- 1Innovation Unit, Hospital Clínico San Carlos, Instituto de Investigación Sanitaria del Hospital Clínico San Carlos (IdISSC), Madrid, Spain
- 2Department of Endocrinology and Nutrition, Hospital Clínico San Carlos, Instituto de Investigación Sanitaria del Hospital Clínico San Carlos (IdISSC), Madrid, Spain
- 3Department of Medicine, Faculty of Medicine, Universidad Complutense de Madrid, Madrid, Spain
- 4Department of Quantitative Methods for Economics and Management, Universidad de Las Palmas de Gran Canaria, Las Palmas de Gran Canaria, Spain
- 5Department of Preventive Medicine, Hospital Clínico San Carlos, Instituto de Investigación Sanitaria del Hospital Clínico San Carlos (IdISSC), Madrid, Spain
- 6Research Unit, Hospital Universitario Nuestra Señora de Candelaria, Santa Cruz de Tenerife, Spain
- 7Department of Market Access and Public Affairs, Novo Nordisk Pharma, Sociedad Anónima (SA.), Madrid, Spain
- 8Department of Clinical, Medical and Regulatory, Novo Nordisk Pharma, Sociedad Anònima (SA.), Madrid, Spain
- 9Department of Clinical Sciences and Community Health, Università degli Studi di Milano, Milan, Italy
- 10Department of Critical Care, Hospital Clínico San Carlos, Instituto de Investigación Sanitaria del Hospital Clínico San Carlos (IdISSC), Madrid, Spain
- 11Department of Hospital Pharmacy, Hospital Clínico San Carlos, Instituto de Investigaciòn Sanitaria del Hospital Clínico San Carlos (IdISSC), Madrid, Spain
Introduction: COVID-19 and obesity relationship has been extensively studied since the COVID-19 outbreak, proving obesity is a risk factor. This study aims to broaden the available information about this association and to evaluate the economic impact of obesity and the COVID-19 disease combination.
Methods: This retrospective study analyzed a sample of 3,402 patients admitted to a Spanish hospital with available body mass index (BMI) data.
Results: The prevalence of obesity was 33.4%. Patients with obesity showed a higher risk of hospitalization (OR 95% ConfidenceInterval [CI]=1.46; [1.24-1.73]; p < 0.001), which increased with the obesity degree (I: OR [95% CI]=1.28 [1.06-1.55], p =0.010; II: OR [95% CI]=1.58 [1.16-2.15], p =0.004; III: OR [95% CI] =2.09 [1.31-3.34], p =0.002). Patients with type III obesity had a significantly higher risk of intensive care unit (ICU) admission (OR [95% CI]= 3.30 [1.67-6.53]; p = 0.001) and invasive mechanical ventilation (IMV) need (OR [95% CI]= 3.98 [2.00-7.94]; p<0.001). The average cost per patient was remarkably higher in patients with obesity (p = 0.007), reaching an excess cost of 28.41% in the study cohort and rising to 56.5% in patients < 70 years. The average cost per patient increased significantly with the degree of obesity (p = 0.007).
Discussion: In conclusion, our results suggest a strong association between obesity and adverse COVID-19 outcomes and higher expenditures in patients with both conditions.
1 Introduction
The coronavirus disease (COVID-19) pandemic has been the most notable global concern of this century. Since its outbreak at the end of 2019, researchers worldwide have made an enormous effort to investigate and draw a picture not only of the disease itself but also of its association with other common diseases. COVID-19 is a respiratory illness caused by infection with severe acute respiratory syndrome coronavirus (SARS-CoV-2). It may present as an asymptomatic, mild, moderate, or severe disease, depending on the patient baseline condition among other factors.
On the other hand, obesity is a metabolic, systemic, chronic, multifactorial disease leading to increased subcutaneous and visceral adipose tissue. It is well known that obesity promotes inflammation and it is associated with the appearance of comorbidities such as diabetes mellitus (DM), arterial hypertension (HT), and/or cardiovascular disease (CVD), strongly related with mortality in the general population (1, 2). Nevertheless, some authors have concluded that not all obese patients have a deteriorated metabolic profile, and they have described different obesity phenotypes referring to their metabolic status independent on the person weight; Metabolically Healthy Obesity (MHO) and Metabolically Obese Normal Weight (MONW). These findings received the name of “obesity paradox”, which is still controversial and debate-generating (3–5). Obesity is present in approximately 13% of adults worldwide, and it continues to rise globally (6). The prevalence of obesity in the Spanish population is worryingly higher, rising to 21.6%, according to the ENPE study published in 2016 (7). The association between obesity and inflammation is well established, as the excess nutrients lead to activation of a metabolic signaling pathway which ends up causing activation of cytokines resulting in a low-grade inflammatory response (8). Clinical evidence has demonstrated that COVID-19 patients express a high level of cytokines, known as “cytokine storm”, and present hyperinflammation, which could be the link between COVID-19 and obesity (9). Obesity already plays a significant role in the hospitalization and mortality rate in other respiratory diseases caused by viral infections, such as other coronaviruses pandemics, SARS and MERS in 2002 and 2012, respectively, or the H1N1 flu pandemic in 2009 (10, 11).
The first published data following the COVID-19 outbreak came from China and the United States, suggesting that age, male sex, obesity, HT, CVD, DM, and chronic kidney disease were factors associated with COVID-19 adverse outcomes (12–14). By 2023, the relationship between obesity and other chronic diseases and COVID-19 has been highly studied. Obesity has been determined to be a risk factor for COVID-19, and it is associated with adverse outcomes in terms of higher rates of hospital admission, intensive care unit (ICU) admission, and need for invasive mechanical ventilation (IMV) (15–17). Moreover, the prevalence of obesity seems to be higher among patients developing acute respiratory distress syndrome (ADRS) (16, 18, 19). The association between obesity and higher mortality in COVID-19 patients is still controversial, with clear evidence being found in many studies (12, 17, 20–25); but lacking a reliable association between both conditions in other investigations (15, 18, 26).
The high rates of hospitalization, ICU admission, and need for IMV occurring during the COVID-19 pandemic, added to the cost of antiviral and coadjuvant drugs, entail an enormous impact on public health expenditure (27). Clearly, these expenses may be higher if patients present other underlying diseases besides COVID-19. The presence of obesity as one of the most prevalent chronic diseases in western countries, and specifically in Spain, and the critical impact seen in Spain during the peak of the pandemic, led us to investigate the use of resources in patients with overweight and obesity, obtaining clear results about the excess cost derived from obesity (28, 29).
This study aimed to assess the clinical and economic impact of the COVID-19 disease in patients diagnosed with obesity alone or obesity along with three specific obesity-related comorbidities: DM, HT and CVD in a Spanish hospital. According to the reviewed literature and the clinicians expertise, we hypothesized the impact of the disease combination to be significantly higher than the impact of COVID-19 infection itself.
2 Materials and methods
2.1 Study design and patients
The OBESITY-COVID study is a retrospective, registry-type study including COVID-19 positive patients admitted to Hospital Clínico San Carlos in Madrid (Spain) during the first wave of the COVID-19 pandemic (from March 1, 2020 to June 30, 2020). The study was conducted in accordance with the Declaration of Helsinki, the study protocol was approved by the Ethics Committee of the participating hospital on 01/02/2021 and registered at https://www.isrctn.com/(ID ISRCTN11242213).
The cohort included 5,517 consecutive patients with a probable or certain diagnosis of COVID-19, with or without microbiological diagnostic confirmation, who had at least one visit to the emergency department (ED) during the study period. Patients met at least one of the following inclusion criteria: primary and/or secondary diagnosis codes (ICD-10) for clinically diagnosed COVID-19 (30); having a positive RT-PCR. Exclusion criteria included: being under 18 years of age, being admitted to a medicalized hotel and/or having an advanced or terminal disease. The study objectives were evaluated in the study cohort with assessed nutritional status (N=3,402) and all the analyses involving patient stratification according to body mass index (BMI) were done for a secondary cohort of 3,371 patients, since the quantitative BMI record was missing in 31 patients. Additionally, the study objectives were analyzed in a sub-cohort of patients aged less than 70 years within the overall cohort, considering N=1,871 patients with an assessed nutritional status and N=1,847 patients with quantitative BMI data.
2.2 Study endpoints
The primary endpoint was to calculate the frequency of obesity and obesity-related comorbidities, specifically DM, HT, and CVD among COVID-19 positive patients to further estimate the clinical impact and cost. The secondary objective was to investigate the association of obesity status, obesity degree and obesity-related comorbidities with the clinical outcomes of hospitalization, ICU hospitalization, need for IVM and mortality, and the outcome of healthcare cost based on resource utilization. Adjustments for secondary endpoints were based on the results of primary endpoint, including adjusting for demographic variables and the presence of DM, HT and CVD at the time of ED visit.
2.3 Data sources and variables
Demographic and clinical data used for this analysis were extracted from the BDCLIN_HCSC_COVID-19 database of the participating hospital, which integrates information from several hospital departments including the ED, microbiology department, and hospital pharmacy. CVD included coronary heart disease, stroke, hypertensive heart disease, inflammatory heart disease, rheumatic heart disease, transient ischemic attack, and other cardiovascular diseases such as tumors, cardiomyopathy, and heart valve diseases. BMI was extracted from the electronic health records at specialized care and/or primary care, in order to have the most updated measurement in each case. For each patient, the BMI value closest to the ED visit was chosen. Records older than 10 years were discarded. Based on their BMI (kg/m2) records, patients were classified into non-obesity and obesity groups (this one additionally subclassified into obesity I, II or III), according to the World Health Organization (WHO) and the Centers for Disease Control and Prevention (CDC) criteria (Obesity: BMI≥30; Obesity I: BMI=30-34.9; Obesity II: BMI=35-39.9; Obesity III: BMI≥40). Data related to ED and hospital stay (conventional hospitalization and ICU) were obtained from the Minimum Basic Hospital Discharge Data Set 2020 and the ICU database. The need for IMV was collected using the related ICD-10-PCS. Mortality outcome was assessed using two different variables 1) 30-day mortality (from the first contact with the hospital to day 30) and in-hospital mortality (from the first contact with the hospital to the event of death occurring at any time during the patient’s admission), analyzed both as a dichotomous yes/no variables. Thirty-day mortality was also analyzed as time to event (survival) using Kaplan-Meier analysis. Regarding cost variables, the categories of health expenditure considered were days in ED, length of stay in conventional hospitalization, length of stay in ICU, pharmacological treatment with a high economic impact, and prolonged IMV (having undergone tracheostomy procedure with IMV for more than 96 hours). For the length of stay in each inpatient unit, the total per patient was calculated, considering the number of admissions in each inpatient unit and stay. Additional costs of medication and procedures were included in the cost of each corresponding inpatient unit. The unit costs were obtained from the hospital departments and external sources (31). The cumulative cost per patient was calculated as the sum of all cost components, obtained by multiplying the unit cost of each health expenditure category by the patient’s use of the corresponding resource.
2.4 Statistical analyses
The analyses were performed in the global cohort and in the subgroup of patients younger than 70 years aiming to decrease the age effect on COVID-19 morbidity and mortality, according to previous research (32, 33). All data processing and analysis were performed using the Statistical Package for the Social Sciences (IBM SPSS Statistics version 26, IBM Corp., Armonk, NY, USA) and Stata 17. All p-values lower than 0.05 were deemed statistically significant.
2.4.1 Analysis of clinical variables
Sociodemographic and clinical characteristics of patients and the use of healthcare resources were analyzed by descriptive statistics. Absolute and relative frequencies were used to describe categorical variables. The Clopper-Pearson exact method was used to calculate confidence intervals for the prevalence of obesity. Quantitative variables were summarized with their mean and standard deviation (SD) and those showing a skewed distribution were summarized with median and interquartile range (IQR). For the comparison of qualitative variables, the Chi-square test or Fisher’s exact test were used, if necessary. Comparisons of means between two independent groups were performed by Student’s t-test if the variables followed a normal distribution, or by the nonparametric Mann-Whitney U test for asymmetric variables. Comparisons of means between more than two independent groups were performed by analysis of variance (ANOVA), or by the nonparametric Kruskal-Wallis test for asymmetric variables. A multivariate logistic regression analysis was performed to assess the risk of hospitalization, risk of ICU admission, need for IMV and risk of mortality associated with obesity diagnosis, obesity degree and obesity-related comorbidities categories. For each of the outcome variables, crude odds ratios (OR) adjusted for age, sex, presence of HT, DM, and history of CVD were calculated. In each table, the reference category is specified. The relationship between obesity and 30-day mortality was also explored by survival analysis using the Kaplan-Meier method, where the log-rank test was used to compare survival functions. Cox regression models were used to obtain the crude and adjusted (age, sex, presence of HT, DM, and history of CVD) effect of obesity on the 30-day mortality rate. The hazard ratio (HR) is presented as a measure of effect.
2.4.2 Analysis of economic variables
For the economic impact assessment, multivariate regression models were performed with log(cost) as the dependent variable, adjusted for covariates that could influence the cost (i.e., age, sex, country of birth, and comorbidities). To correct the selection bias caused by missing values of the variable of interest nutritional status, a missing data multiple imputation statistical procedure was designed (see Supplementary Material Tables S5, S6). An individual obesity status has been imputed to each patient with an unrecorded diagnosis of obesity, estimated from the information available in the sample. The procedure, which is applied to the whole sample and for the specific group of patients under 70 years of age, is as follows: A stratified random sample with replacement, of size 1,000 for the total sample and 800 for the under-70s, is drawn from the population of patients with known obesity status. It is stratified according to the quartiles of the age distribution of the missing group, so that its age composition is similar. A binary probit model (Table S6) of obesity is estimated whose predictors are age and its square and the dummies of sex, CVD, DM and HT, Latin American origin and other countries. The estimated equation is used to predict the probability of obesity of the missing patients, and the binary variable obese/non-obese is defined with a cut-off point of 0.5 on the predicted probability. This process (1-2) is repeated 100 times and the results of the 100 samples are recorded to obtain 100 cost estimates for both patients with and without obesity and their corresponding standard deviations.
3 Results
3.1 Study population
Between March 1, 2020 and June 30, 2020, 5,517 patients meeting the inclusion criteria were identified from the BDCLIN_HCSC_COVID-19 database, of whom 216 patients were excluded consensually from the analysis to minimize the effect of confounding factors, and 1,899 were excluded due to absence of BMI record. Therefore, 3,402 patients were evaluable for the study analysis (Figure 1). Table 1 shows the baseline demographic and clinical characteristics of patients included in the study cohort and in the subgroup of patients aged <70 years. Patient’s baseline characteristics according to obesity degree and the presence or absence of obesity-related comorbidities are shown in Supplementary Tables S1, S4, respectively.
We found a 33.4% (95% CI, 31.8-35.0) prevalence of obesity among the evaluable COVID-19 patients. The rate by sex was 34.2% (32.0-36.5%) in females and 32.5% (30.2-34.8%) in males, with no significant differences between groups (p = 0.287). Patients with obesity were significantly younger than patients without obesity (63.79 ± 17.35 years vs. 65.18 ± 19.57 years; p = 0.035). Regarding the country of origin, the prevalence of obesity was significantly lower in Spanish patients (31.4%) than in patients from Latin America (39.8%) and other countries (37.0%) (p < 0.001).
In the population aged <70 years, the rate of obesity was significantly higher than in patients older than 70 years (35.7%, 95% CI, 33.5-37.9 vs. 30.6%, 95% CI, 28.3-32.9), (p = 0.002).
Several evaluable patients (40.2%) did not show any of the studied comorbidities (DM, HT and CVD). A total of 32.2% of patients had at least one comorbidity, and 20.4% and 7.3% had two or three comorbidities, respectively. Figure 2 shows the prevalence of obesity, independent comorbidities, and combined prevalence, defined as the coexistence of a diagnosed obesity status and at least one of the obesity-related comorbidities already defined in the study cohort. The most predominant combination was obesity and HT (19.2%). There were significant differences in the obesity rate based on the diagnosis of DM (43.6% vs 30.2%, p < 0.001) and HT (37.1% vs 29.5%, p < 0.001), but not with regard to CVD (33.1% vs 33.5%, p = 0.874). In the subgroup aged <70 years, 62.4% of patients had no comorbidities, 26.4% had at least one comorbidity, and 8.9% and 2.2% had 2 and 3 comorbidities, respectively. The prevalence was 14.5% DM, 30.0% HT and 6.4% CVD
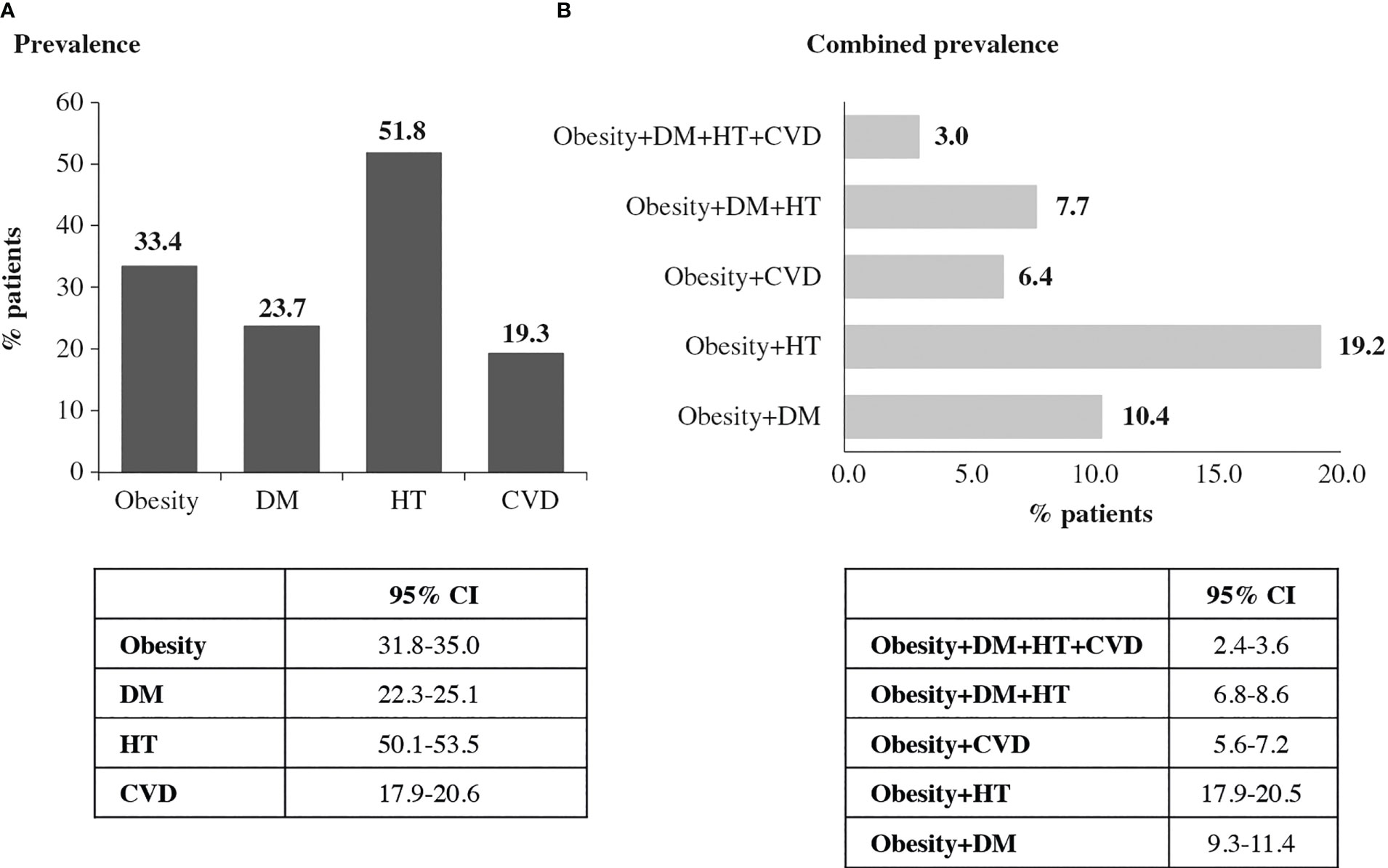
Figure 2 Obesity and obesity-related comorbidities prevalence (N=3,402). (A) Individual obesity and obesity-related comorbidities prevalence. (B) Combined obesity and obesity-related comorbidities prevalence.
3.2 Hospitalization and ICU admission
Table 2 shows the profile of patients in each inpatient unit: ED without admission, conventional hospitalization, and ICU. Overall, 60% of patients required hospitalization. Global comparisons among three groups were always significant for each variable (p < 0.001) Obesity was more prevalent in patients that required hospitalization (35.6%, 95% CI, 33.4-37.8) than in patients only visiting the ED (29.4%, 95% CI, 27.0-31.9; p < 0.001). The prevalence of obesity was also significantly higher in patients requiring ICU admission (41.2%, 95% CI, 33.3-49.4) than in non-admitted patients (35.6% 95% CI 33.4-37.8; p = 0.003). There were statistically significant differences in the distribution of obesity categories between the No admission and non-ICU admission groups (p = 0.02), between the No admission and Inpatient with ICU admission groups (p < 0.001), and between the Inpatient without ICU and Inpatient with ICU admission groups (p < 0.001) (Table 2).
3.3 Risk of hospitalization, ICU admission, IMV, and mortality
There were significant differences in the need for hospitalization according to obesity status (p < 0.001) and obesity categories (p = 0.007). The multivariate logistic regression analysis showed that patients with obesity had a higher risk of hospitalization than patients without obesity (OR [95% CI]=1.46;, [1.24-1.73] p < 0.001), and the risk of hospitalization increases with increasing obesity (type 1: OR[95% CI]= 1.28[1.06-1.55], p =0.010 < type II: OR[95% CI]= 1.58[1.16-2.15], p =0.004 < type III: OR[95% CI]= 2.09[1.31-3.34], p =0.002). There were significant differences in the need for ICU admission and IMV according to obesity categories (p < 0.001). In addition, patients with type III obesity had a significantly higher risk of ICU admission (OR[95% CI]: 3.30 [1.66-6.53]; p = 0.001) and IMV need (OR[95% CI]= 3.98[2.00-7.94] p < 0.001) as compared to those without obesity (Table 3). There were no significant differences in the need for ICU admission according to obesity condition (p = 0.168). There was a significant association between the need for IMV and obesity status (p = 0.032); however, no association between exposure and outcome variables was observed.
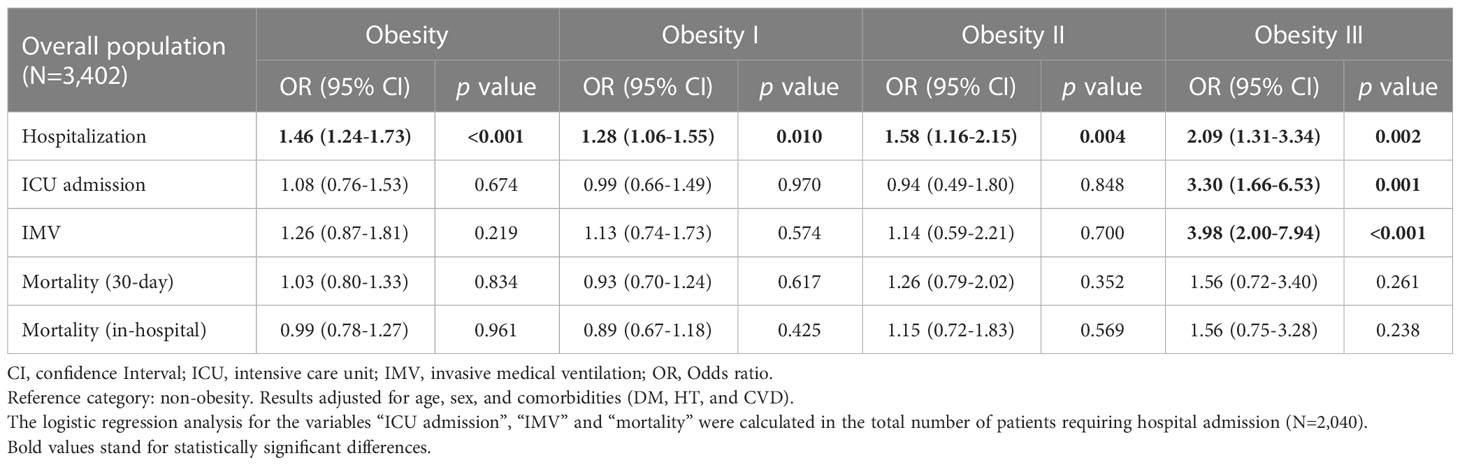
Table 3 Risk of hospitalization, ICU admission, IMV and mortality based on the presence of obesity and obesity degree in the overall population.
Results of the analyses on the combination of obesity status and the presence of obesity-related comorbidities are shown in Table 4. There were significant differences in the need for hospitalization according to comorbidity categories (p < 0.001). Patients with comorbidities (obese and non-obese) showed a higher risk of hospitalization as compared to patients without obesity and without any comorbidity. There were significant differences in the need for ICU admission (p = 0.018) and the need for IMV (p = 0.005) according to comorbidity categories; however, no association between exposure and outcome variables was observed. According to the Kaplan-Meier analysis, survival was significantly longer in patients without comorbidities, regardless of the presence of obesity (p < 0.001). However, the Cox regression model adjusting for age and sex showed that there was no association between comorbidity categories and survival.
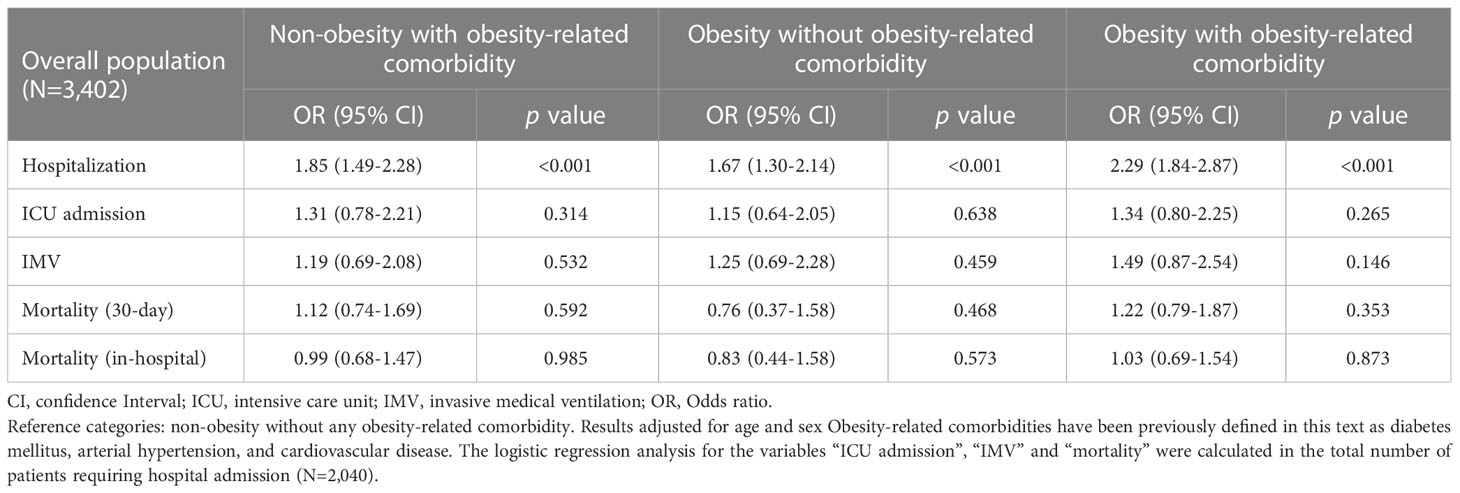
Table 4 Risk of hospitalization, ICU admission, IMV and mortality based on the presence of comorbidities in the overall population.
Post hoc analyses in the subgroup of patients aged <70 showed similar results (Supplementary Materials Tables S2, 3). No significant associations were found with any of the outcome variables in patients over 70 years of age.
3.4 Mortality
The in-hospital mortality rate was significantly higher in patients without obesity than in those with obesity (22.2% vs. 17.8%; p=0.018). There were also significant differences in 30-day mortality from the first ED contact within the cohort of hospitalized patients (N=2,040) according to obesity status, with a higher mortality rate in non-obese patients (21.0% vs. 17.0%; p = 0.029). The regression analysis showed no differences in the risk of in-hospital or 30-day mortality in patients with and without obesity, and neither across obesity degrees (Table 3). The Kaplan-Meier analysis showed a significantly higher survival in patients with obesity compared with non-obese patients (p = 0.032). However, neither of the groups reached the median OS at day 30 (Supplementary Material Figure S1). The results of the Cox regression model adjusting for age, sex and comorbidities show that there was no association between obesity status and overall survival (HR=1.05; 95% CI 0.84-1.30; p=0.669). The median 30-day OS was not reached in any obesity category (Supplementary Material Figure S2), and there were no significant differences in survival according to obesity categories (p = 0.329) and no association between obesity categories and survival was found.
Among patients under 70 years of age, no significant association was found with the mortality outcomes based on obesity status or categories (Table S2). The median 30-day OS was not reached in any of the groups (obesity vs. non-obesity) and no statistically significant differences were found (p = 0.365). Similarly, no obesity category reached the median 30-day OS and no statistically significant differences were found among categories (p = 0.329; Supplementary Material Figures S3, 4). The results of the Cox regression model adjusting for age, sex and comorbidities showed no association between obesity categories and survival (HR=1.31; CI 95% 0.46-3.70; p = 0.612).
3.5 Healthcare resource use and cost
The total average cost per patient for patients with obesity (N=3,402) was €10,805, substantially higher than the average cost per patient in patients without obesity (€8,418.30, p = 0.007). Table 5 shows the mean cost according to obesity status in the overall population and patients under 70 years, including cost for each healthcare resource use and the percentage excess cost associated with obesity status. The excess cost of obesity in the study cohort was 28.4%, reaching 56.5% in patients under 70 years of age. When the data was imputed in those patients with no available BMI record, the magnitude of the obesity excess cost is even higher (Table S6).
There were significant differences in the mean total cost by BMI category (p=0.007), the average cost increased with obesity degree (Figure 3). The lowest cost corresponds to the normal weight category (€ 6,964.42), while the highest cost corresponds to the grade III obesity category (€ 14,523.22). Moreover, the average cost per patient rises with the combination of obesity and two comorbidities (€ 12,676.61) or three OAC (€ 15,039.97) (Figure 4).
4 Discussion
The OBESITY-COVID study evaluated the prevalence of obesity and its associated comorbidities in a large cohort of patients attending the emergency service of a Spanish hospital during the first wave of the COVID-19 pandemic. Our results show a high prevalence of obesity (33.4%) and comorbidities (HT: 51.8%, DM: 23.7% and CVD: 19.3%) among COVID-19 patients in the participating center, an excess cost burden of obesity of 28%, and a higher risk of hospital admission, ICU admission and need for IMV for patients with obesity. However, we did not find a significant association between obesity and COVID-19 mortality. To our knowledge, this is the first Spanish study specifically evaluating the rate and association of obesity and comorbidities with costs in a large cohort of patients.
Our results confirmed obesity as a robust predictor of critical outcomes in COVID-19 patients (21, 24, 34–36). We found a higher rate of obesity in those patients requiring hospitalization (35.6%), and even higher in patients requiring ICU admission (41.2%). The odds of being hospitalized were significantly higher when the patients had obesity, and they increased with BMI, reproducing what has been described in large cohorts (37). Patients with BMI over 40 kg/m2 had a higher risk of hospitalization, ICU admission and to receive IMV, regardless of age, sex and diagnosis of comorbidities. This increased odds for ICU admission in grade III obesity, without being significant for obese status, has been described in similar studies (24, 38). Previous studies used a cut-off point around 70 years of age to stratify the sample, finding a greater association in the need for hospitalization among patients under 65 years (37) or under 70 years of age (39). Other studies have found a more significant association when stratifying the sample below 60 years (40) or even 50 years (38, 41). Our study also describes a higher association between obesity and obesity degrees with the need for hospitalization in those under 70 years of age, with no significant association found in the age range over 70 years. In the need for ICU admission and the need for IMV, no differences were observed stratifying by age, which should be analysed considering that the average age of the patient admitted to the ICU is 60.6 years.
Both in-hospital mortality and 30-day mortality rates were significantly lower in patients with obesity, and we found no association between mortality and obesity in the multivariate analysis. These findings are in line with the results obtained in three relevant studies. One of them is the systematic review and meta-analysis of 120 studies, which could not find an association between obesity status and mortality in COVID-19 patients (26). Moreover, the HOPE-COVID-19 retrospective cohort registry, evaluating a similar-sized cohort of patients, suggested that BMI was not a mortality predictor (15). Finally, a study performed in Madrid with a cohort of patients receiving antiviral treatment for COVID-19 reported a relationship between obesity and the development of acute respiratory distress syndrome, but not between obesity and mortality (18). Nevertheless, some other large cohort studies and meta-analyses demonstrated a reliable link between obesity and mortality (12, 17, 20–25). Based on these controversial results, the potential association between obesity and mortality in COVID-19 patients deserves to be studied in greater depth.
This study showed a rate of obesity of 33.4%, which increases to 35.7% in patients younger than 70 years old. This percentage is substantially higher than the obesity prevalence found in the SIESTA cohort, representing almost all the Spanish territory (14.3%) (20), and in the study by Rodriguez-Gonzalez et al. (15.1%) (18), which included a cohort from the same region as ours. This might be due to differences in patient population, since the SIESTA cohort consisted of a random sample of patients clinically or microbiologically diagnosed with COVID-19, and many were asymptomatic patients not attending the ED, while all patients included in our study came from the ED. The obesity prevalence found in our cohort is within the range of that reported in larger studies, ranging from 25 to 42% (23, 25, 26, 29, 34, 35, 42). The obesity rate by sex and age range is comparable to that reported in the ENRICA study (43),which shows an increase in the prevalence of obesity over 65 years of age (44), suggesting that our cohort is representative of the Spanish population and that the results of our study could be aligned with it.
The DM rate found in our study (23.7%) is slightly higher than the range of prevalence previously reported for this condition in COVID-19 patients (17-22%) (18, 20, 26, 35, 42). Similarly, the HT rate (51.8%) observed in our study was slightly higher than that found in prior reports ranging from 32 to 45% (18, 20, 26, 35, 42). CVD prevalence in COVID-19 patients showed variability between studies (13% (35), 16% (26) and 31.4% (18)). Our data remains within this range (19.3%). In the subgroup of patients under 70 years of age, the prevalence of comorbidities was significantly lower than in the overall cohort.
We found that patients with comorbidities had a higher risk of hospitalization and those without comorbidities showed the highest OS, regardless of their BMI, in line with prior reports (14, 26, 35, 45). However, Spanish investigators could not find an association between the presence of DM and CVD and mortality in the SIESTA cohort (20).
Some studies carried out in Spain had evaluated resource utilization in cohorts of COVID-19 patients at different stages of the disease (18, 27) but, to our knowledge, none of the studies conducted so far has undertaken a comparative analysis between patients with and without obesity. As expected, our data show that the cost per patient was significantly higher in patients with obesity compared to patients without obesity, with an excess cost of 28.4% in the overall cohort and 56.5% in patients under 70 years of age. Additionally, there is a significant increase in the average cost per patient when the obesity degree increases, with the highest excess cost in subjects with grade III obesity. Furthermore, we observed a rising trend in the average cost when the number of comorbidities increases, but it was not statistically significant, likely because the number of patients decreases as the number of comorbidities increases.
This increase in health expenditure in patients with obesity has been previously reported. In a cohort of patients from 273 hospitals located in the U.S., overweight and obesity were associated with higher economic cost of inpatient care, with the highest excess cost in patients with BMI over 45kg/m2 (46). This increased cost associated with obesity status is also reported in a European study evaluating the costs of COVID-19 associated with obesity in the first 6 months of the pandemic (29). According to this study, the average costs for patients without obesity are aligned with ours (taking into account differences in the study timeframe, 6 months vs. 3 months), but the average cost for patients with obesity is substantially higher. Differences with this European study might come from differences in the study design (cost model estimation vs. single-center observational study). The economic excess cost associated with obesity, along with the increased risk of critical outcomes, reinforce the need to address obesity treatment as a health priority.
This investigation has some limitations. First, participation of a single center located in Madrid, which was one of the most severely stricken areas during the COVID-19 pandemic in Spain, may entail bias. Data such as risk for hospitalization, ICU admission and in-hospital mortality in obese patients cannot be extrapolated to other countries or other regions in Spain. Secondly, the timeframe in which this study was conducted corresponds to the pandemic first wave, from March to June 2020, a period in which emergency and inpatient activity, and especially ICU occupancy, were determined by the high occupancy demand of Madrid’s community hospitals (46). Despite its limitations, this study provides valuable data from a large cohort of Spanish patients during the most critical period of the COVID-19 pandemic, assessing clinical and economic aspects of the disease.
We must consider these results carefully due to the rapid evolution of the pandemic since the outbreak at the end of 2019 to the current situation in 2023. The virus strain has been evolving and changing its virulence, the standard of care has changed according to scientific evidence and almost all of the population is fully vaccinated, therefore this must be borne in mind when comparing hospitalization, ICU admission and mortality rates as well as the cost analysis from 2020 to the present.
In conclusion, this study suggests a strong association between obesity and inpatient hospitalization, ICU admission, need for IMV in COVID-19 patients and economic excess cost. The risk for adverse outcomes and cost increases with obesity degree and the presence of comorbidities, regardless of obesity status. Likewise, obesity has been associated with poorer clinical outcomes and higher costs in other pandemics that occurred in this century or other noninfectious diseases, therefore we must promote the management of obesity as a health priority
Data availability statement
The original contributions presented in the study are included in the article/Supplementary Material. Further inquiries can be directed to the corresponding author.
Ethics statement
The studies involving human participants were reviewed and approved by Hospital Clínico San Carlos Ethics Committee. Written informed consent for participation was not required for this study in accordance with the national legislation and the institutional requirements.
Author contributions
T-EM, LM, R-HM, MV and PF contributed to conceptualization of the study; T-EM, F-FM. and GB contributed with the study methodology; F-FM, GB, B-PP and SC performed the statistical analysis; T-EM, R-HM, MV, PF and LM conducted the investigation; T-EM, NA, G-PC participated in resource searching; T-EM, NA, G-PC contributed to data curation; T-EM, MV, and R-HM wrote the original draft; T-EM, R-HM, LM participated in the supervision; T-EM, LM, R-HM, MV, PF, F-FM, GB and SC contributed to manuscript revision. All authors contributed to the article and approved the submitted version.
Funding
The authors declare that this study received funding from Novo Nordisk Pharma. The funder had the following involvement with the study: study design and review of manuscript for publication. Novo Nordisk Pharma had no role in data collection, data analysis, data interpretation, or writing of the study report. The APC was funded by Novo Nordisk Pharma.
Acknowledgments
The authors would like to thank participating patients and both medical and nursing staff involved in the conduct of the study. The authors would also like to acknowledge Reyes Prieto from Dynamic Science S.L.U. (Evidenze Clinical Research) for providing medical writing assistance.
Conflict of interest
R-HM reports professional fees from Novo Nordisk, outside the submitted work. MV and PF are employees of Novo Nordisk.
The remaining authors declare that the research was conducted in the absence of any commercial or financial relationships that could be construed as a potential conflict of interest.
Publisher’s note
All claims expressed in this article are solely those of the authors and do not necessarily represent those of their affiliated organizations, or those of the publisher, the editors and the reviewers. Any product that may be evaluated in this article, or claim that may be made by its manufacturer, is not guaranteed or endorsed by the publisher.
Supplementary material
The Supplementary Material for this article can be found online at: https://www.frontiersin.org/articles/10.3389/fendo.2023.1146517/full#supplementary-material
References
1. Landsberg L, Aronne LJ, Beilin LJ, Burke V, Igel LI, Lloyd-Jones D, et al. Obesity-related hypertension: pathogenesis, cardiovascular risk, and treatment: a position paper of the obesity society and the American society of hypertension. J Clin Hypertens (Greenwich) (2013) 15(1):14–33. doi: 10.1111/jch.12049
2. Andolfi C, Fisichella PM. Epidemiology of obesity and associated comorbidities. J Laparoendosc Adv Surg Tech A (2018) 28(8):919–24. doi: 10.1089/lap.2018.0380
3. Karelis AD, Faraj M, Bastard J-P, St-Pierre DH, Brochu M, Prud’homme D, et al. The metabolically healthy but obese individual presents a favorable inflammation profile. J Clin Endocrinol Metab (2005) 90(7):4145–50. doi: 10.1210/jc.2005-0482
4. Wildman RP, Muntner P, Reynolds K, McGinn AP, Rajpathak S, Wylie-Rosett J, et al. The obese without cardiometabolic risk factor clustering and the normal weight with cardiometabolic risk factor clustering: prevalence and correlates of 2 phenotypes among the US population (NHANES 1999-2004). Arch Intern Med (2008) 168(15):1617–24. doi: 10.1001/archinte.168.15.1617
5. Ortega FB, Lavie CJ, Blair SN. Obesity and cardiovascular disease. Circ Res (2016) 118(11):1752–70. doi: 10.1161/CIRCRESAHA.115.306883
6. World Health Statistics 2022. Monitoring health for the SDGs, sustainable development goals. Geneva: World Health Organization (2022).
7. Aranceta-Bartrina J, Pérez-Rodrigo C, Alberdi-Aresti G, Ramos-Carrera N, Lázaro-Masedo S. Prevalence of general obesity and abdominal obesity in the Spanish adult population (Aged 25-64 years) 2014-2015: the ENPE study. Rev Esp Cardiol (Engl Ed) (2016) 69(6):579–87. doi: 10.1016/j.recesp.2016.02.010
8. Kolb R, Sutterwala FS, Zhang W. Obesity and cancer: inflammation bridges the two. Curr Opin Pharmacol (2016) 29:77–89. doi: 10.1016/j.coph.2016.07.005
9. Kunnumakkara AB, Rana V, Parama D, Banik K, Girisa S, Henamayee S, et al. COVID-19, cytokines, inflammation, and spices: how are they related? Life Sci (2021) 284:119201. doi: 10.1016/j.lfs.2021.119201
10. Morgan OW, Bramley A, Fowlkes A, Freedman DA, Taylor TH, Gargiullo P, et al. Morbid obesity as a risk factor for hospitalization and death due to 2009 pandemic influenza A(H1N1) disease. PloS One (2010) 5(3):e9694. doi: 10.1371/journal.pone.0009694
11. Muniyappa R, Gubbi S. COVID-19 pandemic, coronaviruses, and diabetes mellitus. Am J Physiol Endocrinol Metab (2020) 318(5):E736–41. doi: 10.1152/ajpendo.00124.2020
12. Tartof SY, Qian L, Hong V, Wei R, Nadjafi RF, Fischer H, et al. Obesity and mortality among patients diagnosed with COVID-19: results from an integrated health care organization. Ann Intern Med (2020) 173(10):773–81. doi: 10.7326/M20-3742
13. Petrilli CM, Jones SA, Yang J, Rajagopalan H, O'Donnell L, Chernyak Y, et al. Factors associated with hospital admission and critical illness among 5279 people with coronavirus disease 2019 in new York city: prospective cohort study. BMJ (2020) 369:m1966. doi: 10.1136/bmj.m1966
14. Wu Z, McGoogan JM. Characteristics of and important lessons from the coronavirus disease 2019 (COVID-19) outbreak in China: summary of a report of 72314 cases from the Chinese center for disease control and prevention. JAMA (2020) 323(13):1239–42. doi: 10.1001/jama.2020.2648
15. Abumayyaleh M, Núñez Gil IJ, El-Battrawy I, Estrada V, Becerra-Muñoz VM, Aparisi A, et al. Does there exist an obesity paradox in COVID-19? insights of the international HOPE-COVID-19-registry. Obes Res Clin Pract (2021) 15(3):275–80. doi: 10.1016/j.orcp.2021.02.008
16. Cai SH, Liao W, Chen S-W, Liu L-L, Liu S-Y, Zheng Z-D. Association between obesity and clinical prognosis in patients infected with SARS-CoV-2. Infect Dis Poverty (2020) 9(1):80. doi: 10.1186/s40249-020-00703-5
17. Hendren NS, de Lemos JA, Ayers C, Das SR, Rao A, Carter S, et al. Association of body mass index and age with morbidity and mortality in patients hospitalized with COVID-19: results from the American heart association COVID-19 cardiovascular disease registry. Circulation (2021) 143(2):135–44. doi: 10.1161/CIRCULATIONAHA.120.051936
18. Rodriguez-Gonzalez CG, Chamorro-de-Vega E, Valerio M, Amor-Garcia MA, Tejerina F, Sancho-Gonzalez M, et al. COVID-19 in hospitalised patients in Spain: a cohort study in Madrid. Int J Antimicrob Agents (2021) 57(2):106249. doi: 10.1016/j.ijantimicag.2020.106249
19. Moser JS, Galindo-Fraga A, Ortiz-Hernández AA, Gu W, Hunsberger S, Galán-Herrera J-F, et al. Underweight, overweight, and obesity as independent risk factors for hospitalization in adults and children from influenza and other respiratory viruses. Influenza Other Respir Viruses (2019) 13(1):3–9. doi: 10.1111/irv.12618
20. Gil-Rodrigo A, Miró Ò, Piñera P, Burillo-Putze G, Jiménez S, Martín A, et al. Analysis of clinical characteristics and outcomes in patients with COVID-19 based on a series of 1000 patients treated in Spanish emergency departments. Emergencias (2020) 32(4):233–41.
21. Yang J, Tian C, Ying Chen Y, Zhu C, Chi H, Li J. Obesity aggravates COVID-19: an updated systematic review and meta-analysis. J Med Virol (2021) 93(5):2662–74. doi: 10.1002/jmv.26677
22. Du Y, Lv Y, Zha W, Zhou N, Hong X. Association of body mass index (BMI) with critical COVID-19 and in-hospital mortality: a dose-response meta-analysis. Metabolism (2021) 117:154373. doi: 10.1016/j.metabol.2020.154373
23. Pranata R, Lim MA, Yonas E, Vania R, Lukito AA, Siswanto BB, et al. Body mass index and outcome in patients with COVID-19: a dose-response meta-analysis. Diabetes Metab (2021) 47(2):101178. doi: 10.1016/j.diabet.2020.07.005
24. Popkin BM, Du S, Green WD, Beck MA, Algaith T, Herbst CH, et al. Individuals with obesity and COVID-19: a global perspective on the epidemiology and biological relationships. Obes Rev (2020) 21(11):e13128. doi: 10.1111/obr.13128
25. Ho JSY, Fernando DI, Chan MY, Sia CH. Obesity in COVID-19: a systematic review and meta-analysis. Ann Acad Med Singap (2020) 49(12):996–1008. doi: 10.47102/annals-acadmedsg.2020299
26. Thakur B, Dubey P, Benitez J, Torres JP, Reddy S, Shokar N, et al. A systematic review and meta-analysis of geographic differences in comorbidities and associated severity and mortality among individuals with COVID-19. Sci Rep (2021) 11(1):8562. doi: 10.1038/s41598-021-88130-w
27. Carrera-Hueso FJ, Alvarez-Arroyo L, Poquet-Jornet JE, Vazquez-Ferreiro P, Martinez-Gonzalbez R, El-Qutob E, et al. Hospitalization budget impact during the COVID-19 pandemic in Spain. Health Econ Rev (2021) 11(1):43. doi: 10.1186/s13561-021-00340-0
28. Espallardo O, Busutil R, Torres A, Zozaya N, Villoro R, Hidalgo-Vega A, et al. The impact of severe obesity on healthcare resource utilisation in Spain. Obes Surg (2017) 27(8):2058–66. doi: 10.1007/s11695-017-2596-7
29. Czernichow S, Bain SC, Capehorn M, Bogelund M, Madsen ME, Yssing C, et al. Costs of the COVID-19 pandemic associated with obesity in Europe: a health-care cost model. Clin Obes (2021) 11(2):e12442. doi: 10.1111/cob.12442
30. Organization, W.H. Emergency used ICD codes for COVID-19 disease outbreak. (2020). Available at: https://www.who.int/standards/classifications/classification-of-diseases/emergency-use-icd-codes-for-covid-19-disease-outbreak. (Accessed June 2022).
31. WHO. ORDEN 727/2017, de 7 de agosto, del consejero de sanidad, por la que se fijan los precios públicos por la prestación de los servicios y actividades de naturaleza sanitaria de la red de centros de la comunidad de Madrid. (Madrid) (2017).
32. Sattar N, Ho FK, Gill JM, Ghouri N, Gray SR, Celis-Morales CA, et al. BMI and future risk for COVID-19 infection and death across sex, age and ethnicity: preliminary findings from UK biobank. Diabetes Metab Syndr (2020) 14(5):1149–51. doi: 10.1016/j.dsx.2020.06.060
33. Rubio Herrera MA, Breton Lesmes I. Obesity in the COVID era: a global health challenge. Endocrinol Diabetes Nutr (Engl Ed) (2021) 68(2):123–9. doi: 10.1016/j.jhqr.2022.02.002
34. Yang J, Ma Z, Lei Y. A meta-analysis of the association between obesity and COVID-19. Epidemiol Infect (2020) 149:e11. doi: 10.1017/S0950268820003027
35. Zhou Y, Yang Q, Chi J, Dong B, Lv W, Shen L, et al. Comorbidities and the risk of severe or fatal outcomes associated with coronavirus disease 2019: a systematic review and meta-analysis. Int J Infect Dis (2020) 99:47–56. doi: 10.1016/j.ijid.2020.07.029
36. Foldi M, Farkas N, Kiss S, Zadori N, Vancsa S, Szako L, et al. Obesity is a risk factor for developing critical condition in COVID-19 patients: a systematic review and meta-analysis. Obes Rev (2020) 21(10):e13095. doi: 10.1111/obr.13095
37. Kompaniyets L, Goodman AB, Belay B, Freedman DS, Sucosky MS, Lange SJ, et al. Body mass index and risk for COVID-19-Related hospitalization, intensive care unit admission, invasive mechanical ventilation, and death - united states, march-December 2020. MMWR Morb Mortal Wkly Rep (2021) 70(10):355–61. doi: 10.15585/mmwr.mm7010e4
38. Fresan U, Guevara M, Elia F, Albeniz E, Burgui C, Castilla J, et al. Independent role of severe obesity as a risk factor for COVID-19 hospitalization: a Spanish population-based cohort study. Obes (Silver Spring) (2021) 29(1):29–37. doi: 10.1002/oby.23029
39. Bellini B, Cresci B, Cosentino C, Profili F, Bartolacci S, Scoccimarro D, et al. Obesity as a risk factor for hospitalization in COronaVirus disease-19 (COVID-19) patients: analysis of the Tuscany regional database. Nutr Metab Cardiovasc Dis (2021) 31(3):769–73. doi: 10.1016/j.numecd.2020.11.030
40. Recalde M, Pistillo A, Fernandez-Bertolin S, Roel E, Aragon M, Freisling H, et al. Body mass index and risk of COVID-19 diagnosis, hospitalization, and death: a cohort study of 2 524 926 catalans. J Clin Endocrinol Metab (2021) 106(12):e5030–42. doi: 10.1210/clinem/dgab546
41. Gao M, Piernas C, Astbury NM, Hippisley-Cox J, O'Rahilly S, Aveyard P, et al. Associations between body-mass index and COVID-19 severity in 6.9 million people in England: a prospective, community-based, cohort study. Lancet Diabetes Endocrinol (2021) 9(6):350–9. doi: 10.1016/S2213-8587(21)00089-9
42. Moazzami B, Chaichian S, Kasaeian A, Djalalinia S, Akhlaghdoust M, Eslami M, et al. Metabolic risk factors and risk of covid-19: a systematic review and meta-analysis. PloS One (2020) 15(12):e0243600. doi: 10.1371/journal.pone.0243600
43. Gutierrez-Fisac JL, Leon-Munoz LM, Regidor E, Banegas J, Rodriguez-Artalejo F. Trends in obesity and abdominal obesity in the older adult population of Spain (2000-2010). Obes Facts (2013) 6(1):1–8. doi: 10.1159/000348493
44. Gutierrez-Fisac JL, Guallar-Castillon P, Leon-Munoz LM, Graciani A, Banegas JR, Rodriguez-Artalejo F. Prevalence of general and abdominal obesity in the adult population of Spain, 2008-2010: the ENRICA study. Obes Rev (2012) 13(4):388–92. doi: 10.1111/j.1467-789X.2011.00964.x
45. Singh AK, Gupta R, Ghosh A, Misr A. Diabetes in COVID-19: prevalence, pathophysiology, prognosis and practical considerations. Diabetes Metab Syndr (2020) 14(4):303–10. doi: 10.1016/j.dsx.2020.04.004
Keywords: COVID-19, obesity, invasive mechanical ventilation (IMV), intensive care unit (ICU), economic burden, obesity comorbidities, diabetes mellitus
Citation: M T-E, MA R-H, B G L-V, ME F-F, V M, F P, P B-P, C S, A N, C G-P and M L (2023) Clinical and economic impact of COVID-19 on people with obesity in a Spanish cohort during the first pandemic peak. Front. Endocrinol. 14:1146517. doi: 10.3389/fendo.2023.1146517
Received: 17 January 2023; Accepted: 09 May 2023;
Published: 26 May 2023.
Edited by:
Jeff M. P. Holly, University of Bristol, United KingdomReviewed by:
Neftali Eduardo Antonio-Villa, National Institute of Cardiology Ignacio Chavez, MexicoMax Carlos Ramírez-Soto, University of San Martín De Porres, Peru
Copyright © 2023 M, MA, B, ME, V, F, P, C, A, C and M. This is an open-access article distributed under the terms of the Creative Commons Attribution License (CC BY). The use, distribution or reproduction in other forums is permitted, provided the original author(s) and the copyright owner(s) are credited and that the original publication in this journal is cited, in accordance with accepted academic practice. No use, distribution or reproduction is permitted which does not comply with these terms.
*Correspondence: Rubio-Herrera MA, bWFydWJpb2hAZ21haWwuY29t; Torrego-Ellacuría M, bWFjYXJlbmEudG9ycmVnb0BnbWFpbC5jb20=