- 1Department of Traditional Chinese Medicine, The First Affiliated Hospital of Zhengzhou University, Zhengzhou, China
- 2Department of Pharmacy, The First Hospital of Changsha, Changsha, China
- 3Department of Pharmacy, The Third People’s Hospital of Qingdao, Qingdao, China
- 4Department of Pharmacy, China-Japan Friendship Hospital, Beijing, China
Type 2 diabetes mellitus (T2DM), a major driver of mortality worldwide, is more likely to develop other cardiometabolic risk factors, ultimately leading to diabetes-related mortality. Although a set of measures including lifestyle intervention and antidiabetic drugs have been proposed to manage T2DM, problems associated with potential side-effects and drug resistance are still unresolved. Pharmacomicrobiomics is an emerging field that investigates the interactions between the gut microbiome and drug response variability or drug toxicity. In recent years, increasing evidence supports that the gut microbiome, as the second genome, can serve as an attractive target for improving drug efficacy and safety by manipulating its composition. In this review, we outline the different composition of gut microbiome in T2DM and highlight how these microbiomes actually play a vital role in its development. Furthermore, we also investigate current state-of-the-art knowledge on pharmacomicrobiomics and microbiome’s role in modulating the response to antidiabetic drugs, as well as provide innovative potential personalized treatments, including approaches for predicting response to treatment and for modulating the microbiome to improve drug efficacy or reduce drug toxicity.
1 Introduction
Type 2 diabetes mellitus (T2DM), a major cause of morbidity globally, is a complex disease with environmental and genetic risk factors that ultimately can lead to serious complications (1). It is characterized by peripheral insulin resistance (IR) and impaired insulin secretion (2), and is projected to affect up to 783 million people by 2045 (3). Individuals with T2DM have an increased risk of developing diabetic complications including microvascular events, kidney failure, stroke and limb amputations (4). Although there are several non-pharmacological and pharmacological treatments available for managing T2DM (5, 6), problems associated with potential side-effects and drug resistance remain unresolved.
Over recent years, the human gut microbiota harboring trillions of microbes and other microorganisms forms a complex ecosystem and plays a vital role in health and disease. For instance, gut microbiota functioned as an important contributor in the pathogenesis of obesity and obesity-related metabolic dysfunctions (7). The balance of pathogenic and beneficial bacteria was also reported to be associated with diabetes and cardiovascular diseases (8, 9). Various studies showed the effect of drug intake and drug-induced metabolites on the gut microbiota (10–12), and the gut microbiota could also contribute to an individual’s response to several drugs in turn (13, 14).
Pharmacomicrobiomics, a new branch, has been proposed to describe the influence of microbiome variations on drug response (15). It was useful for investigating how the effect of drugs could be modulated by the gut microbiota. In addition, pharmacomicrobiomics played a crucial role in the development of personalized medicine in order to improve the drug efficacy and reduce adverse drug reactions (16). Undoubtedly, the microbiota modulation associated with pharmacomicrobiomics has the potential to enable the development of microbiota-targeting approaches.
In the present review, we summarize microbiome variations in T2DM and highlight how these microbiomes actually play a preponderant role in its development. Besides, we also investigate pharmacomicrobiomics and microbiome’s role in modulating the response to antidiabetic drugs, focusing particular attention on innovative potential personalized treatments for T2DM.
2 The role of gut microbiota in T2DM
Gut microbiota, known as the “human second genome”, consists of the 10–100 trillion microorganisms including bacteria, archaea and viruses (17), and has 150 times larger gene sets than humans (18). It was a well-known fact that the gut microbiota played an crucial role in the proper functioning of human organisms (19). Due to the advancements in sequencing technologies, researches on gut microbiome have developed rapidly during the past decade. Accumulating evidence indicated that gut microbiota dysbiosis contributed to the onset and development of T2DM (20–22).
Although the complete bacterial counts were similar between healthy controls and T2DM patients (23), the diversity was significantly declined in T2DM (10, 24–27). Furthermore, the Integrative Human Microbiome Project found that prediabetic individuals had distinguishable microbial patterns at baseline from the healthy controls (28). Both humans and animal models with T2DM showed the compositional changes in microbiota profiles, especially at the phyla and genus levels (29, 30). A previous study showed a decrease in the abundance of butyrate-producing bacteria and an increase in several opportunistic pathogens, including Clostridium symbiosum, Clostridium hathewayi and Escherichia coli in Chinese T2DM patients (29). Likewise, Li et al. revealed a notable decrease of butyrate-producing bacteria such as Bifidobacterium and Akkermansia, as well as a significant increase of Dorea in Chinese T2DM individuals (31). Another study in Europe found an increase abundance of four Lactobacillus species and a reduction in the abundance of five Clostridium species in T2DM patients (23). Analogously, a recent study demonstrated that Lactobacillus was significantly higher, whereas Clostridium coccoides and Clostridium leptum were significantly lower in newly diagnosed T2DM patients (32). Furthermore, patients with refractory T2DM revealed reductions in Akkermansia muciniphila and Fusobacterium, as well as a corresponding enrichment of Bacteroides vulgatus and Veillonella denticariosi (33). Yassour and his colleagues suggested that decreased Akkermansia muciniphila could be used as a biomarker for the early diagnosis of T2DM (34). Notably, Bacteroidetes, Firmicutes and Proteobacteria were reported as the main predominant phyla in T2DM patients (27, 35–37). In newly diagnosed T2DM, the phylum Firmicutes significantly increased, along with the phylum Bacteroidetes significantly decreased (27, 35, 37). Sedighi et al. performed a case-control study and found that Firmicutes increased but Bacteroidetes decreased in T2DM patients (24). Uniformly, a recent study recruited 65 T2DM patients and 35 healthy controls and observed a consistent result (36). Interestingly, these studies also highlighted a significant increase (36, 37) or decrease (27, 35) in Proteobacteria respectively. Therefore, it is necessary to reduce the impact of confounding factors (i.e. dietary habits, lifestyle, disease status) and increase the sample size to further verify these inconsistent results.
In addition, the role of gut microbiota in T2DM was also confirmed in several animal models (38–40). 16S rRNA gene sequencing illuminated that the abundance of several butyrate-producing bacterial genera, such as Dialister, Anaerotruncus and some members of Ruminococcaceae, was reduced in diabetic cats (38). Okazaki et al. established a T2DM zebrafish model and revealed a lower bacterial diversity than the control (39), which indicated functional similarities in T2DM individuals. Wang et al. constructed Zucker diabetic fatty (ZDF) rats that were fed with Purina Lab Diet to induce obesity-related T2DM and found twelve potential biomarkers of microbial flora and 357 differential metabolites in ZDF rats, among which three flora, Phocea, Pseudoflavonifractor and Lactobacillus, contributed to the perturbation of metabolites (40). Besides, microbiome analysis demonstrated that the time-dependent alterations in the fecal microbiome were associated with age and disease progression of T2DM in ZDF rats (41). Of interest, Bifidobacterium, Lactobacillus, Ruminococcus, and Allobaculum were the most abundant genera in 15-week-old rats (41). Leptin receptor-deficient db/db mice were commonly used as T2DM murine models (42). Yu et al. found a significant increase in Verrucomicrobia and a significant decrease in Bacteroidaceae in T2DM murine model (43). They also showed that the fecal microbiota transplantation (FMT) from T2DM murine transplanted into pseudo-germ-free mice induced an increase in body weight and fasting blood glucose. Another study exhibited a loss of diurnal oscillations in several certain bacteria, including Akkermansia, Bifidobacterium, Allobaculum, and Oscillospira in T2DM db/db mice (44). In high-fat diet (HFD)/streptozotocin (STZ)-induced T2DM mice model, genistein could alleviate inflammation and IR by increasing the abundance of Bacteroides and Prevotella and decreasing the levels of Helicobacter and Ruminococcus, indicating that the gut microbiota might be a potential target for the treatment of T2DM (45). Recently, increasing evidence showed that several bacterial taxa, including Akkermansia muciniphila (46) and Bacteroides (47), had consistent trends in T2DM patients and animal models. Collectively, gut microbiota is closely related to the onset and development of T2DM (Table 1), as well as may be an important participant in the pathogenesis of T2DM.
As mentioned above, gut microbiota plays a regulatory role in the development of T2DM. There is growing evidence that microbiota and its metabolites are involved in modulating gut permeability, as well as influence immune and inflammatory responses and metabolic homeostasis in T2DM (Figure 1). Intestinal barrier protects the body from intestinal lipopolysaccharide (LPS), and increased intestinal permeability leads to chronic inflammation and is a characteristic of human T2DM (48). A previous study, in turn, verified that hyperglycemia drived intestinal barrier permeability through altering the integrity of tight and adherence junctions (49). Microbial anti-inflammatory molecule derived from Faecalibacterium prausnitzii could restore the intestinal barrier structure and function via stabilizing the cell permeability and increasing zonula occludens-1 expression in T2DM mouse model (50). Akkermansia muciniphila-derived extracellular vesicles (AmEVs) were reported to decrease in the fecal samples of patients with T2DM, and AmEV administration reduced intestinal permeability by enhancing tight junction function and thus improved glucose homeostasis in HFD-induced diabetic mice (51). Strikingly, numerous clinical and preclinical researches have shown that gut microbial imbalance is closely interconnected to IR. For example, an observational study found that reduced fecal Akkermansia muciniphila abundance increased the severity of IR in Asians with T2DM, particularly those who were lean in weight (52). Similarly, another study reported that butyrate-producing bacteria, such as Fecalibacterium prausnitzii, alleviated IR by inducing glucagon-like peptide-1 receptor (GLP-1) secretion from colonic L cells via fatty acid receptor GPR43 (53). Of note, the levels of fecal and serum LPS were elevated in HFD/STZ-induced T2DM model (54). Subsequent studies have confirmed that when LPS is transported to metabolic tissues, it induces a pro-inflammatory response through the activation of toll-like receptor 4 (TLR4) pathway, ultimately leading to IR (55). Moreover, Amuc_1100, a purified membrane protein from Akkermansia muciniphila, improved the integrity of the intestinal barrier by interacting with toll-like receptor 2 (TLR2), thus alleviating IR in HFD-fed mice (56). It was well known that microbiota and its metabolites stimulated anti-inflammatory cytokines and decreased inflammatory markers, as well as improved glucose metabolism. For instance, Lactobacillus plantarum had potential hypoglycaemic ability and improved glucose metabolism by increasing the levels of interleukin-10 and reducing the levels of malondialdehyde and tumour necrosis factor-α, thus ameliorating IR and systemic inflammation in HFD/STZ-induced T2DM mice (57, 58). Furthermore, Lactobacillus casei and rhamnosus also decreased the levels of the inflammatory markers tumor necrosis factor-α and interleukin-6 in HFD/STZ-induced T2DM rats (59, 60), thereby improving glucose metabolism and attenuating symptoms of T2DM. Although several potential detrimental microbes, such as Fusobacterium nucleatum and Ruminococcus gnavus could increase several inflammatory cytokines in inflammatory diseases (61, 62), its similar role in T2DM remained to be further investigated. Taken together, more studies are needed to deepen our understanding of the role of gut microbiota in T2DM.
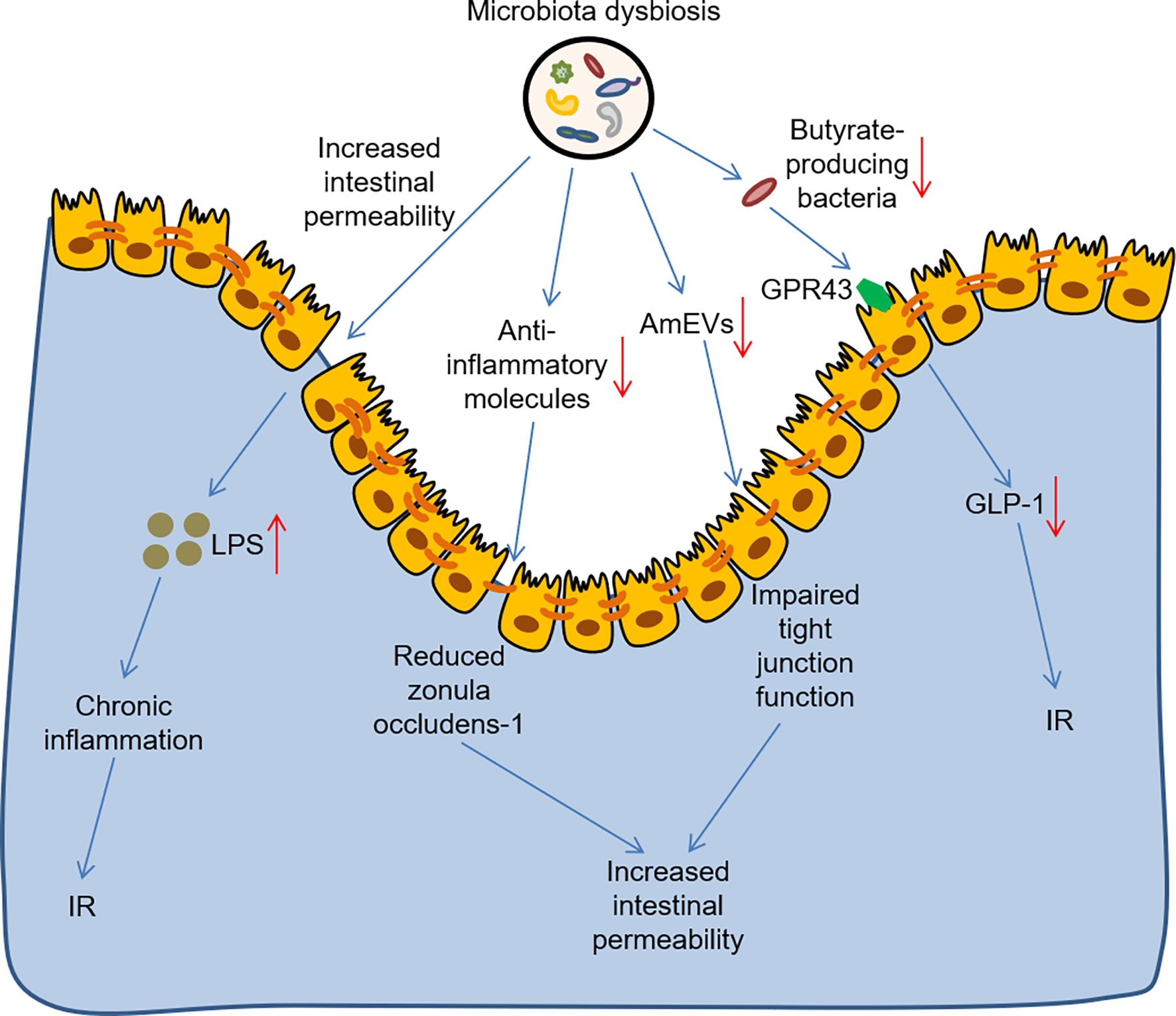
Figure 1 The role of gut microbiota dysbiosis in the development of T2DM. Microbiota dysbiosis increased intestinal barrier permeability and increased the level of LPS, thus leading to chronic inflammation and IR. Microbiota dysbiosis reduced the anti-inflammatory molecule and AmEVs levels, thus impairing the intestinal barrier structure and increasing intestinal barrier permeability. Microbiota dysbiosis also reduced butyrate-producing bacteria, which contributed to IR by inhibiting GLP-1 secretion from colonic L cells via the fatty acid receptor GPR43. LPS, lipopolysaccharide; IR, insulin resistance; AmEVs, Akkermansia muciniphila-derived extracellular vesicles GLP-1, glucagon-like peptide-1 receptor.
3 Pharmacomicrobiomics focuses on T2DM
Given that the preponderant role of gut microbiota in T2DM, there is growing interest in pharmacomicrobiomics and microbiome’s role in T2DM. Pharmacomicrobiomic studies have been proposed to describe the bidirectional effects between the gut microbiome and antidiabetic drugs, including metformin, thiazolidinedione (TZD), α-glucosidase inhibitors (α-GIs), sodium-glucose cotransporter 2 (SGLT2) inhibitors, glucagon-like peptide-1 receptor agonists (GLP-1 RAs), dipeptidyl peptidase-4 (DPP-4) inhibitors and traditional Chinese medicines (TCMs), appropriately investigating the interactions between the host, gut microbiome and drug action (Figure 2).
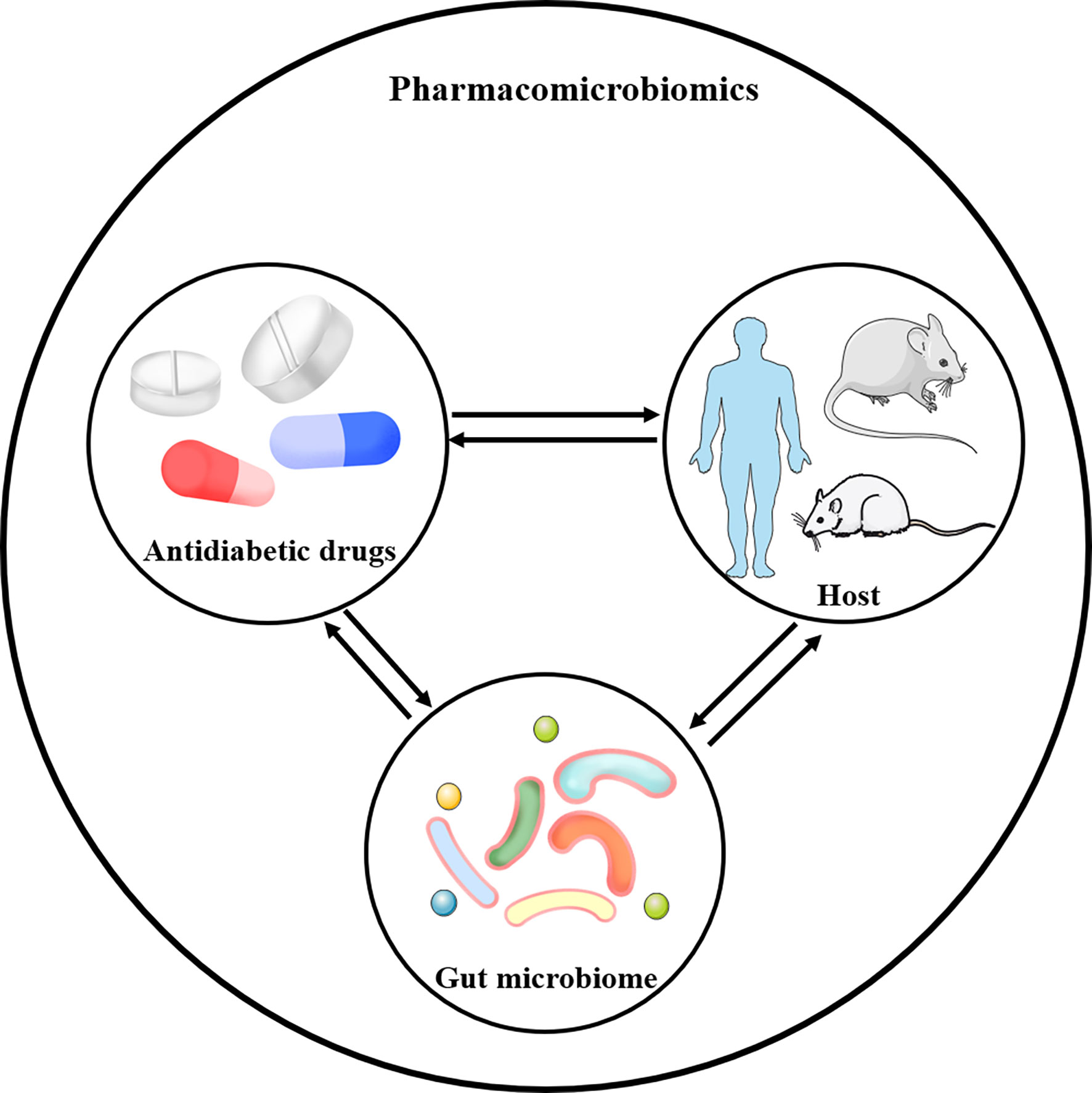
Figure 2 Pharmacomicrobiomics studies drug-microbe-host interactions. Antidiabetic drugs-microbe interactions could result in alterations in microbial composition and changes in the chemical structure of compounds, which could in turn directly or indirectly affect the drug response in host, including T2DM patients, mice and rats.
3.1 Metformin-microbiome-host interactions
Metformin, the most commonly used glucose-lowering drug, can alleviate patients’ hyperglycemia via the suppression of hepatic glucose production and the increase of glucose uptake and utilization in adipocytes and muscle cells (63). A vast body of studies revealed that metformin altered the gut microbiota community in T2DM (11, 64–66). A multicenter, randomized clinical trial suggested that metformin ameliorated hyperglycemia and hyperlipidemia in T2DM patients via increasing beneficial bacteria, such as Blautia and Faecalibacterium (64). Another randomized, placebo-controlled study showed that metformin perturbed the gut microbiome in individuals with treatment-naive T2DM (11). The authors also transplanted the fecal samples from donors (treated with metformin for 4 months) to germ-free mice and observed that glucose tolerance was improved by increasing the production of short-chain fatty acids (SCFAs) or altering plasma bile acid composition, suggesting a direct metabolic benefits of metformin. Similarily, Sun et al. demonstrated that Bacteroides fragilis was decreased and the bile acid glycoursodeoxycholic acid (GUDCA) was increased in newly diagnosed T2DM individuals treated with metformin, and the benefits of metformin were abrogated in HFD-fed mice colonizaed with Bacteroides fragilis, implicating that Bacteroides fragilis–GUDCA–intestinal farnesoid X receptor (FXR) axis mediated the glucose-lowering effect of metformin (67). A recent systematic review disclosed that pre-diabetes and newly diagnosed T2DM patients treated with metformin were correlated with increases in specific taxa associated with metabolic control, such as Enterobacteriales and Akkermansia muciniphila (68). In line with clinical research, studies in animal models further confirmed that metformin increased SCFAs production, reduced circulation lipopolysaccharides and inhibited intestinal proinflammatory signaling activities (65, 69, 70), thus contributing to improving metabolic disoders. Notably, gut microbiota could also mediate the side effects of metformin. Forslund et al. emphasized that a relative increase in abundance of Escherichia could enrich virulence factors and gas metabolism genes (10), which contributed to the gastrointestinal side effects of metformin.
3.2 TZD-microbiome-host interactions
TZD drugs belong to peroxisome proliferative activated receptor (PPARG) agonists and improve insulin sensitivity for T2DM (71). It reduced hepatic glucose production and increased peripheraltion of glucose and lipid metabolism, thus improving glycemic control. Few studies have discussed the interaction between gut microbiome homeostasis and insulin sensitizers and insulin in T2DM (72, 73). Full-length bacterial 16S rRNA sequencing and RNA sequencing analysis presented that rosiglitazone improved insulin sensitivity without altering the composition of gut microbiome but modifying gene expression signatures associated with lipid and carbohydrate metabolism as well as immune regulation in diabetic mice (72). Moreover, insulin improved taurine and hypotaurine metabolism via increasing Fusobacterium and up-regulating the genes involved in triglyceride and arachidonic acid metabolism (73).
3.3 α-GIs-microbiome-host interactions
α-GIs, including acarbose, voglibose and miglitol, are considered to postpone the digestion of carbohydrates in the intestinal tract and reduce postprandial hyperglycemia in noninsulin-dependent T2DM (74). They are commonly used oral glucose-lowering drugs in China and many Asian countries. A randomized clinical study revealed that acarbose increased the abundance of Bifidobacterium, Eubacterium and Lactobacillus, and lowered the abundance of Bacteroides in Japanese patients with T2DM (75). Likewise, in Chinese patients with T2DM, Su et al. found that acarbose treatment increased the content of Bifidobacterium and Enterococcus, as well as decreased some inflammatory cytokines (76). Mechanistically, Gu et al. highlighted that acarbose altered the relative abundance of microbial genes involved in bile acid metabolism and improved metabolic parameters (12). Interestingly, acarbose also increased the relative abundance of Ruminococcus and Bifidobacterium in ZDF rats (77). On the other hand, acarbose was an inhibitor of both human and bacterial α-glucosidases, which might limite the ability of the target microbiome to metabolize complex carbohydrates, thus leading to the resistance of acarbose (78). Additionally, due to the weakened microbial enzyme activities, the metabolism of voglibose was reduced, along with significantly glucose-lowering effects were presented in antibiotic pretreatment mice (79), suggesting that gut microbiota mediated the effect of α-glucosidase inhibitors.
3.4 SGLT2 inhibitors-microbiome-host interactions
SGLT2 is expressed in the renal proximal tubule and accounts for reabsorbing the filtered glucose. SGLT2 inhibitors exert the glucose-lowering effect by blocking glucose reabsorption in the renal proximal tubule and increasing urinary glucose excretion, accompanied with pleiotropic benefits in cardiovascular and renal protection (80, 81). Several studies have explored the alteration of gut microbiota with SGLT2 inhibitor treatment (82–85). After a 3-month intervention, empagliflozin improved cardiovascular disease (CVD) risk factors in patients with T2DM, which might be attributed to the significantly altered gut microbiota, including the elevated levels of SCFA-producing bacteria (Roseburia, Eubacterium, and Faecalibacterium) and a reduction in several harmful bacteria (Escherichia–Shigella, Bilophila, and Hungatella) (82). Whereas, van Bommel et al. reported that 2-week treatment with dapagliflozin and gliclazide did not affect either gut microbiome alpha diversity or composition in T2DM patients treated with metformin (83). This discrepancy might be due to the fact that all the participants had already been treated with metformin, which could overshadow the possible impact of dapagliflozin on the gut microbiome. In T2DM mice, dapagliflozin treatment showed a trend for increased Akkermansia muciniphila and decreased Oscillospira and Firmicutes/Bacteroidetes ratios (84). However, another study demonstrated that dapagliflozin did not increase the abundance of beneficial bacteria (85). Therefore, more rigorous clincial studies with greater sample size are needed to figure out the interactions between SGLT2 inhibitors and gut microbiota.
3.5 GLP-1 RAs-microbiome-host interactions
GLP-1 secreted by enteroendocrine L cells is an incretin hormone and stimulates glucose-dependent insulin secretion. GLP-1 RAs, a new type of hypoglycemic drugs, mimic the effects of endogenous GLP-1, as well as improve glycemic control and cardiovascular outcomes for T2DM patients (86, 87). Accumulating evidence reported that GLP-1 RAs were linked to the changed composition of gut microbiota (88–91). In liraglutide-treated diabetic male rats, several SCFA-producing bacteria, such as Bacteroides, Lachnospiraceae, and probiotic bacteria, Bifidobacterium, were selectively enhanced, which might alleviate systemic inflammation and improve glucose control (88). Besides, germ-free mice colonized with microbiota from liraglutide-treated diabetic mice were shown to improve glucose-induced insulin secretion and regulate the intestinal immune system (91). Also, in T2DM patients, microbial interaction network was altered in patients treated with liraglutide. The distribution of community structure differed between the pre-liraglutide-treatment group (21 species of bacteria were abundant) and post-liraglutide-treatment group (15 species were abundant) (89). Nevertheless, a recent study enrolling 51 T2DM adults with initial therapy of metformin and/or sulphonylureas showed that the diversity and composition of the intestinal microbiota did not change after 12-week liraglutide intervention (92). This inconsistency might be attributed to the initial therapy of metformin and/or sulphonylureas, which could counteract the effect of liraglutide. Recently, Tsai et al. found that gut microbiota contributed to the heterogenicity of GLP-1 RA responses in T2DM patients (90). To sum up, the positive microbial signatures, mainly including Bacteroides and Roseburia, with immunomodulation effects were dominant in GLP-1 RA responders, while the negative microbial signatures, such as Prevotella, Butyricimonas, Mitsuokella and Dialister, with pro-inflammatory properties were dominant in GLP-1 RA non-responders (90). Thus, gut microbiota may be a potential target to improve the GLP-1 resistance.
3.6 DPP-4 inhibitors-microbiome-host interactions
DPP-4 inhibitors improve hyperglycemic conditions by stabilizing GLP-1 and glucose-dependent insulinotropic polypeptides (93). A series of studies considered that DPP-4 inhibitors reshaped the microbial composition and increased fecal SCFAs to improve metabolic homeostasis (94–97). Liao et al. demonstrated that DPP-4 inhibitors promoted a functional shift of the altered microbiome induced by HFD, especially increasing the abundance of Bacteroidetes, which contributed to improving glucose homeostasis (94). Another study revealed that DPP-4 inhibitors displayed significantly decreased Firmicutes/Bacteroidetes ratios, and elevated levels of butyrate-producing Ruminococcus and Dorea in HFD-induced mice (97). Likewise, vildagliptin treatment also reduced the ratio of Fimicutes/Bacteroidetes, and increased butyrate-producing bacteria, including Baceroides and Erysipelotrichaeae, in HFD-induced SD rats (96). Furthermore, vildagliptin significantly reduced DPP-4 activity mainly by decreasing Oscillibacter and increasing Lactobacillus (95), which provided new therapeutic uses of DPP-4 inhibition to tackle gut microbiome dysfunctions in T2DM.
3.7 TCMs-microbiome-host interactions
TCMs, known as botanical medicine or phytomedicine, could significantly improve glucose control by enhancing insulin sensitivity, simulating insulin secretion and protecting β-cells (98). In recent years, increasing evidence confirmed that TCMs improved glucose metabolisms and alleviated T2DM at least partly by modulating gut microbiota (99).
A number of studies in animal models of T2DM have extensively explored the interactions between TCMs and gut microbiota (100–107). Zhou et al. found that ginsenoside Rb1, one of the most valuable herbal medicine, increased the abundance of Parasutterella, and decreased Alistipes, Prevotellaceae, Odoribacter and Anaeroplasma in T2DM mice model, thus attenuating IR and metabolic disorders (100). In T2DM rats model, Baihu Rensheng decoction (BHRS) increased the relative abundance of Lactobacillus, Blautia, and Anaerostipes, as well as decreased the Allobaculum, Candidatus Saccharimonas, and Ruminococcus (101). Mechanically, BHRS was considered to repair gut barrier and inhibit TLR4/NF-κB-mediated inflammatory response. Similarly, Buyang Huanwu decoction (BYHWD), a widely used TCM formula, decreased the Firmicutes/Bacteroidetes ratio and increased the abundance of Lactobacillus and Blautia (102). Another study suggested that Fufang Fanshiliu decoction enriched the abundance of Lactobacillus, Akkermansia, and Proteus, and reduced the abundance of Alistipes, Desulfovibrio, and Helicobacter in T2DM rats model (104). Moreover, Liu-Wei-Di-Huang Pills improved glucose metabolism by promoting the abundance of Lactobacillus, Allobaculum, and Ruminococcus, and increasing SCFAs levels in T2DM rats model (106), which might be related to the SCFAs-GPR43/41-GLP-1 pathway. Shenqi compound (SQC), a TCM formula, has been widely used for T2DM. It showed that SQC exerted a beneficial role by decreasing the Firmicutes/Bacteroidetes ratio and modulating metabolites in different pathways (107). Gegen Qinlian Decoction exerted the glucose-lowering effect by significantly modulating the overall gut microbiota structure and enriching butyrate-producing bacteria, including Faecalibacterium and Roseburia, which subsequently attenuated intestinal inflammation (108). Ge-Gen-Jiao-Tai-Wan formula alleviated symptoms ofT2DM rats by increasing the beneficial phylum Firmicutes and bile-acid-related genus Lactobacillus, thus promoting the production of primary bile acids, and upregulating the PBA-FXR/TGR5-GLP-1 pathway (109). Intriguingly, a current study suggested that the Scrophulariae Radix and Atractylodes sinensis (XC) pair could assist metformin in improving postprandial hyperglycemia by inhibiting the increase of Bacteroides in T2DM rats model (105), which could effectively apply to clinical practice in treating T2DM. In addition to the animal studies, a clinical trial in newly diagnosed T2DM patients also underlined that the hypoglycemic effect of berberine was related to the inhibition of DCA biotransformation by Ruminococcus bromii (110). Collectively, these findings address the effect of antidiabetic drugs on gut microbiota (Figure 3) and emphasize the host-microbe-drug interactions, providing promising microbiome-targeting approaches to treat T2DM.
4 Innovative therapeutics and translational implications of pharmacomicrobiomic studies in T2DM
With the host-microbe-drug interactions in mind, the innovative therapeutics and translational applicability of pharmacomicrobiomics are highly relevant to our understanding of drug efficacy and adverse reactions in T2DM (Figure 4). Given that the magnitude of response to antidiabetic durgs is known to have a unpredictable and high interindividual variability, personalized treatments based on novel technologies and features of the gut microbiome can help to guide a more rational use of these treatments.
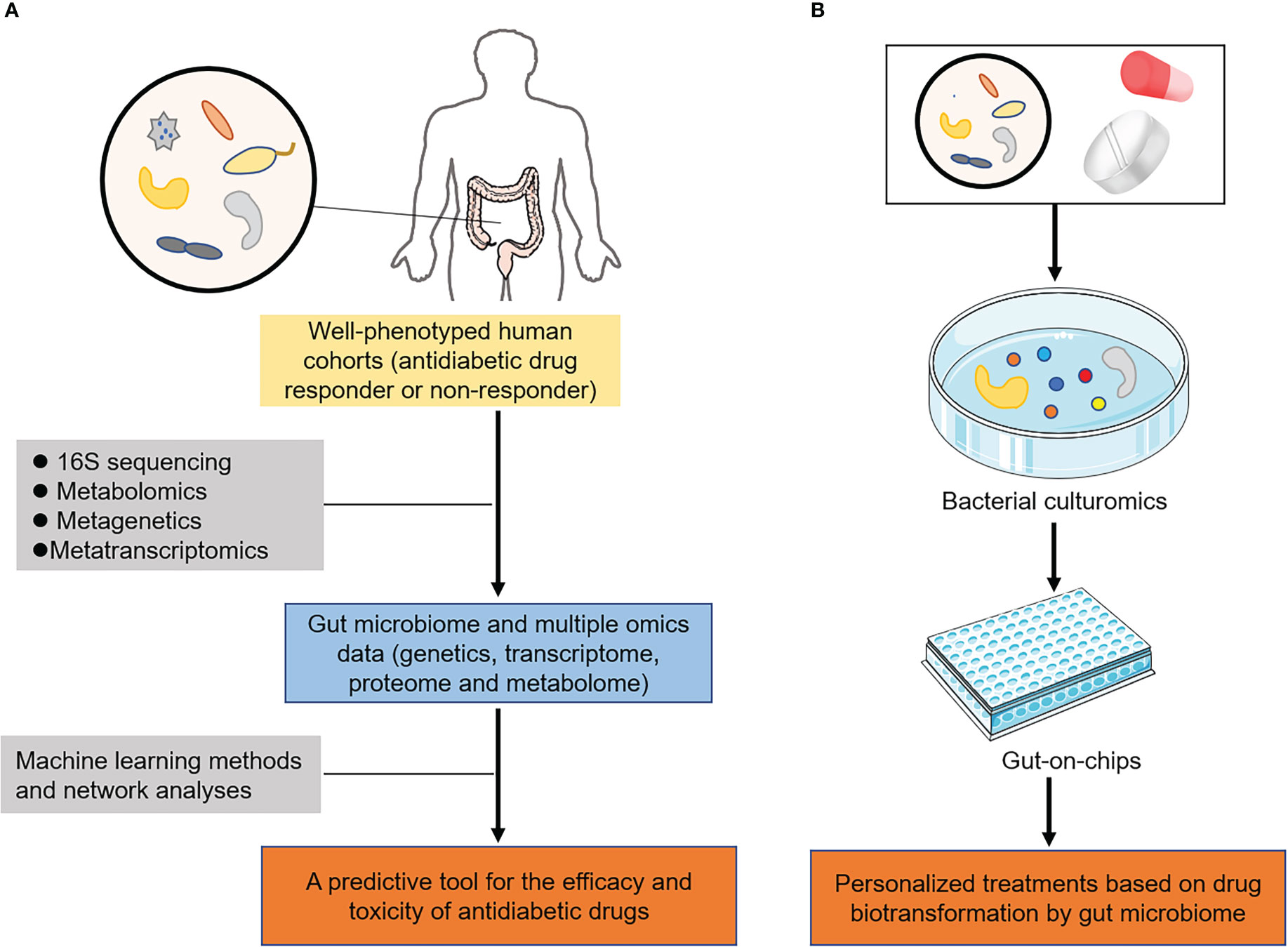
Figure 4 Innovative therapeutics and translational implications of pharmacomicrobiomic studies in T2DM. (A) In well-phenotyped patient populations, microbial features and multiple omics could be integrated via machine learning and network analyses to predict the efficacy and toxicity of antidiabetic drugs. (B) Novel technologies, including organs-on-chips and bacterial culturomics assessed their biotransformation by gut microbiome, providing new insights into personalized medicine in T2DM.
4.1 Developing predictive tools via machine learning and network analyses
With the completion of the human genome and the human microbiome projects (111), a number of large biobanks including gut microbiome and multiple omics data (information on genetics, transcriptome, proteome and metabolome) had been established, such as UK biobank and TwinsUK cohort (112, 113). These biobanks utilized clinical studies, involving well-characterized human cohorts with extensive clinical and demographic details, exploring the host-microbe-drug interactions. In parallel with the existing data, there was continuous need for digging deeper into the unknown filed of drug-microbiome interactions. The accumulated data in literature calls for the construction of predictive tools or models that consider all such parameters to provide accurate predictions (114, 115). Machine learning methods and network analyses, including decision-tree algorithms and random forest, could then be applied to create a predictive tool for the efficacy and toxicity of antidiabetic drugs in T2DM (Figure 4A). For instance, the T2DM prediction model based on the characteristics of the salivary microbiota (microbiome data) was established by random forest in elderly patients with T2DM (116). Another study showed that machine learning tools with gut microbiome profiling exhibited the highest overall predictive power for improving early prediction of T2DM (117). These findings not only had the ability to rapidly inform clinical practice but also elucidated hypotheses regarding the mechanisms in which microbial transformations of drugs changed their pharmacokinetic properties.
4.2 Novel technologies for developing personalized treatments
Given the interplay between the host, gut microbiome and drug metabolism, there is increasing awareness that we should take microbiome profile based on novel technologies into account when considering personalized medicine.
Considering that microbiome profiling of human samples provided evidence for microorganism-mediated drug metabolism, further experimental studies are required to identify the specific microbiome responsible for drug biotransformation. Experimental manipulations of gut microbiota incorporated the use of humanized gnotobiotic mice models to further investigate the specific role of the microbiota in modulating drug pharmacokinetics (118). Humanized gnotobiotic mice are typically either germ-free animals or those colonized with defined microbiota and achieved by transplanting human faecal microbiota into germ-free mice (119). As discussed, these models have already proven successful for the treatment of several diseases, including T2DM (120). In recent years, organs-on-chips and bacterial culturomics as emerging technologies also have been developed (121, 122), making functional validation of gut microbiome finally possible. Antidiabetic drugs of interest can be incubated via these technologies to assess their biotransformation by gut microbiome, enabling the development of personalized medicine in T2DM (Figure 4B).
5 Concluding remarks and future perspectives
There is a mountain of evidence linking gut microbiota to T2DM and its hypoglycemic therapy. In recent years, a growing body of research now focuses on the bidirectional effects between the gut microbiome and antidiabetic drugs (123, 124). In this review, we summarize the microbe-drug-host interactions and provide a novel perspective towards possible personalized treatment for T2DM.
With the advancement of the studies on the pharmacomicrobiomics (interactions between drugs, microbial communities and host) (125–127), manipulation of microbiota can be a promising target to improve therapeutic outcomes and alleviate adverse drug effects in T2DM. For instance, prebiotics could modulate intestinal microbiota and increase the relative abundance of beneficial bacteria including SCFAs (128, 129), and the combination of hypoglycemic drugs and certain prebiotics could enhance the glucose-lowering effects (130). In addition, FMT, a process of transferring stool from a healthy donor or antidiabetes treatment subjects to mice, displayed a significant improvement in microbial composition and metabolic homeostasis (131). A prospective study revealed that FMT could bring benefits for the management of T2DM via modulating levels of certain microbiome such as Rikenellaceae and Anaerotruncus (132).
Given the great diversity of microbial signatures and the complex drug-microbe-host interactions, a systems-based approach including the integration of multi-omics data with microbiome data and the utilization of bacterial culturomics are required to understand the underlying mechanisms, thus exploring the new therapeutic interventions and potential personalized strategies.
Author contributions
LJ and SH contributed towards the concept and manuscript writing. BS, YS and CZ revised and supervised overall project. All authors read and approved the final version of manuscript. All authors contributed to the article and approved the submitted version.
Funding
This work was financially supported by the National Natural Science Foundation of China (82204701) and the Joint Co-construction Project of Henan Medical Science and Technology Research Plan (LHGJ20220421).
Conflict of interest
The authors declare that the research was conducted in the absence of any commercial or financial relationships that could be construed as a potential conflict of interest.
Publisher’s note
All claims expressed in this article are solely those of the authors and do not necessarily represent those of their affiliated organizations, or those of the publisher, the editors and the reviewers. Any product that may be evaluated in this article, or claim that may be made by its manufacturer, is not guaranteed or endorsed by the publisher.
References
1. American Diabetes Association. Introduction: Standards of medical care in diabetes-2018. Diabetes Care (2018) 41(Suppl 1):S1–s2. doi: 10.2337/dc18-Sint01
2. Roden M, Shulman GI. The integrative biology of type 2 diabetes. Nature. (2019) 576(7785):51–60. doi: 10.1038/s41586-019-1797-8
3. International Diabetes Federation. IDF diabetes atlas. 10th edn. Brussels, Belgium (2021). Available at: https://wwwdiabetesatlasorg. 2021
4. American Diabetes Association. 11. microvascular complications and foot care: Standards of medical care in diabetes-2020. Diabetes Care (2020) 43(Suppl 1):S135–s51. doi: 10.2337/dc20-S011
5. Peter PR, Lupsa BC. Personalized management of type 2 diabetes. Curr Diabetes Rep (2019) 19(11):115. doi: 10.1007/s11892-019-1244-0
6. Leite R, Banzato LR, Galendi JSC, Mendes AL, Bolfi F, Veroniki AA, et al. Effectiveness of non-pharmacological strategies in the management of type 2 diabetes in primary care: a protocol for a systematic review and network meta-analysis. BMJ Open (2020) 10(1):e034481. doi: 10.1136/bmjopen-2019-034481
7. Bouter KE, van Raalte DH, Groen AK, Nieuwdorp M. Role of the gut microbiome in the pathogenesis of obesity and obesity-related metabolic dysfunction. Gastroenterology. (2017) 152(7):1671–8. doi: 10.1053/j.gastro.2016.12.048
8. Vamanu E, Pelinescu D, Sarbu I. Comparative fingerprinting of the human microbiota in diabetes and cardiovascular disease. J Med Food (2016) 19(12):1188–95. doi: 10.1089/jmf.2016.0085
9. Umirah F, Neoh CF, Ramasamy K, Lim SM. Differential gut microbiota composition between type 2 diabetes mellitus patients and healthy controls: A systematic review. Diabetes Res Clin Pract (2021) 173:108689. doi: 10.1016/j.diabres.2021.108689
10. Forslund K, Hildebrand F, Nielsen T, Falony G, Le Chatelier E, Sunagawa S, et al. Disentangling type 2 diabetes and metformin treatment signatures in the human gut microbiota. Nature. (2015) 528(7581):262–6. doi: 10.1038/nature15766
11. Wu H, Esteve E, Tremaroli V, Khan MT, Caesar R, Mannerås-Holm L, et al. Metformin alters the gut microbiome of individuals with treatment-naive type 2 diabetes, contributing to the therapeutic effects of the drug. Nat Med (2017) 23(7):850–8. doi: 10.1038/nm.4345
12. Gu Y, Wang X, Li J, Zhang Y, Zhong H, Liu R, et al. Analyses of gut microbiota and plasma bile acids enable stratification of patients for antidiabetic treatment. Nat Commun (2017) 8(1):1785. doi: 10.1038/s41467-017-01682-2
13. Routy B, Le Chatelier E, Derosa L, Duong CPM, Alou MT, Daillère R, et al. Gut microbiome influences efficacy of PD-1-based immunotherapy against epithelial tumors. Science. (2018) 359(6371):91–7. doi: 10.1126/science.aan3706
14. Gopalakrishnan V, Spencer CN, Nezi L, Reuben A, Andrews MC, Karpinets TV, et al. Gut microbiome modulates response to anti-PD-1 immunotherapy in melanoma patients. Science (2018) 359(6371):97–103. doi: 10.1126/science.aan4236
15. Saad R, Rizkallah MR, Aziz RK. Gut pharmacomicrobiomics: the tip of an iceberg of complex interactions between drugs and gut-associated microbes. Gut Pathog (2012) 4(1):16. doi: 10.1186/1757-4749-4-16
16. Hassan R, Allali I, Agamah FE, Elsheikh SSM, Thomford NE, Dandara C, et al. Drug response in association with pharmacogenomics and pharmacomicrobiomics: towards a better personalized medicine. Brief Bioinform (2021) 22(4):bbaa292. doi: 10.1093/bib/bbaa292
17. Allin KH, Nielsen T, Pedersen O. Mechanisms in endocrinology: Gut microbiota in patients with type 2 diabetes mellitus. Eur J Endocrinol (2015) 172(4):R167–77. doi: 10.1530/EJE-14-0874
18. Qin J, Li R, Raes J, Arumugam M, Burgdorf KS, Manichanh C, et al. A human gut microbial gene catalogue established by metagenomic sequencing. Nature. (2010) 464(7285):59–65. doi: 10.1038/nature08821
19. Vrancken G, Gregory AC, Huys GRB, Faust K, Raes J. Synthetic ecology of the human gut microbiota. Nat Rev Microbiol (2019) 17(12):754–63. doi: 10.1038/s41579-019-0264-8
20. Gurung M, Li Z, You H, Rodrigues R, Jump DB, Morgun A, et al. Role of gut microbiota in type 2 diabetes pathophysiology. EBioMedicine. (2020) 51:102590. doi: 10.1016/j.ebiom.2019.11.051
21. Hou K, Zhang S, Wu Z, Zhu D, Chen F, Lei ZN, et al. Reconstruction of intestinal microecology of type 2 diabetes by fecal microbiota transplantation: Why and how. Bosn J Basic Med Sci (2022) 22(3):315–25. doi: 10.17305/bjbms.2021.6323
22. Sharma S, Tripathi P. Gut microbiome and type 2 diabetes: where we are and where to go? J Nutr Biochem (2019) 63:101–8. doi: 10.1016/j.jnutbio.2018.10.003
23. Karlsson FH, Tremaroli V, Nookaew I, Bergström G, Behre CJ, Fagerberg B, et al. Gut metagenome in European women with normal, impaired and diabetic glucose control. Nature. (2013) 498(7452):99–103. doi: 10.1038/nature12198
24. Sedighi M, Razavi S, Navab-Moghadam F, Khamseh ME, Alaei-Shahmiri F, Mehrtash A, et al. Comparison of gut microbiota in adult patients with type 2 diabetes and healthy individuals. Microb Pathog (2017) 111:362–9. doi: 10.1016/j.micpath.2017.08.038
25. Que Y, Cao M, He J, Zhang Q, Chen Q, Yan C, et al. Gut bacterial characteristics of patients with type 2 diabetes mellitus and the application potential. Front Immunol (2021) 12:722206. doi: 10.3389/fimmu.2021.722206
26. Chávez-Carbajal A, Pizano-Zárate ML, Hernández-Quiroz F, Ortiz-Luna GF, Morales-Hernández RM, De Sales-Millán A, et al. Characterization of the gut microbiota of individuals at different T2D stages reveals a complex relationship with the host. Microorganisms (2020) 8(1):94. doi: 10.3390/microorganisms8010094
27. Bhute SS, Suryavanshi MV, Joshi SM, Yajnik CS, Shouche YS, Ghaskadbi SS. Gut microbial diversity assessment of Indian type-2-Diabetics reveals alterations in eubacteria, archaea, and eukaryotes. Front Microbiol (2017) 8:214. doi: 10.3389/fmicb.2017.00214
28. Integrative HMP (iHMP) Research Network Consortium. The integrative human microbiome project. Nature (2019) 569(7758):641–8. doi: 10.1038/s41586-019-1238-8
29. Qin J, Li Y, Cai Z, Li S, Zhu J, Zhang F, et al. A metagenome-wide association study of gut microbiota in type 2 diabetes. Nature. (2012) 490(7418):55–60. doi: 10.1038/nature11450
30. Larsen N, Vogensen FK, van den Berg FW, Nielsen DS, Andreasen AS, Pedersen BK, et al. Gut microbiota in human adults with type 2 diabetes differs from non-diabetic adults. PloS One (2010) 5(2):e9085. doi: 10.1371/journal.pone.0009085
31. Li Q, Chang Y, Zhang K, Chen H, Tao S, Zhang Z. Implication of the gut microbiome composition of type 2 diabetic patients from northern China. Sci Rep (2020) 10(1):5450. doi: 10.1038/s41598-020-62224-3
32. Chen PC, Chien YW, Yang SC. The alteration of gut microbiota in newly diagnosed type 2 diabetic patients. Nutrition (2019) 63-64:51–6. doi: 10.1016/j.nut.2018.11.019
33. Shih CT, Yeh YT, Lin CC, Yang LY, Chiang CP. Akkermansia muciniphila is negatively correlated with hemoglobin A1c in refractory diabetes. Microorganisms. (2020) 8(9):1360. doi: 10.3390/microorganisms8091360
34. Yassour M, Lim MY, Yun HS, Tickle TL, Sung J, Song YM, et al. Sub-Clinical detection of gut microbial biomarkers of obesity and type 2 diabetes. Genome Med (2016) 8(1):17. doi: 10.1186/s13073-016-0271-6
35. Nuli R, Cai J, Kadeer A, Zhang Y, Mohemaiti P. Integrative analysis toward different glucose tolerance-related gut microbiota and diet. Front Endocrinol (2019) 10:295. doi: 10.3389/fendo.2019.00295
36. Zhao L, Lou H, Peng Y, Chen S, Zhang Y, Li X. Comprehensive relationships between gut microbiome and faecal metabolome in individuals with type 2 diabetes and its complications. Endocrine. (2019) 66(3):526–37. doi: 10.1007/s12020-019-02103-8
37. Gaike AH, Paul D, Bhute S, Dhotre DP, Pande P, Upadhyaya S, et al. The gut microbial diversity of newly diagnosed diabetics but not of prediabetics is significantly different from that of healthy nondiabetics. mSystems (2020) 5(2):e00578-19. doi: 10.1128/mSystems.00578-19
38. Kieler IN, Osto M, Hugentobler L, Puetz L, Gilbert MTP, Hansen T, et al. Diabetic cats have decreased gut microbial diversity and a lack of butyrate producing bacteria. Sci Rep (2019) 9(1):4822. doi: 10.1038/s41598-019-41195-0
39. Okazaki F, Zang L, Nakayama H, Chen Z, Gao ZJ, Chiba H, et al. Microbiome alteration in type 2 diabetes mellitus model of zebrafish. Sci Rep (2019) 9(1):867. doi: 10.1038/s41598-018-37242-x
40. Wang Y, Ouyang M, Gao X, Wang S, Fu C, Zeng J, et al. Phocea, pseudoflavonifractor and lactobacillus intestinalis: Three potential biomarkers of gut microbiota that affect progression and complications of obesity-induced type 2 diabetes mellitus. Diabetes Metab Syndr Obes (2020) 13:835–50. doi: 10.2147/DMSO.S240728
41. Zhou W, Xu H, Zhan L, Lu X, Zhang L. Dynamic development of fecal microbiome during the progression of diabetes mellitus in zucker diabetic fatty rats. Front Microbiol (2019) 10:232. doi: 10.3389/fmicb.2019.00232
42. Suriano F, Vieira-Silva S, Falony G, Roumain M, Paquot A, Pelicaen R, et al. Novel insights into the genetically obese (ob/ob) and diabetic (db/db) mice: two sides of the same coin. Microbiome. (2021) 9(1):147. doi: 10.1186/s40168-021-01097-8
43. Yu F, Han W, Zhan G, Li S, Jiang X, Wang L, et al. Abnormal gut microbiota composition contributes to the development of type 2 diabetes mellitus in db/db mice. Aging (2019) 11(22):10454–67. doi: 10.18632/aging.102469
44. Beli E, Prabakaran S, Krishnan P, Evans-Molina C, Grant MB. Loss of diurnal oscillatory rhythms in gut microbiota correlates with changes in circulating metabolites in type 2 diabetic db/db mice. Nutrients. (2019) 11(10):2310. doi: 10.3390/nu11102310
45. Yang R, Jia Q, Mehmood S, Ma S, Liu X. Genistein ameliorates inflammation and insulin resistance through mediation of gut microbiota composition in type 2 diabetic mice. Eur J Nutr (2021) 60(4):2155–68. doi: 10.1007/s00394-020-02403-0
46. Zhang J, Ni Y, Qian L, Fang Q, Zheng T, Zhang M, et al. Decreased abundance of akkermansia muciniphila leads to the impairment of insulin secretion and glucose homeostasis in lean type 2 diabetes. Adv Sci (2021) 8(16):e2100536. doi: 10.1002/advs.202100536
47. Zhang Y, Wu T, Li W, Zhao Y, Long H, Liu R, et al. Lactobacillus casei LC89 exerts antidiabetic effects through regulating hepatic glucagon response and gut microbiota in type 2 diabetic mice. Food Funct (2021) 12(18):8288–99. doi: 10.1039/D1FO00882J
48. Scheithauer TPM, Rampanelli E, Nieuwdorp M, Vallance BA, Verchere CB, van Raalte DH, et al. Gut microbiota as a trigger for metabolic inflammation in obesity and type 2 diabetes. Front Immunol (2020) 11:571731. doi: 10.3389/fimmu.2020.571731
49. Thaiss CA, Levy M, Grosheva I, Zheng D, Soffer E, Blacher E, et al. Hyperglycemia drives intestinal barrier dysfunction and risk for enteric infection. Science. (2018) 359(6382):1376–83. doi: 10.1126/science.aar3318
50. Xu J, Liang R, Zhang W, Tian K, Li J, Chen X, et al. Faecalibacterium prausnitzii-derived microbial anti-inflammatory molecule regulates intestinal integrity in diabetes mellitus mice via modulating tight junction protein expression. J Diabetes. (2020) 12(3):224–36. doi: 10.1111/1753-0407.12986
51. Chelakkot C, Choi Y, Kim DK, Park HT, Ghim J, Kwon Y, et al. Akkermansia muciniphila-derived extracellular vesicles influence gut permeability through the regulation of tight junctions. Exp Mol Med (2018) 50(2):e450. doi: 10.1038/emm.2017.282
52. Pai CS, Wang CY, Hung WW, Hung WC, Tsai HJ, Chang CC, et al. Interrelationship of gut microbiota, obesity, body composition and insulin resistance in asians with type 2 diabetes mellitus. J Pers Med (2022) 12(4):617. doi: 10.3390/jpm12040617
53. Lee CJ, Sears CL, Maruthur N. Gut microbiome and its role in obesity and insulin resistance. Ann N Y Acad Sci (2020) 1461(1):37–52. doi: 10.1111/nyas.14107
54. Deng L, Yang Y, Xu G. Empagliflozin ameliorates type 2 diabetes mellitus-related diabetic nephropathy via altering the gut microbiota. Biochim Biophys Acta Mol Cell Biol Lipids. (2022) 1867(12):159234. doi: 10.1016/j.bbalip.2022.159234
55. Wang Y, Liu H, Zheng M, Yang Y, Ren H, Kong Y, et al. Berberine slows the progression of prediabetes to diabetes in zucker diabetic fatty rats by enhancing intestinal secretion of glucagon-like peptide-2 and improving the gut microbiota. Front Endocrinol (2021) 12:609134. doi: 10.3389/fendo.2021.609134
56. Plovier H, Everard A, Druart C, Depommier C, Van Hul M, Geurts L, et al. A purified membrane protein from akkermansia muciniphila or the pasteurized bacterium improves metabolism in obese and diabetic mice. Nat Med (2017) 23(1):107–13. doi: 10.1038/nm.4236
57. Li X, Wang N, Yin B, Fang D, Jiang T, Fang S, et al. Effects of lactobacillus plantarum CCFM0236 on hyperglycaemia and insulin resistance in high-fat and streptozotocin-induced type 2 diabetic mice. J Appl Microbiol (2016) 121(6):1727–36. doi: 10.1111/jam.13276
58. Chen P, Zhang Q, Dang H, Liu X, Tian F, Zhao J, et al. Antidiabetic effect of lactobacillus casei CCFM0412 on mice with type 2 diabetes induced by a high-fat diet and streptozotocin. Nutrition. (2014) 30(9):1061–8. doi: 10.1016/j.nut.2014.03.022
59. Wang G, Li X, Zhao J, Zhang H, Chen W. Lactobacillus casei CCFM419 attenuates type 2 diabetes via a gut microbiota dependent mechanism. Food Funct (2017) 8(9):3155–64. doi: 10.1039/C7FO00593H
60. Singh S, Sharma RK, Malhotra S, Pothuraju R, Shandilya UK. Lactobacillus rhamnosus NCDC17 ameliorates type-2 diabetes by improving gut function, oxidative stress and inflammation in high-fat-diet fed and streptozotocintreated rats. Benef Microbes (2017) 8(2):243–55. doi: 10.3920/BM2016.0090
61. Yang Y, Weng W, Peng J, Hong L, Yang L, Toiyama Y, et al. Fusobacterium nucleatum increases proliferation of colorectal cancer cells and tumor development in mice by activating toll-like receptor 4 signaling to nuclear factor-κB, and up-regulating expression of MicroRNA-21. Gastroenterology. (2017) 152(4):851–66.e24. doi: 10.1053/j.gastro.2016.11.018
62. Hall AB, Yassour M, Sauk J, Garner A, Jiang X, Arthur T, et al. A novel ruminococcus gnavus clade enriched in inflammatory bowel disease patients. Genome Med (2017) 9(1):103. doi: 10.1186/s13073-017-0490-5
63. He L. Metformin and systemic metabolism. Trends Pharmacol Sci (2020) 41(11):868–81. doi: 10.1016/j.tips.2020.09.001
64. Tong X, Xu J, Lian F, Yu X, Zhao Y, Xu L, et al. Structural alteration of gut microbiota during the amelioration of human type 2 diabetes with hyperlipidemia by metformin and a traditional Chinese herbal formula: a multicenter, randomized, open label clinical trial. mBio (2018) 9(3):e02392-17. doi: 10.1128/mBio.02392-17
65. Ahmadi S, Razazan A, Nagpal R, Jain S, Wang B, Mishra SP, et al. Metformin reduces aging-related leaky gut and improves cognitive function by beneficially modulating gut Microbiome/Goblet Cell/Mucin axis. J Gerontol A Biol Sci Med Sci (2020) 75(7):e9–e21. doi: 10.1093/gerona/glaa056
66. Nakajima H, Takewaki F, Hashimoto Y, Kajiyama S, Majima S, Okada H, et al. The effects of metformin on the gut microbiota of patients with type 2 diabetes: A two-center, quasi-experimental study. Life (2020) 10(9):195. doi: 10.3390/life10090195
67. Sun L, Xie C, Wang G, Wu Y, Wu Q, Wang X, et al. Gut microbiota and intestinal FXR mediate the clinical benefits of metformin. Nat Med (2018) 24(12):1919–29. doi: 10.1038/s41591-018-0222-4
68. Cao TTB, Wu KC, Hsu JL, Chang CS, Chou C, Lin CY, et al. Effects of non-insulin anti-hyperglycemic agents on gut microbiota: A systematic review on human and animal studies. Front Endocrinol (2020) 11:573891. doi: 10.3389/fendo.2020.573891
69. Zhang W, Xu JH, Yu T, Chen QK. Effects of berberine and metformin on intestinal inflammation and gut microbiome composition in db/db mice. BioMed Pharmacother. (2019) 118:109131. doi: 10.1016/j.biopha.2019.109131
70. Liu Z, Liao W, Zhang Z, Sun R, Luo Y, Chen Q, et al. Metformin affects gut microbiota composition and diversity associated with amelioration of dextran sulfate sodium-induced colitis in mice. Front Pharmacol (2021) 12:640347. doi: 10.3389/fphar.2021.640347
71. Ahmadian M, Suh JM, Hah N, Liddle C, Atkins AR, Downes M, et al. PPARγ signaling and metabolism: the good, the bad and the future. Nat Med (2013) 19(5):557–66. doi: 10.1038/nm.3159
72. Madsen MSA, Grønlund RV, Eid J, Christensen-Dalsgaard M, Sommer M, Rigbolt K, et al. Characterization of local gut microbiome and intestinal transcriptome responses to rosiglitazone treatment in diabetic db/db mice. BioMed Pharmacother. (2021) 133:110966. doi: 10.1016/j.biopha.2020.110966
73. Zhang F, Wang M, Yang J, Xu Q, Liang C, Chen B, et al. Response of gut microbiota in type 2 diabetes to hypoglycemic agents. Endocrine. (2019) 66(3):485–93. doi: 10.1007/s12020-019-02041-5
74. Dirir AM, Daou M, Yousef AF, Yousef LF. A review of alpha-glucosidase inhibitors from plants as potential candidates for the treatment of type-2 diabetes. Phytochem Rev (2022) 21(4):1049–79. doi: 10.1007/s11101-021-09773-1
75. Takewaki F, Nakajima H, Takewaki D, Hashimoto Y, Majima S, Okada H, et al. Habitual dietary intake affects the altered pattern of gut microbiome by acarbose in patients with type 2 diabetes. Nutrients (2021) 13(6):2107. doi: 10.3390/nu13062107
76. Su B, Liu H, Li J, Sunli Y, Liu B, Liu D, et al. Acarbose treatment affects the serum levels of inflammatory cytokines and the gut content of bifidobacteria in Chinese patients with type 2 diabetes mellitus. J Diabetes. (2015) 7(5):729–39. doi: 10.1111/1753-0407.12232
77. Zhang M, Feng R, Yang M, Qian C, Wang Z, Liu W, et al. Effects of metformin, acarbose, and sitagliptin monotherapy on gut microbiota in zucker diabetic fatty rats. BMJ Open Diabetes Res Care (2019) 7(1):e000717. doi: 10.1136/bmjdrc-2019-000717
78. Balaich J, Estrella M, Wu G, Jeffrey PD, Biswas A, Zhao L, et al. The human microbiome encodes resistance to the antidiabetic drug acarbose. Nature. (2021) 600(7887):110–5. doi: 10.1038/s41586-021-04091-0
79. Nepal MR, Kang MJ, Kim GH, Cha DH, Kim JH, Jeong TC. Role of intestinal microbiota in metabolism of voglibose In vitro and in vivo. Diabetes Metab J (2020) 44(6):908–18. doi: 10.4093/dmj.2019.0147
80. Wiviott SD, Raz I, Bonaca MP, Mosenzon O, Kato ET, Cahn A, et al. Dapagliflozin and cardiovascular outcomes in type 2 diabetes. N Engl J Med (2019) 380(4):347–57. doi: 10.1056/NEJMoa1812389
81. Perkovic V, Jardine MJ, Neal B, Bompoint S, Heerspink HJL, Charytan DM, et al. Canagliflozin and renal outcomes in type 2 diabetes and nephropathy. N Engl J Med (2019) 380(24):2295–306. doi: 10.1056/NEJMoa1811744
82. Deng X, Zhang C, Wang P, Wei W, Shi X, Wang P, et al. Cardiovascular benefits of empagliflozin are associated with gut microbiota and plasma metabolites in type 2 diabetes. J Clin Endocrinol Metab (2022) 107(7):1888–96. doi: 10.1210/clinem/dgac210
83. van Bommel EJM, Herrema H, Davids M, Kramer MHH, Nieuwdorp M, van Raalte DH. Effects of 12-week treatment with dapagliflozin and gliclazide on faecal microbiome: Results of a double-blind randomized trial in patients with type 2 diabetes. Diabetes Metab (2020) 46(2):164–8. doi: 10.1016/j.diabet.2019.11.005
84. Lee DM, Battson ML, Jarrell DK, Hou S, Ecton KE, Weir TL, et al. SGLT2 inhibition via dapagliflozin improves generalized vascular dysfunction and alters the gut microbiota in type 2 diabetic mice. Cardiovasc Diabetol (2018) 17(1):62. doi: 10.1186/s12933-018-0708-x
85. Yang M, Shi FH, Liu W, Zhang MC, Feng RL, Qian C, et al. Dapagliflozin modulates the fecal microbiota in a type 2 diabetic rat model. Front Endocrinol (2020) 11:635. doi: 10.3389/fendo.2020.00635
86. Bethel MA, Patel RA, Merrill P, Lokhnygina Y, Buse JB, Mentz RJ, et al. Cardiovascular outcomes with glucagon-like peptide-1 receptor agonists in patients with type 2 diabetes: a meta-analysis. Lancet Diabetes Endocrinol (2018) 6(2):105–13. doi: 10.1016/S2213-8587(17)30412-6
87. Giugliano D, Scappaticcio L, Longo M, Caruso P, Maiorino MI, Bellastella G, et al. GLP-1 receptor agonists and cardiorenal outcomes in type 2 diabetes: an updated meta-analysis of eight CVOTs. Cardiovasc Diabetol (2021) 20(1):189. doi: 10.1186/s12933-021-01366-8
88. Zhang Q, Xiao X, Zheng J, Li M, Yu M, Ping F, et al. Featured article: Structure moderation of gut microbiota in liraglutide-treated diabetic male rats. Exp Biol Med (2018) 243(1):34–44. doi: 10.1177/1535370217743765
89. Shang J, Liu F, Zhang B, Dong K, Lu M, Jiang R, et al. Liraglutide-induced structural modulation of the gut microbiota in patients with type 2 diabetes mellitus. PeerJ. (2021) 9:e11128. doi: 10.7717/peerj.11128
90. Tsai CY, Lu HC, Chou YH, Liu PY, Chen HY, Huang MC, et al. Gut microbial signatures for glycemic responses of GLP-1 receptor agonists in type 2 diabetic patients: A pilot study. Front Endocrinol (2021) 12:814770. doi: 10.3389/fendo.2021.814770
91. Charpentier J, Briand F, Lelouvier B, Servant F, Azalbert V, Puel A, et al. Liraglutide targets the gut microbiota and the intestinal immune system to regulate insulin secretion. Acta Diabetol (2021) 58(7):881–97. doi: 10.1007/s00592-020-01657-8
92. Smits MM, Fluitman KS, Herrema H, Davids M, Kramer MHH, Groen AK, et al. Liraglutide and sitagliptin have no effect on intestinal microbiota composition: A 12-week randomized placebo-controlled trial in adults with type 2 diabetes. Diabetes Metab (2021) 47(5):101223. doi: 10.1016/j.diabet.2021.101223
93. Singh AK, Yadav D, Sharma N, Jin JO. Dipeptidyl peptidase (DPP)-IV inhibitors with antioxidant potential isolated from natural sources: A novel approach for the management of diabetes. Pharmaceuticals (2021) 14(6):586. doi: 10.3390/ph14060586
94. Liao X, Song L, Zeng B, Liu B, Qiu Y, Qu H, et al. Alteration of gut microbiota induced by DPP-4i treatment improves glucose homeostasis. EBioMedicine. (2019) 44:665–74. doi: 10.1016/j.ebiom.2019.03.057
95. Olivares M, Neyrinck AM, Pötgens SA, Beaumont M, Salazar N, Cani PD, et al. The DPP-4 inhibitor vildagliptin impacts the gut microbiota and prevents disruption of intestinal homeostasis induced by a Western diet in mice. Diabetologia. (2018) 61(8):1838–48. doi: 10.1007/s00125-018-4647-6
96. Zhang Q, Xiao X, Li M, Yu M, Ping F, Zheng J, et al. Vildagliptin increases butyrate-producing bacteria in the gut of diabetic rats. PloS One (2017) 12(10):e0184735. doi: 10.1371/journal.pone.0184735
97. Ryan PM, Patterson E, Carafa I, Mandal R, Wishart DS, Dinan TG, et al. Metformin and dipeptidyl peptidase-4 inhibitor differentially modulate the intestinal microbiota and plasma metabolome of metabolically dysfunctional mice. Can J Diabetes. (2020) 44(2):146–55.e2. doi: 10.1016/j.jcjd.2019.05.008
98. Tian J, Jin D, Bao Q, Ding Q, Zhang H, Gao Z, et al. Evidence and potential mechanisms of traditional Chinese medicine for the treatment of type 2 diabetes: A systematic review and meta-analysis. Diabetes Obes Metab (2019) 21(8):1801–16. doi: 10.1111/dom.13760
99. Zheng Y, Ding Q, Wei Y, Gou X, Tian J, Li M, et al. Effect of traditional Chinese medicine on gut microbiota in adults with type 2 diabetes: A systematic review and meta-analysis. Phytomedicine. (2021) 88:153455. doi: 10.1016/j.phymed.2020.153455
100. Zhou R, He D, Zhang H, Xie J, Zhang S, Tian X, et al. Ginsenoside Rb1 protects against diabetes-associated metabolic disorders in kkay mice by reshaping gut microbiota and fecal metabolic profiles. J Ethnopharmacol (2023) 303:115997. doi: 10.1016/j.jep.2022.115997
101. Yao B, Pan B, Tian T, Su X, Zhang S, Li H, et al. Baihu renshen decoction ameliorates type 2 diabetes mellitus in rats through affecting gut microbiota enhancing gut permeability and inhibiting TLR4/NF-κB-mediated inflammatory response. Front Cell Infect Microbiol (2022) 12:1051962. doi: 10.3389/fcimb.2022.1051962
102. Liu M, Zhao Q, Liu J, Huang A, Xia X. Buyang huanwu decoction affects gut microbiota and lipid metabolism in a ZDF rat model of co-morbid type 2 diabetes mellitus and obesity: An integrated metabolomics analysis. Front Chem (2022) 10:1036380. doi: 10.3389/fchem.2022.1036380
103. Wang WK, Fan L, Ge F, Li Z, Zhu J, Yin K, et al. Effects of danggui buxue decoction on host gut microbiota and metabolism in GK rats with type 2 diabetes. Front Microbiol (2022) 13:1029409. doi: 10.3389/fmicb.2022.1029409
104. Li L, Huang G, Chen T, Lin H, Xu R, Cheng J, et al. Fufang fanshiliu decoction revealed the antidiabetic effect through modulating inflammatory response and gut microbiota composition. Evid Based Complement Alternat Med (2022) 2022:3255401. doi: 10.1155/2022/3255401
105. Guo X, Wang C, Zhang R, Hao X, Lv L, Ni Y, et al. Scrophulariae radix-atractylodes sinensis pair and metformin inhibit inflammation by modulating gut microbiota of high-fat diet/streptozotocin-induced diabetes in rats. Front Microbiol (2022) 13:900021. doi: 10.3389/fmicb.2022.900021
106. Yi ZY, Chen L, Wang Y, He D, Zhao D, Zhang SH, et al. The potential mechanism of liu-Wei-Di-Huang pills in treatment of type 2 diabetic mellitus: from gut microbiota to short-chain fatty acids metabolism. Acta Diabetol (2022) 59(10):1295–308. doi: 10.1007/s00592-022-01922-y
107. Zhang X, Wang H, Xie C, Hu Z, Zhang Y, Peng S, et al. Shenqi compound ameliorates type-2 diabetes mellitus by modulating the gut microbiota and metabolites. J Chromatogr B Analyt Technol BioMed Life Sci (2022) 1194:123189. doi: 10.1016/j.jchromb.2022.123189
108. Xu X, Gao Z, Yang F, Yang Y, Chen L, Han L, et al. Antidiabetic effects of gegen qinlian decoction via the gut microbiota are attributable to its key ingredient berberine. Genomics Proteomics Bioinf (2020) 18(6):721–36. doi: 10.1016/j.gpb.2019.09.007
109. Chen H, Yao Y, Wang W, Wang D. Ge-Gen-Jiao-Tai-Wan affects type 2 diabetic rats by regulating gut microbiota and primary bile acids. Evid Based Complement Alternat Med (2021) 2021:5585952. doi: 10.1155/2021/5585952
110. Zhang Y, Gu Y, Ren H, Wang S, Zhong H, Zhao X, et al. Gut microbiome-related effects of berberine and probiotics on type 2 diabetes (the PREMOTE study). Nat Commun (2020) 11(1):5015. doi: 10.1038/s41467-020-18414-8
111. Turnbaugh PJ, Ley RE, Hamady M, Fraser-Liggett CM, Knight R, Gordon JI. The human microbiome project. Nature. (2007) 449(7164):804–10. doi: 10.1038/nature06244
112. Sudlow C, Gallacher J, Allen N, Beral V, Burton P, Danesh J, et al. UK Biobank: an open access resource for identifying the causes of a wide range of complex diseases of middle and old age. PloS Med (2015) 12(3):e1001779. doi: 10.1371/journal.pmed.1001779
113. Goodrich JK, Waters JL, Poole AC, Sutter JL, Koren O, Blekhman R, et al. Human genetics shape the gut microbiome. Cell. (2014) 159(4):789–99. doi: 10.1016/j.cell.2014.09.053
114. Saa P, Urrutia A, Silva-Andrade C, Martín AJ, Garrido D. Modeling approaches for probing cross-feeding interactions in the human gut microbiome. Comput Struct Biotechnol J (2022) 20:79–89. doi: 10.1016/j.csbj.2021.12.006
115. Bishop SL, Drikic M, Wacker S, Chen YY, Kozyrskyj AL, Lewis IA. Moving beyond descriptive studies: harnessing metabolomics to elucidate the molecular mechanisms underpinning host-microbiome phenotypes. Mucosal Immunol (2022) 15(6):1071–84. doi: 10.1038/s41385-022-00553-4
116. Omori M, Kato-Kogoe N, Sakaguchi S, Kamiya K, Fukui N, Gu YH, et al. Characterization of salivary microbiota in elderly patients with type 2 diabetes mellitus: a matched case-control study. Clin Oral Investig (2022) 26(1):493–504. doi: 10.1007/s00784-021-04027-y
117. Ge X, Zhang A, Li L, Sun Q, He J, Wu Y, et al. Application of machine learning tools: Potential and useful approach for the prediction of type 2 diabetes mellitus based on the gut microbiome profile. Exp Ther Med (2022) 23(4):305. doi: 10.3892/etm.2022.11234
118. Nguyen TL, Vieira-Silva S, Liston A, Raes J. How informative is the mouse for human gut microbiota research? Dis Model Mech (2015) 8(1):1–16. doi: 10.1242/dmm.017400
119. Heiss CN, Olofsson LE. Gut microbiota-dependent modulation of energy metabolism. J Innate Immun (2018) 10(3):163–71. doi: 10.1159/000481519
120. Singhvi N, Gupta V, Gaur M, Sharma V, Puri A, Singh Y, et al. Interplay of human gut microbiome in health and wellness. Indian J Microbiol (2020) 60(1):26–36. doi: 10.1007/s12088-019-00825-x
121. Lagier JC, Khelaifia S, Alou MT, Ndongo S, Dione N, Hugon P, et al. Culture of previously uncultured members of the human gut microbiota by culturomics. Nat Microbiol (2016) 1:16203. doi: 10.1038/nmicrobiol.2016.203
122. Doestzada M, Vila AV, Zhernakova A, Koonen DPY, Weersma RK, Touw DJ, et al. Pharmacomicrobiomics: a novel route towards personalized medicine? Protein Cell (2018) 9(5):432–45. doi: 10.1007/s13238-018-0547-2
123. Liu W, Luo Z, Zhou J, Sun B. Gut microbiota and antidiabetic drugs: Perspectives of personalized treatment in type 2 diabetes mellitus. Front Cell Infect Microbiol (2022) 12:853771. doi: 10.3389/fcimb.2022.853771
124. Li H, He J, Jia W. The influence of gut microbiota on drug metabolism and toxicity. Expert Opin Drug Metab Toxicol (2016) 12(1):31–40. doi: 10.1517/17425255.2016.1121234
125. Zimmermann M, Zimmermann-Kogadeeva M, Wegmann R, Goodman AL. Mapping human microbiome drug metabolism by gut bacteria and their genes. Nature. (2019) 570(7762):462–7. doi: 10.1038/s41586-019-1291-3
126. Javdan B, Lopez JG, Chankhamjon P, Lee YJ, Hull R, Wu Q, et al. Personalized mapping of drug metabolism by the human gut microbiome. Cell. (2020) 181(7):1661–79.e22. doi: 10.1016/j.cell.2020.05.001
127. Heinken A, Basile A, Hertel J, Thinnes C, Thiele I. Genome-scale metabolic modeling of the human microbiome in the era of personalized medicine. Annu Rev Microbiol (2021) 75:199–222. doi: 10.1146/annurev-micro-060221-012134
128. Snelson M, de Pasquale C, Ekinci EI, Coughlan MT. Gut microbiome, prebiotics, intestinal permeability and diabetes complications. Best Pract Res Clin Endocrinol Metab (2021) 35(3):101507. doi: 10.1016/j.beem.2021.101507
129. Guo Y, Yu Y, Li H, Ding X, Li X, Jing X, et al. Inulin supplementation ameliorates hyperuricemia and modulates gut microbiota in uox-knockout mice. Eur J Nutr (2021) 60(4):2217–30. doi: 10.1007/s00394-020-02414-x
130. Palacios T, Vitetta L, Coulson S, Madigan CD, Lam YY, Manuel R, et al. Targeting the intestinal microbiota to prevent type 2 diabetes and enhance the effect of metformin on glycaemia: A randomised controlled pilot study. Nutrients (2020) 12(7):2041. doi: 10.3390/nu12072041
131. Hanssen NMJ, de Vos WM, Nieuwdorp M. Fecal microbiota transplantation in human metabolic diseases: From a murky past to a bright future? Cell Metab (2021) 33(6):1098–110. doi: 10.1016/j.cmet.2021.05.005
Keywords: type 2 diabetes mellitus, pharmacomicrobiomics, gut microbiome, antidiabetic drugs, treatments
Citation: Jia L, Huang S, Sun B, Shang Y and Zhu C (2023) Pharmacomicrobiomics and type 2 diabetes mellitus: A novel perspective towards possible treatment. Front. Endocrinol. 14:1149256. doi: 10.3389/fendo.2023.1149256
Received: 21 January 2023; Accepted: 14 March 2023;
Published: 23 March 2023.
Edited by:
Jian-Quan Luo, Central South University, ChinaReviewed by:
Boxun Zhang, Guang’anmen Hospital, China Academy of Chinese Medical Sciences, ChinaXuedong An, Guang’anmen Hospital, China Academy of Chinese Medical Sciences, China
Kaijian Hou, Shantou University, China
Copyright © 2023 Jia, Huang, Sun, Shang and Zhu. This is an open-access article distributed under the terms of the Creative Commons Attribution License (CC BY). The use, distribution or reproduction in other forums is permitted, provided the original author(s) and the copyright owner(s) are credited and that the original publication in this journal is cited, in accordance with accepted academic practice. No use, distribution or reproduction is permitted which does not comply with these terms.
*Correspondence: Yongguang Shang, d2Fqc3lnQDE2My5jb20=; Chunsheng Zhu, emh1Y2h1bnNoZW5nNkAxNjMuY29t
†These authors have contributed equally to this work