- 1Graduate School, Hebei Medical University, Shijiazhuang, Hebei, China
- 2Department of Endocrinology, Hebei General Hospital, Shijiazhuang, Hebei, China
- 3Department of Rehabilitation, Hebei General Hospital, Shijiazhuang, Hebei, China
- 4Department of Internal Medicine, Hebei Medical University, Shijiazhuang, Hebei, China
Background: Non-fasting lipid assessment can help predict cardiovascular disease risks and is linked to multiple diseases, particularly diabetes. The significance of non-fasting lipid levels in routine screening and postprandial lipid tests for potential dyslipidemia has not been conclusively determined. Various new lipid-lowering strategies have been developed to improve non-fasting dyslipidemia. Therefore, analysis of scientific outputs over the past decade is essential to reveal trends, hotspots, and frontier areas for future research in this field.
Methods: The Science Citation Index Expanded in the Web of Science Core Collection database was searched for publications related to non-fasting lipid research from 2012 to 2022. The regional distributions, authors, disciplines, journals, references, and keywords of the studies were analyzed using the bibliometric software VOSviewer and CiteSpace.
Results: A total of 4160 articles and reviews that met the inclusion criteria were included in this study. The output trend was established to be stable and the number of citation indices has been persistently increasing. A total of 104 countries/regions, 4668 organizations, and 20782 authors were involved in this research area. In terms of country, the United States had the largest number of publications (979). The University of Copenhagen was the most productive institution, publishing 148 papers. Professor Børge G Nordestgaard has made the most significant contribution to this field. Nutrients was the most productive journal while the American Journal of Clinical Nutrition was the highest co-cited journal. Analysis of co-cited references indicated that lipid-lowering strategies, statin therapy, high-fat meals, insulin resistance, physical exercise, and fructose were hotspots. Analysis of co-cited keywords revealed that apolipoprotein B, especially apolipoprotein B48, is becoming a key research focus. The keywords “gut microbiota” and “meal timing” were the most extensively studied.
Conclusion: The causal relationship between non-fasting dyslipidemia and diseases is currently being explored and the standards for non-fasting or postprandial lipid assessment are continuously being updated. Among the hotspots, lipid-lowering strategies are a potential research direction. Apolipoprotein B48, gut microbiota, and chrononutrition are the research frontiers. This initial bibliometric analysis of non-fasting lipids will enable researchers to monitor swift transformations and recognize novel concepts for upcoming research.
1 Introduction
Lipid profile standard measurements include triglycerides (TG) and cholesterol. They also include lipoprotein (a), apolipoprotein B (Apo B), apolipoprotein A1 (Apo A1), lipoprotein subfractions, other apolipoproteins, and metabolomics phenotyping in expanded or additional lipid profiles (1). Guidelines and consensus statements from nearly ten years ago mandated that lipid profile samples be collected after a period of fasting. Study subjects were required to fast overnight for at least 8 h before blood samples were drawn. However, compared to fasting TG, non-fasting TG performed better in cardiovascular risk prediction (2). Evidence from prospective cohort studies based on large populations revealed that elevated non-fasting TG levels are independently associated with increased risks of myocardial infarctions, ischemic heart disease, death (3), total cardiovascular events (2), and ischemic stroke (4). Furthermore, blood sampling in the non-fasting state was convenient for both laboratory personnel and patients (5). In 2009, the Danish Society of Clinical Biochemistry was the first society to recommend the testing of lipid profiles in the non-fasting state to predict cardiovascular risk (6). Currently, the debate on fasting and non-fasting lipid measurements is ongoing. Societies, guidelines, and statements from the United States (7–9), Europe (10, 11), Canada (12), Brazil (13), and India (14) recommend routine screening via non-fasting lipid measurements.
The fasting state only occurs in a short period before breakfast, which does not reflect the real lipid concentration throughout a 24-hour day (5). Intestinal-derived lipoproteins, especially postprandial triglyceride-rich lipoproteins (TRL), can be assessed in blood samples after meals as supplementary to liver-origin lipoproteins in fasting samples. Among the lipid profiles, TG levels fluctuate with different meals and meal times. Immensely high TG levels interfere with estimation of LDL-C levels (15). Therefore, establishing optimal cut-offs for non-fasting lipid profiles is a major challenge in clinical practice. Postprandial TG concentration, which can be detected by an oral fat tolerance test (OFTT), is also a cardiometabolic risk factor (16). The OFTT standards have not been fully established (10).
Non-fasting lipid metabolism is not only associated with cardiovascular disease (CVD), but also with diabetes (17), metabolic syndromes (18), non-alcoholic fatty liver disease (19), and polycystic ovarian syndrome (20) among others. The pathophysiological processes associated with postprandial metabolic responses have a connection with insulin resistance (21), oxidative stress (22), endothelial dysfunctions (23), and gut microbiota (24) among others. Nutrition (25), physical exercise and medication (26) play an important role in prevention and treatment strategies. Given the high number of studies, it is important to summarize the worldwide status, trends, and major topics in non-fasting lipid research.
Bibliometrics is a cross-disciplinary technique applied to the quantitative and qualitative analysis of knowledge. It can reveal trends and hotspots by countries/regions, institutions, researchers, keywords, and references based on publications in a field. In this study, we used bibliometrics software to analyze the characteristics of emerging trends and directions for future research on non-fasting lipids.
2 Methods
2.1 Literature search and data collection
We searched the Science Citation Index Expanded 1900-present (SCI-E) of Web of Science Core Collection (WoSCC) from 1st January 2012 to 1st November 2022. This database contains impactful global academic publications, and the downloadable data information is suitable with the bibliometrics software. The search strategy was composed of the following topic words with MESH Unique ID and free words: (“Lipids (D008055)” OR “Hyperlipidemias (D006949)” OR “Triglycerides (D014280)” OR “Hypertriglyceridemia (D015228)” OR “Cholesterol (D002784)” OR “Hypercholesterolemia (D006937)”) AND (“Postprandial period (D019518)” OR “non-fasting” OR “nonfasting”). The articles and reviews written in the English language were included in this study. Data were collected as plain text files with full records and cited references. The data acquisition flow chart is shown in Figure 1.
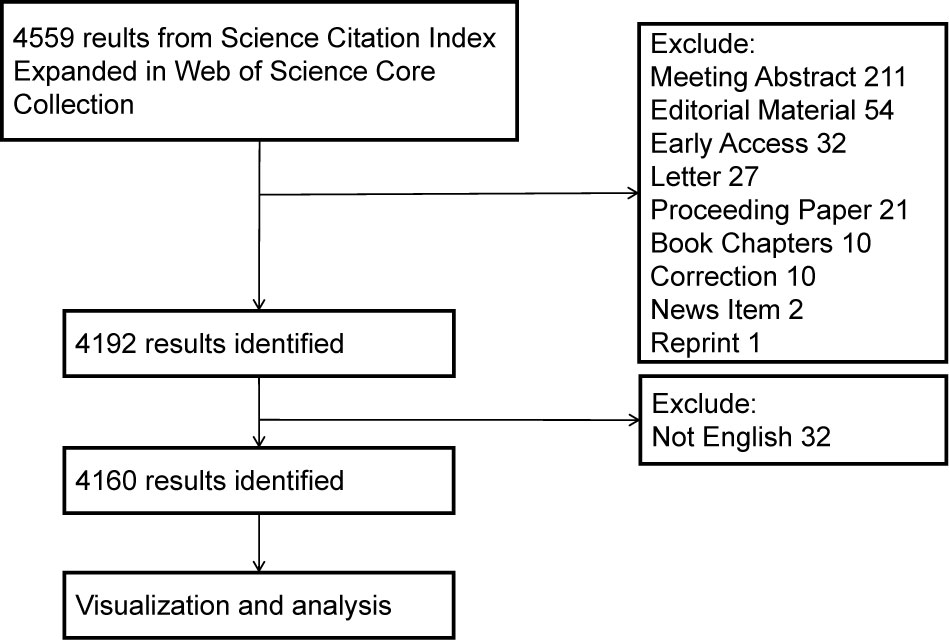
Figure 1 Retrieval and screening process flowchart. CiteSpace allows efficient evaluation of network visualization, while the VOSviewer provides clearer, user-friendly visualization.
2.2 Data analysis and visualization
VOSviewer (1.6.18 version), CiteSpace (6.1.R4 basic version) and Microsoft Excel (2019 version) were used for bibliometric analyses and visualization. VOSviewer is a computer program supported by the Centre of Science at Leiden University in the Netherlands. VOSviewer was utilized to conduct co-authorship analysis, co-occurrence analysis, and bibliographic coupling, followed by visualizing intellectual structure (27). CiteSpace, created by Chaomei Chen at Drexel University in the United States, is a Java-based application (28). Evolution analysis was performed to investigate the development process of the research based on an understanding of its structure using CiteSpace. VOSviewer and CiteSpace use colorful nodes and links to display the characteristics and connections using information extracted from publications. Node sizes refer to the occurrences of a trait. The color of the nodes and connections depends on the cluster. Different clusters are diversely colorized.
First, we summarized the annual publications and citations and built a graph using Microsoft Excel. Second, we used VOSviewer to create a knowledge map based on co-authorship of countries/regions and organizations. The global geographical distribution of publications was established on www.mapchart.net. Third, the networks of authors and co-cited authors were built by VOSviewer. Author productivity and impact are measured by the H-Index in Web of Science. The H-index is defined by the number of publications h, where each publication has at least h citations (29). Fourth, the research areas summary was downloaded from the literature search website. The dual-map overlay of journals was created by CiteSpace. Fifth, bibliographic coupling journals and co-cited journals visualization were achieved using VOSviewer. Sixth, analysis of co-cited references by CiteSpace provided structural metrics, such as betweenness centrality (BC), citation burst, and cluster mapping (30). Nodes with BC (> 0.10) represented significant connections between clusters. To identify emerging hotspots, burst detection was conducted for a specific period. Burst strength can reflect the influence of an article or a keyword over time. Cluster view of the co-citation network automatically acquired the cluster labels by using “All in One” function of CiteSpace. The Modularity Q value and Sihouette S value were important in interpreting the cluster network. The Q value range was [0, 1]. A clustering modularization was considered significant when the Q value exceeded 0.3. The cluster network was considered better with elevated Q value. The S value range was [-1, 1]. When the S value is close to 1, the cluster is isolated from others. When the S value surmounted 0.3, 0.5, or 0.7, the cluster network was considered homogenous, reasonable, and highly credible. Finally, author keywords for each publication were extracted to create a timeline view of cluster networks and show the strongest citation bursts using CiteSpace. The number of publications can provide a general estimate of the amount of work produced by a team or facility, while citation rates are commonly considered as measures of a paper’s quality, relevance, or interest, indicating the research’s impact. Bibliometric maps provide a great deal of detail. Exploring fields and comparing maps to users’ own expertise should enable users to access this underlying information. A cluster of research specialties is often identified. The map is used to visualize the relationship between countries and research fields (subfields). Co-citations provide a forward-looking assessment of document similarity. Citations can still vary over time due to changes in academic fields. A co-word analysis is used to identify a research field’s structure based on keyword co-occurrences in publications.
3 Results
3.1 Annual trend of publications and citations
The annual distribution of publications and citations is shown in Figure 2. Based on our search strategy, we identified 4,160 papers (3,599 articles and 561 reviews), with no duplicates. In the past decade, the number of outputs in the non-fasting lipid field stably fluctuated between 300 and 450 per year. The peak of publications was in 2021, with 439 papers. The H index of this field, as reported by WoSCC (SCIE), was 110 and the average citation per item was 22.09. A regression model [y = 87.958x2 + 920.95x - 609.32, R² = 0.9973] was adopted to fit the citation over time (2012–2021). 319 publications and 13576 citations from January to November 2022. The findings indicate that there is increasing attention being paid to the field of lipid research that is not limited to fasting conditions.
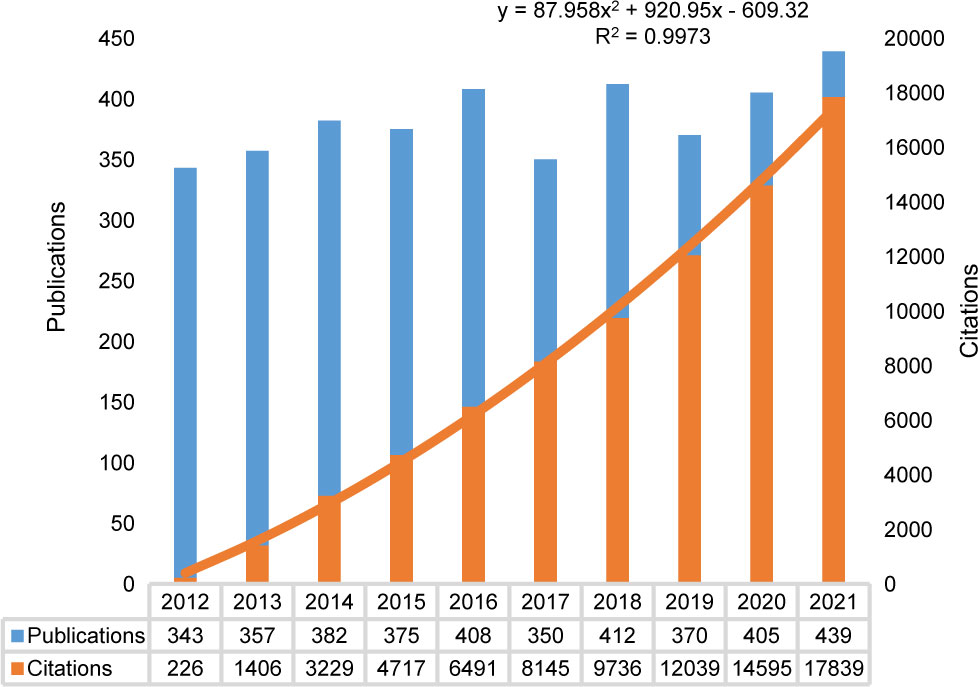
Figure 2 Annual trend of publications and citations. The orange line showed a regression model to illustrate citations per year (2012-2021). A total of 319 publications and 13576 citations were identified from January to November 2022.
3.2 Distribution of countries/regions and institutions
The obtained papers in the non-fasting lipid field were from 104 countries or regions. The top 10 countries/regions contributing to publications and citations were shown in Figure 3. The world map of publication distribution was shown in Supplementary Figure S1A. Almost half of the output was papers from the United States (979, 18.38%), China (608, 11.41%), the United Kingdom (432, 8.11%), Japan (301, 5.65%), and Canada (267, 5.01%). Publications from the United States (27957, 19.50%), the United Kingdom (12432, 8.67%), Denmark (9411, 6.56%), and China (8757, 6.11%) received the most citations. Regarding the average citation per item, the highest was Italy (30.49), followed by Canada (30.36), the United States (27.13), Netherlands (26.93), and Australia (26.8). Denmark, which had 193 outputs, ranked 11th among the productive countries and had 48.76 average citations per paper. Thirty countries published more than 30 papers. Co-authorship countries/regions network was as shown in Supplementary Figure S1B. Studies are active throughout countries in Western Europe, North America, Asia, Oceania, and South America. Cooperation among countries was found to be comprehensive (for instance, China collaborated actively with the United States, the United Kingdom, Canada, Denmark, and the Netherlands).
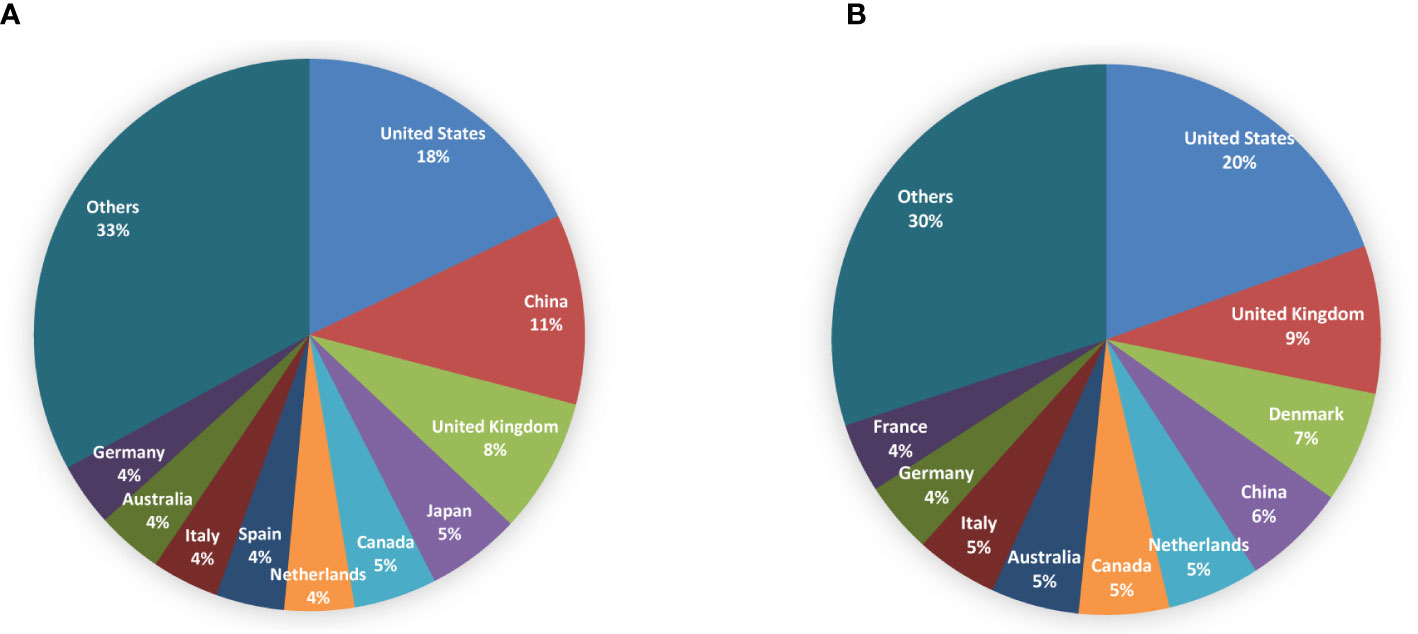
Figure 3 Pie charts for top 10 countries/regions contributing to publications (A) and citations (B) in the field of non-fasting lipid.
The analysis revealed 4668 organizations which were engaged in non-fasting lipid research. In Table 1, among the top 10 institutions, the University of Copenhagen ranked first in terms of the number of papers (148) and citations (8295). A total of 63 institutions published at least 20 papers in this field. The co-authorship institution network was as presented in Supplementary Figure S1C. European institutions, such as the University of Copenhagen, Maastricht University, and Instituto de Salud Carlos III had close cooperation with the University of Toronto and Harvard Medical School in North America, Shanghai Jiao Tong University and National University of Singapore in Asia, University of Western Australia in Oceania, as well as the University of São Paulo in South America.
3.3 Authors and co-cited authors
Globally, 20782 authors were involved in research on non-fasting lipid. The top 10 productive and co-cited authors, most of whom were from Europe, are shown in Table 2. Børge G Nordestgaard at the University of Copenhagen in Denmark ranked first with 43 publications. Out of the authors who published more than 10 papers in this particular field, a total of 89 authors were identified, and 58 of them had formed a collaboration network (Figure 4A). Børge G Nordestgaard closely cooperated with José López-Miranda, Pablo Perez-Martinez, and Marja-Riitta Taskinen among others. In Figure 4B, Børge G Nordestgaard was the highest co-cited author, with 1144 co-citations. Eighty-four authors were co-cited at least 100 times. Scott M Grundy, Jens Juul Holst, Børge G Nordestgaard, and Marja-Riitta Taskinen each had an H-index higher than 100, suggesting their contributions were significant.
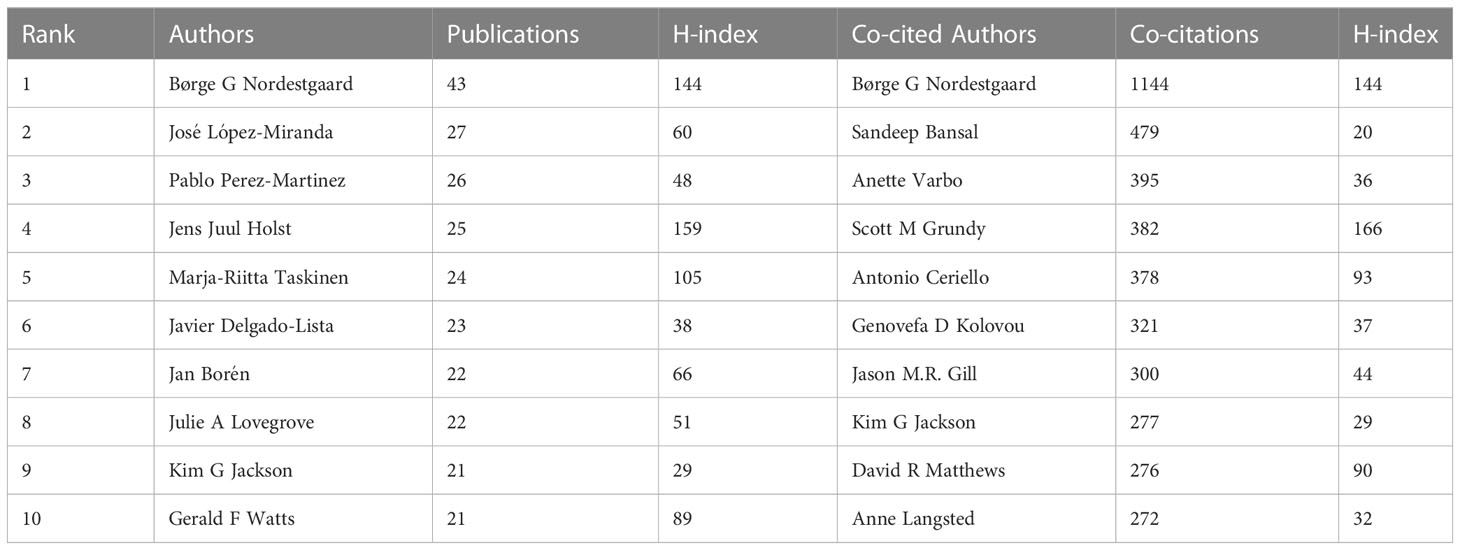
Table 2 Top 10 productive authors and co-citation authors in the research field of non-fasting lipid.
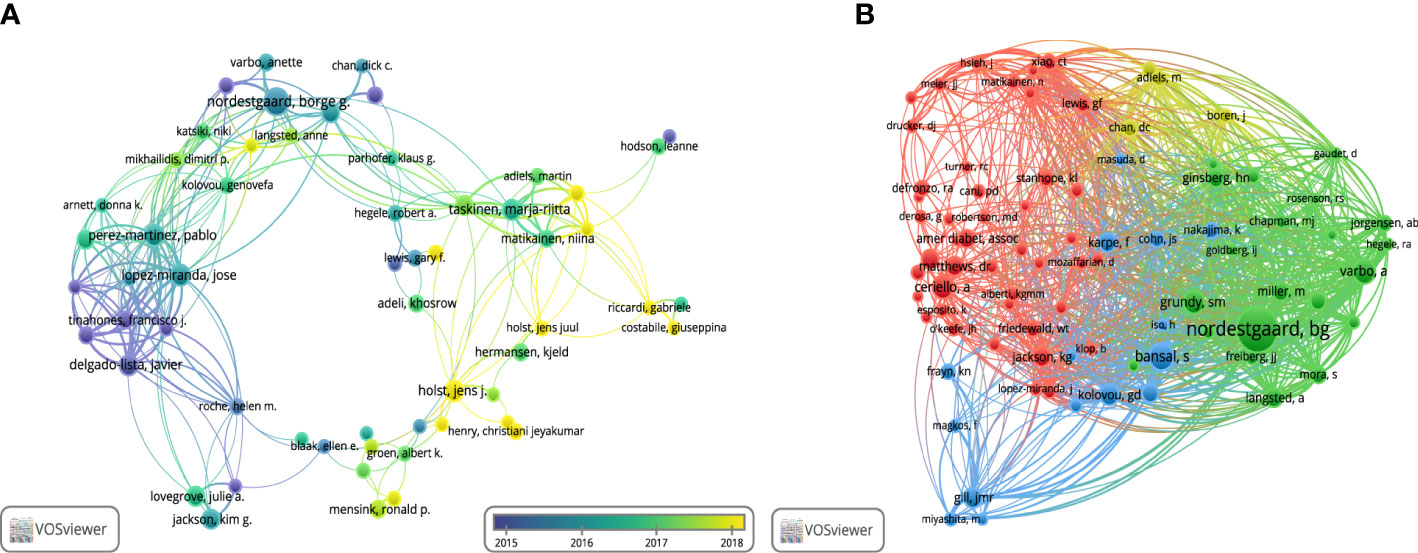
Figure 4 Visualization of authors. (A) Collaboration network of co-authors. (B) Network visualization of co-citation authors.
3.4 Distribution of disciplines
The top 10 disciplines of non-fasting lipid research in WoSCC (SCI-E) are categorized in Figure 5A. Most of the papers referred to the disciplines of Nutrition Dietetics, followed by Endocrinology Metabolism, Biochemistry Molecular Biology, Food Science Technology, and Pharmacology Pharmacy among others.
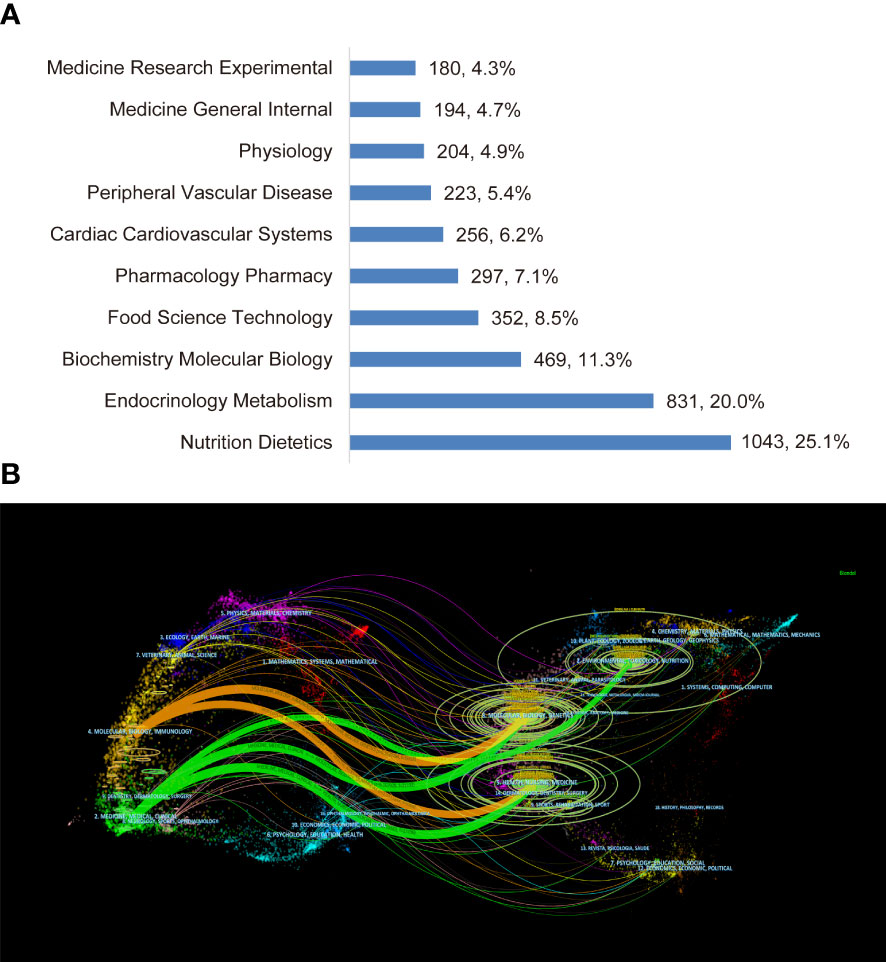
Figure 5 Visualization of disciplines. (A) The top 10 disciplines. (B) The dual-map overlay of journals.
In the dual-map overlay of journals (Figure 5B), major associations between disciplines of citing and cited journals are presented by the orange and green curves. The orange curve represents papers belonging to molecular/biology/genetics and health/nursing/medicine that are cited by molecular/biology/genetics. The green curve represents research output belonging to molecular/biology/genetics, health/nursing/medicine and environmental/toxicology/nutrition that are cited by medicine/medical/clinical researchers.
3.5 Journals and co-cited journals
Among the most productive journals, Nutrients (169, 4.1%) had the largest output, followed by Plos One (104, 2.5%), British Journal of Nutrition (84, 2%), American Journal of Clinical Nutrition (66, 1.6%), Journal of Clinical Endocrinology & Metabolism (59, 1.4%), and Journal of Nutrition (58, 1.4%) among others. Of note, 41 of the 940 journals published more than 20 papers in the non-fasting lipid field. The bibliographic coupling network of journals is shown in Figure 6A. The network of the co-cited journals is shown in Figure 6B. The American Journal of Clinical Nutrition, which was co-cited 6501 times, ranked first, followed by Diabetes Care (5272), Diabetes (4377), Circulation (4170), Journal of Lipid Research (3677), Atherosclerosis (3570), Journal of Clinical Endocrinology & Metabolism (3238), and Journal of Nutrition (3159). The New England Journal of Medicine and JAMA-Journal of the American Medical Association had the highest impact factors of 176.082 and 157.375, according to JCR 2021.
3.6 Co-cited references and references bursts
Co-cited references analysis was performed using CiteSpace, with setting parameters: timeline from 2012 to 2022, 1 year per slice, node type of references, and selection criteria g index (k = 5). We established a 245-node 1204-link network (Supplementary Figure S2A). The nodes surrounded by purple circles had relatively high BC, and were considered key turning points in the non-fasting lipid research field. Guidelines from European Atherosclerosis Society (EAS) about lipids (Chapman MJ, 2011; BC = 0.22) had the highest BC (31), followed by results from the Copenhagen City Heart Study (Nordestgaard BG, 2007; BC = 0.21) (3), a meta-analysis about diabetes and CVD (Sarwar N, 2010; BC = 0.12) (32), and a clinical trial about fructose and fat (Stanhope KL, 2009; BC = 0.11) (33).
We used the “All in One” button to automatically cluster and label the co-cited references. The network, consisting of 7 major clusters (Supplementary Figure S2B), was significant with a Q value of 0.472, and highly reliable with an S value of 0.832. From cluster #0 to #6, the number of articles in each cluster declined. The largest cluster #0 lipid-lowering strategies (n = 41, S = 0.814), followed by cluster #1 statin-treated patient (n = 40, S = 0.804), cluster #2 high-fat meal (n = 40, S = 0.67), cluster #3 metabolic response (n = 24, S = 0.901), cluster #4 insulin-resistant state (n = 23, S = 0.941), cluster #5 prior exercise (n = 19, S = 0.977), and cluster #6 syrup-sweetened beverage (n = 17, S = 0.914). The annual transformation of co-cited references is presented in Figure 7.
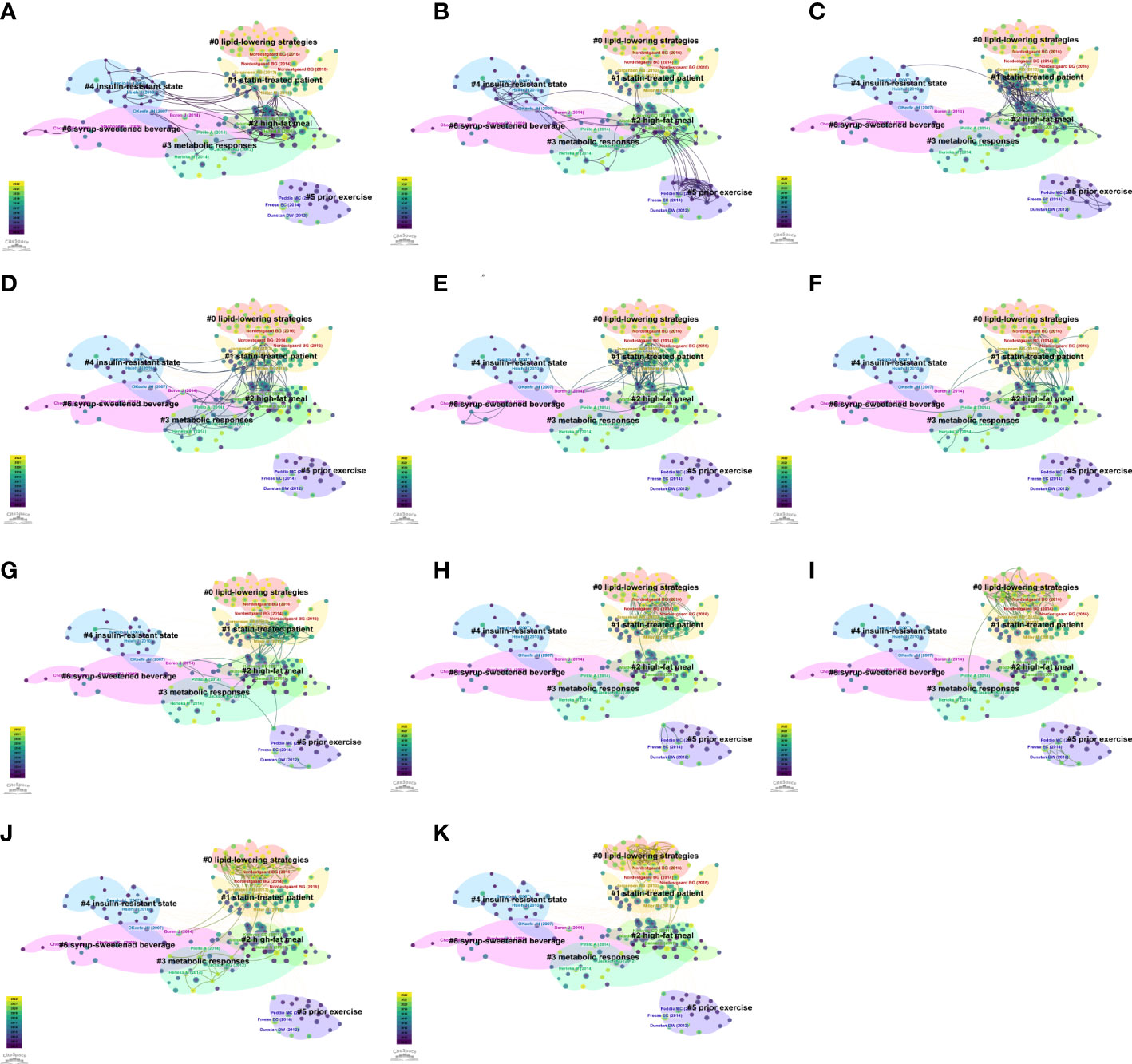
Figure 7 The annual trends of co-citation references network. (A) 2012. (B) 2013. (C) 2014. (D) 2015. (E) 2016. (F) 2017. (G) 2018. (H) 2019. (I) 2020. (J) 2021. (K) 2022.
The burst detection function was run to screen citation bursts (Supplementary Figure S2C). The articles with the most powerful burst strengths were two prospective follow-up studies published in JAMA-Journal of the American Medical Association in 2007. One with a burst of 69.82 has been mentioned above (Nordestgaard BG, 2007) (3), the other with a burst of 60.04 reported on the association between non-fasting TG levels and cardiovascular events (Bansal S, 2007) (2). Review of TRL and a statement about determination of lipid profiles in non-fasting state (Nordestgaard BG, 2016) (34, 35) have received continuous attention from 2017 to 2022. Recent studies by Bhatt DL et al. (36), Ference BA et al. (37), Anderson TJ et al. (12), Mach F et al. (15), Ference BA et al. (38), Langsted A et al. (5), and Grundy SM et al. (7) have achieved significance.
3.7 Keyword evolution and bursts
Author keywords reflect the most crucial information in articles. These keywords were analyzed using CiteSpace with parameters; from 2012 to 2022, 1 year per slice, node types of keywords, with the selection criteria as the top 50 per slice. The “All in One” function was used to automatically recognize the clusters and labels. We established a 279-node 1283-link cluster network in Supplementary Figure S3A. The timeline view of author keywords revealed the evolution of research (Figure 8). Duplicates of keywords such as “triglycerides” and “triglyceride” were merged by thesaurus. Clusters were significant, with a Q value of 0.3263, and reasonable with an S value of 0.6561. The cluster label was the most important keyword for each cluster. The most outstanding cluster was cluster #0 apolipoprotein B, followed by cluster #1 type 2 diabetes, cluster #2 insulin resistance, cluster #3 cardiovascular disease, cluster #4 physical activity, cluster #5 fatty acids, and cluster #6 lipid metabolism. Among the nodes of clusters, postprandial lipemia (n = 176, BC = 0.17, #0), type 2 diabetes (n = 358, BC = 0.47, #1), insulin resistance (n = 188, BC = 0.13, #2), metabolic syndrome (n = 152, BC = 0.12, #2), cardiovascular disease (n = 248, BC = 0.37, #3), lipid metabolism (n = 94, BC = 0.12, #6) acted as joints between clusters with high frequencies of co-citation and BC. Oxidative stress (n = 98, #4) and fatty acid (n = 56, #5) have also been exhaustively investigated. Apo B48 (n = 27, #0), one of Apo B transcripts, has particularly become important in research.
The top 20 keywords with citation bursts are shown in Supplementary Figure S3B and are also highlighted with red nodes in Figure 8. The keyword with the strongest citation burst was “systematic review” (strength = 5.82), followed by “gut microbiota” (strength = 5.44), and “oral fat tolerance test” (strength = 5.34). These were potential topics that were associated with transformative discoveries. The keywords, “remnant cholesterol”, “blood glucose”, “gut microbiota”, “systematic review”, “lipid profile”, “weight loss”, and “meal timing”, showed strong citation bursts until 2022.
4 Discussion
4.1 General information
A scientometric analysis and visualization were performed using studies on non-fasting lipid published from 2012 to 2022. The number of publications maintained a steady increase, with rapid citations. This implies that the non-fasting lipid research field has a bright future. Studies in this field were found to be relatively mature in the United States and the United Kingdom, with both productive publications and high citations per document. Although Denmark was the 11th most productive country, the average citation times for each document was the highest, indicating that Danish papers are of a higher quality. In terms of publications, the outputs are burgeoning and booming in China, however, the citations per document are relatively low, suggesting that there is still a long way for Chinese researchers. There were strong collaborations among countries/regions regarding research in this field. Globally, the University of Copenhagen in Denmark is the most influential center in this field, and exhibited the most active connections with other institutions. Professor Børge G Nordestgaard, who is affiliated to the University of Copenhagen, made the most outstanding contribution to this field. The dual-map overlaps and subject category list suggest this is a multidisciplinary area. Clinical medicine and its sub-groups, nutrition and food science, biochemistry and molecular biology complement each other in this field. In terms of journals, Nutrients is the most popular journal, with the largest number of publications on non-fasting lipid. Among the most co-citation journals, American Journal of Clinical Nutrition ranked the first.
4.2 Emerging trends, hotspots, and frontiers
Co-citation reference networks reveal the research structure in the non-fasting lipid field. If a reference is co-cited by numerous publications, it is considered a basis in the field. The top 20 references with the strongest bursts and cluster transformation illuminated the trends of the field. Among the co-citation references, four papers had a high BC, indicating that potential structural variations or revolutionary points may occur at these nodes (3, 31–33). Through analysis of context in references, we concluded that cluster labels in Figure 7 from #0 to #6 represent the following topics: #0 lipid-lowering strategies, #1 statin therapy, #2 high-fat meal, #3 metabolic response, #4 insulin resistance, #5 physical exercise, #6 fructose. The timeline view of co-cited keywords shows that hotspots in the non-fasting lipid area varied over time. Cluster analysis of keywords revealed the hot topics. Keywords with the strongest bursts represent alterations of frontiers. The #0 cluster label of co-occurrence keywords was Apo B, indicating that research related to Apo B recently received the most interest. Based on occurrence time, burst strength and maintenance period, “oral fat tolerance test”, “gut microbiota”, and “meal timing” keywords were the most noteworthy topics.
The co-cited references and keyword networks showed that research focus in this field has shifted from observational studies recognizing hazards of non-fasting lipid disorders in diverse diseases to investigating management methods and implementation of preventive strategies. Causal relationships among non-fasting dyslipidemia, CVD, and type 2 diabetes are still under investigation. The major aspects of management approaches include non-fasting lipid routine test and OFTT to identify potential postprandial dyslipidemia. Prevention strategies include lipid-lowering medications, lipid modification by anti-diabetic medications, and lifestyle interventions, such as physical exercise and diet changes. The association between gut microbiota and non-fasting lipid are being investigated.
4.2.1 Observational evidence
Two prospective cohort studies published in 2007 are milestones in this field. One of them provided powerful evidence on the importance of non-fasting rather than fasting TG as the crucial risk factor for cardiovascular events (2). The other study, which showed that elevated non-fasting TG levels are associated with an increased risk of myocardial infarction, ischemic heart disease, and death acted as a junction for researches about #1 statin therapy, #2 high-fat meal, #3 metabolic response, #4 insulin resistance, and #6 fructose from 2012 to 2016 (3). Elevated non-fasting TG levels have also been shown to be a risk factor for ischemic stroke (4). However, due to the complexity of TG metabolism, it has not been established whether TG causes CVD. When TG levels are elevated, TRL, chylomicrons and very low-density lipoprotein (VLDL) contains more TG or increases in number of particles. In 2016, it was proven that elevated TRL, which cannot be fully reflected in a fasting sample, is a causal risk factor for inflammation, CVD and mortality (34). Recently, mendelian randomization analysis showed that suppressed Apo B levels reflect the benefits of reducing TG and LDL-C concentrations for a low risk of coronary heart disease, complied with genetic variants (38). Based on increasing evidence, routine Apo B assessments should be considered in clinical practice. A previous meta-analysis reporting that diabetes and fasting blood glucose are risk factors for various vascular diseases formed the bond in researches on #1 statin therapy, #2 high-fat meal, #3 metabolic responses, #4 insulin resistance, and #6 fructose from 2012 to 2017 (32). The research article reported that it was fructose and not glucose that induces insulin resistance and dyslipidemia, which led to researches on #6 fructose with #4 insulin-resistance and #3 metabolic response from 2015 to 2017 (33). Research heat on #6 fructose faded in 2018.
4.2.2 Screening strategies
In the past decade, global guidelines and consensus have emphasized non-fasting lipid management. According to the 2011 European Society of Cardiology (ESC)/EAS guidelines for dyslipidemia management, high-density lipoprotein cholesterol (HDL-C), TC, Apo B, and Apo A1 levels can be assayed in non-fasting samples, whereas TG levels and LDL-C, which is calculated by the Friedewald formula should be assessed in fasting samples (39). The 2011 EAS guidelines for patients who achieved the LDL-C goal but still had high cardiovascular risks recommend that elevated TG, TRL and remnant cholesterol, and/or low HDL-C levels require further clinical management. The 2011 EAS guidelines also acted as the most influential bridge among researches on #4 insulin resistance, #2 high-fat meals, and #2 statin therapy from 2012 to 2019 (31). The 2013 American College of Cardiology/American Heart Association guidelines for cholesterol reduction to prevent atherosclerotic cardiovascular risk provided the non-fasting non-HDL-C and TG cut-off points for reappraisal. However, the guidelines preferred fasting lipid assessments (40). In 2016, the EAS/European Federation of Clinical Chemistry and Laboratory Medicine joint consensus provided a recommendation that non-fasting blood samples be used for routine screening of lipid profiles. Among the lipid profiles, TG, TC, and calculated concentrations of LDL-C, remnant cholesterol, and non-HDL-C are mostly affected by non-fasting status, whereas concentrations of HDL-C, Apo A1, Apo B, and lipoprotein(a) are not affected. Thus, the guidelines provided the cut-off levels for situations where fasting lipids are required to complement routine measurements of non-fasting lipid (35). The Canadian guidelines for dyslipidemia management also recommended non-fasting lipid and lipoprotein examinations during routine screening (12). The 2019 ESC/EAS guidelines showed that in terms of screening for prognostic outcomes of cardiovascular risks, high TG levels of 0.3 mmol/L (27 mg/dL) in non-fasting samples had equivalent clinical significance to fasting samples (15). However, fasting samples are recommended for patients with metabolic syndromes, diabetes, severe dyslipidemia, and follow-up for patients with hypertriglyceridemia (15, 41).
4.2.3 Postprandial metabolic responses
Almost 40 years ago, Zilversmit hypothesized that atherogenesis might occur over a postprandial period when chylomicrons and/or remnants are elevated (42). Postprandial responses represent a typical non-fasting state (43). Based on available evidence, appropriate strategies should be developed to screen for and treat postprandial dysmetabolism to prevent CVD and diabetes (44). The OFTT is used to identify patients with postprandial lipidemia. However, this approach should be standardized. In 2011, experts established guidelines on non-fasting and postprandial TG levels, including classified concentrations, a practical project of OFTT, and treatment recommendations (16). However, evidence of elevated postprandial TG is not conclusive. In 2019, OFTT trials were summarized in a series of metabolic diseases and non-fasting TG is still considered as evidence for postprandial hypertriglyceridemia measurements (10). Implementable schemes and the benefits of fat overload have not been fully established. Metabolic responses of fat overload tests that had been adjusted to body weight versus standardized high-fat meals were compared in a randomized crossover study, suggesting that postprandial TG levels of healthy participants fluctuate in an acceptable scope with both interventions, supporting the feasibility of standardized meals in both scientific studies and clinical practice (45). Apo C3 reflects the risk of postprandial TG disorders (46). Instead of fat distribution, postprandial TG levels may reflect early fat metabolic disturbances (47). Chinese people with fasting TG levels in the range of 1.0 to 1.7 mmol/L may benefit most from OFTT to identify postprandial dyslipidemia (48). The triglyceride-insulin-glucose-glucagon-like peptide-1 model was built to assess glucose, insulin, and incretin responses following a high-fat meal (49). The OFTT can help patients identify insulin sensitivity impairments, insulin resistance, beta-cell dysfunctions and secretion disorders of gut hormones, including glucose-dependent insulinotropic polypeptides and glucagon-like peptide-1 (21, 50).
Apo B is considered to be an indicator of atherogenic lipoproteins. Particularly, Apo B48 is an emerging hotspot that is increasingly being studied. After a meal, TGs are packaged into chylomicrons particles and transported to various tissues. Apo B48 which is synthesized by the intestines is a unique biomarker of postprandial intestinal chylomicron metabolites, which indicates the absorption of TG (51). Observational studies have reported that elevated fasting Apo B48 remnants associated with fat intolerance can predict postprandial Apo B48 disorder and increase the risk of CVD in adolescents with obesity (52). Postprandial Apo B48 contributes to subclinical atherosclerosis in patients with rheumatoid arthritis (53). The intestinal FGF15/19-SHP-TFEB pathway is activated after a meal, which mediates lipophagy and regulates postprandial TG and chylomicron via Apo B48. This study revealed a novel target for postprandial dyslipidemia treatment (54). Statin therapy can prevent the production of Apo B48 in intestines and promote the catabolism of Apo B48 to reduce postprandial TG synthesis (55). Semaglutide can decrease the production of postprandial Apo B48 levels (56). T2DM patients treated with liraglutide showed decreased levels of Apo B48, swelling of chylomicrons, reduced chylomicrons and remnant formation (57). Diet composition can also affect Apo B48 levels. A comparison of two common saturated fat of mixed meals, higher palmitic-acid intake found lower Apo B48 levels than higher stearic-acid intake (58).
4.2.4 Medication regimens
Evidence from many studies has confirmed that LDL-C causes atherosclerotic cardiovascular disease (37). The guidelines show that statin therapy should be the preferred treatment to reduce LDL-C levels compared with other lipids-lowering strategies because it can prevent and improve the prognosis of cardiovascular events (7, 12, 15). Elevated TG cannot be underestimated, especially in a non-fasting state (59).The results of the IMPROVE-IT trial revealed that the combination of ezetimibe and statin therapy was more effective and did not cause adverse effects (60). In 2017, evolocumab combined with statin therapy was found to reduce the risk of cardiovascular events in the FOURIER trial (61). In 2019, the results of REDUCE-IT trial showed that icosapent ethyl decreased the risk of ischemic events among patients with hypertriglyceridemia receiving statin therapy (36). As shown in the co-citation reference networks from 2019 to 2022, #1 statin therapy was changed to #0 lipid-lowering strategies, suggesting that other drugs for dyslipidemia treatment are under investigation.
For the cluster #4 insulin resistance, the main research topic was the cardiovascular protective effects of anti-diabetic medications due to improved postprandial lipids metabolism, particularly, incretin-based drugs including dipeptidyl peptidase-4 inhibitors, glucagon-like peptide-1 receptor agonists (62, 63). Some of these medications have been proven to reduce TRL significantly (57, 64).
4.2.5 Lifestyle interventions
Lifestyle interventions included physical exercise and chrononutrition. Research on the application of #5 physical exercise to reduce postprandial lipidemia increased in the 2013-2014 and 2018-2020 period. From the cluster analysis of keywords, we found that oxidative stress was highly linked to physical exercise. Endurance exercise can have a beneficial impact on postprandial TG levels, however, it does not seem to affect markers of oxidative stress (65). Regular physical exercise even walking can improve postprandial lipidemia and blood glucose (66, 67). Aerobic exercise has similar effects with statin therapy in terms of reducing postprandial TG levels as reported in a previous meta-analysis (68). In 2017, scientists won the Nobel Prize for elucidating the biological clock. Since then, research on the circadian variation of lipids has experienced bursts into hotspots. Meal timing strongly affects postprandial lipid profiles (69). Nighttime food intake may also increase the risk of dyslipidemia and hence potential of CVD due to postprandial TG metabolic dysfunction (70). Feeding time restricted within eight hours for five days can increase the fat oxidation rate in both fasting and non-fasting state, but cannot affect postprandial lipemia (71).
4.2.6 Gut microbiota
Variation in intestinal microbiota composition and drugs targeted at gut microbiota have been investigated from animals to human beings (9). In animal studies, a high-fat/high-fructose diet causes gut microbial alteration in hamsters leading to metabolic syndrome (24). Extracts of fermented green tea were reported to reduce high-fat diet-induced hypertriglyceridemia in hamsters by decreasing the proportion of phylum Firmicutes in gut microbiota (72). In human studies, the PREMOTE trial showed that the combination of berberine and probiotic improved postprandial dyslipidemia among T2DM patients, providing a novel therapy targeting gut microbiota (73). Importantly, the PREDICT 1 trial built a machine-learning model to evaluate metabolic responses to food intake involving meal nutrients, meal timing, physical exercise, diurnal rhythm, and gut microbiota. This model can function as an algorithmic tool that allows for the accurate customization of nutritional strategies in the treatment of illnesses (25).
4.3 Advantages and limitations
This is the first bibliometric analysis to provide a credible account of the current state of research on non-fasting lipids. The findings of this study are expected to shed light on new directions and reveal ideas for further investigations. However, there are still some limitations. First, owing to the nature of the bibliometric software, we only analyzed data from the WoSCC (SCI-E) database. Second, only English-language papers were analyzed. Therefore, this study may not fully represent the entire spectrum of this field. Third, some new ideas may have been overlooked because the most recently published papers often have lower citations.
5 Conclusions
This bibliometric analysis of non-fasting lipid from 2012 to 2022 was conducted to visualize trends, hotspots and frontiers. The study of lipids in a non-fasting state is highly important in various fields including nutrition, endocrinology, cardiology, pharmacology, molecular biology, and sports medicine. In this field, researchers have switched from observing hazards of non-fasting dyslipidemia to elucidating the causal relationship of non-fasting dyslipidemia in diseases, especially cardiovascular diseases and diabetes. The guidelines and expert consensus are constantly being updated regarding the recommendations for non-fasting lipid assessment. The investigation of standardized OFTT to identify potential postprandial dyslipidemia is ongoing. The lipid-lowering strategies, statin therapy, high-fat meal, insulin resistance, physical exercise, and fructose are hotspots. Identification of optimal lipid-lowering strategies should be intensified with a focus on lipid-lowering drugs, lipid-reducing effects of anti-diabetic drugs (especially incretin-based drugs), physical exercise and nutrition therapies. Apo B48, gut microbiota, and chrononutrition are the frontiers in the research field of non-fasting lipid.
Data availability statement
The datasets presented in this study can be found in online repositories. The names of the repository/repositories and accession number(s) can be found in the article/Supplementary Material.
Author contributions
YH: Conceptualization, Methodology, Formal analysis, Data Curation, Visualization, Writing - Original Draft. ZA: Validation, Formal analysis, Data Curation. XH and YG: Validation, Supervision. GS: Conceptualization, Supervision, Writing - Review and Editing. All authors contributed to the article and approved the submitted version.
Funding
This work was supported by the National Natural Science Foundation of China (82170878).
Acknowledgments
The authors would like to thank all the reviewers who participated in the review and MJEditor (www.mjeditor.com) for its linguistic assistance during the preparation of this manuscript.
Conflict of interest
The authors declare that the research was conducted in the absence of any commercial or financial relationships that could be construed as a potential conflict of interest.
Publisher’s note
All claims expressed in this article are solely those of the authors and do not necessarily represent those of their affiliated organizations, or those of the publisher, the editors and the reviewers. Any product that may be evaluated in this article, or claim that may be made by its manufacturer, is not guaranteed or endorsed by the publisher.
Supplementary material
The Supplementary Material for this article can be found online at: https://www.frontiersin.org/articles/10.3389/fendo.2023.1136048/full#supplementary-material
References
1. Nordestgaard BG. A test in context: lipid profile, fasting versus nonfasting. J Am Coll Cardiol (2017) 70(13):1637–46. doi: 10.1016/j.jacc.2017.08.006
2. Bansal S, Buring JE, Rifai N, Mora S, Sacks FM, Ridker PM. Fasting compared with nonfasting triglycerides and risk of cardiovascular events in women. Jama (2007) 298(3):309–16. doi: 10.1001/jama.298.3.309
3. Nordestgaard BG, Benn M, Schnohr P, Tybjaerg-Hansen A. Nonfasting triglycerides and risk of myocardial infarction, ischemic heart disease, and death in men and women. Jama (2007) 298(3):299–308. doi: 10.1001/jama.298.3.299
4. Freiberg JJ, Tybjaerg-Hansen A, Jensen JS, Nordestgaard BG. Nonfasting triglycerides and risk of ischemic stroke in the general population. Jama (2008) 300(18):2142–52. doi: 10.1001/jama.2008.621
5. Langsted A, Nordestgaard BG. Nonfasting versus fasting lipid profile for cardiovascular risk prediction. Pathology (2019) 51(2):131–41. doi: 10.1016/j.pathol.2018.09.062
6. Nordestgaard BG, Hilsted L, Stender S. [Plasma lipids in non-fasting patients and signal values of laboratory results]. Ugeskr Laeger (2009) 171(13):1093.
7. Grundy SM, Stone NJ, Bailey AL, Beam C, Birtcher KK, Blumenthal RS, et al. 2018 AHA/ACC/AACVPR/AAPA/ABC/ACPM/ADA/AGS/APhA/ASPC/NLA/PCNA guideline on the management of blood cholesterol: a report of the American college of Cardiology/American heart association task force on clinical practice guidelines. J Am Coll Cardiol (2019) 73(24):e285–350. doi: 10.1016/j.jacc.2018.11.003
8. Virani SS, Morris PB, Agarwala A, Ballantyne CM, Birtcher KK, Kris-Etherton PM, et al. 2021 ACC expert consensus decision pathway on the management of ASCVD risk reduction in patients with persistent hypertriglyceridemia. J Am Coll Cardiol (2021) 78(9):960–93. doi: 10.1016/j.jacc.2021.06.011
9. Wilson PWF, Jacobson TA, Martin SS, Jackson EJ, Le NA, Davidson MH, et al. Lipid measurements in the management of cardiovascular diseases: practical recommendations a scientific statement from the national lipid association writing group. J Clin Lipidol (2021) 15(5):629–48. doi: 10.1016/j.jacl.2021.09.046
10. Kolovou GD, Watts GF, Mikhailidis DP, Pérez-Martínez P, Mora S, Bilianou H, et al. Postprandial hypertriglyceridaemia revisited in the era of non-fasting lipid profile testing: a 2019 expert panel statement, main text. Curr Vasc Pharmacol (2019) 17(5):498–514. doi: 10.2174/1570161117666190507110519
11. Nordestgaard BG, Langlois MR, Langsted A, Chapman MJ, Aakre KM, Baum H, et al. Quantifying atherogenic lipoproteins for lipid-lowering strategies: consensus-based recommendations from EAS and EFLM. Atherosclerosis (2020) 294:46–61. doi: 10.1016/j.atherosclerosis.2019.12.005
12. Anderson TJ, Grégoire J, Pearson GJ, Barry AR, Couture P, Dawes M, et al. 2016 Canadian Cardiovascular society guidelines for the management of dyslipidemia for the prevention of cardiovascular disease in the adult. Can J Cardiol (2016) 32(11):1263–82. doi: 10.1016/j.cjca.2016.07.510
13. Scartezini M, Ferreira CED, Izar MCO, Bertoluci M, Vencio S, Campana GA, et al. Positioning about the flexibility of fasting for lipid profiling. Arq Bras Cardiol (2017) 108(3):195–7. doi: 10.5935/abc.20170039
14. Saboo B, Agarwal S, Makkar BM, Chawla R, Ghosh S, Viswanathan V, et al. RSSDI consensus recommendations for dyslipidemia management in diabetes mellitus. Int Diabetes Dev Ctries (2022) 42(1):3–28. doi: 10.1007/s13410-022-01063-6
15. Mach F, Baigent C, Catapano AL, Koskinas KC, Casula M, Badimon L, et al. 2019 ESC/EAS guidelines for the management of dyslipidaemias: lipid modification to reduce cardiovascular risk. Eur Heart J (2020) 41(1):111–88. doi: 10.1093/eurheartj/ehz455
16. Kolovou GD, Mikhailidis DP, Kovar J, Lairon D, Nordestgaard BG, Ooi TC, et al. Assessment and clinical relevance of non-fasting and postprandial triglycerides: an expert panel statement. Curr Vasc Pharmacol (2011) 9(3):258–70. doi: 10.2174/157016111795495549
17. Lim S, Kim YJ, Khang AR, Eckel RH. Postprandial dyslipidemia after a standardized high-fat meal in BMI-matched healthy individuals, and in subjects with prediabetes or type 2 diabetes. Clin Nutr (2021) 40(11):5538–46. doi: 10.1016/j.clnu.2021.09.004
18. Ittermann T, Dorr M, Markus MRP, Nauck M, Jurgens C, Schipf S, et al. Variability of biomarkers used for the classification of metabolic syndrome: a repeated measurements study. Nutr Metab Carbiovasc Dis (2022) 32(7):1693–702. doi: 10.1016/j.numecd.2022.03.022
19. Hou XY, Guan YP, Tang Y, Song A, Zhao JJ, Ren LP, et al. A correlation study of the relationships between nonalcoholic fatty liver disease and serum triglyceride concentration after an oral fat tolerance test. Lipids Health Dis (2021) 20(1):12. doi: 10.1186/s12944-021-01483-z
20. Lewis RD, Narayanaswamy AK, Farewell D, Rees DA. Complement activation in polycystic ovary syndrome occurs in the postprandial and fasted state and is influenced by obesity and insulin sensitivity. Clin Endocrinol (2021) 94(1):74–84. doi: 10.1111/cen.14322
21. Liu LF, Hou XY, Song A, Guan YP, Tian PP, Wang C, et al. Oral fat tolerance testing identifies abnormal pancreatic beta-cell function and insulin resistance in individuals with normal glucose tolerance. J Diabetes Investig (2022) 13(11):1805–13. doi: 10.1111/jdi.13867
22. Bonatto NCM, de Oliveira PL, Mancebo AM, Costa LR, Bosculo MRM, Bosco AM, et al. Postprandial lipemia causes oxidative stress in dogs. Res Vet Sci (2021) 136:277–86. doi: 10.1016/j.rvsc.2021.03.008
23. Gorzelak-Pabis P, Wozniak E, Wojdan K, Chalubinski M, Broncel M. Single triglyceride-rich meal destabilizes barrier functions and initiates inflammatory processes of endothelial cells. J Interferon Cytokine Res (2020) 40(1):43–53. doi: 10.1089/jir.2018.0173
24. Horne RG, Yu YJ, Zhang R, Abdalqadir N, Rossi L, Surette M, et al. High fat-high fructose diet-induced changes in the gut microbiota associated with dyslipidemia in Syrian hamsters. Nutrients (2020) 12(11):20. doi: 10.3390/nu12113557
25. Berry SE, Valdes AM, Drew DA, Asnicar F, Mazidi M, Wolf J, et al. Human postprandial responses to food and potential for precision nutrition. Nat Med (2020) 26(6):964–+. doi: 10.1038/s41591-020-0934-0
26. Mora-Rodriguez R, Ortega JF, Morales-Palomo F, Ramirez-Jimenez M, Moreno-Cabanas A. Effects of statin therapy and exercise on postprandial triglycerides in overweight individuals with hypercholesterolaemia. Br J Clin Pharmacol (2020) 86(6):1089–99. doi: 10.1111/bcp.14217
27. Van Eck N, Waltman L. Software survey: VOSviewer, a computer program for bibliometric mapping. scientometrics (2010) 84(2):523–38. doi: 10.1007/s11192-009-0146-3
28. Chen C. System and method for automatically generating systematic reviews of a scientific field. U.S. Patent (2013) 8(566):360.
29. Garner RM, Hirsch JA, Albuquerque FC, Fargen KM. Bibliometric indices: defining academic productivity and citation rates of researchers, departments and journals. J neurointerv Surg (2018) 10(2):102–6. doi: 10.1136/neurintsurg-2017-013265
30. Chen C, Ibekwe-SanJuan F, Hou J. The structure and dynamics of cocitation clusters: a multiple-perspective cocitation analysis. J Am Soc Inf Sci Technol (2010) 61(7):1386–409. doi: 10.1002/asi.21309
31. Chapman MJ, Ginsberg HN, Amarenco P, Andreotti F, Borén J, Catapano AL, et al. Triglyceride-rich lipoproteins and high-density lipoprotein cholesterol in patients at high risk of cardiovascular disease: evidence and guidance for management. Eur Heart J (2011) 32(11):1345–61. doi: 10.1093/eurheartj/ehr112
32. Sarwar N, Gao P, Seshasai SR, Gobin R, Kaptoge S, Di Angelantonio E, et al. Diabetes mellitus, fasting blood glucose concentration, and risk of vascular disease: a collaborative meta-analysis of 102 prospective studies. Lancet (2010) 375(9733):2215–22. doi: 10.1016/s0140-6736(10)60484-9
33. Stanhope KL, Schwarz JM, Keim NL, Griffen SC, Bremer AA, Graham JL, et al. Consuming fructose-sweetened, not glucose-sweetened, beverages increases visceral adiposity and lipids and decreases insulin sensitivity in overweight/obese humans. J Clin Invest (2009) 119(5):1322–34. doi: 10.1172/jci37385
34. Nordestgaard BG. Triglyceride-rich lipoproteins and atherosclerotic cardiovascular disease: new insights from epidemiology, genetics, and biology. Circ Res (2016) 118(4):547–63. doi: 10.1161/circresaha.115.306249
35. Nordestgaard BG, Langsted A, Mora S, Kolovou G, Baum H, Bruckert E, et al. Fasting is not routinely required for determination of a lipid profile: clinical and laboratory implications including flagging at desirable concentration cut-points-a joint consensus statement from the European atherosclerosis society and European federation of clinical chemistry and laboratory medicine. Eur Heart J (2016) 37(25):1944–58. doi: 10.1093/eurheartj/ehw152
36. Bhatt DL, Steg PG, Miller M, Brinton EA, Jacobson TA, Ketchum SB, et al. Cardiovascular risk reduction with icosapent ethyl for hypertriglyceridemia. N Engl J Med (2019) 380(1):11–22. doi: 10.1056/NEJMoa1812792
37. Ference BA, Ginsberg HN, Graham I, Ray KK, Packard CJ, Bruckert E, et al. Low-density lipoproteins cause atherosclerotic cardiovascular disease. 1. evidence from genetic, epidemiologic, and clinical studies. a consensus statement from the European atherosclerosis society consensus panel. Eur Heart J (2017) 38(32):2459–72. doi: 10.1093/eurheartj/ehx144
38. Ference BA, Kastelein JJP, Ray KK, Ginsberg HN, Chapman MJ, Packard CJ, et al. Association of triglyceride-lowering LPL variants and LDL-C-Lowering LDLR variants with risk of coronary heart disease. Jama (2019) 321(4):364–73. doi: 10.1001/jama.2018.20045
39. Reiner Z, Catapano AL, De Backer G, Graham I, Taskinen MR, Wiklund O, et al. ESC/EAS guidelines for the management of dyslipidaemias: the task force for the management of dyslipidaemias of the European society of cardiology (ESC) and the European atherosclerosis society (EAS). Eur Heart J (2011) 32(14):1769–818. doi: 10.1093/eurheartj/ehr158
40. Stone NJ, Robinson JG, Lichtenstein AH, Bairey Merz CN, Blum CB, Eckel RH, et al. 2013 ACC/AHA guideline on the treatment of blood cholesterol to reduce atherosclerotic cardiovascular risk in adults: a report of the American college of Cardiology/American heart association task force on practice guidelines. Circulation (2014) 129(25 Suppl 2):S1–45. doi: 10.1161/01.cir.0000437738.63853.7a
41. Catapano AL, Graham I, De Backer G, Wiklund O, Chapman MJ, Drexel H, et al. 2016 ESC/EAS guidelines for the management of dyslipidaemias: the task force for the management of dyslipidaemias of the European society of cardiology (ESC) and European atherosclerosis society (EAS) developed with the special contribution of the European assocciation for cardiovascular prevention & rehabilitation (EACPR). Atherosclerosis (2016) 253:281–344. doi: 10.1016/j.atherosclerosis.2016.08.018
42. Zilversmit DB. Atherogenesis: a postprandial phenomenon. Circulation (1979) 60(3):473–85. doi: 10.1161/01.cir.60.3.473
43. Lopez-Miranda J, Williams C, Lairon D. Dietary, physiological, genetic and pathological influences on postprandial lipid metabolism. Br J Nutr (2007) 98(3):458–73. doi: 10.1017/s000711450774268x
44. O'Keefe JH, Bell DS. Postprandial hyperglycemia/hyperlipidemia (postprandial dysmetabolism) is a cardiovascular risk factor. Am J Cardiol (2007) 100(5):899–904. doi: 10.1016/j.amjcard.2007.03.107
45. Keirns BH, Sciarrillo CM, Hart SM, Emerson SR. Comparison of a standardized high-fat meal versus a high-fat meal scaled to body mass for measuring postprandial triglycerides: a randomized crossover study. Metabolites (2022) 12(1):81. doi: 10.3390/metabo12010081
46. Guan Y, Hou X, Tian P, Ren L, Tang Y, Song A, et al. Elevated levels of apolipoprotein CIII increase the risk of postprandial hypertriglyceridemia. Front Endocrinol (Lausanne) (2021) 12:646185. doi: 10.3389/fendo.2021.646185
47. Stenkula KG, Klemendz LE, Fryklund C, Wierup N, Alsalim W, Landin-Olsson M, et al. Postprandial triglyceride levels rather than fat distribution may reflect early signs of disturbed fat metabolism in Iraqi immigrants. Lipids Health Dis (2022) 21(1):68. doi: 10.1186/s12944-022-01679-x
48. Hou X, Song A, Guan Y, Tian P, Ren L, Tang Y, et al. Identification of the Chinese population that can benefit most from postprandial lipid testing: validation of the use of oral fat tolerance testing in clinical practice. Front Endocrinol (Lausanne) (2022) 13:831435. doi: 10.3389/fendo.2022.831435
49. Leohr J, Kjellsson MC. Impact of obesity on postprandial triglyceride contribution to glucose homeostasis, assessed with a semimechanistic model. Clin Pharmacol Ther (2022) 112(1):112–24. doi: 10.1002/cpt.2604
50. Yamamoto Y, Ozamoto Y, Kobayashi M, Tezuka Y, Azuma C, Sekine O, et al. Effects of a new 75 g glucose- and high fat-containing cookie meal test on postprandial glucose and triglyceride excursions in morbidly obese patients. Endocr J (2022) 69(6):689–703. doi: 10.1507/endocrj.EJ21-0615
51. Nakajima K, Nagamine T, Fujita MQ, Ai M, Tanaka A, Schaefer E. Apolipoprotein b-48: a unique marker of chylomicron metabolism. Adv Clin Chem (2014) 64:117–77. doi: 10.1016/B978-0-12-800263-6.00003-3
52. Krysa JA, Ball GDC, Vine DF, Jetha M, Proctor SD. ApoB-lipoprotein remnant dyslipidemia and high-fat meal intolerance is associated with markers of cardiometabolic risk in youth with obesity. Pediatr Obes (2021) 16(5):e12745. doi: 10.1111/ijpo.12745
53. Mena-Vázquez N, Rojas-Gimenez M, Jimenez Nuñez FG, Manrique-Arija S, Rioja J, Ruiz-Limón P, et al. Postprandial apolipoprotein B48 is associated with subclinical atherosclerosis in patients with rheumatoid arthritis. J Clin Med (2020) 9(8):2483. doi: 10.3390/jcm9082483
54. Seok S, Kim YC, Zhang Y, Kong B, Guo G, Ma J, et al. Feeding activates FGF15-SHP-TFEB-mediated lipophagy in the gut. EMBO J (2022) 41(17):e109997. doi: 10.15252/embj.2021109997
55. Alvarez-Jimenez L, Moreno-Cabañas A, Ramirez-Jimenez M, Morales-Palomo F, Ortega JF, Mora-Rodriguez R. Effects of statins and exercise on postprandial lipoproteins in metabolic syndrome vs metabolically healthy individuals. Br J Clin Pharmacol (2021) 87(3):955–64. doi: 10.1111/bcp.14447
56. Hjerpsted JB, Flint A, Brooks A, Axelsen MB, Kvist T, Blundell J. Semaglutide improves postprandial glucose and lipid metabolism, and delays first-hour gastric emptying in subjects with obesity. Diabetes Obes Metab (2018) 20(3):610–9. doi: 10.1111/dom.13120
57. Taskinen MR, Björnson E, Matikainen N, Söderlund S, Pietiläinen KH, Ainola M, et al. Effects of liraglutide on the metabolism of triglyceride-rich lipoproteins in type 2 diabetes. Diabetes Obes Metab (2021) 23(5):1191–201. doi: 10.1111/dom.14328
58. van Rooijen MA, Plat J, Zock PL, Blom WAM, Mensink RP. Effects of two consecutive mixed meals high in palmitic acid or stearic acid on 8-h postprandial lipemia and glycemia in healthy-weight and overweight men and postmenopausal women: a randomized controlled trial. Eur J Nutr (2021) 60(7):3659–67. doi: 10.1007/s00394-021-02530-2
59. Nordestgaard BG, Varbo A. Triglycerides and cardiovascular disease. Lancet (2014) 384(9943):626–35. doi: 10.1016/s0140-6736(14)61177-6
60. Cannon CP, Blazing MA, Giugliano RP, McCagg A, White JA, Theroux P, et al. Ezetimibe added to statin therapy after acute coronary syndromes. N Engl J Med (2015) 372(25):2387–97. doi: 10.1056/NEJMoa1410489
61. Sabatine MS, Giugliano RP, Keech AC, Honarpour N, Wiviott SD, Murphy SA, et al. Evolocumab and clinical outcomes in patients with cardiovascular disease. N Engl J Med (2017) 376(18):1713–22. doi: 10.1056/NEJMoa1615664
62. Hsieh J, Longuet C, Baker CL, Qin B, Federico LM, Drucker DJ, et al. The glucagon-like peptide 1 receptor is essential for postprandial lipoprotein synthesis and secretion in hamsters and mice. Diabetologia (2010) 53(3):552–61. doi: 10.1007/s00125-009-1611-5
63. Baggio LL, Drucker DJ. Biology of incretins: GLP-1 and GIP. Gastroenterology (2007) 132(6):2131–57. doi: 10.1053/j.gastro.2007.03.054
64. Xiao C, Dash S, Morgantini C, Patterson BW, Lewis GF. Sitagliptin, a DPP-4 inhibitor, acutely inhibits intestinal lipoprotein particle secretion in healthy humans. Diabetes (2014) 63(7):2394–401. doi: 10.2337/db13-1654
65. Wu CL, Yang TJ, Wu MH, Liang HJ, Chen YL, Wu SL, et al. Walking exercise reduces postprandial lipemia but does not influence postprandial hemorheological properties and oxidative stress. Metabolites (2022) 12(11):1038. doi: 10.3390/metabo12111038
66. Dunstan DW, Kingwell BA, Larsen R, Healy GN, Cerin E, Hamilton MT, et al. Breaking up prolonged sitting reduces postprandial glucose and insulin responses. Diabetes Care (2012) 35(5):976–83. doi: 10.2337/dc11-1931
67. Peddie MC, Rehrer NJ, Perry TL. Physical activity and postprandial lipidemia: are energy expenditure and lipoprotein lipase activity the real modulators of the positive effect? Prog Lipid Res (2012) 51(1):11–22. doi: 10.1016/j.plipres.2011.11.002
68. Alvarez-Jimenez L, Moreno-Cabanas A, Ramirez-Jimenez M, Morales-Palomo F, Ortega JF, Mora-Rodriguez R. Effectiveness of statins vs. exercise on reducing postprandial hypertriglyceridemia in dyslipidemic population: a systematic review and network meta-analysis. J Sport Health Sci (2022) 11(5):567–77. doi: 10.1016/j.jshs.2021.07.006
69. Kessler K, Gerl MJ, Hornemann S, Damm M, Klose C, Petzke KJ, et al. Shotgun lipidomics discovered diurnal regulation of lipid metabolism linked to insulin sensitivity in nondiabetic men. J Clin Endocrinol Metab (2020) 105(5):1501–14. doi: 10.1210/clinem/dgz176
70. Grant LK, Czeisler CA, Lockley SW, Rahman SA. Time-of-day and meal size effects on clinical lipid markers. J Clin Endocrinol Metab (2021) 106(3):e1373–e9. doi: 10.1210/clinem/dgaa739
71. Chiu CH, Chen CH, Wu MH, Lan PT, Hsieh YC, Lin ZY, et al. 5 days of time-restricted feeding increases fat oxidation rate but not affect postprandial lipemia: a crossover trial. Sci Rep (2022) 12(1):9295. doi: 10.1038/s41598-022-13387-8
72. Seo DB, Jeong HW, Kim YJ, Kim S, Kim J, Lee JH, et al. Fermented green tea extract exhibits hypolipidaemic effects through the inhibition of pancreatic lipase and promotion of energy expenditure. Br J Nutr (2017) 117(2):177–86. doi: 10.1017/s0007114516004621
73. Wang S, Ren H, Zhong H, Zhao X, Li C, Ma J, et al. Combined berberine and probiotic treatment as an effective regimen for improving postprandial hyperlipidemia in type 2 diabetes patients: a double blinded placebo controlled randomized study. Gut Microbes (2022) 14(1):2003176. doi: 10.1080/19490976.2021.2003176
Keywords: non-fasting lipid, postprandial lipid, cardiovascular diseases, diabetes, oral fat tolerance test, bibliometric analysis, VOSviewer, citespace
Citation: Hou Y, An Z, Hou X, Guan Y and Song G (2023) A bibliometric analysis and visualization of literature on non-fasting lipid research from 2012 to 2022. Front. Endocrinol. 14:1136048. doi: 10.3389/fendo.2023.1136048
Received: 02 January 2023; Accepted: 06 April 2023;
Published: 19 April 2023.
Edited by:
Yun Kyung Cho, Asan Medical Center, Republic of KoreaReviewed by:
Biqiang Zhu, Huazhong University of Science and Technology, ChinaAbraham Wall-Medrano, Universidad Autónoma de Ciudad Juárez, Mexico
Copyright © 2023 Hou, An, Hou, Guan and Song. This is an open-access article distributed under the terms of the Creative Commons Attribution License (CC BY). The use, distribution or reproduction in other forums is permitted, provided the original author(s) and the copyright owner(s) are credited and that the original publication in this journal is cited, in accordance with accepted academic practice. No use, distribution or reproduction is permitted which does not comply with these terms.
*Correspondence: Guangyao Song, sguangyao2@163.com