to mitochondrial RNA species (33,929,857 to 22,296,699; endometrioma, Student’s t-test, P< 0.01). More reads were assigned to protein-coding genes in benign endometrioma (98,430,773 reads) than OCCC with concurrent endometriosis (70,984,332 reads, Student’s t-test, P< 0.01).
Transcriptomic profiles of the endometrioma and OCCC with concurrent endometriosis samples were first evaluated using multidimensional scaling (MDS) analysis (73). The MDS plot shows a significant differential clustering of the OCCC samples with concurrent endometriosis from endometriomas (Figure 1A). This difference is most apparent at the MDS1 level. Similar clustering was noted on uniform manifold approximation and projection (UMAP) and principal component analysis (PCA) for dimension reduction plots (Supplementary Figures S1A, B). At a global level, malignant OCCC with concurrent endometriosis is molecularly distinct from benign endometriomas.
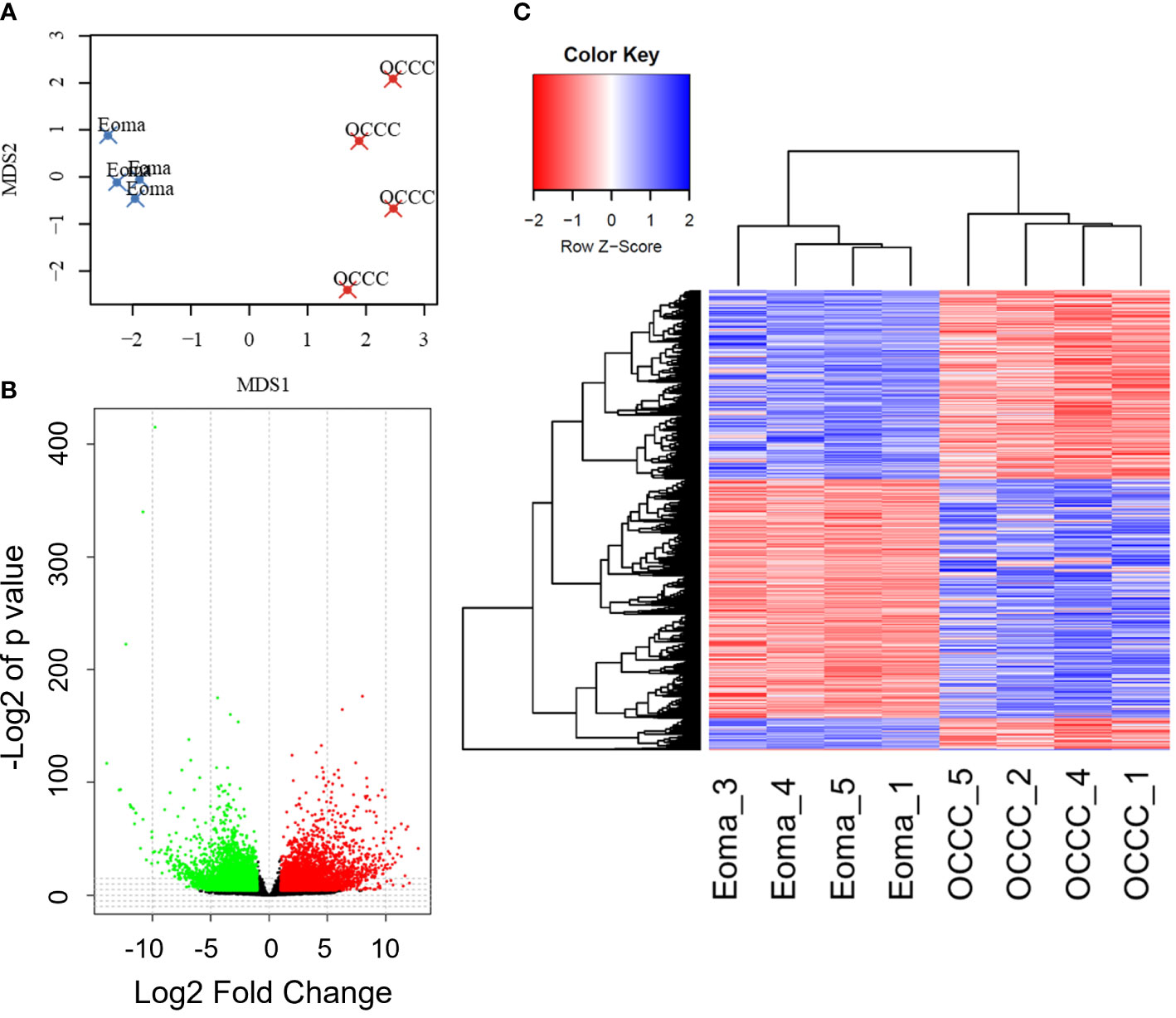
Figure 1 The OCCC with concurrent endometriosis transcriptomic profile is molecularly distinct from benign endometrioma. (A) Multidimensional scaling (MDS) plot of transcriptomic profiles for endometrioma (Eoma, blue X’s) and ovarian clear cell carcinoma (OCCC, red X’s) with concurrent endometriosis. (B) Volcano plot representation of protein-coding transcripts overexpressed (red dots), similarly expressed (black dots), and under-expressed (green dots) in OCCC with concurrent endometriosis versus endometriomas (Padj<0.05; Log2-Fold change<|1|. (C) Heat map representation of 5575 differentially expressed unique protein-coding gene transcripts overexpressed (red) and under-expressed (blue). Dendrogram of hierarchical clustering. Rows, protein-coding gene transcripts; columns, profiled samples.
We directly compared transcriptomic profiles of OCCC with concurrent endometriosis (n = 4) to endometriomas (n = 4). Endometrioma was used as a comparison tissue due to its strong increase in risk for the development of OCCC, studies supporting increased molecular mutations in atypical endometriosis and concurrent OCCC, strong genomic correlation and causal relationship between endometriosis and OCCC, and the high incidence of concurrent endometriosis seen in women with OCCC (4, 11, 17, 18, 22, 23). Differential gene expression analysis was conducted with DESeq2. Significant differential expression was determined using a false discovery rate<0.05, giving 6865 protein-coding transcripts significantly differentially expressed. Limiting to log2 fold-change >|1| identified 2449 upregulated and 3131 downregulated unique protein-coding genes (Figures 1B, C, Supplementary Tables S5, S6). Hierarchical clustering shows that endometriomas cluster separately from OCCC with concurrent endometriosis (Figure 1C).
Matrisome, secreted factors, cell cycle, and DNA replication pathways are dysregulated in OCCC with concurrent endometriosis
To explore potentially impactful molecular processes, we performed pathway enrichment analysis of the upregulated and downregulated genes using a hypergeometric test and Gene Set Enrichment Analysis (GSEA) against MSigDB v6 canonical pathway set, with a significant cutoff determined by P< 0.005 (46–48). Complete lists of the pathways significantly enriched by upregulated and downregulated genes are given in Supplementary Tables S7, S8. We observed a limited list of pathways from upregulated genes in OCCC with concurrent endometriosis (Supplementary Table S7). The upregulated genes showed significant enrichment in cell cycle and DNA replication pathways, including cyclin A B1-mediated G2-M transition, G1-S transcription, and E2F-mediated DNA replication (Supplementary Figures S2A-C). Previous work has shown that OCCC exhibited dysregulation of p27-related cell cycle effects (74). Important drivers of p27-related cell cycle dysregulation that were upregulated in OCCC with concurrent endometriosis include SKP2 (S-phase kinase-associated protein 2, log2 fold-change = 1.3, P = 1.1e-2), CKS2 (CDC28 protein kinase regulatory subunit 2, log2 fold-change = 2.2, P = 6.6e-9), CCNA2 (Cyclin A2, log2 fold-change = 1.7, P = 2.9e-3), and CCNE1 (Cyclin E1, log2 fold-change = 5.2, P = 8.5e-10). GSEA plots of the cyclin A-mediated G2-M transition (P = 8.95e-6) and E2F-mediated DNA replication (P = 8.61e-4) top enriched pathways from upregulated genes are shown in Supplementary Figures S2B, C. Supplementary Table S9 lists the upregulated genes involved in the cell cycle with their fold change.
There were many more significantly downregulated pathways in OCCC with concurrent endometriosis. Significantly downregulated genes in OCCC with concurrent endometriosis showed significant enrichment in the pathways of matrisome, secreted factors, GPCR signaling, and cytokine-cytokine-receptor interaction (Figure 2A). GSEA plots of matrisome (P = 2.64e-69) and cytokine-cytokine receptor interaction (P = 2.43e-20) pathways from downregulated genes are shown in Figures 2B, C. Key genes involved in the cytokine-cytokine receptor interaction pathway are largely upregulated in endometriomas (7, 26, 75–78). Supplementary Table S10 shows the downregulated genes in OCCC with concurrent endometriosis compared to endometrioma in the cytokine-cytokine receptor interaction pathway.
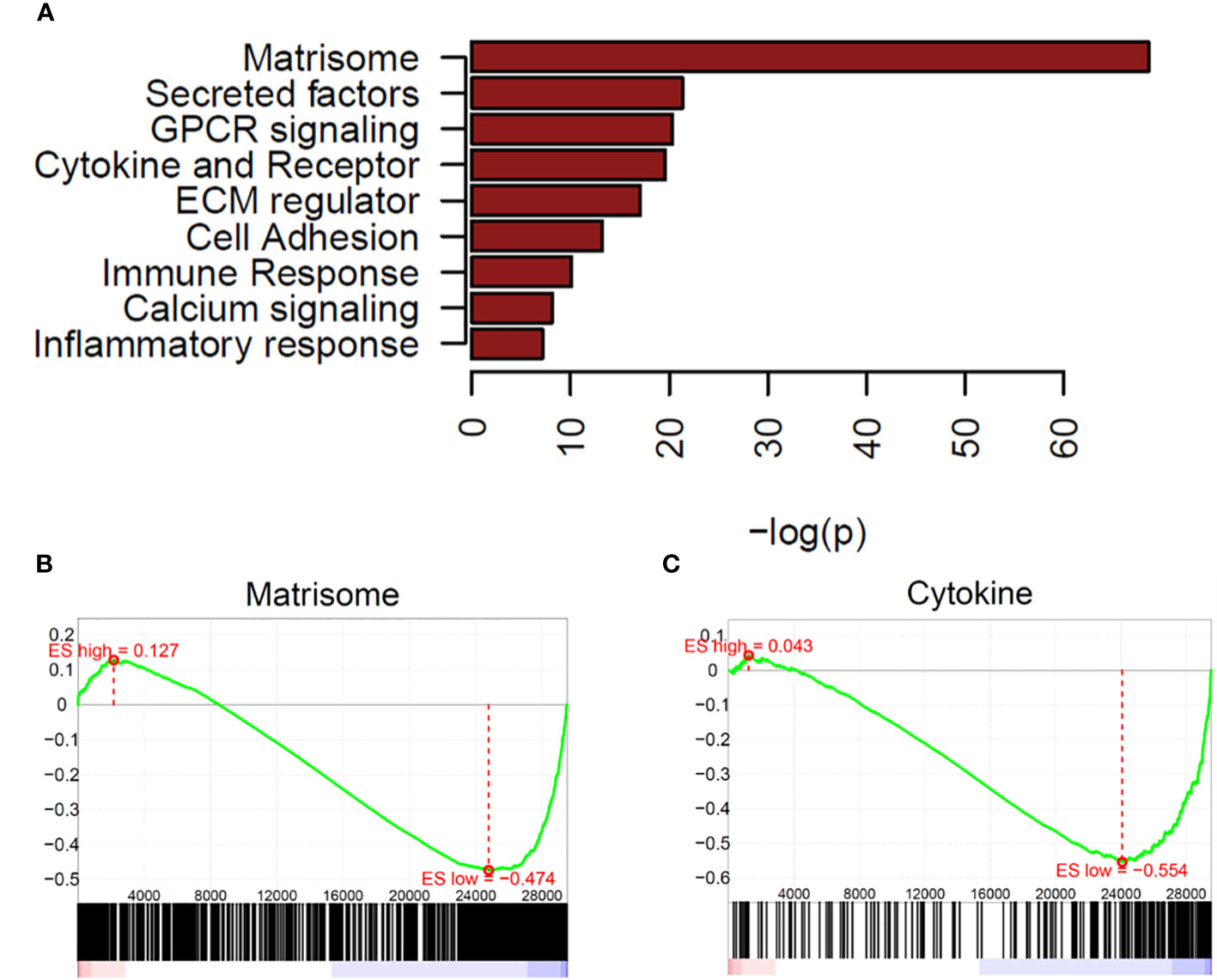
Figure 2 Matrisome and cytokine pathways are enriched in downregulated genes from OCCC with concurrent endometriosis. (A) Waterfall plot of significantly downregulated pathways in OCCC with concurrent endometriosis. Gene set enrichment plots for (B) NABA_MATRISOME and (C) KEGG_CYTOKINE_CYTOKINE_RECEPTOR_INTERACTION.
Determining a model cell line of OCCC with concurrent endometriosis based on human gene expression by RNA sequencing
Genomically, OCCC cell lines frequently classify as OCCC rather than other histological subtypes of epithelial ovarian cancer (79–84). However, none of the widely shared or commercially available OCCC cell lines are characterized as being derived from OCCC with concurrent endometriosis. To determine which available cell lines most closely recapitulate our transcriptomic profiling data from OCCC with concurrent endometriosis, we used bioinformatic analysis of datasets from the Cancer Cell Line Encyclopedia (CCLE) (49). Gene expression datasets for ovarian carcinoma cell lines [endometrioid/clear-cell (IGROV-1, SKOV3, A2780), clear cell (TOV-21G, OVTOKO, OVISE, OVMANA, JHOC_5), p53-altered clear-cell (RMG-I, ES-2), endometrioid (TOV112D), high-grade serous (OVCAR8), and low-grade serous (HEYA8)], endometrial cancer cell lines (AN3CA, HEC1A, ISHIKAWA, HEC1B), and a leukemia cell line (JURKAT) were downloaded and used. For the RNA-seq gene expression data from the OCCC with concurrent endometriosis tissue samples and endometrioma samples, we utilized multiclass logistic regression with variable selection by L1 penalty. We selected pathways whose pathway activity scores (PAS) are most predictive of cancer types. PAS of the 394 cancer-types predictive pathways were computed for OCCC with concurrent endometriosis tissue, endometrioma, and the selected cell line samples. We computed a Pearson correlation between the PAS of 394 pathways in tissue and cell line data to measure the similarity.
Examination of the PAS results (Figure 3) showed that endometrioma samples (Eoma1, Eoma4, Eoma5) clustered most closely to endometrioma sample 3 (Eoma3), ES2, and HEYA8. ES-2 is considered a p53-altered OCCC cell line (81, 85), and HEYA8 is considered a KRAS-mutant low-grade serous line (85). Studies suggest that both HEYA8 and ES-2 most closely represent low-grade serous (83). The dendrogram suggests that ES2 and HEYA8 cluster more closely to each other than Eoma3. OCCC samples with concurrent endometriosis (OCCC4, OCCC2) clustered together along with OCCC5, OVTOKO, OVISE, RMG-I, and OVMANA. OVTOKO, OVISE, and OVMANA are considered clear-cell ovarian cancer cell lines as they were derived from metastatic lesions of OCCC and contain a mutant ARID1A (57, 80, 83). RMG-I may be a p53-mutant clear cell type rather than a mutant ARID1A type (16, 83, 84). OCCC sample with concurrent endometriosis 1 (OCCC1) clustered with IGROV-1, JHOC5, SKOV3, and TOV-21G. IGROV-1 is derived from a mixed histology tumor and contains mutant ARID1A and PIK3CA and could be considered a clear cell-like line (81–83, 85). SKOV3 was derived from ascites of ovarian adenocarcinoma and contains mutant ARID1A and PIK3CA, and is frequently more closely associated with clear-cell tumors (63, 81–83, 85). TOV-21G is considered a clear cell line as it was derived from OCCC and contains mutations in ARID1A and PIK3CA (54, 81–83, 85) (Supplementary Table S3).
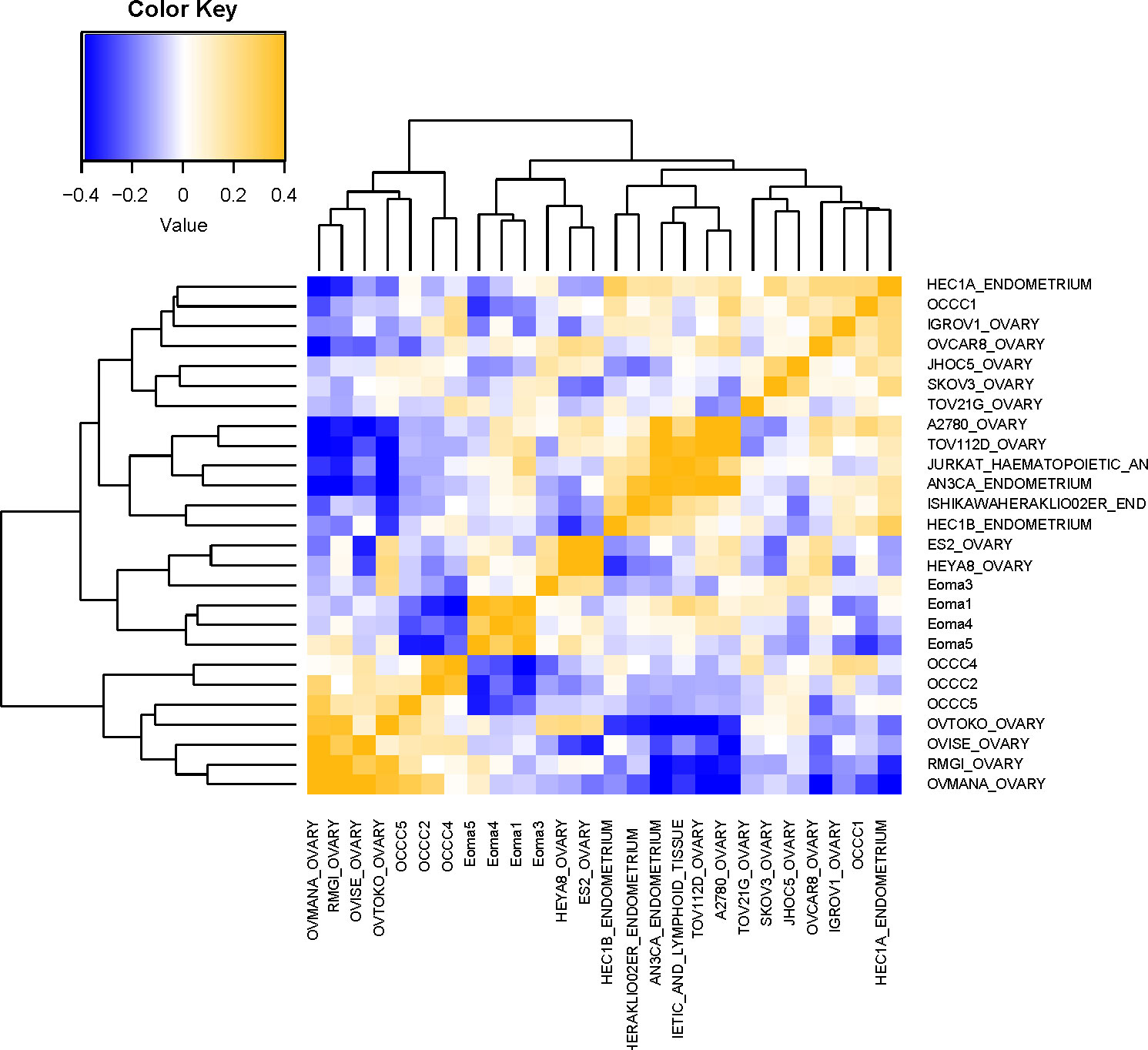
Figure 3 Molecular comparison of cell line transcriptomic profiles with clinical samples. Computational analysis of the molecular similarity of the clinical samples (Eoma, benign endometrioma or OCCC, OCCC with concurrent endometriosis) and cell lines from CCLE. A pathway activation score (PAS) was first computed for each pathway and each sample. Then the similarity between the samples was assessed by Pearson Correlation Coefficients (PCC) of the PAS. Cell line nomenclature is the CCLE name of the line_tissue type. A yellow color represents a higher PCC; blue, a lower PCC.
OCCC with concurrent endometriosis samples have dysregulated miRNA expression
MiRNAs are impactful for their potential as disease biomarkers and role as upstream regulators of multiple signaling pathways in diseases of the female reproductive tract (24, 25). However, large-scale profiling of miRNAs has focused broadly on epithelial ovarian cancers without a direct analysis of OCCC, included only a small number of OCCC samples, or did not describe any samples with a history of or pathology-proven endometriosis (86–90). To complement our protein-coding transcriptomic studies, we profiled miRNAs on RNA isolated from clinical samples (n = 8), including endometrioma (n = 4) and OCCC with concurrent endometriosis (n = 4).
Small RNA sequencing gave over 43 million reads (mean: 5,382,728 ± 644,063 mapped reads per clinical sample). There were no significant differences in the mirDeep2 total mapped count percentage between endometrioma and OCCC with concurrent endometriosis (Supplementary Table S11). Of the 2588 human mature miRNA molecules, 446 were expressed in at least one clinical sample. Principal component analysis (Figure 4A) with miRNA expression profiles showed PC1 and PC2 differential clustering of the OCCC with concurrent endometriosis from the benign endometrioma. Differential miRNA expression analysis was conducted with DESeq2. A comparison of dysregulated miRNAs between endometrioma and OCCC with endometriosis is shown on the volcano plot (Figure 4B). Significant differential expression was determined using a false discovery rate< 0.05, giving 128 significantly differentially expressed mature miRNA molecules. Limiting to log2 fold change > |1| identified 64 upregulated and 61 downregulated mature miRNA molecules (Supplementary Tables S12, S13).
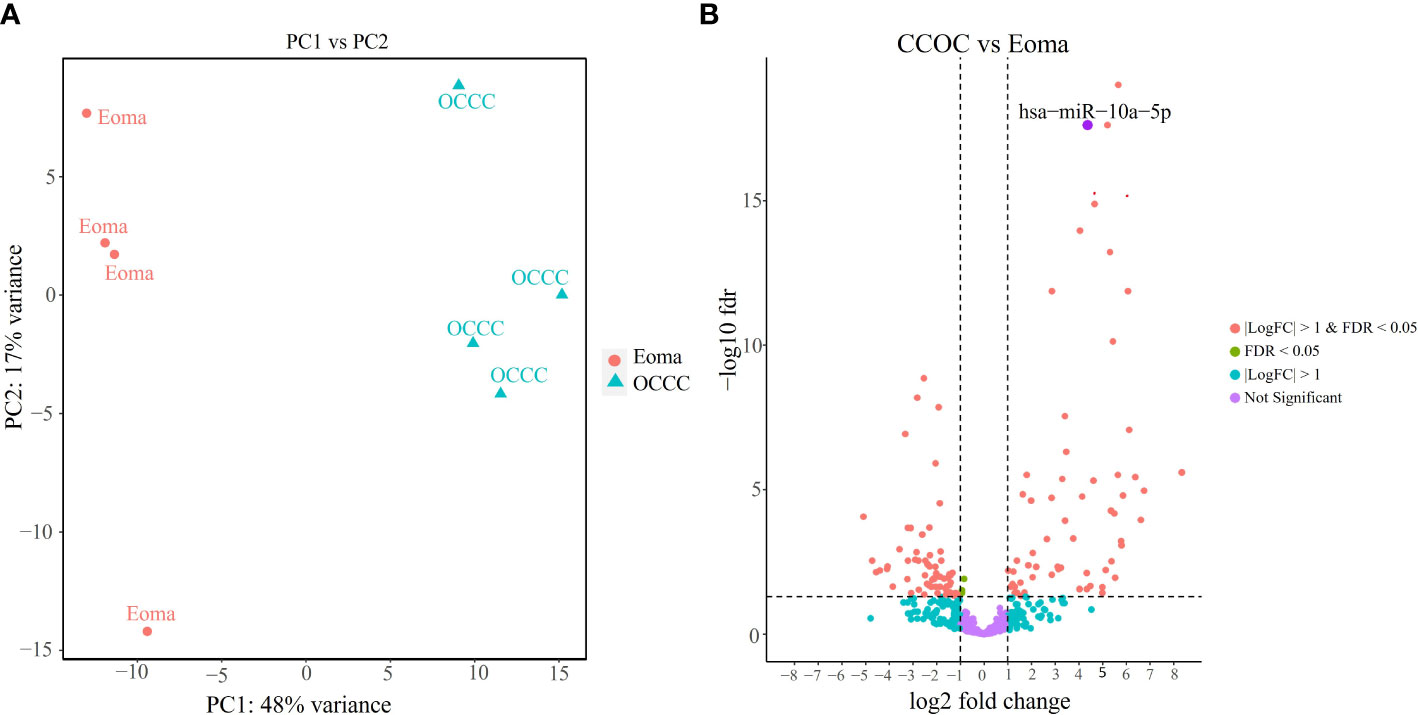
Figure 4 OCCC with concurrent endometriosis is molecularly distinct from benign endometrioma using miRNA expression. (A) Principal component (PC) analysis of malignant OCCC with concurrent endometriosis (triangles, OCCC) clusters separately from benign endometrioma samples (circles, Eoma), using PC1 and PC2. (B) Volcano plot of the significantly dysregulated miRNAs. Red represents miRNAs with P< 0.05, log2 fold-change > |1|. Blue dots represent miRNAs log2 fold-change > |1|. Green represents miRNAs with P< 0.05. Purple dots represent non-statistically significant miRNAs. The labeled dot represents hsa-miR-10a-5p.
Because fold change up- or downregulated is a relative number, we examined the most abundant miRNA molecules in endometriomas with statistically significant lower expression in OCCC with concurrent endometriosis. The most abundant miRNA in endometriomas was hsa-miR-143-3p, representing 14.5%. Hsa-miR-146b-5p represented nearly 5% (or 4.47%) of endometrioma miRNAs. Table 2 shows the top ten downregulated mature miRNA molecules in OCCC with concurrent endometriosis. Table 3 shows the top ten upregulated mature miRNA molecules in OCCC with concurrent endometriosis. MiR-10a-5p was the most abundant miRNA in OCCC with concurrent endometriosis, representing nearly a quarter of all miRNAs (21.5%). Other significantly abundant and upregulated miRNAs included hsa-miR-30a-5p (6.1%), two other miR-30 family members [hsa-miR30d-5p (0.71%) and hsa-miR-30c-5p (0.12%)], and hsa-miR-141-3p (1.35%). Three mature miRNA molecules had a log2 fold change >4 and were in the top ten in terms of abundance in OCCC with concurrent endometriosis: hsa-miR-10a-5p (log2 fold change = 4.37, P = 2.43e-18), hsa-miR-141-3p (log2 fold change = 4.67, P = 1.31e-15), and hsa-miR-183-5p (log2 fold change = 4.62, P = 4.90e-6).
Overexpression of miR-10a has been found in breast, cervical, acute myeloid leukemia, and pancreatic ductal adenocarcinomas (91–98). Further, miR-10a overexpression was correlated with an increased risk of recurrent breast cancer and decreased response to platinum agents in vitro (92, 96, 97, 99). Disease progression on first-line platinum therapy is a hallmark of OCCC, with response rates to chemotherapy ranging as low as 11% (100–105). While platinum resistance is the most common reason for death from recurrence across all ovarian cancers, progression on platinum therapy is more prevalent in OCCC (103, 104, 106). Thus, we explored the cellular and molecular effects of miR-10a in OCCC. QPCR expression showed that benign endometrioma exhibited a significantly lower expression of miR-10a-5p than OCCC with or without endometriosis (one-way ANOVA, P< 0.05, Figure 5). OCCC with concurrent endometriosis exhibited an 8-fold overexpression of miR-10a-5p compared to benign endometrioma (Student’s t-test, P = 0.01). However, there was no statistically significant difference in miR-10a-5p expression between OCCC with concurrent endometriosis and OCCC without endometriosis (Student’s t-test, P = 0.90).
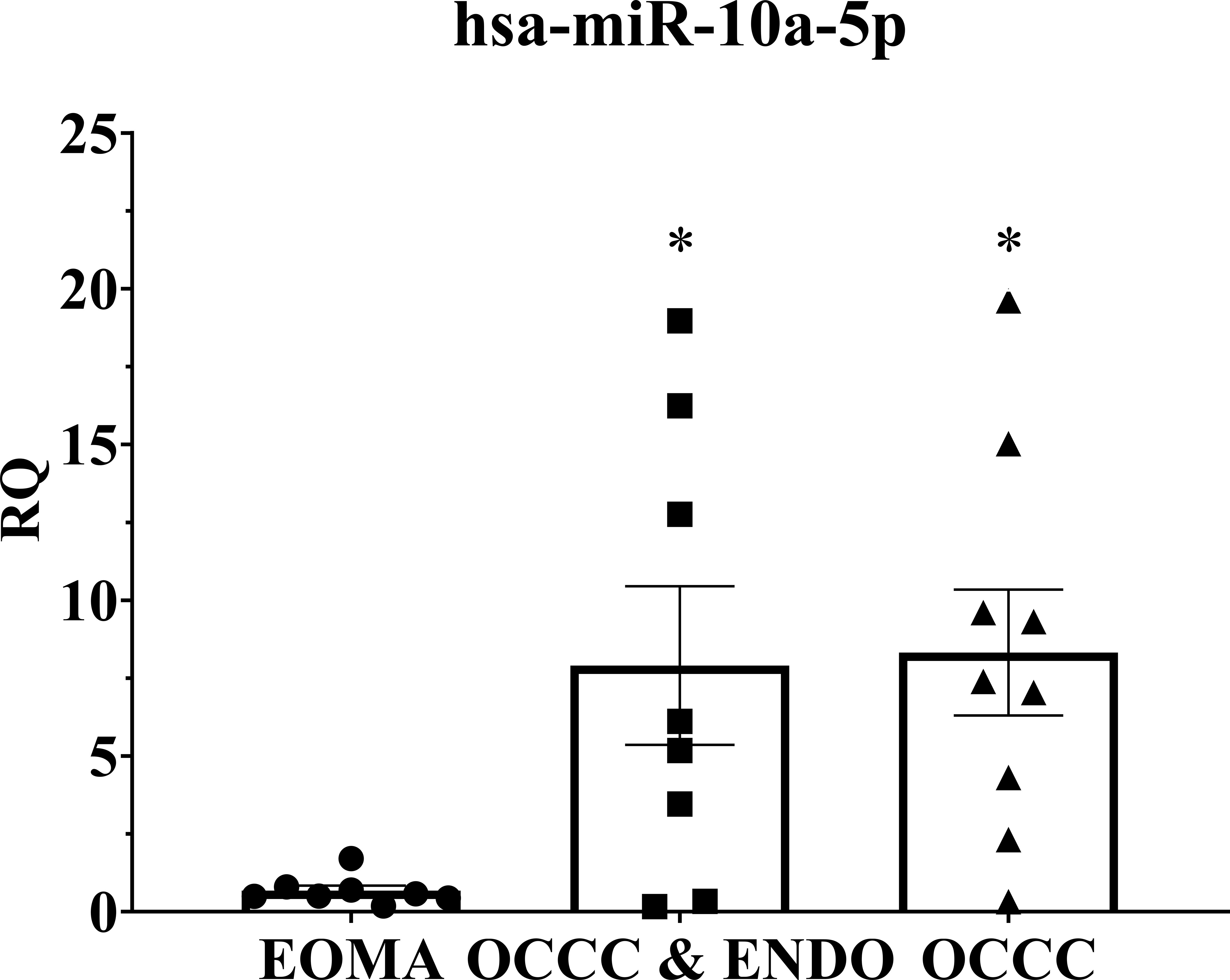
Figure 5 MiR-10a-5p is significantly upregulated in human tissue samples of ovarian clear cell carcinoma. Examination of miR-10a-5p expression in endometrioma (EOMA, n = 8), ovarian clear cell carcinoma from women with concurrent endometriosis (OCCC & ENDO, n = 8), and ovarian clear cell carcinoma from women without pathologically confirmed endometriosis (OCCC, n = 9). RQ, the relative quantity of hsa-miR-10a-5p to U6 snRNA, normalized to EOMA. Error bars represent ± SEM. *P< 0.05, one-way ANOVA.
MiR-10a-5p expression in human OCCC cell lines correlates with carboplatin IC50
To explore the role of miR-10a in OCCC, the expression of miR-10a-5p was examined in a panel of human ovarian cancer cell lines (Figure 6A). There appeared to be two groups of cell lines – those with low miR-10a expression (A2780, OVISE, TOV-21G, OVTOKO, KK, and SMOV-2) and those with high miR-10a expression (A2780CR5, SKOV3ip1, RMG-I, SKOV3, OVAS, ES-2). To confirm similar results, we analyzed the next-generation small RNA sequencing data from Nagaraja et al. (27). The relative expression of miR-10a in each cell line was similar to our expression. There was a low miR-10a expression in OVISE, TOV-21G, SMOV-2, and KK, and those with high relative miR-10a expression included RMG-I, ES-2, and OVAS (Supplementary Figure S3). ES-2 transcriptomically clustered with endometriomas (Figure 3) and exhibited relatively high expression of miR-10a in both our qPCR analyses (Figure 6A) and next-generation sequencing data from Nagaraja et al. (27). RMG-I and SKOV3 transcriptomically clustered with OCCC with concurrent endometriosis (Figure 3) and showed high expression of miR-10a (Figure 6A). On the other hand, OVISE, TOV-21G, and OVTOKO transcriptomically clustered with OCCC with concurrent endometriosis (Figure 3) but exhibited low expression of miR-10a (Figure 6A).
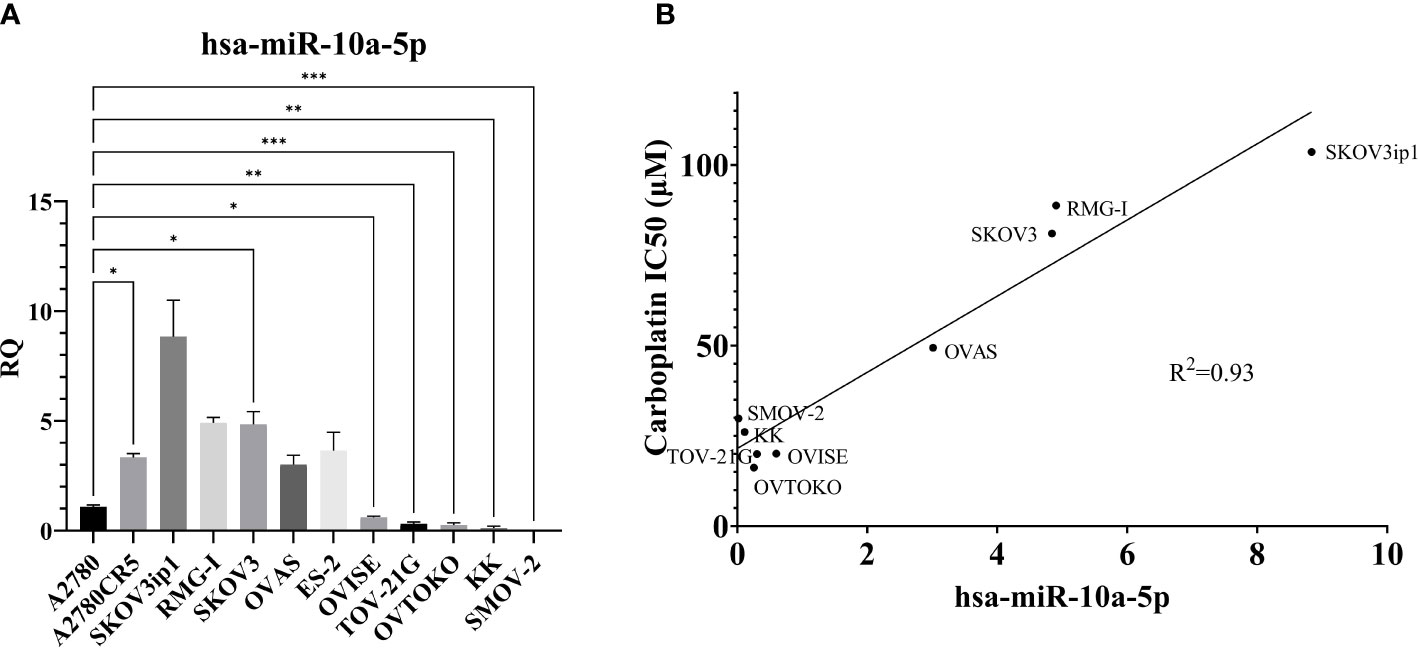
Figure 6 MiR-10a-5p expression across a panel of ovarian cancer cell lines. (A) RQ, the relative quantity of hsa-miR-10a-5p to U6 snRNA, normalized to A2780. ***P<0.001, **P<0.001, *P< 0.05, Brown-Forsythe and Welch ANOVA. A2780 (n = 6), A2780CR5 (n = 2), SKOV3ip1 (n = 3), RMG-I (n = 2), SKOV3 (n = 4), OVAS (n = 4), ES-2 (n = 4), OVISE (n = 9), TOV-21G (n = 4), OVTOKO (n = 7), KK (n = 3), and SMOV-2 (n = 6). (B) Correlation of miR-10a-5p expression to carboplatin IC50 in OCCC cell lines. RMG-I (n = 2), OVAS (n = 4), ES-2 OVISE (n = 9), TOV-21G (n = 2), OVTOKO (n = 7), KK (n = 2), and SMOV-2 (n = 6). Carboplatin IC50 is n ≥ 5 for each cell line.
A2780CR5 cells are an isogenic line of A2780 that is resistant to platinum (62). Interestingly, the miR-10a-5p expression was 3.3-fold higher in the platinum-resistant line, A2780CR5 (Mann-Whitney, P< 0.01) than in A2780. Increased miR-10a expression has previously been correlated with platinum resistance in lung cancer (99, 107). As a result of this increased miR-10a-5p expression in the platinum-resistant line, carboplatin response was compared across OCCC cell lines. Carboplatin response was expressed as the half maximum inhibitory capacity (IC50) and correlated with miR-10a-5p expression. A positive correlation (R2 = 0.93) was found between miR-10a expression and carboplatin IC50 (Figure 6B).
MiR-10a-5p overexpression decreases cellular proliferation.
SKOV3ip1cells are a xenograft-derived line of SKOV3. Previous work showed increased cellular proliferation of SKOV3ip1 cells compared to SKOV3 (56). The miR-10a-5p expression was almost 2-fold higher in SKOV3ip1 (un-paired t-test, P<0.05) than SKOV3. In vitro studies overexpressing miR-10a-5p showed potentially cancer type specific effects on cellular proliferation. For example, overexpression of miR-10a in melanoma, acute myeloid leukemia, and laryngeal squamous cell carcinoma cells decreased cellular proliferation in these cancers (108–110).
To study the effects of miR-10a overexpression on proliferation in OCCC cell lines, SMOV-2 and KK were transiently transfected with a mature miR-10a-5p mimic. After optimization of transfection conditions (data not shown), overexpression of miR-10a was confirmed (Supplementary Figure S4). SMOV-2 and KK miR-10a overexpressing cells (SMOV2-10a and KK-10a) had a statistically significant and sustained decrease in cellular proliferation compared to the non-targeting control transfected cells (SMOV2-10actl and KK-10actl) (Figure 7). For SMOV-2 cells, there was a statistically significant decrease in proliferation beginning at 96 hours. Overexpression of miR-10a in SMOV-2 cells showed an almost 2-fold increase in doubling time, from 42.8 hours to 84.9 hours. A statistically significant decrease in cell density was noted beginning at 72 hours in KK cells overexpressing miR-10a compared to a non-targeting control and continuing through 120 hours, there was a statistically significant decrease in proliferation. Overexpression of miR-10a in KK cells lengthened the double time from 40.9 hours to 47.2 hours.
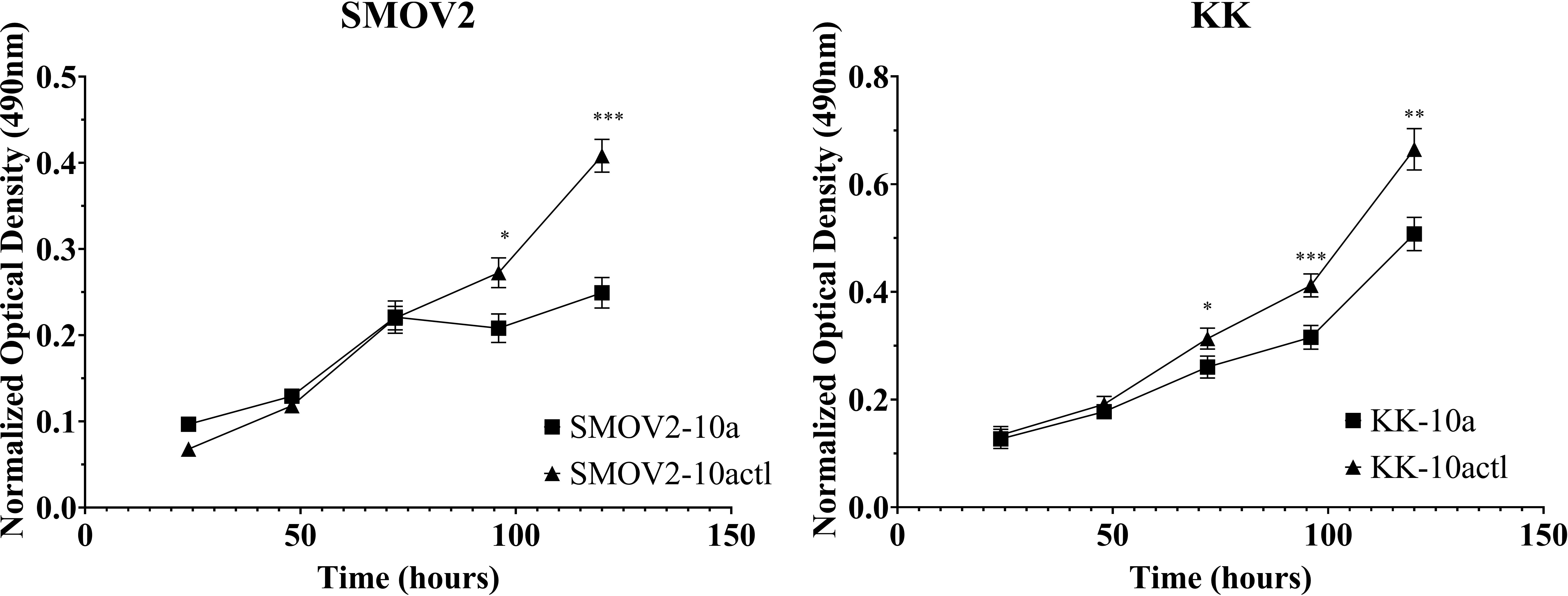
Figure 7 MiR-10a overexpression resulted in a significant decrease in cellular proliferation in SMOV2 and KK cells. MTS was normalized to the growth medium for each measurement. Cells transfected with mature miRNA mimic for miR-10a (SMOV2-10a and KK-10a) were compared to cells transfected with negative control #1 (SMOV2-10actl and KK-10actl) for each cell line and each time point. ***P< 0.0001, **P< 0.001, *P< 0.05, Student’s two-tailed t-test at each time point. n =6 for each cell line, timepoint, and transfection condition.
MiR-10a-5p overexpression shifts cells from S and G2 to G1 phase.
Cell cycle distribution was analyzed in SMOV-2 and KK cells overexpressing miR-10a-5p compared to non-targeting control transfected cells. SMOV-2 and KK cells overexpressing miR-10a-5p had a statistically significant increase in the G1 population and a decrease in S and G2 populations (P< 0.001) (Figure 8). SMOV2-10a had more than a 6% increase in the G1 population with a 4.5% decreased percent in S phase (P< 0.0001). KK-10a had a similar 7% increase in cells in the G1 phase, but KK-10a cells had a more distributed decrease in S phase (4%, P = 0.01) and G2 (3%, P = 0.002). The amount of cellular debris was not significantly changed in either SMOV-2 or KK samples (Figure 8).
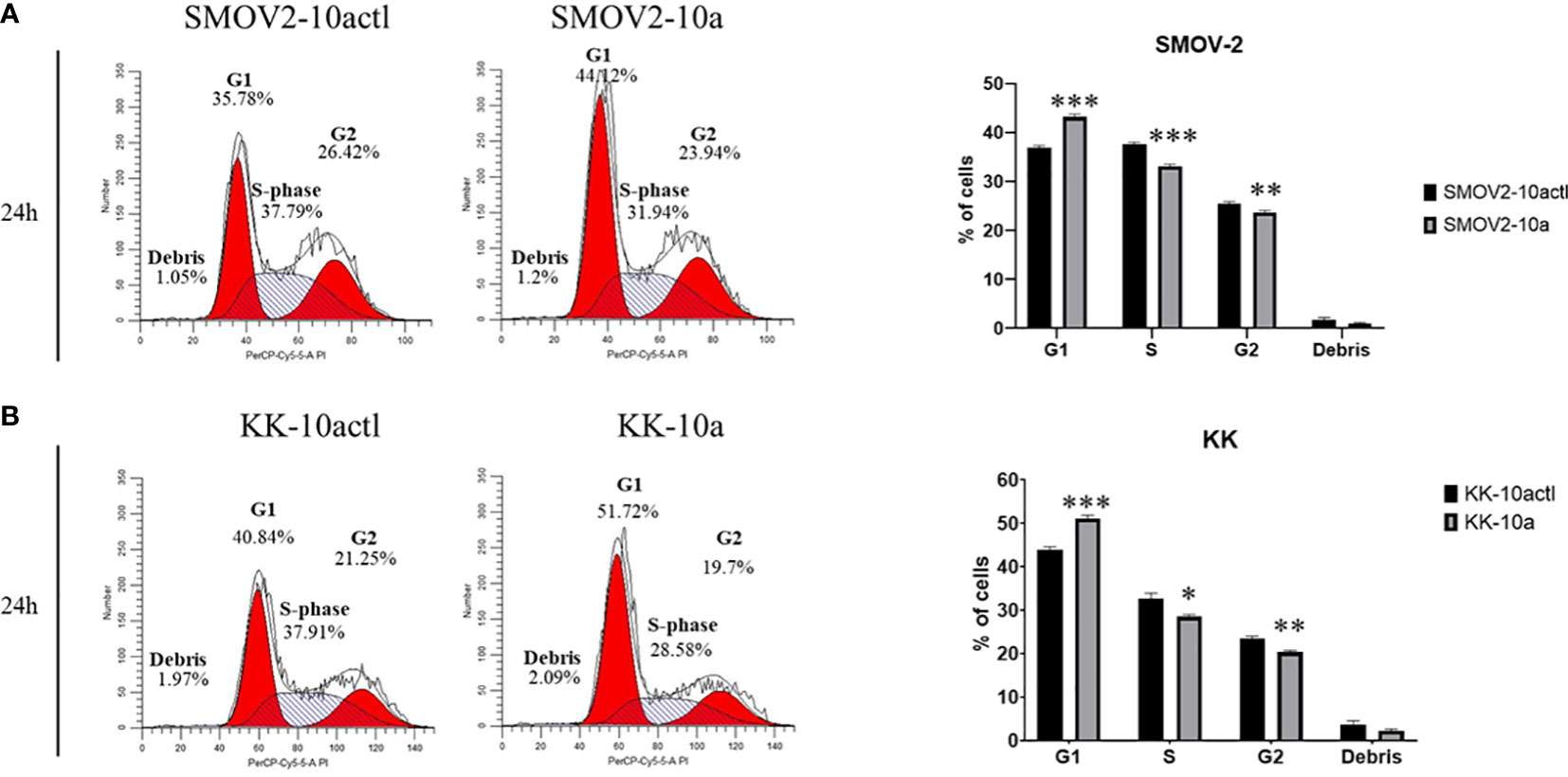
Figure 8 Overexpression of hsa-miR-10a-5p shifts cell cycle from S and G2 phase to G1. Cells transfected with mature miRNA mimic for miR-10a (SMOV2-10a and KK-10a) were compared to cells transfected with negative control #1 (SMOV2-10actl and KK-10actl) for each cell line. The flow histograms depict a representative biological replicate for (A) SMOV-2 and (B) KK. Graphical depictions represent n = 6 for each cell line and transfection condition. Statistical analysis was conducted using a 2-tailed Student’s t-test: ***P< 0.0001, **P< 0.001, *P< 0.05.
Predicted miR-10a-5p target genes dysregulated in OCCC with endometriosis play a role in signaling receptor binding.
MiRNA molecules are considered epigenetic regulators of gene expression (111). Overexpression of miRNA molecules leads to downregulation largely by destabilization of mRNA transcripts. Importantly, most mRNA molecules are targets of miRNAs (112). Each miRNA molecule has relative specificity of gene targets based on nucleotide sequence in the 3’UTR of the target gene. In silico prediction of genes that could be targeted by individual miRNA families is available in several databases. Target Scan predicts miRNA binding through complementary binding of the seed region of the mature miRNA molecule to the mRNA molecule, typically within the 3’UTR (66–68). As a slightly different algorithm for miRNA target gene predictions, miRDB uses in silico predicted miRNA binding to mRNA targets and downregulation of target gene expression from high-throughput sequencing data to identify putative targets. Additional predictions are added to miRDB from computational modeling and literature curation (69, 70). As another resource, miRTarBase uses natural language processing (NLP) to extract miRNA-predicted target gene data across the literature, to give miRNA-target interactions (MTIs). Examples of MTIs from miRTarBase include direct interaction studies of miRNA and target genes from CLIP-seq data, in silico seed sequence binding to mRNA from miRanda and miRBase, and experimental validation through reporter assays, western blots, or qPCR (71). To determine which dysregulated genes in OCCC with concurrent endometriosis were predicted targets of miR-10a-5p, miRNA:mRNA functional interaction prediction was undertaken using datasets from Target Scan v7.2, miRDB, and miRTarBase 2022 (65). Target Scan predicted 61 (Supplementary Table S14), miRDB predicted 62 (Supplementary Table S15), and miRTarBase predicted 67 (Supplementary Table S16) unique protein-coding genes downregulated in OCCC with concurrent endometriosis to be putative miR-10a-5p targets.
While 151 unique protein-coding genes were predicted to be miR-10a-5p target genes in at least one of the three algorithms, BDNF (brain-derived neurotrophic factor, log2 fold-change -2.42, P = 6.8e-5), RORA (RAR related orphan receptor A, log2 fold-change -1.98, P = 1.48e-7), CSRNP3 (cysteine and serine-rich nuclear protein3, log2 fold-change -1.93, P = 1.57e-3), CHL1 (cell adhesion molecule L1 like, log2 fold-change -3.08, P = 2.09e-5), LIX1L (limb and CNS expressed 1 like, log2 fold-change -1.13, P = 7.11e-5), and RAP2A (RAP2A, member of RAS oncogene family, log2 fold-change -1.52, P = 2.63e-5) were genes predicted to be miR-10a-5p targets in each of the three datasets. Using the 151 genes as input, the WEB-based Gene SeT AnaLysis Toolkit [WebGestalt (113)] revealed that the top network for miRNA targeting was the miR-10 family as expected (enrichment ratio = 13.67, Supplementary Table S17). Gene ontology molecular function analysis (Supplementary Table S18) showed enrichment in signaling receptor binding genes (enrichment ratio = 1.92, P = 1.5e-3). Pathway analysis (Supplementary Table S18) showed enrichment in cellular senescence genes (enrichment ratio = 4.71, P = 4.24e-3) and TGFβ signaling pathway (enrichment ratio = 7.17, P = 2.34e-3). A listing of the downregulated predicted miR-10a-5p target genes from the signaling receptor binding molecular function is listed in Table 4. Signaling receptor binding, cellular senescence, and TGFβ-signaling all involve the cell cycle.
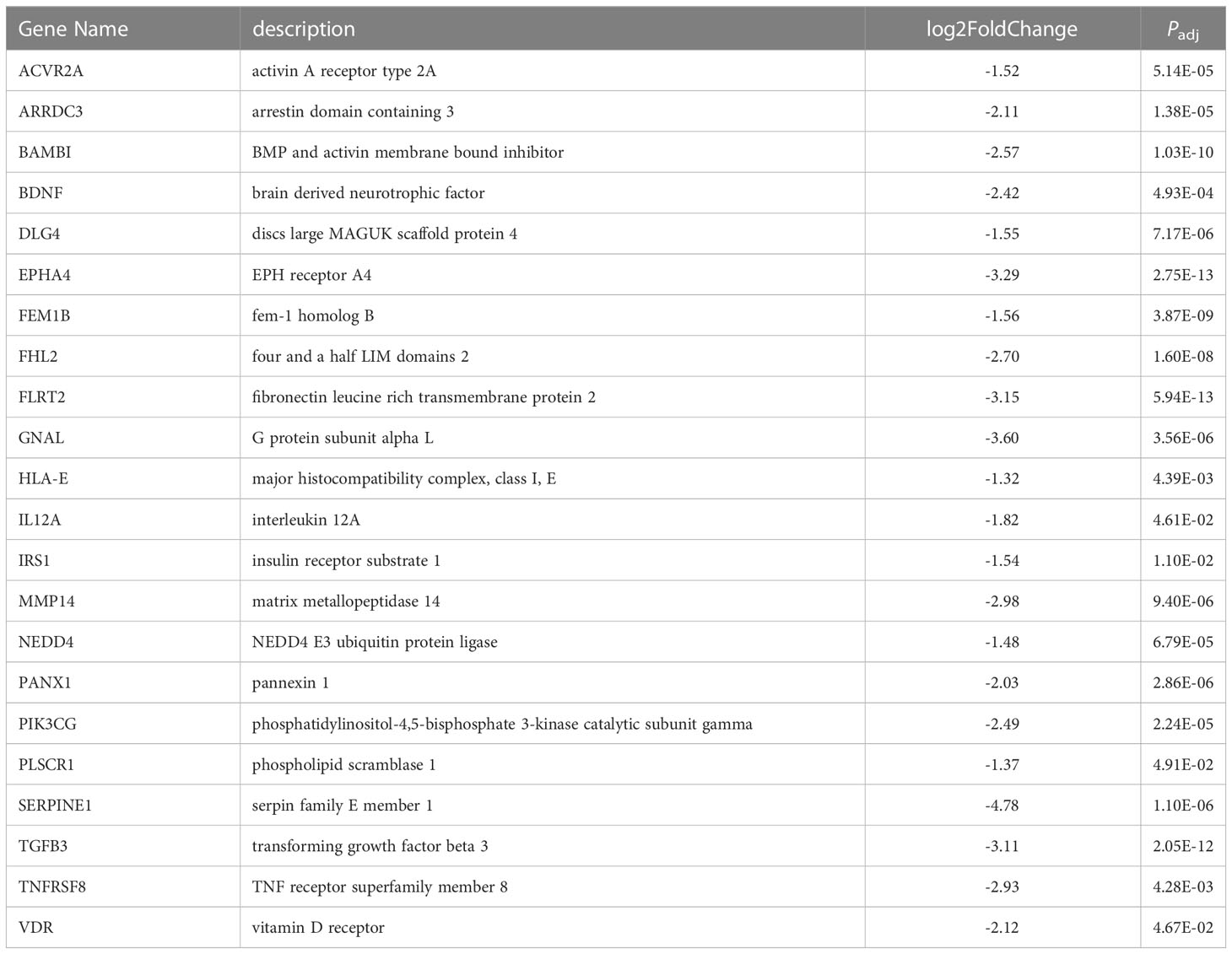
Table 4 Putative miR-10a-5p target genes downregulated in OCCC with concurrent endometriosis within the receptor signaling pathway.
MiR-10a-5p overexpression downregulating genes involved in proliferation and cell cycle progression.
The 151 genes that were downregulated in OCCC with concurrent endometriosis and were putative miR-10a-5p target genes were hand-annotated for functional roles in proliferation or cell cycle. To examine the association of miR-10a-5p expression on these hand-selected putative target genes, mature miR-10a-5p was overexpressed in OCCC cell lines and target gene expression was examined by qPCR. Overexpression of miR-10a resulted in a nearly 2-fold decrease in PALM2-AKAP2 in SMOV2 cells (P< 0.01, Figure 9A). A smaller, non-statistically significant effect was demonstrated in KK cells overexpressing miR-10a (P = 0.12, Figure 9B). PALM2-AKAP2 is a newly named fusion gene with a yet unknown function, but it has been correlated with functions similar to the previously distinct PALM2 and AKAP2 genes, such as proliferation in colorectal cancer cell lines (114). Similarly, decreased AKAP2 was shown to decrease cellular proliferation in ovarian cancer and decreased proliferation through regulation of ERK1/2 (115, 116). Overexpression of miR-10a-5p was associated with a 2.4-fold decrease in Cyclin dependent kinase 6 (CDK6) gene expression in both SMOV2 and KK cells (P< 0.05, Figures 9A, B). CDK6 is a critical molecule for cellular proliferation and cell cycle progression from G1 to S phase (117, 118). Dysregulation of CDK6 is common in cancers and has been previously been implicated in dysfunctional proliferation and disease progression in ovarian carcinomas (119, 120). Overexpression of miR-10a-5p was associated with a 2-fold decrease in RAP2A, member of RAS oncogene family (RAP2A) gene expression in SMOV2 (P< 0.01, Figure 9A) and 3-fold decrease in KK cells (P< 0.001, Figure 9B). RAP2A is involved in cellular proliferation, has been positively correlated with increased platinum resistance in gastric cancer cells, and is a downstream target of TP53 in cell cycle regulation (121–123). Overexpression of miR-10a-5p was associated with a more than 3-fold decrease in Serpin Family E Member 1 (SERPINE1) gene expression in KK cells (P = 0.01, Figure 9B). SERPINE1 has been found to increase cancer cell proliferation through its regulation by miR-10a in clear cell renal carcinoma (124). Overexpression of miR-10a-5p was associated with a non-statistically significant decrease in Ephrin type A receptor 4 (EPHA4) gene expression in SMOV2 cells (P< 0.07, Figure 9A). EPHA4 is a receptor involved in cancer cell proliferation in breast cancer cells through AKT signaling, where downregulation of EPHA4 decreased proliferation and increasing EPHA4 increased proliferation (125, 126). EPHA4 is not expressed in KK cells (data not shown).
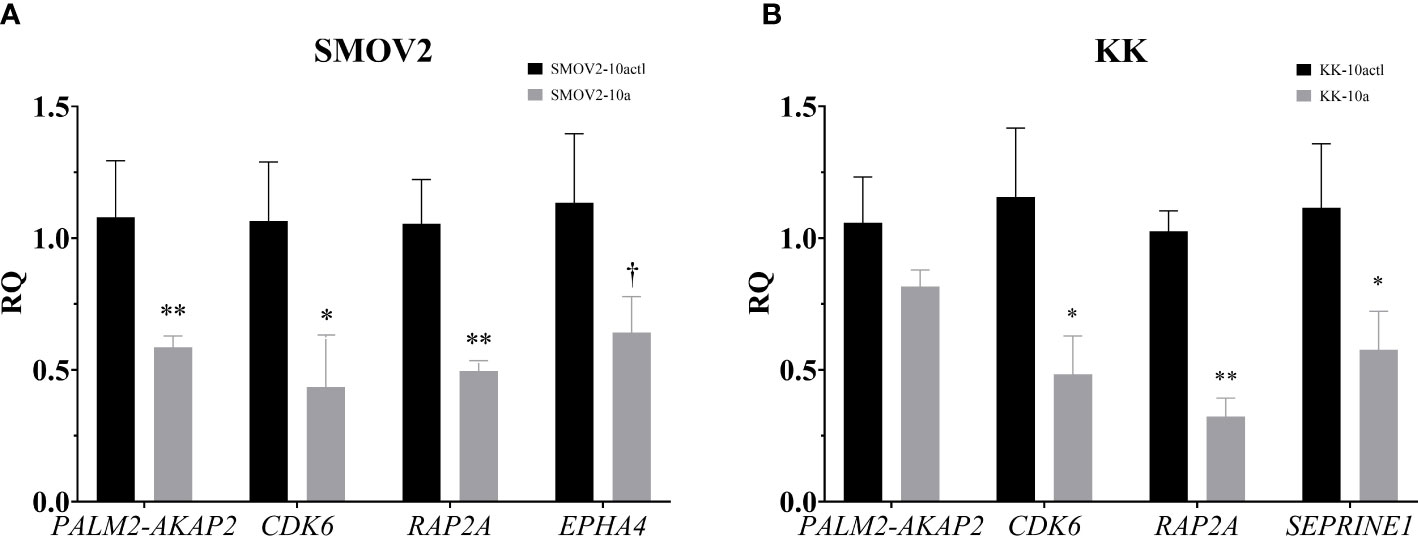
Figure 9 Putative miR-10a target genes, involved in cellular proliferation and the G1/S checkpoint, are downregulated with miR-10a-5p overexpression. Cells transfected with mature miRNA mimic for miR-10a (SMOV2-10a and KK-10a) were compared to cells transfected with negative control #1 (SMOV2-10actl and KK-10actl) for each cell line. (A) SMOV2 and (B) KK gene expression panels. RQ, the relative quantity of gene of interest to ACTB, normalized to negative control #1. Expression is plotted as mean ± SEM. Each gene was run with n = 6 for each cell line and treatment group. **P< 0.001, *P< 0.05, †P< 0.07 using unpaired Mann-Whitney or Welch’s one-tailed t-test within cell lines.
Discussion
Ovarian carcinomas are the fifth leading cause of cancer-related death for women in the United States, accounting for over 13,000 deaths annually (127). While multi-platform analyses are attempting to categorize epithelial ovarian cancers beyond histology to discover molecular features that will modulate therapeutic benefit (1–3), current first-line therapy for women with ovarian carcinomas remains similar for all histological subtypes and includes surgical debulking to remove maximum tumor tissue and six cycles of carboplatin and paclitaxel or neoadjuvant chemotherapy (128). Fortunately, 70% of women with high-grade serous ovarian carcinomas show a complete response to these standard regimens (129). Unfortunately, up to 89% of women with OCCC show progression of disease with this standard protocol (100, 130, 131). These epidemiological data highlight a critical need for further understanding of the molecular features of OCCC to improve treatment options and discoveries.
Towards this need for understanding the molecular underpinnings of ovarian cancer, large sample size, multi-platform epigenetic (i.e., DNA methylation, histone binding), genomic (i.e., whole genome sequencing, exome sequencing, targeted gene sequencing, copy number variant), transcriptomic (i.e., bulk RNA, small RNA, target gene expression), and proteomic (i.e., targeted immunohistochemistry, reverse phase protein array) studies have been undertaken. Many of the multi-platform studies utilize the much more abundant sample numbers from high-grade serous tumors (3). Publicly-available transcriptomic datasets for OCCC are available within the Gene Expression Omnibus (Supplementary Table S19), and many of these datasets are published (16–21). Some studies on OCCC utilize transcriptomic profiles from OCCC cell lines (20, 27). For example, Yamaguchi et al. (20) created an OCCC signature from OCCC cell lines and compared it to multiple published or publicly available OCCC datasets. Nagaraja et al. (27) integrated transcriptomic microarray data with small RNA data from next-generation sequencing of a panel of OCCC cell lines compared to primary cultures of normal ovarian surface epithelium.
While up to 50% of OCCCs are associated with endometriosis (22, 23), most transcriptomic studies of primary tumors classified as OCCC do not characterize samples as coming from women with concurrent endometriosis, pathology-proven endometriosis or even a history of endometriosis (16–21). One of the studies that did delineate endometriosis was Bolton et al. (16) that performed the most extensive multi-platform sequencing of OCCC. They used both genomic (n = 421 samples) and transcriptomic (n = 211 samples) profiling. While more than 10% of their samples of OCCC were classified as coming from women with endometriosis (16), they did not analyze data from OCCC with concurrent endometriosis independently from those without endometriosis. This lack of concurrent endometriosis analysis could have been due to the endometriosis being based on patient-reported history and only confirmed by histology on samples from one institution. Shih et al. (18) included endometriosis from women without OCCC, endometriosis adjacent to OCCC, atypical endometriosis, and OCCC without endometriosis. The results showed that transcriptomic profiles from endometriosis adjacent to OCCC were most similar to atypical endometriosis. Similar to our results, they showed that endometriosis from women without OCCC was distinct from OCCC (18). Although they used laser capture microdissection, Shih et al. (18) did not examine OCCC samples from women with endometriosis. They focused more on the endometriosis samples and the transcriptomic transformation from endometriosis to atypical endometriosis to OCCC. Therefore, our study is unique for its inclusion and analyses of OCCC samples with pathologically confirmed endometriosis. Similar to Shih et al. (18), we used endometrioma samples without OCCC as a comparison tissue. All OCCC with concurrent endometriosis samples were primary tumor tissue with concurrent endometriosis confirmed by pathology reports with concurrent endometriosis. Unfortunately, these strict inclusion criteria and the limited availability of samples due to OCCC’s rarity restricted the sample size in our study. While we had OCCC samples without endometriosis available for study, we did not compare OCCC transcriptomic profiles with and without endometriosis. Previously published transcriptomic studies of OCCC without endometriosis (16–21) had a significant number of samples already profiled (>200) and publicly available within GEO. Thus, we focused on well-characterized samples of OCCC with pathology-proven concurrent endometriosis compared to ovarian endometriomas, as this analysis had not been undertaken previously. Zhang et al. (7) showed unique molecular profiling in ovarian endometrioid carcinomas with and without endometriosis. Future studies will focus on analyses of publicly available OCCC datasets from women with and without endometriosis. Additionally, future studies will focus on obtaining matched endometrioma and adjacent OCCC samples from the same patient. Given the likelihood of a contribution of the endometriotic tumor microenvironment, evaluation using spatial transcriptomics would provide considerable insight.
On our well-characterized samples, we performed poly-A bulk RNA. Like previous studies in ovarian endometrioid carcinomas with concurrent endometriosis (7), we identified signaling pathways dysregulated in OCCC with concurrent endometriosis, including cytokine-cytokine receptor interaction, GPCR signaling, matrisome, and cell cycle and DNA repair pathways. Ovarian cancer cell lines are widely used as a model for epithelial ovarian carcinomas, and multiple studies have shown representative mutational, transcriptomic, and histological similarities between primary OCCC and OCCC cell lines (54, 57, 79–85). Many of these cell lines, including those derived from endometriosis-associated ovarian carcinomas (OCCC and ovarian endometrioid carcinomas), are not characterized by endometriosis status. In order to identify cell lines as the closest model of OCCC with concurrent endometriosis, our study utilized publicly available transcriptomic data for cancer cell lines, differentially expressed genes, and pathway activation scores. From this analysis we found that the OVTOKO, OVISE, RMG1, OVMANA, TOV21G, IGROV1, and JHOC5 cell lines are the cell lines with the most similar molecular profile to our OCCC with concurrent endometriosis dataset. Future studies with larger sample sizes of clinical samples would allow for a more comprehensive study of the subtle molecular nuances of the cell lines.
As a multi-platform transcriptomic approach, we also profiled small RNA molecules. To our knowledge, this represents the first small RNA sequencing from OCCC with concurrent endometriosis. Small RNA sequencing identified miR-141-3p, miR-183-5p, and miR-10a-5p as the top three most upregulated miRNAs in OCCC with concurrent endometriosis. MiR-141-3p overexpression has been demonstrated in a panel of platinum-resistant cell ovarian cancer lines (132). Further, increased expression of miR-141-3p was associated with increased cellular proliferation in esophageal cancer (133). Like miR-141-3p, studies of miR-183-5p in ovarian carcinoma are limited, but bioinformatic analysis in high-grade serous ovarian carcinoma correlated miR-183-5p with platinum-resistance (134). The specific role of these miRNAs in OCCC is currently unknown and will be crucial components in future studies.
MiR-10a-5p was the most abundant miRNA in OCCC with concurrent endometriosis, comprising 21% of the miRNA molecules. MiR-10a has been found to be upregulated in primary ductal breast carcinomas, squamous cell cervical carcinomas, acute myeloid leukemia, and pancreatic ductal adenocarcinomas and correlated with disease progression and platinum-resistance (91–98, 135, 136). Similar to other cancers, our study observed a strong, positive relationship between miR-10a-5p expression and platinum response in a panel of OCCC cell lines (R2 = 0.93). Focusing on benign disease and ovarian function, previous studies have shown that miR-10a-5p expression is significantly lower in endometriomas compared to matched and unmatched eutopic endometrium (26, 137). Further, increased expression of miR-10a-5p in granulosa cells resulted in decreased proliferation (138), consistent with our results in OCCC cell lines. Moreover, increased expression of miR-10a-5p in granulosa cells led to cell cycle deficiencies, mediated through indirect regulation of cyclin-dependent kinase 2 (138). Dysregulated genes in the cell cycle and DNA repair pathways have been implicated in OCCC and associated with its disease progression and platinum-resistant phenotype: RAP2A (139–141), CDK6 (119, 120, 142), SERPINE1 (143, 144), and EPHA4 (145–148) are involved in disease progression, drug response, and markers of progression. Overexpression of miR-10a-5p in OCCC cell lines showed an associated decrease in the expression of these putative miR-10a-5p target genes. These studies do not prove a direct effect of miR-10a-5p on this target gene expression. However, several studies have shown direct effects or associated effects of miR-10a on SERPINE1 (124) and EPHA4 (148, 149) gene expression. Figure 10 shows our working hypothesis of the role of miR-10a-5p effects on cell cycle progression in OCCC. In summary, we found a significant decrease in cellular proliferation with overexpression of miR-10a-5p. This decrease in proliferation may be due to a deficit in the G1/S checkpoint as a significant increase in cell population in G1 was seen in cell cycle analysis while also having a significant decrease in cells in S and G2 phases. Upon further evaluation of miR-10a target genes involved in proliferation, genes involved in regulating the G1/S checkpoint were downregulated in SMOV-2 and KK cells transfected to overexpress miR-10a. More specifically, the miR-10a-5p target gene, CDK6, is well known for its regulation of a cell’s progression to the S phase through its dimer with Cyclin-dependent kinase 4 (CDK4) (150). Other genes, including EPHA4 (126), RAP2A (123), and SERPINE1 (151) have been implicated in cell cycle progression. Of particular interest to the increased number of cells in G1 are CDK6 and EPHA4 with downregulation of both being linked to cells remaining in G0/G1 (126, 152). The increase in this population of cells is clinically significant for the phases of the cell cycle in which platinum and taxanes show efficacy (153, 154). These drugs are commonly effective during S and M phases causing DNA and mitotic spindle damage leading to cell death. However, if OCCC cells are overexpressing miR-10a and stuck in a senescent or earlier stage of the cell cycle for an extended period, they will be less likely to undergo damage and death from cytotoxic platinum and taxane agents, leading to resistant tumors. Future studies, including the evaluation of phosphorylated proteins, are needed to explore this hypothesis further.
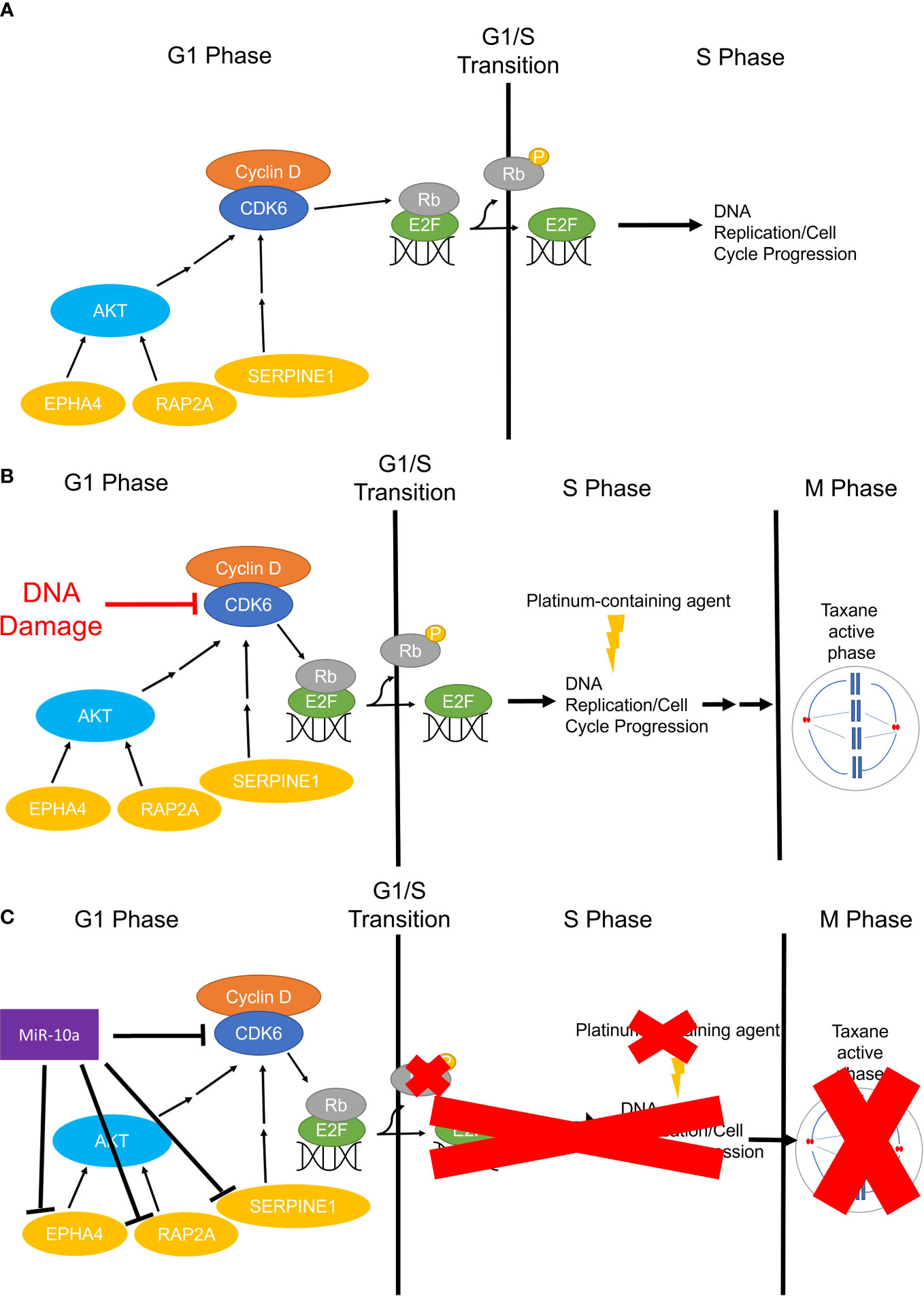
Figure 10 The working hypothesis for the mechanism of miR-10a-5p overexpression in OCCC G0 or G1/S Checkpoint. (A) Simplified representation of cell cycle progression function in non-cancerous cells, whereby CDK6 phosphorylates Rb freeing E2F for DNA replication in the S phase. (B) Infographic representation of cell cycle progression in non-malignant cells treated with platinum and/or taxane-containing agents. Cells will sustain DNA and/or microtubule damage resulting in no continued progression through the cell cycle and subsequent cell death. (C) Represents the working hypothesis for cell cycle progression in miR-10a-5p overexpressing OCCC cells. MiR-10a-5p downregulates CDK6 and other important regulators of the cell cycle slowing or halting phosphorylation of Rb leading to inactive or prolonged inactivation of E2F and transition to S Phase and DNA replication. Cells slowed in G1 or senescing in G0 miss the critical chemotherapeutic effects in the S and M phases (red “X”s).
Data availability statement
The data presented in the study are deposited in the Gene Expression Omnibus (GEO), accession number GSE230956.
Ethics statement
The studies involving human participants were reviewed and approved by Institutional Review Board (IRB) at Indiana University (#1812764043). Written informed consent for participation was not required for this study in accordance with the national legislation and the institutional requirements.
Author contributions
KC, XW, AB, DR, CZ, and SH contributed to conception and design of the study. AB performed RNA sequencing analysis. KC, XW, YK, ND, MM, and SH performed bench experiments. KC, AB, DR, CZ, KS, CC, and SH performed bioinformatic and computational analysis. KC and SH wrote the first draft of the manuscript. KC, XW, YK, ND, MM, CZ, KS, AB, DR, and SH wrote sections of the manuscript. All authors contributed to the article and approved the submitted version.
Funding
The Indiana Clinical and Translational Sciences Institute funded, in part, by Award Number UL1TR002529 from the National Institutes of Health, National Center for Advancing Translational Sciences, Clinical and Translational Sciences Award provided funds for sequencing. Sequencing analysis was carried out in the Center for Medical Genomics at Indiana University School of Medicine, which is partially supported by the Indiana University Grand Challenges Precision Health Initiative. The Biospecimen Collection and Banking Core (BC2) at the IU Simon Comprehensive Cancer Center funded by IUSCCC Support Grant P30 CA082709 provided tissue procurement and distribution service in support of this study. The NRG Oncology Biospecimen Bank (NRG BB) provided tissues from the GOG Tissue Bank (U24CA114793). The Strategic Research Initiative at Indiana University Health (to SH) and a predoctoral training fellowship through the Cancer Biology Training Program at the IU Simon Cancer Center (to KC) provided support.
Acknowledgments
We would like to thank the Center for Genomics and Bioinformatics at Indiana University Bloomington for their assistance with the RNA-seq experiments, especially James Ford for library construction and sequencing. We thank contributors, including Indiana University, who collected samples and/or data used in this study, as well as study participants whose help and participation made this work possible.
Conflict of interest
The authors declare that the research was conducted in the absence of any commercial or financial relationships that could be construed as a potential conflict of interest.
Publisher’s note
All claims expressed in this article are solely those of the authors and do not necessarily represent those of their affiliated organizations, or those of the publisher, the editors and the reviewers. Any product that may be evaluated in this article, or claim that may be made by its manufacturer, is not guaranteed or endorsed by the publisher.
Supplementary material
The Supplementary Material for this article can be found online at: https://www.frontiersin.org/articles/10.3389/fendo.2023.1162786/full#supplementary-material
References
1. Fridley BL, Dai J, Raghavan R, Li Q, Winham SJ, Hou X, et al. Transcriptomic characterization of endometrioid, clear cell, and high-grade serous epithelial ovarian carcinoma. Cancer Epidemiol Biomarkers Prev (2018) 27(9):1101–9. doi: 10.1158/1055-9965.Epi-17-0728
2. Bast RC Jr., Matulonis UA, Sood AK, Ahmed AA, Amobi AE, Balkwill FR, et al. Critical questions in ovarian cancer research and treatment: report of an American association for cancer research special conference. Cancer (2019) 125(12):1963–72. doi: 10.1002/cncr.32004
3. Cancer Genome Atlas Research N. Integrated genomic analyses of ovarian carcinoma. Nature (2011) 474(7353):609–15. doi: 10.1038/nature10166
4. Wiegand KC, Shah SP, Al-Agha OM, Zhao Y, Tse K, Zeng T, et al. Arid1a mutations in endometriosis-associated ovarian carcinomas. N Engl J Med (2010) 363(16):1532–43. doi: 10.1056/NEJMoa1008433
5. Jones S, Wang TL, Shih Ie M, Mao TL, Nakayama K, Roden R, et al. Frequent mutations of chromatin remodeling gene arid1a in ovarian clear cell carcinoma. Science (2010) 330(6001):228–31. doi: 10.1126/science.1196333
6. Ayhan A, Mao TL, Seckin T, Wu CH, Guan B, Ogawa H, et al. Loss of arid1a expression is an early molecular event in tumor progression from ovarian endometriotic cyst to clear cell and endometrioid carcinoma. Int J Gynecol Cancer (2012) 22(8):1310–5. doi: 10.1097/IGC.0b013e31826b5dcc
7. Zhang C, Wang X, Anaya Y, Parodi L, Cheng L, Anderson ML, et al. Distinct molecular pathways in ovarian endometrioid adenocarcinoma with concurrent endometriosis. Int J Cancer (2018) 143(10):2505–15. doi: 10.1002/ijc.31768
8. Wendel JRH, Wang X, Hawkins SM. The endometriotic tumor microenvironment in ovarian cancer. Cancers (Basel) (2018) 10(8):E261. doi: 10.3390/cancers10080261
9. Practice bulletin no. 114: management of endometriosis. Obstet Gynecol (2010) 116(1):223–36. doi: 10.1097/AOG.0b013e3181e8b073
10. Zondervan KT, Becker CM, Missmer SA. Endometriosis. N Engl J Med (2020) 382(13):1244–56. doi: 10.1056/NEJMra1810764
11. Saavalainen L, Lassus H, But A, Tiitinen A, Harkki P, Gissler M, et al. Risk of gynecologic cancer according to the type of endometriosis. Obstet Gynecol (2018) 131(6):1095–102. doi: 10.1097/AOG.0000000000002624
12. Pearce CL, Templeman C, Rossing MA, Lee A, Near AM, Webb PM, et al. Association between endometriosis and risk of histological subtypes of ovarian cancer: A pooled analysis of case-control studies. Lancet Oncol (2012) 13(4):385–94. doi: 10.1016/S1470-2045(11)70404-1
13. Seidman JD, Horkayne-Szakaly I, Haiba M, Boice CR, Kurman RJ, Ronnett BM. The histologic type and stage distribution of ovarian carcinomas of surface epithelial origin. Int J Gynecol Pathol (2004) 23(1):41–4. doi: 10.1097/01.pgp.0000101080.35393.16
14. Machida H, Matsuo K, Yamagami W, Ebina Y, Kobayashi Y, Tabata T, et al. Trends and characteristics of epithelial ovarian cancer in Japan between 2002 and 2015: A jsgo-jsog joint study. Gynecol Oncol (2019) 153(3):589–96. doi: 10.1016/j.ygyno.2019.03.243
15. Kobel M, Kalloger SE, Huntsman DG, Santos JL, Swenerton KD, Seidman JD, et al. Differences in tumor type in low-stage versus high-stage ovarian carcinomas. Int J Gynecol Pathol (2010) 29(3):203–11. doi: 10.1097/PGP.0b013e3181c042b6
16. Bolton KL, Chen D, Corona de la Fuente R, Fu Z, Murali R, Kobel M, et al. Molecular subclasses of clear cell ovarian carcinoma and their impact on disease behavior and outcomes. Clin Cancer Res (2022) 28(22):4947–56. doi: 10.1158/1078-0432.CCR-21-3817
17. Mortlock S, Corona RI, Kho PF, Pharoah P, Seo JH, Freedman ML, et al. A multi-level investigation of the genetic relationship between endometriosis and ovarian cancer histotypes. Cell Rep Med (2022) 3(3):100542. doi: 10.1016/j.xcrm.2022.100542
18. Shin HY, Yang W, Chay DB, Lee EJ, Chung JY, Kim HS, et al. Tetraspanin 1 promotes endometriosis leading to ovarian clear cell carcinoma. Mol Oncol (2021) 15(4):987–1004. doi: 10.1002/1878-0261.12884
19. Suryawanshi S, Huang X, Elishaev E, Budiu RA, Zhang L, Kim S, et al. Complement pathway is frequently altered in endometriosis and endometriosis-associated ovarian cancer. Clin Cancer Res (2014) 20(23):6163–74. doi: 10.1158/1078-0432.CCR-14-1338
20. Yamaguchi K, Mandai M, Oura T, Matsumura N, Hamanishi J, Baba T, et al. Identification of an ovarian clear cell carcinoma gene signature that reflects inherent disease biology and the carcinogenic processes. Oncogene (2010) 29(12):1741–52. doi: 10.1038/onc.2009.470
21. Marquez RT, Baggerly KA, Patterson AP, Liu J, Broaddus R, Frumovitz M, et al. Patterns of gene expression in different histotypes of epithelial ovarian cancer correlate with those in normal fallopian tube, endometrium, and colon. Clin Cancer Res (2005) 11(17):6116–26. doi: 10.1158/1078-0432.CCR-04-2509
22. Jimbo H, Yoshikawa H, Onda T, Yasugi T, Sakamoto A, Taketani Y. Prevalence of ovarian endometriosis in epithelial ovarian cancer. Int J Gynaecol Obstet (1997) 59(3):245–50. doi: 10.1016/s0020-7292(97)00238-5
23. Stamp JP, Gilks CB, Wesseling M, Eshragh S, Ceballos K, Anglesio MS, et al. Baf250a expression in atypical endometriosis and endometriosis-associated ovarian cancer. Int J Gynecol Cancer (2016) 26(5):825–32. doi: 10.1097/IGC.0000000000000698
24. Logan M, Hawkins SM. Role of micrornas in cancers of the female reproductive tract: insights from recent clinical and experimental discovery studies. Clin Sci (Lond) (2015) 128(3):153–80. doi: 10.1042/CS20140087
25. Wang X, Ivan M, Hawkins SM. The role of microrna molecules and microrna-regulating machinery in the pathogenesis and progression of epithelial ovarian cancer. Gynecol Oncol (2017) 147(2):481–7. doi: 10.1016/j.ygyno.2017.08.027
26. Hawkins SM, Creighton CJ, Han DY, Zariff A, Anderson ML, Gunaratne PH, et al. Functional microrna involved in endometriosis. Mol Endocrinol (2011) 25(5):821–32. doi: 10.1210/me.2010-0371
27. Nagaraja AK, Creighton CJ, Yu Z, Zhu H, Gunaratne PH, Reid JG, et al. A link between mir-100 and frap1/mtor in clear cell ovarian cancer. Mol Endocrinol (2010) 24(2):447–63. doi: 10.1210/me.2009-0295
28. Chen S, Zhou Y, Chen Y, Gu J. Fastp: an ultra-fast all-in-one fastq preprocessor. Bioinformatics (2018) 34(17):i884–i90. doi: 10.1093/bioinformatics/bty560
29. Kim D, Langmead B, Salzberg SL. Hisat: A fast spliced aligner with low memory requirements. Nat Methods (2015) 12(4):357–60. doi: 10.1038/nmeth.3317
30. Langmead B, Salzberg SL. Fast gapped-read alignment with bowtie 2. Nat Methods (2012) 9(4):357–9. doi: 10.1038/nmeth.1923
31. Liao Y, Smyth GK, Shi W. Featurecounts: an efficient general purpose program for assigning sequence reads to genomic features. Bioinformatics (2014) 30(7):923–30. doi: 10.1093/bioinformatics/btt656
32. Frankish A, Diekhans M, Ferreira AM, Johnson R, Jungreis I, Loveland J, et al. Gencode reference annotation for the human and mouse genomes. Nucleic Acids Res (2019) 47(D1):D766–D73. doi: 10.1093/nar/gky955
33. Frankish A, Diekhans M, Jungreis I, Lagarde J, Loveland JE, Mudge JM, et al. Gencode 2021. Nucleic Acids Res (2021) 49(D1):D916–D23. doi: 10.1093/nar/gkaa1087
34. Friedlander MR, Chen W, Adamidi C, Maaskola J, Einspanier R, Knespel S, et al. Discovering micrornas from deep sequencing data using mirdeep. Nat Biotechnol (2008) 26(4):407–15. doi: 10.1038/nbt1394
35. Kozomara A, Griffiths-Jones S. Mirbase: annotating high confidence micrornas using deep sequencing data. Nucleic Acids Res (2014) 42(Database issue):D68–73. doi: 10.1093/nar/gkt1181
36. Kozomara A, Birgaoanu M, Griffiths-Jones S. Mirbase: from microrna sequences to function. Nucleic Acids Res (2019) 47(D1):D155–D62. doi: 10.1093/nar/gky1141
37. Langmead B, Trapnell C, Pop M, Salzberg SL. Ultrafast and memory-efficient alignment of short DNA sequences to the human genome. Genome Biol (2009) 10(3):R25. doi: 10.1186/gb-2009-10-3-r25
38. Love MI, Huber W, Anders S. Moderated estimation of fold change and dispersion for rna-seq data with deseq2. Genome Biol (2014) 15(12):550. doi: 10.1186/s13059-014-0550-8
39. Banu SK, Lee J, Starzinski-Powitz A, Arosh JA. Gene expression profiles and functional characterization of human immortalized endometriotic epithelial and stromal cells. Fertil Steril (2008) 90(4):972–87. doi: 10.1016/j.fertnstert.2007.07.1358
40. QPCR standard curve . Available at: https://www.sigmaaldrich.com/US/en/technical-documents/protocol/genomics/qpcr/qpcr-efficiency-determination.
41. Bustin SA, Benes V, Garson JA, Hellemans J, Huggett J, Kubista M, et al. Primer sequence disclosure: A clarification of the miqe guidelines. Clin Chem (2011) 57(6):919–21. doi: 10.1373/clinchem.2011.162958
42. Yu Z, Kim J, He L, Creighton CJ, Gunaratne PH, Hawkins SM, et al. Functional analysis of mir-34c as a putative tumor suppressor in high-grade serous ovarian cancer. Biol Reprod (2014) 91(5):113. doi: 10.1095/biolreprod.114.121988
43. Livak KJ, Schmittgen TD. Analysis of relative gene expression data using real-time quantitative pcr and the 2(-delta delta C(T)) method. Methods (2001) 25(4):402–8. doi: 10.1006/meth.2001.1262
44. Faul F, Erdfelder E, Lang AG, Buchner A. G*Power 3: A flexible statistical power analysis program for the social, behavioral, and biomedical sciences. Behav Res Methods (2007) 39(2):175–91. doi: 10.3758/bf03193146
45. Faul F, Erdfelder E, Buchner A, Lang AG. Statistical power analyses using G*Power 3.1: tests for correlation and regression analyses. Behav Res Methods (2009) 41(4):1149–60. doi: 10.3758/BRM.41.4.1149
46. Subramanian A, Tamayo P, Mootha VK, Mukherjee S, Ebert BL, Gillette MA, et al. Gene set enrichment analysis: A knowledge-based approach for interpreting genome-wide expression profiles. Proc Natl Acad Sci U.S.A. (2005) 102(43):15545–50. doi: 10.1073/pnas.0506580102
47. Liberzon A, Birger C, Thorvaldsdóttir H, Ghandi M, Mesirov JP, Tamayo P. The molecular signatures database (Msigdb) hallmark gene set collection. Cell Syst (2015) 1(6):417–25. doi: 10.1016/j.cels.2015.12.004
48. Liberzon A, Subramanian A, Pinchback R, Thorvaldsdottir H, Tamayo P, Mesirov JP. Molecular signatures database (Msigdb) 3.0. Bioinformatics (2011) 27(12):1739–40. doi: 10.1093/bioinformatics/btr260
49. Barretina J, Caponigro G, Stransky N, Venkatesan K, Margolin AA, Kim S, et al. The cancer cell line encyclopedia enables predictive modelling of anticancer drug sensitivity. Nature (2012) 483(7391):603–7. doi: 10.1038/nature11003
50. Lu X, Tu SW, Chang W, Wan C, Wang J, Zang Y, et al. Ssmd: A semi-supervised approach for a robust cell type identification and deconvolution of mouse transcriptomics data. Brief Bioinform (2021) 22(4):1–13. doi: 10.1093/bib/bbaa307
51. Wan C, Chang W, Zhang Y, Shah F, Lu X, Zang Y, et al. Ltmg: A novel statistical modeling of transcriptional expression states in single-cell rna-seq data. Nucleic Acids Res (2019) 47(18):e111. doi: 10.1093/nar/gkz655
52. Lau DH, Lewis AD, Ehsan MN, Sikic BI. Multifactorial mechanisms associated with broad cross-resistance of ovarian carcinoma cells selected by cyanomorpholino doxorubicin. Cancer Res (1991) 51(19):5181–7.
53. Lau DH, Ross KL, Sikic BI. Paradoxical increase in DNA cross-linking in a human ovarian carcinoma cell line resistant to cyanomorpholino doxorubicin. Cancer Res (1990) 50(13):4056–60.
54. Provencher DM, Lounis H, Champoux L, Tetrault M, Manderson EN, Wang JC, et al. Characterization of four novel epithelial ovarian cancer cell lines. In Vitro Cell Dev Biol Anim (2000) 36(6):357–61. doi: 10.1290/1071-2690(2000)036<0357:COFNEO>2.0.CO;2
55. Alley MC, Scudiero DA, Monks A, Hursey ML, Czerwinski MJ, Fine DL, et al. Feasibility of drug screening with panels of human tumor cell lines using a microculture tetrazolium assay. Cancer Res (1988) 48(3):589–601.
56. Yu D, Wolf JK, Scanlon M, Price JE, Hung MC. Enhanced C-erbb-2/neu expression in human ovarian cancer cells correlates with more severe Malignancy that can be suppressed by E1a. Cancer Res (1993) 53(4):891–8.
57. Gorai I, Nakazawa T, Miyagi E, Hirahara F, Nagashima Y, Minaguchi H. Establishment and characterization of two human ovarian clear cell adenocarcinoma lines from metastatic lesions with different properties. Gynecol Oncol (1995) 57(1):33–46. doi: 10.1006/gyno.1995.1097
58. Morisawa T, Kuramoto H, Shimoda T, Sakamoto I, Kato Y, Hamano M. [Establishment and characterization of a new scc antigen producing cell line (Hcs-2) from a carcinoma of the uterine cervix]. Hum Cell (1988) 1(3):308–14.
59. Sasa H, Ishii K, Hirata J, Kikuchi Y, Nagata I, Kawai T, et al. Establishment and characterization of a ca125-producing human ovarian clear cell carcinoma cell line. Hum Cell (1993) 6(4):279–86.
60. Yonamine K, Hayashi K, Iida T. Establishment and characterization of human ovarian clear cell adenocarcinoma cell line (Smov-2), and its cytotoxity by anticancer agents. Hum Cell (1999) 12(3):139–48.
61. Eva A, Robbins KC, Andersen PR, Srinivasan A, Tronick SR, Reddy EP, et al. Cellular genes analogous to retroviral onc genes are transcribed in human tumour cells. Nature (1982) 295(5845):116–9. doi: 10.1038/295116a0
62. Li M, Balch C, Montgomery JS, Jeong M, Chung JH, Yan P, et al. Integrated analysis of DNA methylation and gene expression reveals specific signaling pathways associated with platinum resistance in ovarian cancer. BMC Med Genomics (2009) 2:34. doi: 10.1186/1755-8794-2-34
63. Fogh J, Fogh JM, Orfeo T. One hundred and twenty-seven cultured human tumor cell lines producing tumors in nude mice. J Natl Cancer Inst (1977) 59(1):221–6. doi: 10.1093/jnci/59.1.221
64. Nozawa S, Tsukazaki K, Sakayori M, Jeng CH, Iizuka R. Establishment of a human ovarian clear cell carcinoma cell line (Rmg-I) and its single cell cloning–with special reference to the stem cell of the tumor. Hum Cell (1988) 1(4):426–35.
65. Creighton CJ, Nagaraja AK, Hanash SM, Matzuk MM, Gunaratne PH. A bioinformatics tool for linking gene expression profiling results with public databases of microrna target predictions. RNA (2008) 14(11):2290–6. doi: 10.1261/rna.1188208
66. Lewis BP, Burge CB, Bartel DP. Conserved seed pairing, often flanked by adenosines, indicates that thousands of human genes are microrna targets. Cell (2005) 120(1):15–20. doi: 10.1016/j.cell.2004.12.035
67. Lewis BP, Shih IH, Jones-Rhoades MW, Bartel DP, Burge CB. Prediction of mamMalian microrna targets. Cell (2003) 115(7):787–98. doi: 10.1016/s0092-8674(03)01018-3
68. Agarwal V, Bell GW, Nam JW, Bartel DP. Predicting effective microrna target sites in mamMalian mrnas. Elife (2015) 4:e05005. doi: 10.7554/eLife.05005
69. Liu W, Wang X. Prediction of functional microrna targets by integrative modeling of microrna binding and target expression data. Genome Biol (2019) 20(1):18. doi: 10.1186/s13059-019-1629-z
70. Chen Y, Wang X. Mirdb: an online database for prediction of functional microrna targets. Nucleic Acids Res (2020) 48(D1):D127–D31. doi: 10.1093/nar/gkz757
71. Huang HY, Lin YC, Cui S, Huang Y, Tang Y, Xu J, et al. Mirtarbase update 2022: an informative resource for experimentally validated mirna-target interactions. Nucleic Acids Res (2022) 50(D1):D222–D30. doi: 10.1093/nar/gkab1079
72. Prat J, Belhadj H, Berek J, Bermudez A, Bhatla N, Cain J, et al. Abridged republication of figo's staging classification for cancer of the ovary, fallopian tube, and peritoneum. Eur J Gynaecol Oncol (2015) 36(4):367–9.
73. Chen Y, Meltzer PS. Gene expression analysis via multidimensional scaling. Curr Protoc Bioinf (2005) 10:7.11.1–7.11.9. doi: 10.1002/0471250953.bi0711s10
74. Yamamoto S, Tsuda H, Miyai K, Takano M, Tamai S, Matsubara O. Cumulative alterations of P27-related cell-cycle regulators in the development of endometriosis-associated ovarian clear cell adenocarcinoma. Histopathology (2010) 56(6):740–9. doi: 10.1111/j.1365-2559.2010.03551.x
75. Hever A, Roth RB, Hevezi P, Marin ME, Acosta JA, Acosta H, et al. Human endometriosis is associated with plasma cells and overexpression of B lymphocyte stimulator. Proc Natl Acad Sci U.S.A. (2007) 104(30):12451–6. doi: 10.1073/pnas.0703451104
76. Chand AL, Murray AS, Jones RL, Hannan NJ, Salamonsen LA, Rombauts L. Laser capture microdissection and cdna array analysis of endometrium identify ccl16 and ccl21 as epithelial-derived inflammatory mediators associated with endometriosis. Reprod Biol Endocrinol (2007) 5:18. doi: 10.1186/1477-7827-5-18
77. Lawson C, Bourcier N, Al-Akoum M, Maheux R, Naud F, Akoum A. Abnormal interleukin 1 receptor types I and II gene expression in eutopic and ectopic endometrial tissues of women with endometriosis. J Reprod Immunol (2008) 77(1):75–84. doi: 10.1016/j.jri.2007.04.002
78. Zhao H, Wang Q, Bai C, He K, Pan Y. A cross-study gene set enrichment analysis identifies critical pathways in endometriosis. Reprod Biol Endocrinol (2009) 7:94. doi: 10.1186/1477-7827-7-94
79. Beaufort CM, Helmijr JC, Piskorz AM, Hoogstraat M, Ruigrok-Ritstier K, Besselink N, et al. Ovarian cancer cell line panel (Occp): clinical importance of in vitro morphological subtypes. PloS One (2014) 9(9):e103988. doi: 10.1371/journal.pone.0103988
80. Anglesio MS, Wiegand KC, Melnyk N, Chow C, Salamanca C, Prentice LM, et al. Type-specific cell line models for type-specific ovarian cancer research. PloS One (2013) 8(9):e72162. doi: 10.1371/journal.pone.0072162
81. Papp E, Hallberg D, Konecny GE, Bruhm DC, Adleff V, Noe M, et al. Integrated genomic, epigenomic, and expression analyses of ovarian cancer cell lines. Cell Rep (2018) 25(9):2617–33. doi: 10.1016/j.celrep.2018.10.096
82. Barnes BM, Nelson L, Tighe A, Burghel GJ, Lin IH, Desai S, et al. Distinct transcriptional programs stratify ovarian cancer cell lines into the five major histological subtypes. Genome Med (2021) 13(1):140. doi: 10.1186/s13073-021-00952-5
83. McCabe A, Zaheed O, McDade SS, Dean K. Investigating the suitability of in vitro cell lines as models for the major subtypes of epithelial ovarian cancer. Front Cell Dev Biol (2023) 11:1104514. doi: 10.3389/fcell.2023.1104514
84. Tan TZ, Ye J, Yee CV, Lim D, Ngoi NYL, Tan DSP, et al. Analysis of gene expression signatures identifies prognostic and functionally distinct ovarian clear cell carcinoma subtypes. EBioMedicine (2019) 50:203–10. doi: 10.1016/j.ebiom.2019.11.017
85. Domcke S, Sinha R, Levine DA, Sander C, Schultz N. Evaluating cell lines as tumour models by comparison of genomic profiles. Nat Commun (2013) 4:2126. doi: 10.1038/ncomms3126
86. Ditto A, De Cecco L, Paolini B, Alberti P, Martinelli F, Leone Roberti Maggiore U, et al. Validation of mirovar, a microrna-based predictor of early relapse in early stage epithelial ovarian cancer as a new strategy to optimise patients' Prognostic assessment. Eur J Cancer (2022) 161:55–63. doi: 10.1016/j.ejca.2021.11.003
87. Bagnoli M, Canevari S, Califano D, Losito S, Maio MD, Raspagliesi F, et al. Development and validation of a microrna-based signature (Mirovar) to predict early relapse or progression of epithelial ovarian cancer: A cohort study. Lancet Oncol (2016) 17(8):1137–46. doi: 10.1016/S1470-2045(16)30108-5
88. Vilming Elgaaen B, Olstad OK, Haug KB, Brusletto B, Sandvik L, Staff AC, et al. Global mirna expression analysis of serous and clear cell ovarian carcinomas identifies differentially expressed mirnas including mir-200c-3p as a prognostic marker. BMC Cancer (2014) 14:80. doi: 10.1186/1471-2407-14-80
89. Wyman SK, Parkin RK, Mitchell PS, Fritz BR, O'Briant K, Godwin AK, et al. Repertoire of micrornas in epithelial ovarian cancer as determined by next generation sequencing of small rna cdna libraries. PloS One (2009) 4(4):e5311. doi: 10.1371/journal.pone.0005311
90. Dahiya N, Sherman-Baust CA, Wang TL, Davidson B, Shih Ie M, Zhang Y, et al. Microrna expression and identification of putative mirna targets in ovarian cancer. PloS One (2008) 3(6):e2436. doi: 10.1371/journal.pone.0002436
91. Yu T, Liu L, Li J, Yan M, Lin H, Liu Y, et al. Mirna-10a is upregulated in nsclc and may promote cancer by targeting pten. Oncotarget (2015) 6(30):30239–50. doi: 10.18632/oncotarget.4972
92. Chang CH, Fan TC, Yu JC, Liao GS, Lin YC, Shih AC, et al. The prognostic significance of runx2 and mir-10a/10b and their inter-relationship in breast cancer. J Transl Med (2014) 12:257. doi: 10.1186/s12967-014-0257-3
93. Xiong G, Huang H, Feng M, Yang G, Zheng S, You L, et al. Mir-10a-5p targets tfap2c to promote gemcitabine resistance in pancreatic ductal adenocarcinoma. J Exp Clin Cancer Res (2018) 37(1):76. doi: 10.1186/s13046-018-0739-x
94. Zeng T, Li G. Microrna−10a enhances the metastatic potential of cervical cancer cells by targeting phosphatase and tensin homologue. Mol Med Rep (2014) 10(3):1377–82. doi: 10.3892/mmr.2014.2370
95. Long MJ, Wu FX, Li P, Liu M, Li X, Tang H. Microrna-10a targets chl1 and promotes cell growth, migration and invasion in human cervical cancer cells. Cancer Lett (2012) 324(2):186–96. doi: 10.1016/j.canlet.2012.05.022
96. Sun W, Ma Y, Chen P, Wang D. Microrna-10a silencing reverses cisplatin resistance in the A549/cisplatin human lung cancer cell line via the transforming growth factor-beta/smad2/stat3/stat5 pathway. Mol Med Rep (2015) 11(5):3854–9. doi: 10.3892/mmr.2015.3181
97. Pogribny IP, Filkowski JN, Tryndyak VP, Golubov A, Shpyleva SI, Kovalchuk O. Alterations of micrornas and their targets are associated with acquired resistance of mcf-7 breast cancer cells to cisplatin. Int J Cancer (2010) 127(8):1785–94. doi: 10.1002/ijc.25191
98. Lee JW, Choi CH, Choi JJ, Park YA, Kim SJ, Hwang SY, et al. Altered microrna expression in cervical carcinomas. Clin Cancer Res (2008) 14(9):2535–42. doi: 10.1158/1078-0432.CCR-07-1231
99. Huang T, Ren K, Ding G, Yang L, Wen Y, Peng B, et al. Mir10a increases the cisplatin resistance of lung adenocarcinoma circulating tumor cells via targeting pik3ca in the pi3k/akt pathway. Oncol Rep (2020) 43(6):1906–14. doi: 10.3892/or.2020.7547
100. Sugiyama T, Okamoto A, Enomoto T, Hamano T, Aotani E, Terao Y, et al. Randomized phase iii trial of irinotecan plus cisplatin compared with paclitaxel plus carboplatin as first-line chemotherapy for ovarian clear cell carcinoma: jgog3017/gcig trial. J Clin Oncol (2016) 34(24):2881–7. doi: 10.1200/JCO.2016.66.9010
101. Utsunomiya H, Akahira J, Tanno S, Moriya T, Toyoshima M, Niikura H, et al. Paclitaxel-platinum combination chemotherapy for advanced or recurrent ovarian clear cell adenocarcinoma: A multicenter trial. Int J Gynecol Cancer (2006) 16(1):52–6. doi: 10.1111/j.1525-1438.2006.00289.x
102. Goff BA, Sainz de la Cuesta R, Muntz HG, Fleischhacker D, Ek M, Rice LW, et al. Clear cell carcinoma of the ovary: A distinct histologic type with poor prognosis and resistance to platinum-based chemotherapy in stage III disease. Gynecol Oncol (1996) 60(3):412–7. doi: 10.1006/gyno.1996.0065
103. Sugiyama T, Kamura T, Kigawa J, Terakawa N, Kikuchi Y, Kita T, et al. Clinical characteristics of clear cell carcinoma of the ovary: A distinct histologic type with poor prognosis and resistance to platinum-based chemotherapy. Cancer (2000) 88(11):2584–9. doi: 10.1002/1097-0142(20000601)88:11<2584::AID-CNCR22>3.0.CO;2-5
104. Takano M, Kikuchi Y, Yaegashi N, Kuzuya K, Ueki M, Tsuda H, et al. Clear cell carcinoma of the ovary: A retrospective multicentre experience of 254 patients with complete surgical staging. Br J Cancer (2006) 94(10):1369–74. doi: 10.1038/sj.bjc.6603116
105. Ho CM, Huang YJ, Chen TC, Huang SH, Liu FS, Chang Chien CC, et al. Pure-type clear cell carcinoma of the ovary as a distinct histological type and improved survival in patients treated with paclitaxel-platinum-based chemotherapy in pure-type advanced disease. Gynecol Oncol (2004) 94(1):197–203. doi: 10.1016/j.ygyno.2004.04.004
106. Pectasides D, Fountzilas G, Aravantinos G, Kalofonos C, Efstathiou H, Farmakis D, et al. Advanced stage clear-cell epithelial ovarian cancer: the hellenic cooperative oncology group experience. Gynecol Oncol (2006) 102(2):285–91. doi: 10.1016/j.ygyno.2005.12.038
107. Sun W, Ma Y, Chen P, Wang D. Microrna-10a silencing reverses cisplatin resistance in the A549/cisplatin human lung cancer cell line via the transforming growth factor-β/smad2/stat3/stat5 pathway. Mol Med Rep (2015) 11(5):3854–9. doi: 10.3892/mmr.2015.3181
108. Zhu H, Kang M, Bai X. Tcf21 regulates mir-10a-5p/lin28b signaling to block the proliferation and invasion of melanoma cells. PloS One (2021) 16(8):e0255971. doi: 10.1371/journal.pone.0255971
109. Yuan Z, Wang W. Lncrna snhg4 regulates mir-10a/pten to inhibit the proliferation of acute myeloid leukemia cells. Hematology (2020) 25(1):160–4. doi: 10.1080/16078454.2020.1754636
110. Zhu M, Liu C, Chen S, Zhang C, Zhou P, Sun J, et al. Circrna circsept9 downregulates mir-10a through methylation to promote cell proliferation in laryngeal squamous cell carcinoma. Crit Rev Eukaryot Gene Expr (2021) 31(6):17–22. doi: 10.1615/CritRevEukaryotGeneExpr.2021039092
111. He L, Hannon GJ. Micrornas: small rnas with a big role in gene regulation. Nat Rev Genet (2004) 5(7):522–31. doi: 10.1038/nrg1379
112. Friedman RC, Farh KK, Burge CB, Bartel DP. Most mamMalian mrnas are conserved targets of micrornas. Genome Res (2009) 19(1):92–105. doi: 10.1101/gr.082701.108
113. Wang J, Vasaikar S, Shi Z, Greer M, Zhang B. Webgestalt 2017: A more comprehensive, powerful, flexible and interactive gene set enrichment analysis toolkit. Nucleic Acids Res (2017) 45(W1):W130–W7. doi: 10.1093/nar/gkx356
114. Bracker TU, Sommer A, Fichtner I, Faus H, Haendler B, Hess-Stumpp H. Efficacy of ms-275, a selective inhibitor of class I histone deacetylases, in human colon cancer models. Int J Oncol (2009) 35(4):909–20. doi: 10.3892/ijo_00000406
115. Li X, Wang C, Zhang G, Liang M, Zhang B. Akap2 is upregulated in ovarian cancer, and promotes growth and migration of cancer cells. Mol Med Rep (2017) 16(4):5151–6. doi: 10.3892/mmr.2017.7286
116. Wang B, Jiang B, Li Y, Dai Y, Li P, Li L, et al. Akap2 overexpression modulates growth plate chondrocyte functions through erk1/2 signaling. Bone (2021) 146:115875. doi: 10.1016/j.bone.2021.115875
117. Meyerson M, Harlow E. Identification of G1 kinase activity for cdk6, a novel cyclin D partner. Mol Cell Biol (1994) 14(3):2077–86. doi: 10.1128/mcb.14.3.2077-2086.1994
118. Romero-Pozuelo J, Figlia G, Kaya O, Martin-Villalba A, Teleman AA. Cdk4 and cdk6 couple the cell-cycle machinery to cell growth via mtorc1. Cell Rep (2020) 31(2):107504. doi: 10.1016/j.celrep.2020.03.068
119. Cao J, Zhang Y, Mu J, Yang D, Gu X, Zhang J. Exosomal mir-21-5p contributes to ovarian cancer progression by regulating cdk6. Hum Cell (2021) 34(4):1185–96. doi: 10.1007/s13577-021-00522-2
120. Duan L, Yan Y, Wang G, Xing YL, Sun J, Wang LL. Muir-182-5p functions as a tumor suppressor to sensitize human ovarian cancer cells to cisplatin through direct targeting the cyclin dependent kinase 6 (Cdk6). J BUON (2020) 25(5):2279–86.
121. Wang T, Wang J, Liang R, He J, Liao Q, Jiang Y. Rap2a/P-erk1/2 is involved in glioma proliferation and migration. Curr Mol Med (2022). doi: 10.2174/1566524022666220804152049
122. Zhang J, Wei Y, Min J, Wang Y, Yin L, Cao G, et al. Knockdown of rap2a gene expression suppresses cisplatin resistance in gastric cancer cells. Oncol Lett (2020) 19(1):350–8. doi: 10.3892/ol.2019.11086
123. Wu JX, Zhang DG, Zheng JN, Pei DS. Rap2a is a novel target gene of P53 and regulates cancer cell migration and invasion. Cell Signal (2015) 27(6):1198–207. doi: 10.1016/j.cellsig.2015.02.026
124. Liu RJ, Xu ZP, Li SY, Yu JJ, Feng NH, Xu B, et al. Bap1-related cerna (Neat1/mir-10a-5p/serpine1) promotes proliferation and migration of kidney cancer cells. Front Oncol (2022) 12:852515. doi: 10.3389/fonc.2022.852515
125. Dong Y, Liu Y, Jiang A, Li R, Yin M, Wang Y. Microrna-335 suppresses the proliferation, migration, and invasion of breast cancer cells by targeting epha4. Mol Cell Biochem (2018) 439(1-2):95–104. doi: 10.1007/s11010-017-3139-1
126. Ding L, Shen Y, Ni J, Ou Y, Ou Y, Liu H. Epha4 promotes cell proliferation and cell adhesion-mediated drug resistance via the akt pathway in multiple myeloma. Tumour Biol (2017) 39(3):1010428317694298. doi: 10.1177/1010428317694298
127. Siegel RL, Miller KD, Wagle NS, Jemal A. Cancer statistics, 2023. CA Cancer J Clin (2023) 73(1):17–48. doi: 10.3322/caac.21763
128. Armstrong DK, Alvarez RD, Bakkum-Gamez JN, Barroilhet L, Behbakht K, Berchuck A, et al. Nccn guidelines insights: ovarian cancer, version 1.2019. J Natl Compr Canc Netw (2019) 17(8):896–909. doi: 10.6004/jnccn.2019.0039
129. Bast RC Jr., Hennessy B, Mills GB. The biology of ovarian cancer: new opportunities for translation. Nat Rev Cancer (2009) 9(6):415–28. doi: 10.1038/nrc2644
130. Itamochi H, Kigawa J, Sugiyama T, Kikuchi Y, Suzuki M, Terakawa N. Low proliferation activity may be associated with chemoresistance in clear cell carcinoma of the ovary. Obstet Gynecol (2002) 100(2):281–7. doi: 10.1016/s0029-7844(02)02040-9
131. Itamochi H, Kigawa J, Terakawa N. Mechanisms of chemoresistance and poor prognosis in ovarian clear cell carcinoma. Cancer Sci (2008) 99(4):653–8. doi: 10.1111/j.1349-7006.2008.00747.x
132. Ying HC, Xu HY, Lv J, Ying TS, Yang Q. Microrna signatures of platinum-resistance in ovarian cancer. Eur J Gynaecol Oncol (2015) 36(1):16–20.
133. Phatak P, Noe M, Asrani K, Chesnick IE, Greenwald BD, Donahue JM. Microrna-141-3p regulates cellular proliferation, migration, and invasion in esophageal cancer by targeting tuberous sclerosis complex 1. Mol Carcinog (2021) 60(2):125–37. doi: 10.1002/mc.23274
134. Qi X, Yu C, Wang Y, Lin Y, Shen B. Network vulnerability-based and knowledge-guided identification of microrna biomarkers indicating platinum resistance in high-grade serous ovarian cancer. Clin Transl Med (2019) 8(1):28. doi: 10.1186/s40169-019-0245-6
135. Ujifuku K, Mitsutake N, Takakura S, Matsuse M, Saenko V, Suzuki K, et al. Mir-195, mir-455-3p and mir-10a( *) are implicated in acquired temozolomide resistance in glioblastoma multiforme cells. Cancer Lett (2010) 296(2):241–8. doi: 10.1016/j.canlet.2010.04.013
136. Slaby O, Svoboda M, Michalek J, Vyzula R. Micrornas in colorectal cancer: translation of molecular biology into clinical application. Mol Cancer (2009) 8:102. doi: 10.1186/1476-4598-8-102
137. Haikalis ME, Wessels JM, Leyland NA, Agarwal SK, Foster WG. Microrna expression pattern differs depending on endometriosis lesion type. Biol Reprod (2018) 98(5):623–33. doi: 10.1093/biolre/ioy019
138. Li D, Li X, He H, Zhang Y, He H, Sun C, et al. Mir-10a-5p inhibits chicken granulosa cells proliferation and progesterone(P4) synthesis by targeting mapre1 to suppress cdk2. Theriogenology (2022) 192:97–108. doi: 10.1016/j.theriogenology.2022.08.019
139. Han Y, Wang X, Mao E, Shen B, Huang L. Lncrna flvcr1−As1 drives colorectal cancer progression via modulation of the mir−381/rap2a axis. Mol Med Rep (2021) 23(2):139. doi: 10.3892/mmr.2020.11778
140. Wang X, Meng R, Hu QM. Linc00319-mediated mir-3127 repression enhances bladder cancer progression through upregulation of rap2a. Front Genet (2020) 11:180. doi: 10.3389/fgene.2020.00180
141. Wu F, Lin X, Shan SK, Li F, Xu F, Zhong JY, et al. The suppression of mir-199a-3p by promoter methylation contributes to papillary thyroid carcinoma aggressiveness by targeting rap2a and dnmt3a. Front Cell Dev Biol (2020) 8:594528. doi: 10.3389/fcell.2020.594528
142. Weiner-Gorzel K, Dempsey E, Milewska M, McGoldrick A, Toh V, Walsh A, et al. Overexpression of the microrna mir-433 promotes resistance to paclitaxel through the induction of cellular senescence in ovarian cancer cells. Cancer Med (2015) 4(5):745–58. doi: 10.1002/cam4.409
143. Pan JX, Qu F, Wang FF, Xu J, Mu LS, Ye LY, et al. Aberrant serpine1 DNA methylation is involved in carboplatin induced epithelial-mesenchymal transition in epithelial ovarian cancer. Arch Gynecol Obstet (2017) 296(6):1145–52. doi: 10.1007/s00404-017-4547-x
144. Zhao C, Liu Z. Microrna 617 targeting serpine1 inhibited the progression of oral squamous cell carcinoma. Mol Cell Biol (2021) 41(6):e0056520. doi: 10.1128/MCB.00565-20
145. Giaginis C, Tsoukalas N, Bournakis E, Alexandrou P, Kavantzas N, Patsouris E, et al. Ephrin (Eph) receptor A1, A4, A5 and A7 expression in human non-small cell lung carcinoma: associations with clinicopathological parameters, tumor proliferative capacity and patients' Survival. BMC Clin Pathol (2014) 14(1):8. doi: 10.1186/1472-6890-14-8
146. Kori M, Yalcin Arga K. Potential biomarkers and therapeutic targets in cervical cancer: insights from the meta-analysis of transcriptomics data within network biomedicine perspective. PloS One (2018) 13(7):e0200717. doi: 10.1371/journal.pone.0200717
147. Saintigny P, Peng S, Zhang L, Sen B, Wistuba II, Lippman SM, et al. Global evaluation of eph receptors and ephrins in lung adenocarcinomas identifies epha4 as an inhibitor of cell migration and invasion. Mol Cancer Ther (2012) 11(9):2021–32. doi: 10.1158/1535-7163.MCT-12-0030
148. Yan Y, Luo YC, Wan HY, Wang J, Zhang PP, Liu M, et al. Microrna-10a is involved in the metastatic process by regulating eph tyrosine kinase receptor A4-mediated epithelial-mesenchymal transition and adhesion in hepatoma cells. Hepatology (2013) 57(2):667–77. doi: 10.1002/hep.26071
149. Luo L, Yu ZP, Qin H, Zhu ZX, Liao MH, Liao HT, et al. Exosomal microrna-10a is associated with liver regeneration in rats through downregulation of epha4. Chin Med J (Engl) (2018) 131(4):454–60. doi: 10.4103/0366-6999.225057
150. Goel S, Bergholz JS, Zhao JJ. Targeting cdk4 and cdk6 in cancer. Nat Rev Cancer (2022) 22(6):356–72. doi: 10.1038/s41568-022-00456-3
151. Szoltysek K, Janus P, Zajac G, Stokowy T, Walaszczyk A, Widlak W, et al. Rrad, il4i1, cdkn1a, and serpine1 genes are potentially co-regulated by nf-kappab and P53 transcription factors in cells exposed to high doses of ionizing radiation. BMC Genomics (2018) 19(1):813. doi: 10.1186/s12864-018-5211-y
152. Goel S, DeCristo MJ, Watt AC, BrinJones H, Sceneay J, Li BB, et al. Cdk4/6 inhibition triggers anti-tumour immunity. Nature (2017) 548(7668):471–5. doi: 10.1038/nature23465
153. Horwitz SB, Lothstein L, Manfredi JJ, Mellado W, Parness J, Roy SN, et al. Taxol: mechanisms of action and resistance. Ann N Y Acad Sci (1986) 466:733–44. doi: 10.1111/j.1749-6632.1986.tb38455.x
Keywords: endometriosis, ovarian endometrioma, ovarian clear-cell carcinoma, transcriptomic profiling, miRNA
Citation: Collins KE, Wang X, Klymenko Y, Davis NB, Martinez MC, Zhang C, So K, Buechlein A, Rusch DB, Creighton CJ and Hawkins SM (2023) Transcriptomic analyses of ovarian clear-cell carcinoma with concurrent endometriosis. Front. Endocrinol. 14:1162786. doi: 10.3389/fendo.2023.1162786
Received: 10 February 2023; Accepted: 17 July 2023;
Published: 09 August 2023.
Edited by:
Lusine Aghajanova, Stanford Healthcare, United StatesReviewed by:
Anze Urh, Northwell Health, United StatesMichael Goodheart, The University of Iowa, United States
Copyright © 2023 Collins, Wang, Klymenko, Davis, Martinez, Zhang, So, Buechlein, Rusch, Creighton and Hawkins. This is an open-access article distributed under the terms of the Creative Commons Attribution License (CC BY). The use, distribution or reproduction in other forums is permitted, provided the original author(s) and the copyright owner(s) are credited and that the original publication in this journal is cited, in accordance with accepted academic practice. No use, distribution or reproduction is permitted which does not comply with these terms.
*Correspondence: Shannon M. Hawkins, c2hoYXdraW5ASVUuZWR1