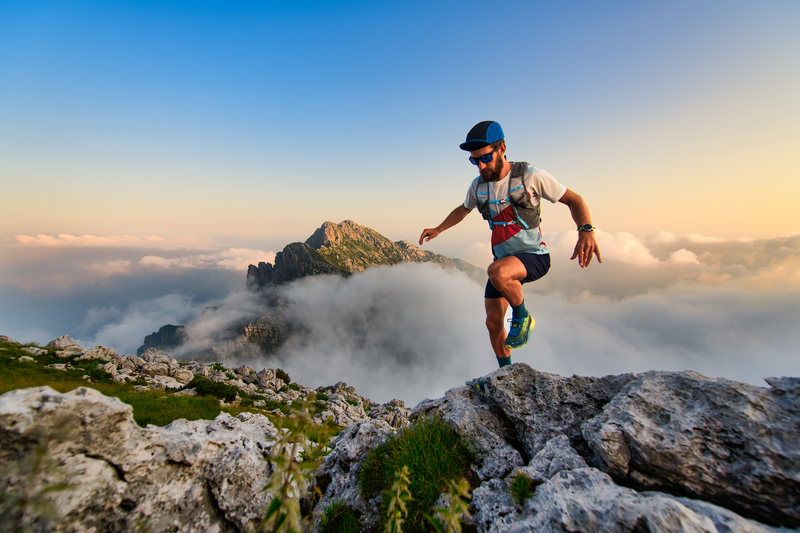
95% of researchers rate our articles as excellent or good
Learn more about the work of our research integrity team to safeguard the quality of each article we publish.
Find out more
ORIGINAL RESEARCH article
Front. Endocrinol. , 06 March 2023
Sec. Cancer Endocrinology
Volume 14 - 2023 | https://doi.org/10.3389/fendo.2023.1142796
This article is part of the Research Topic Substance and Energy Metabolism Associated with Neuroendocrine Regulation in Tumor Cells View all 41 articles
Purpose: The aim of this study was to established a dynamic nomogram for assessing the risk of bone metastasis in patients with thyroid cancer (TC) and assist physicians to make accurate clinical decisions.
Methods: The clinical data of patients with TC admitted to the First Affiliated hospital of Nanchang University from January 2006 to November 2016 were included in this study. Demographic and clinicopathological parameters of all patients at primary diagnosis were analyzed. Univariate and multivariate logistic regression analysis was applied to build a predictive model incorporating parameters. The discrimination, calibration, and clinical usefulness of the nomogram were evaluated using the C-index, ROC curve, calibration plot, and decision curve analysis. Internal validation was evaluated using the bootstrapping method.
Results: A total of 565 patients were enrolled in this study, of whom 25 (4.21%) developed bone metastases. Based on logistic regression analysis, age (OR=1.040, P=0.019), hemoglobin (HB) (OR=0.947, P<0.001) and alkaline phosphatase (ALP) (OR=1.006, P=0.002) levels were used to construct the nomogram. The model exhibited good discrimination, with a C-index of 0.825 and good calibration. A C-index value of 0.815 was achieved on interval validation analysis. Decision curve analysis showed that the nomogram was clinically useful when intervention was decided at a bone metastases possibility threshold of 1%.
Conclusions: This dynamic nomogram, with relatively good accuracy, incorporating age, HB, and ALP, could be conveniently used to facilitate the prediction of bone metastasis risk in patients with TC.
Thyroid cancer (TC) is an uncommon endocrine cancer that accounts for approximately 1% of all new malignancies, roughly 0 - 5% of cancers in men and 1 - 5% of cancers in women (1–3). However, the incidence of thyroid cancer has been increasing for more decades (4). In the United States, the incidence of thyroid cancer tripled from 4.5 per 100,000 population in 1974 to 14.4 in 2013 (5). Differentiated thyroid cancer (DTC) of low malignancy accounted for the highest proportion of thyroid cancer (90%), including papillary carcinoma (70-75%) and follicular carcinoma (15-20%) (6). Undifferentiated carcinomas, which are anaplastic malignancies, accounts for less than 5% (6, 7). Therefore, the prognosis of patients with thyroid cancer is generally good, with a 10-year survival rate of 80-95% (8). Distant metastasis is an important risk factor for patients with thyroid cancer. Compared with simple DTC patients, the 10-year survival rate of patients with distant metastasis is decreased by about 50% (3, 8). Bone is the third most common metastasis site in patients with TC, occurring in 2-13% of DTC patients (9–11) Compared with other distant metastases, bone metastases cause bone pain, pathological fractures and spinal cord compression, which significantly impaired their quality of life (12). Early diagnosis and intervention in such patients was important role for increasing patient survival rates (13, 14).
Bone scintigraphy and other nuclear studies, such as FDG-PET and SPECT, have high sensitivity and specificity for the early detection of bone metastases (15). However, their use is often limited due to high cost and radiation damage to patients (16). Thus, it is of great significance to develop a simple and feasible new method for early prediction of thyroid cancer bone metastasis. Nomograms have proven useful as models for predicting the occurrence of clinical events, and can allow visualization of incidence (17). In this study, we aimed to developed a valid nomogram model for assessing the risk of bone metastases in patients with TC to assist physicians in making accurate clinical decision.
This study was approved by the Ethics Committee of the First Affiliated Hospital of Nanchang University, and all participants signed written informed consent form. From January 2006 to November 2016, patients newly diagnosed with TC in our hospital were included in this study. All diagnoses were confirmed by needle biopsy or open surgical biopsy. The exclusion criteria were as follows: (1) Patients with other primary malignancies; (2) Patients with renal and/or liver insufficiency; (3) Patients with bone metabolic disorders; (4) Patients with significant hematological disease; (5) Missing critical information. The detailed screening process is shown in Figure 1.
Bone scintigraphy was used to identify possible bone metastases in patients. If necessary, magnetic resonance imaging and local computed tomography were conducted to confirm possible diagnoses of bone metastases.
Demographic and clinicopathological parameters of all patients at primary diagnosis (before receiving clinical treatment) were collected, including age, serum concentrations of calcium, hemoglobin (HB), free triiodothyronine, free thyroxine 4, thyroid stimulating hormone, alkaline phosphatase (ALP), and common tumor markers (carcinoembryonic antigen, alpha fetoprotein, cancer antigen-125 (CA125), CA153, and CA199). Correlations between clinicopathological parameters and bone metastases were analyzed in patients with TC.
All statistical analyses were performed using SPSS (version 26) and R (version 3.6.3) software. Qualitative variables were analyzed by Chi-square test, and quantitative variables were analyzed by Student’s t-test. Univariate analyses were initially used to identify variables that may affect bone metastases, and correlated variables (P<0.05) were included in multivariate logistic regression analysis to identify independent predictors of bone metastasis of thyroid cancer. Then, the selected independent risk factors were used to construct a dynamic nomogram for predicting bone metastases. C-index, ROC curve, calibration plot, and decision curve analysis were used to evaluate the performance and clinical usefulness of the model. The consistency index, Harrell’s C-index, was used to evaluate the predictive performance of the nomogram. And a ROC curve was established to compare the performance of nomogram model with independent predictors. Further, the nomogram was subjected to bootstrapping validation with 1,000 replications, to calculate an adjusted C-index and a calibration curve was used to judge predictive consistency. Decision curve analysis was conducted to determine the clinical usefulness of the nomogram by quantifying the net benefits at different probability thresholds.
A total of 565 patients with TC were enrolled in this study, of whom 25 (4.21%) had bone metastases and 540 (95.79%) had no bone metastases at initial diagnosis. Patient clinical characteristics are detailed in Table 1. Age, ALP and HB were significantly different between the bone metastasis group and the non-bone metastasis group (P<0.05). The average age, ALP and HB in the bone metastasis group were 53 years, 119.68U/L and 101.23g/L, respectively. The average age, ALP and HB in the non-bone metastasis group were 43.37 years, 68.83U/L and 123.07g/L, respectively.
Table 1 Comparison of clinical characteristics between bone metastasis and non-bone metastasis groups.
Logistic regression analysis was used to screen parameters. Univariate logistic regression analysis showed that age, ALP and HB were significantly correlated with bone metastasis of TC (p<0.05). Then the three related variables were included in the multivariate logistic regression analysis, and the results showed that age, ALP and HB were independent risk factors of bone metastasis of TC (P<0.05), so these three variables were used to construct the nomogram model (Table 2).
Table 2 Univariate analysis and multivariate analysis for prediction factors of bone metastasis in patients with thyroid cancer.
The results of logistic regression analysis including age, ALP, and HB are presented in Table 2. A model that incorporated the above independent predictors was developed and presented as a nomogram (Figure 2). For patients with TC, the total points calculated using the nomogram could be visually converted to the risk of bone metastases. However, the nomogram can become more cumbersome to read and use when there are higher order interaction and smoothers items in the model. To better address this issue, we established a dynamic nomogram based on shiny to simplify the operation of users (Figure 3) (18). The dynamic nomogram can be used to predict the probability of bone metastasis (and corresponding 95% Confidence interval) for any combination of predictor values. The shiny tabs display the predicted values graphically and numerically, and the model summary presents the underlying information of the model. Everyone can simply use the dynamic nomogram by clicking on the hyperlink (https://liuwencai.shinyapps.io/thyroid/).
Figure 2 Nomogram for prediction of bone metastases. Bone metastases prediction nomogram, developed based on patient age, HB, and ALP levels.
Figure 3 The dynamic nomogram for prediction of bone metastases. The plot displays probability (with 95% confidence interval) of bone metastases for patients with TC. The actual explanatory values and their corresponding predictions are given in the “Numerical Summary” tab.
The C-index for the prediction nomogram was 0.825, and bootstrapping validation confirmed a C-index of 0.815, indicating that the model has good discriminatory power. The ROC curve showed that nomogram had good predictive accuracy, which was higher than that of single independent predictor (Figure 4). The calibration curve of the nomogram for predicting of bone metastases in patients with TC demonstrated good agreement (Figure 5). The apparent performance of the nomogram showed good prediction capability.
Figure 5 Calibration curves for the bone metastasis prediction nomogram. The x-axis represents the predicted bone metastasis risk. The y-axis represents actual diagnoses of bone metastases. The diagonal dotted line represents a perfect prediction by an ideal model. The solid line represents the performance of the nomogram, where a closer fit to the diagonal dotted line represents a better prediction.
Decision curve analysis for the nomogram is presented in Figure 6. It showed that if the threshold probability of a patient or doctor is > 1% and < 67%, respectively, using the nomogram developed in the current study to predict bone metastasis risk added more benefit than either the intervention-all-patients or intervention-none scheme. Within this range, the net benefit based on the nomogram was comparable, with several overlaps.
Figure 6 Decision curve analysis for the bone metastasis prediction nomogram. The dotted line represents the bone metastasis risk nomogram. The thin solid line represents the assumption that all patients have bone metastases. The thick solid line represents the assumption that no patients have bone metastases. The y-axis measures the net benefit. The net benefit was calculated by subtracting the proportion of all patients who are false positive from the proportion who are true positive, weighting by the relative harm of forgoing treatment compared with the negative consequences of an unnecessary treatment. Here, the relative harm was calculated by pt/(1-pt). “pt” (threshold probability) is where the expected benefit of treatment is equal to the expected benefit of avoiding treatment; at which time a patient will opt for treatment informs us of how a patient weighs the relative harms of false-positive results and false-negative results ([a - c]/[b -d] = [1 - pt]/pt); (a–c) is the harm from a false-negative result; (b–d) is the harm from a false positive result. a, b, c and d give, respectively, the value of true positive, false positive, false negative, and true negative (19). The decision curve showed that if the threshold probability of a patient or doctor is > 1% and < 67%, respectively, using the nomogram in the current study to predict bone metastases adds more benefit than the intervention-all-patients or intervention-none schemes. For example, if the personal threshold probability of a patient is 20% (ie, the patient would opt for treatment if his probability of bone metastasis was 20%), then the net benefit is 0.151 when using the nomogram to make the decision of whether to undergo treatment, with added benefit than the intervention-all-patients or intervention-none schemes. The net benefit was comparable, with several overlaps, on the basis of the nomogram.
In previous studies, there were many studies related to bone metastasis of TC. Orita Y et al. observed bone metastases in 52 (3.7%) of 1398 patients with DTC (20). Choksi et al. studied the incidence of bone related events in thyroid cancer and found that the incidence of bone metastasis in TP was 3.9% (21, 22). Here, we found that the probability of bone metastasis in thyroid cancer was 4.21%, which was similar to previous studies. In general, the probability of bone metastasis of TC is not high, but once bone metastasis occurs, its complicated bone pain, spinal cord compression and pathological fracture will seriously affect the quality of life of patients. Bone scintigraphy is usually used to identify possible bone metastases in patients newly diagnosed with TC. However, the American Society of Clinical Oncology has reported the overuse and associated costs of BS in patients with extremely low risk of metastasis (23, 24). In recent years, many scholars have used artificial intelligence and machine learning techniques to predict cancer metastasis (25–27), and although they have better performance, they are slightly lacking in interpretability due to the black box characteristics of complex algorithms. Currently, nomograms are widely used as prognostic devices in oncology and medicine; these instruments employ user-friendly digital interfaces to increase accuracy, and provide easily understood prognoses, which facilitates better clinical decision making (17, 28). Therefore, based on the clinical data of 565 thyroid cancer patients, we identified independent risk factors for bone metastasis and constructed a nomogram model to predict the risk of BM in patients with newly diagnosed TC.
In the present study, we found three independent risk factors associated with BM, including age, ALP and HB. More importantly, based on the three variables, we developed and validated a practical dynamic nomogram for assessing the risk of bone metastases in newly diagnosed TC patients. Incorporating demographic and clinicopathological feature risk factors into an easy-to-use dynamic nomogram could facilitate prediction of bone metastases. Internal validation in the patient cohort demonstrated good discrimination and calibration of the model. The high C-index indicated that the nomogram model has high accuracy and can be widely used (28). Overall, the present study provides an accurate prediction tool for bone metastases in patients with TC.
In previous studies, age has been demonstrated to influence the prognosis of patients with TC (29–32). Further, age has been reported as a risk factor for bone metastases in patients with lung and breast cancer, and younger patients more prone to bone metastasis (33–35). However, few studies have confirmed an association between age and bone metastases in TC. In this study, we found a statistical correlation between age and bone metastases in patients with TC, and the risk of bone metastases increased with age. This may be related to genetic variation in TC cells in patients at different ages (36).
Several studies have reported that a shortage of red blood cells containing HB was a common complication in cancer patients, affecting more than 50% of cancer patients (37). Kawai et al. (38) identified HB levels is related to bone metastases associated with prostate cancer, while Henke et al. (39) reported that HB level is a significant prognostic factor in breast cancer metastases. Moreover, Chen et al. (40, 41) found the HB is an independent risk factor for bone metastases of breast and renal cell cancer. In this study, HB levels were significantly lower in patients with TC who had bone metastases than those without, which could predict the probability of bone metastasis of TC. The reason why patients with low HB levels are more prone to bone metastases may be that low HB concentrations can promote tumor cell adherence to bone marrow, thereby promoting bone metastases (38).
As a bone formation marker, total serum ALP was widely used for assessment of bone metastases in breast and prostate cancers, and can effectively reflect osteogenic activity in human patients (42, 43). Sun et al. (44) reported that bone ALP was a surrogate marker of bone metastasis in patients with gastric cancer, while Chen et al. (40) showed that ALP was a risk factor for bone metastases in breast cancer, and Huang et al. (45) reported that ALP was also a risk factor for bone metastases in bladder cancer. Rao et al. (46) showed that, tumor-derived ALP regulated epithelial plasticity, tumor growth, and disease-free survival in patients with metastatic prostate cancer. In this study, both bone-specific ALP and total ALP levels differed significantly between patients with and without bone metastases, where patients with TC and high levels of ALP were more likely to develop bone metastases.
In the analysis of risk factors, age, HB, and ALP were associated with bone metastases in patients with TC. And our nomogram suggested that advanced age, lower HB, and higher ALP may be the key individual factors that determine risk of bone metastases for patients with TC. In the model, we considered the weight of each predictor according to the statistical coefficient, and finally obtained a visual prediction chart, which could combine age, HB and ALP to help predict the probability of bone metastasis in TC patients. Further, compared with the traditional nomogram, the dynamic nomogram could easily calculate the incidence of bone metastases in patients with TC for any chosen set of values of the explanatory variables when there were higher-order interaction terms and smoother in the model. Therefore, this dynamic nomogram can be used to evaluate the risk of bone metastasis of TC simply, conveniently and quickly, and assist clinicians to make accurate clinical decisions.
However, there are some limitations in this study. Firstly it was based on retrospective data from a single institution, which will inevitably lead to inherent data bias. Thus, prospective and multi-center studies are required to validate our model. Secondly, the consistency of our nomogram was tested robustly using internal validation by bootstrap testing, but external validation is needed. Therefore, we will continue to collect cases to further validate the model, and we hope that other researchers could modify the prediction model with external data. Thirdly, clinical markers that may affect bone metastasis of cancer, including BRAF mutation, calcitonin, TERT mutation, and Ki-67 index, were not included in this study (9, 47, 48), and we will continue to expand our data collection to further improve our model in the future.
In conclusion, here we identified age, ALP, and HB as independent factors of bone metastases in patients with TC. Based on this information, we developed a practical dynamic nomogram, with a relatively good accuracy, to help clinicians predict the risk of bone metastases in patients with TC. The ability to estimate of individual risk provides clinicians and patients with more power to make decisions regarding medical interventions. Nevertheless, the nomogram requires external validation, and further research is needed.
The raw data supporting the conclusions of this article will be made available by the authors, without undue reservation.
The studies involving human participants were reviewed and approved by the Ethics Committee of the First Affiliated Hospital of Nanchang University, and all participants signed written informed consent form. The patients/participants provided their written informed consent to participate in this study.
W-CL conceived of and designed the study. W-CL, M-PL, W-YH, Y-XZ, and B-LS performed analysis and generated the figures and tables. W-CL and M-PL wrote the manuscript and S-HH, Z-LL, and J-ML critically reviewed the manuscript. All authors contributed to the article and approved the submitted version.
This work is supported by the central government guides local funds for scientific and technological development (No. 20222ZDH04095); Jiangxi Province “Double Thousand Plan” Talent Project.
The authors declare that the research was conducted in the absence of any commercial or financial relationships that could be construed as a potential conflict of interest.
All claims expressed in this article are solely those of the authors and do not necessarily represent those of their affiliated organizations, or those of the publisher, the editors and the reviewers. Any product that may be evaluated in this article, or claim that may be made by its manufacturer, is not guaranteed or endorsed by the publisher.
1. Roodman GD. Mechanisms of bone metastasis. New Engl J Med (2004) 350(16):1655–64. doi: 10.1056/NEJMra030831
2. Muresan M, Olivier P, Leclere J, Sirveaux F, Brunaud L, Klein M, et al. Bone metastases from differentiated thyroid carcinoma. Endocrine-related canc (2008) 15(1):37. doi: 10.1677/ERC-07-0229
4. Davies L, Welch HG. Increasing incidence of thyroid cancer in the united states, 1973-2002. Jama (2006) 295(18):2164–7. doi: 10.1001/jama.295.18.2164
5. Lim H, Devesa SS, Sosa JA, Check D, Kitahara CM. Trends in thyroid cancer incidence and mortality in the united states, 1974-2013. Jama (2017) 317(13):1338–48. doi: 10.1001/jama.2017.2719
6. Ramadan S, Ugas MA, Berwick RJ, Notay M, Cho H, Jerjes W, et al. Spinal metastasis in thyroid cancer. Head Neck Oncol (2012) 4(1):1–19. doi: 10.1186/1758-3284-4-39
7. Runowicz CD, Leach CR, Henry NL, Henry KS, Mackey HT, Cowens-Alvarado RL, et al. American Cancer society/American society of clinical oncology breast cancer survivorship care guideline. CA: Cancer J Clin (2016) 66(1):43–73. doi: 10.3322/caac.21319
8. Eustatia-Rutten C, Romijn J, Guijt M, Vielvoye G, Van den Berg R, Corssmit E, et al. Outcome of palliative embolization of bone metastases in differentiated thyroid carcinoma. J Clin Endocrinol Metab (2003) 88(7):3184–9. doi: 10.1210/jc.2003-030231
9. Fornetti J, Welm AL, Stewart SA. Understanding the bone in cancer metastasis. J Bone mineral Res: Off J Am Soc Bone Mineral Res (2018) 33(12):2099–113. doi: 10.1002/jbmr.3618
10. Durante C, Haddy N, Baudin E, Leboulleux S, Hartl D, Travagli J, et al. Long-term outcome of 444 patients with distant metastases from papillary and follicular thyroid carcinoma: benefits and limits of radioiodine therapy. J Clin Endocrinol Metab (2006) 91(8):2892–9. doi: 10.1210/jc.2005-2838
11. Wexler JA. Approach to the thyroid cancer patient with bone metastases. J Clin Endocrinol Metab (2011) 96(8):2296–307. doi: 10.1210/jc.2010-1996
12. Tsuzuki S, Park SH, Eber MR, Peters CM, Shiozawa Y. Skeletal complications in cancer patients with bone metastases. Int J Urol (2016) 23(10):825–32. doi: 10.1111/iju.13170
13. Bhatia R, Ravulapati S, Befeler A, Dombrowski J, Gadani S, Poddar N. Hepatocellular carcinoma with bone metastases: Incidence, prognostic significance, and management-Single-Center experience. J Gastrointest Canc (2017) 48(4):321–5. doi: 10.1007/s12029-017-9998-6
14. Makino H, Nishio S, Tsubamoto H, Shimada M, Nishikawa R, Kai K, et al. Treatment and prognosis of bone metastasis from cervical cancer (KCOG-G1202s). J Obstet Gynaecol Res (2016) 42(6):701–6. doi: 10.1111/jog.12956
15. Harel R, Angelov L. Spine metastases: current treatments and future directions. Eur J Canc (2010) 46(15):2696–707. doi: 10.1016/j.ejca.2010.04.025
16. Wood SL, Brown JE. Skeletal metastasis in renal cell carcinoma: current and future management options. Cancer Treat Rev (2012) 38(4):284–91. doi: 10.1016/j.ctrv.2011.06.011
17. Iasonos A, Schrag D, Raj GV, Panageas KS. How to build and interpret a nomogram for cancer prognosis. J Clin Oncol: Off J Am Soc Clin Oncol (2008) 26(8):1364–70. doi: 10.1200/JCO.2007.12.9791
18. Jalali A, Alvarez-Iglesias A, Roshan D, Newell J. Visualising statistical models using dynamic nomograms. PloS One (2019) 14(11):e0225253–e. doi: 10.1371/journal.pone.0225253
19. Vickers AJ, Cronin AM, Elkin EB, Gonen M. Extensions to decision curve analysis, a novel method for evaluating diagnostic tests, prediction models and molecular markers. BMC Med Inf Dec Mak (2008) 8:1–17. doi: 10.1186/1472-6947-8-53
20. Orita Y, Sugitani I, Matsuura M, Ushijima M, Tsukahara K, Fujimoto Y, et al. Prognostic factors and the therapeutic strategy for patients with bone metastasis from differentiated thyroid carcinoma. Surgery (2010) 147(3):424–31. doi: 10.1016/j.surg.2009.10.009
21. Choksi P, Papaleontiou M, Guo C, Worden F, Banerjee M, Haymart M. Skeletal complications and mortality in thyroid cancer: A population-based study. J Clin Endocrinol Metab (2017) 102(4):1254–60. doi: 10.1210/jc.2016-3906
22. Liu WC, Li ZQ, Luo ZW, Liao WJ, Liu ZL, Liu JM. Machine learning for the prediction of bone metastasis in patients with newly diagnosed thyroid cancer. Cancer Med (2021) 10(8):2802–11. doi: 10.1002/cam4.3776
23. Schnipper LE, Smith TJ, Raghavan D, Blayney DW, Ganz PA, Mulvey TM, et al. American Society of clinical oncology identifies five key opportunities to improve care and reduce costs: the top five list for oncology. J Clin Oncol: Off J Am Soc Clin Oncol (2012) 30(14):1715–24. doi: 10.1200/JCO.2012.42.8375
24. Schnipper LE, Lyman GH, Blayney DW, Hoverman JR, Raghavan D, Wollins DS, et al. American Society of clinical oncology 2013 top five list in oncology. J Clin Oncol: Off J Am Soc Clin Oncol (2013) 31(34):4362–70. doi: 10.1200/JCO.2013.53.3943
25. Liu W-C, Li M-X, Qian W-X, Luo Z-W, Liao W-J, Liu Z-L, et al. Application of machine learning techniques to predict bone metastasis in patients with prostate cancer. Cancer Manage Res (2021) 13:8723. doi: 10.2147/CMAR.S330591
26. Li W, Zhou Q, Liu W, Xu C, Tang ZR, Dong S, et al. A machine learning-based predictive model for predicting lymph node metastasis in patients with ewing’s sarcoma. Front Med (Lausanne). (2022) 9:832108. doi: 10.3389/fmed.2022.832108
27. Li W, Dong Y, Liu W, Tang Z, Sun C, Lowe S, et al. A deep belief network-based clinical decision system for patients with osteosarcoma. Front Immunol (2022) 13:1003347. doi: 10.3389/fimmu.2022.1003347
28. Wei L, Champman S, Li X, Li X, Li S, Chen R, et al. Beliefs about medicines and non-adherence in patients with stroke, diabetes mellitus and rheumatoid arthritis: a cross-sectional study in China. BMJ Open (2017) 7(10):e017293. doi: 10.1136/bmjopen-2017-017293
29. Kazaure HS, Roman SA, Sosa JA. The impact of age on thyroid cancer staging. Curr Opin Endocrinol Diabet Obes (2018) 25(5):330–4. doi: 10.1097/MED.0000000000000430
30. Mazurat A, Torroni A, Hendrickson-Rebizant J, Benning H, Nason RW, Pathak KA. The age factor in survival of a population cohort of well-differentiated thyroid cancer. Endocr Connect. (2013) 2(3):154–60. doi: 10.1530/EC-13-0056
31. Kim SJ, Myong JP, Suh H, Lee KE, Youn YK. Optimal cutoff age for predicting mortality associated with differentiated thyroid cancer. PloS One (2015) 10(6):e0130848. doi: 10.1371/journal.pone.0130848
32. Nixon IJ, Wang LY, Migliacci JC, Eskander A, Campbell MJ, Aniss A, et al. An international multi-institutional validation of age 55 years as a cutoff for risk stratification in the AJCC/UICC staging system for well-differentiated thyroid cancer. Thyroid: Off J Am Thyroid Assoc (2016) 26(3):373–80. doi: 10.1089/thy.2015.0315
33. Purushotham A, Shamil E, Cariati M, Agbaje O, Muhidin A, Gillett C, et al. Age at diagnosis and distant metastasis in breast cancer–a surprising inverse relationship. Eur J canc (2014) 50(10):1697–705. doi: 10.1016/j.ejca.2014.04.002
34. Liu W, Lee M, Wu S-N, Tong W-L, Li A-A, Sun B-L, et al. Using machine learning methods to predict bone metastases in breast infiltrating ductal carcinoma patients. Front Public Health (2022) 10:922510. doi: 10.3389/fpubh.2022.922510
35. Li MP, Liu WC, Sun BL, Zhong NS, Liu ZL, Huang SH, et al. Prediction of bone metastasis in non-small cell lung cancer based on machine learning. Front Oncol (2022) 12:1054300. doi: 10.3389/fonc.2022.1054300
36. Kim SM, Kim SY, Park CS, Chang HS, Park KC. Impact of age-related genetic differences on the therapeutic outcome of papillary thyroid cancer. Cancers (2020) 12(2):448. doi: 10.3390/cancers12020448
37. Yazdani A, Dorri S, Atashi A, Shirafkan H, Zabolinezhad H. Bone metastasis prognostic factors in breast cancer. Breast Canc: Bas Clin Res (2019) 13:1178223419830978. doi: 10.1177/1178223419830978
38. Kawai N, Kunimatsu M, Tozawa K, Sasaki M, Kohri K. Human prostate cancer cells adhere specifically to hemoglobin: a possible role in bone-specific metastasis. Cancer letters. (2001) 171(2):201–7. doi: 10.1016/S0304-3835(01)00595-X
39. Henke M, Sindlinger F, Ikenberg H, Gerds T, Schumacher M. Blood hemoglobin level and treatment outcome of early breast cancer. Strahlentherapie und Onkologie: Organ der Deutschen Rontgengesellschaft [et al]. (2004) 180(1):45–51. doi: 10.1007/s00066-004-1123-7
40. Chen WZ, Shen JF, Zhou Y, Chen XY, Liu JM, Liu ZL. Clinical characteristics and risk factors for developing bone metastases in patients with breast cancer. Sci Rep (2017) 7(1):11325. doi: 10.1038/s41598-017-11700-4
41. Chen XY, Lan M, Zhou Y, Chen WZ, Hu D, Liu JM, et al. Risk factors for bone metastasis from renal cell cancer. J Bone Oncol (2017) 9:29–33. doi: 10.1016/j.jbo.2017.10.004
42. Bishop MC, Hardy JG, Taylor MC, Wastie ML, Lemberger RJ. Bone imaging and serum phosphatases in prostatic carcinoma. Br J Urol (1985) 57(3):317–24. doi: 10.1111/j.1464-410X.1985.tb06353.x
43. Du WX, Duan SF, Chen JJ, Huang JF, Yin LM, Tong PJ. Serum bone-specific alkaline phosphatase as a biomarker for osseous metastases in patients with malignant carcinomas: a systematic review and meta-analysis. J Cancer Res Ther (2014) 10 Suppl:C140–3. doi: 10.4103/0973-1482.145842
44. Lim SM, Kim YN, Park KH, Kang B, Chon HJ, Kim C, et al. Bone alkaline phosphatase as a surrogate marker of bone metastasis in gastric cancer patients. BMC canc (2016) 16:385. doi: 10.1186/s12885-016-2415-x
45. Huang P, Lan M, Peng AF, Yu QF, Chen WZ, Liu ZL, et al. Serum calcium, alkaline phosphotase and hemoglobin as risk factors for bone metastases in bladder cancer. PloS One (2017) 12(9):e0183835. doi: 10.1371/journal.pone.0183835
46. Rao SR, Snaith AE, Marino D, Cheng X, Lwin ST, Orriss IR, et al. Tumour-derived alkaline phosphatase regulates tumour growth, epithelial plasticity and disease-free survival in metastatic prostate cancer. Br J canc (2017) 116(2):227–36. doi: 10.1038/bjc.2016.402
47. Min Y, Liu X, Hu D, Chen H, Chen J, Xiang K, et al. Risk factors, prognostic factors, and nomogram for distant metastasis in breast cancer patients without lymph node metastasis. Front Endocrinol (2021) 12:771226. doi: 10.3389/fendo.2021.771226
Keywords: nomogram, prediction, bone metastasis, thyroid cancer, risk
Citation: Liu W-C, Li M-P, Hong W-Y, Zhong Y-X, Sun B-L, Huang S-H, Liu Z-L and Liu J-M (2023) A practical dynamic nomogram model for predicting bone metastasis in patients with thyroid cancer. Front. Endocrinol. 14:1142796. doi: 10.3389/fendo.2023.1142796
Received: 12 January 2023; Accepted: 17 February 2023;
Published: 06 March 2023.
Edited by:
Ruiqin Han, Chinese Academy of Medical Sciences, ChinaReviewed by:
Bo Zhang, Tianjin Medical University, ChinaCopyright © 2023 Liu, Li, Hong, Zhong, Sun, Huang, Liu and Liu. This is an open-access article distributed under the terms of the Creative Commons Attribution License (CC BY). The use, distribution or reproduction in other forums is permitted, provided the original author(s) and the copyright owner(s) are credited and that the original publication in this journal is cited, in accordance with accepted academic practice. No use, distribution or reproduction is permitted which does not comply with these terms.
*Correspondence: Jia-Ming Liu, bGl1amlhbWluZ2RyQGhvdG1haWwuY29t
†These authors have contributed equally to this work
Disclaimer: All claims expressed in this article are solely those of the authors and do not necessarily represent those of their affiliated organizations, or those of the publisher, the editors and the reviewers. Any product that may be evaluated in this article or claim that may be made by its manufacturer is not guaranteed or endorsed by the publisher.
Research integrity at Frontiers
Learn more about the work of our research integrity team to safeguard the quality of each article we publish.