- 1Department of Neonatology, the First Hospital of Jilin University, Changchun, Jilin, China
- 2Department of Hepatobiliary and Pancreatic Surgery, the First Hospital of Jilin University, Changchun, Jilin, China
Background and objective: Uterine leiomyoma is the most common benign tumor in females of reproductive age. However, its causes have never been fully understood. The objective of our study was to analyze the causal association between various factors and uterine leiomyoma using Mendelian randomization (MR).
Methods: Genetic variables associated with risk factors were obtained from genome-wide association studies. Summary-level statistical data for uterine leiomyoma were obtained from FinnGen and the UK Biobank (UKB) consortium. We used inverse variance weighted, MR-Egger, and weighted median methods in univariate analysis. Multivariable MR analysis was used to identify independent risk factors. A fixed-effect model meta-analysis was used to combine the results of the FinnGen and UKB data.
Results: In the FinnGen data, higher genetically predicted age at natural menopause, systolic blood pressure (SBP), diastolic blood pressure (DBP), and fasting insulin were associated with an increased risk of uterine leiomyoma, while higher age at menarche was associated with a reduced risk of uterine leiomyoma. Multivariable MR analysis of SBP and DBP showed that higher DBP might be an independent risk factor of uterine leiomyoma. In the UKB data, the results for age at natural menopause, SBP, DBP, and age at menarche were replicated. The result of the meta-analysis suggested that uterine leiomyoma could also be affected by polycystic ovary syndrome (PCOS), endometriosis, and 2-hour glucose level.
Conclusion: Our MR study confirmed that earlier menstrual age, hypertension, obesity, and elevated 2-hour glucose post-challenge were risk factors for uterine leiomyoma, and the causal relationship between smoking and uterine leiomyoma was ruled out. In addition, later age of menopause and endometriosis were found to increase the risk of uterine leiomyoma, while PCOS was found to decrease the risk.
1 Introduction
Uterine leiomyomas (fibroids) are the most common benign tumor in females of reproductive age. Due to differences in race, diagnostic criteria, and study designs, epidemiological reports of uterine leiomyoma incidence vary widely, ranging from 4.5 to 68.6% (1). Since many uterine leiomyomas are asymptomatic and the histological incidence is more than twice as high as the clinical incidence, its true incidence may be underestimated in most studies (2). Johnson et al. (3) reported that the incidence of uterine leiomyoma increased with age, with a cumulative incidence of over 70% by menopause. In the United States, uterine leiomyoma accounts for 29% of gynecological hospitalizations among women aged 15-54 and 40-60% of all hysterectomies (4, 5).
Uterine leiomyoma has been shown to seriously impair woman’s quality of life and may lead to endometrial cancer (6). It can cause extensive or prolonged menstrual bleeding (leading to anemia, fatigue, and dysmenorrhea), abdominal swelling, painful intercourse, bladder or bowel dysfunction (leading to urinary incontinence or retention, pain, or constipation), and reproductive problems (such as impaired fertility, pregnancy complications, and miscarriage) (7, 8). If left untreated, it can even lead to death (9).
At present, the main treatment of uterine leiomyoma is hysterectomy, which is expensive and affects fertility. Nearly a quarter of women who have tried non-surgical treatment for fibroids choose to have surgery within a year (10). Many women opt for minimally invasive treatments to preserve their uterus, such as myomectomy, uterine artery embolization, and endometrial ablation. However, relapse is common after treatment (10). Therefore, it is necessary to clarify the risk factors of uterine leiomyoma for early prevention.
Several risk factors such as early age at menarche, early age at first birth, obesity, and hypertension have been established as increasing the risk of uterine leiomyoma (5, 11). However, due to the large number of undetected patients and the large bias of epidemiological data and risk factor evidence, its etiology is still far from being fully understood. In addition, some conflicting conclusions make it difficult to discover the true cause of uterine leiomyoma. For example, earlier studies have shown that smoking has a protective effect on fibroids (12, 13), while subsequent studies have shown that smoking increases the risk of uterine leiomyoma (14). One study showed that, among black women, those who self-reported PCOS had a 65% increased risk of fibroids compared with those who did not self-report PCOS (15). However, another study has shown that patients with PCOS had a lower risk of fibroids than women with normal ovaries (16). Additionally, some studies have found an inverse correlation between diabetes and uterine leiomyoma (13, 15, 17), and other researchers hypothesize that insulin stimulates fibroid growth (18, 19).
Therefore, whether there is a causal relationship between uterine leiomyoma and these factors still needs further analysis. Mendelian randomization (MR) is an emerging method of epidemiological causal inference, which uses genetic variation to determine the causal relationships between risk factors and outcomes. It relies on the natural random assortment of genetic variation during meiosis to distribute genetic variation randomly in a population, reducing bias caused by confounding or reverse causation (20). In our study, MR was used to explore the causal relationship between 20 risk factors and uterine leiomyomas. To our knowledge, this is the first MR study to examine the risk factors for uterine leiomyoma.
2 Methods
2.1 Summary statistics for risk factors
The summary statistics of anthropometric traits were from the GIANT (Genetic Investigation of Anthropometric Traits) consortium. For body mass index (BMI), the genome-wide association study (GWAS) included 234,069 Europeans and used sex, age, age squared, and principal components as covariates (21). For waist circumference, hip circumference, and waist-to-hip ratio, the GWAS included 210,088 Europeans and adjusted for age, age square, and study-specific covariates if necessary (22).
The summary statistics of DBP and SBP were obtained from the International Consortium for Blood Pressure, with 757,601 participants of European ancestry, and sex, age, and age squared were adjusted (23).
The summary statistics of serum 25-hydroxyvitamin D concentrations were from the SUNLIGHT consortium with 79,366 participants of European ancestry (24). The lead genetic variants of plasma vitamin C were derived from a GWAS meta-analysis of 52,018 Europeans from the Fenland study, the European Prospective Investigation into Cancer and Nutrition (EPIC)-InterAct study, the EPIC-Norfolk study, and the EPIC-CVD study (25).
In terms of smoking and drinking, the GWAS was conducted by the Sequencing Consortium of Alcohol and Nicotine use, which included 249,752 European participants for smoking and 335,394 European participants for drinking (26). Smoking was defined as the average number of cigarettes smoked per day, while drinking was the average number of alcoholic drinks consumed per week (including all types of alcohol). Age, sex, age-by-sex interaction, and the top 10 genetic principal components were used as covariates.
Three reproductive traits were involved in our study. The summary statistics of age at menarche (AAM) were from the largest meta-analysis of the ReproGen consortium, 23andMe, and the UK Biobank cumulatively including 329,345 women of European ancestry (27). The summary statistics of age at natural menopause (ANM) were from the ReproGen consortium with 69,360 European women (28). The summary statistics of age at first birth (AFB) were from a GWAS with 69,360 European individuals (29).
The summary statistics of PCOS were from a large-scale genome-wide meta-analysis with 10,074 PCOS cases and 103,164 controls of European ancestry (30). Cases were diagnosed with PCOS based on National Institutes of Health (NIH) or Rotterdam Criteria or by self-report, and age and BMI were used as covariables. The summary data for endometriosis included 17,045 endometriosis cases and 191,858 controls, 93% of whom were European (31).
The summary-level genetic data of T2D (Type 2 diabetes) were from the Diabetes Genetics Replication and Meta analysis consortium (32). A total of 74,124 T2D cases and 824,006 controls of European ancestry from 32 GWAS (with and without adjustment for BMI) were included.
The GWAS summary statistics of glycemic traits, including fasting glucose, fasting insulin, glycated hemoglobin (HbA1c), and 2-hour glucose post-challenge in an oral glucose tolerance test, were obtained from MAGIC (Meta-Analyses of Glucose and Insulin-related traits Consortium) (33). The GWAS included 281,416 participants, 70% of whom were of European ancestry. Our study used only European summary statistics and adjusted for covariates specific to the study.
2.2 GWAS summary statistics of uterine leiomyoma from the FinnGen and UKB consortia data sets
The GWAS summary statistics of uterine leiomyoma were obtained from FinnGen (https://r4.finngen.fi/) and the UKB. In the FinnGen data, the GWAS included 18,060 cases and 105,519 controls of European ancestry. The GWAS from the UKB included 4,351 cases and 332,848 controls and was conducted by the Neale Lab (http://www.nealelab.is/uk-biobank). Uterine leiomyoma is defined as ICD (International Classification of Diseases) 10: D25. When assessing causality, the FinnGen GWAS was used as the discovery set and the UKB GWAS as the validation set, considering that the FinnGen data had a higher proportion of cases. The main design of this study is shown in Supplementary Figure 1.
2.3 Ethics and consent statement
Specific ethical and consent statements for each GWAS in this study can be found in the original GWAS publications. The FinnGen Biobank GWAS was approved by the FinnGen Steering Committee. The Neale Lab received approval to conduct the GWAS from the Ethics Advisory Committee of the UKB. All of these data are de-identified, freely downloadable, and can be used without restriction.
2.4 Statistical analysis
We used a two-sample Mendelian randomization analysis to explore the potential causal relationship between 20 risk factors and uterine leiomyoma. Single nucleotide polymorphism (SNPs) with genome-wide significance (P < 5 × 10-8) and minor allele frequency >0.01 were included. Then, these SNPs were clumped based on the linkage disequilibrium r2< 0.01. The power of each SNP was assessed using F statistics (34) (F = beta2/se2), and the general F statistics of each exposure were also calculated. SNPs with weak statistical power were deleted (F statistics< 10).
We used inverse variance weighted (IVW) analysis as the primary statistical method. Although this method assumes that there is no heterogeneity between genetic variants (potentially due to pleiotropy), it has the strongest power to detect associations (35). In addition, we used two sensitivity analyses methods, including the MR-Egger (36) and weighted median (37) methods, as supplements to IVW. MR-Egger intercept and MR-PRESSO (38) methods were used to detect horizontal pleiotropy, and Cochran’s Q statistic was used to assess the heterogeneity. When there were outliers, the MR-PRESSO-corrected results would be reported in the main results. If heterogeneity still exists, the median based estimation was used as primary analysis. A false discovery rate (FDR) was used to adjust for multiple testing. In multivariable MR (MVMR) analysis, the IVW model was also the main method and the MR-Egger method was the complementary method.
A fixed-effects model meta-analysis was used to combine the results of the training set and verification set. All statistical analyses were performed using R software 4.1.2 (https://www.r-project.org/). The IVW, MR-Egger and weighted median methods were performed using the R packages “Two Sample MR” and “Mendelian Randomization”. The MVMR was performed using the R packages “Mendelian Randomization” and “MVMR”. P<0.05 was used as significance threshold. The mRnd was used to calculate the statistical power (39) for MR (https://cnsgenomics.shinyapps.io/mRnd/).
3 Results
3.1 Summary characteristics of risk factors
The number of SNPs ranged from 6 to 821, explaining 0.15% to 7.01% of the variance. The F statistics of each SNP and exposure were greater than 10, indicating that all instrumental variables had sufficient validity (Table 1).
3.2 Discovery results of uterine leiomyoma in the FinnGen consortium data set
In the FinnGen data set, a higher genetically predicted age at natural menopause (OR=1.0864 per standard deviation of age at natural menopause increase, 95%CI=1.0429-1.1317,P=6.97×10-5), SBP (OR=1.0073 per standard deviation of SBP increase, 95%CI=1.0026-1.0120,P=2.30×10-3), DBP (OR=1.0118 per standard deviation of DBP increase, 95%CI=1.0040-1.0197,P=3.09×10-3), and fasting insulin (OR=1.7342 per standard deviation of fasting insulin increase, 95%CI=1.1455-2.6253, P=9.25×10-3) were associated with an increased risk of uterine leiomyoma, while a genetically predicted higher age at menarche (OR=0.8435 per standard deviation of age of menarche increase, 95%CI=0.7999-0.8894, P=3.27×10-10) was associated with a reduced risk of uterine leiomyoma (FDR<0.05). T2D, endometriosis, and BMI showed a positive association with uterine leiomyoma risk (FDR> 0.05 and IVW P< 0.05). Both SBP and DBP are indicators of blood pressure, and some SNPs may be associated with both SBP and DBP. Therefore, we used multivariable MR to adjust the results of SBP and DBP. Multivariable MR analysis of SBP and DBP showed that a higher DBP might be an independent risk factor of uterine leiomyoma (adjusted OR=1.0309, 95%CI=1.0091-1.0533, P=5.34×10-3), while SBP was not significant (adjusted OR=0.9913, 95%CI=0.9787-1.0041, P=0.184).
The results of heterogeneity, pleiotropy, weighted median, and MR-Egger are shown in Table 2. There was heterogeneity in age at natural menopause, SBP, DBP, fasting insulin, age of menarche, T2D, and endometriosis, and they all showed MR-PRESSO-corrected results if outliers were detected. No horizontal pleiotropy was found. For some robust MR estimators, such as endometriosis, age at menarche, age at natural menopause, fasting insulin, T2D, and SBP, their IVW results were supported by MR-Egger or weighted median. However, the IVW results of waist-to-hip ratio and DBP were not supported by MR-Egger or weighted median, which may be related to the presence of horizontal pleiotropy and the detected outliers. The statistical power for the FinnGen outcome ranged from 96% to 100%.
3.3 Validation results of uterine leiomyoma in the UKB consortium data set
In the validation set, the MR results of SBP, DBP, age at menarche, and age at natural menopause were consistent with the training set. A higher age at natural menopause, SBP, and DBP were associated with an increased risk of uterine leiomyoma, while a higher age of menarche was associated with a reduced risk of uterine leiomyoma (Figure 1). No horizontal pleiotropy was found for these risk factors. After removing outliers, the odds of uterine leiomyoma increased per 1-SD increase in SBP (OR=1.0002, 95%CI=1.0001-1.0003, P=1.01×10-4), age at natural menopause (OR=1.0013, 95%CI=1.0006-1.0020, P=2.08×10-4), and DBP (OR=1.0002, 95%CI=1.0001-1.0004, P=8.95×10-3). Moreover, 1-SD increase in age at menarche (OR=0.9980, 95%CI=0.9969-0.9990, P=1.73×10-4) was associated with a reduced risk of uterine leiomyoma.
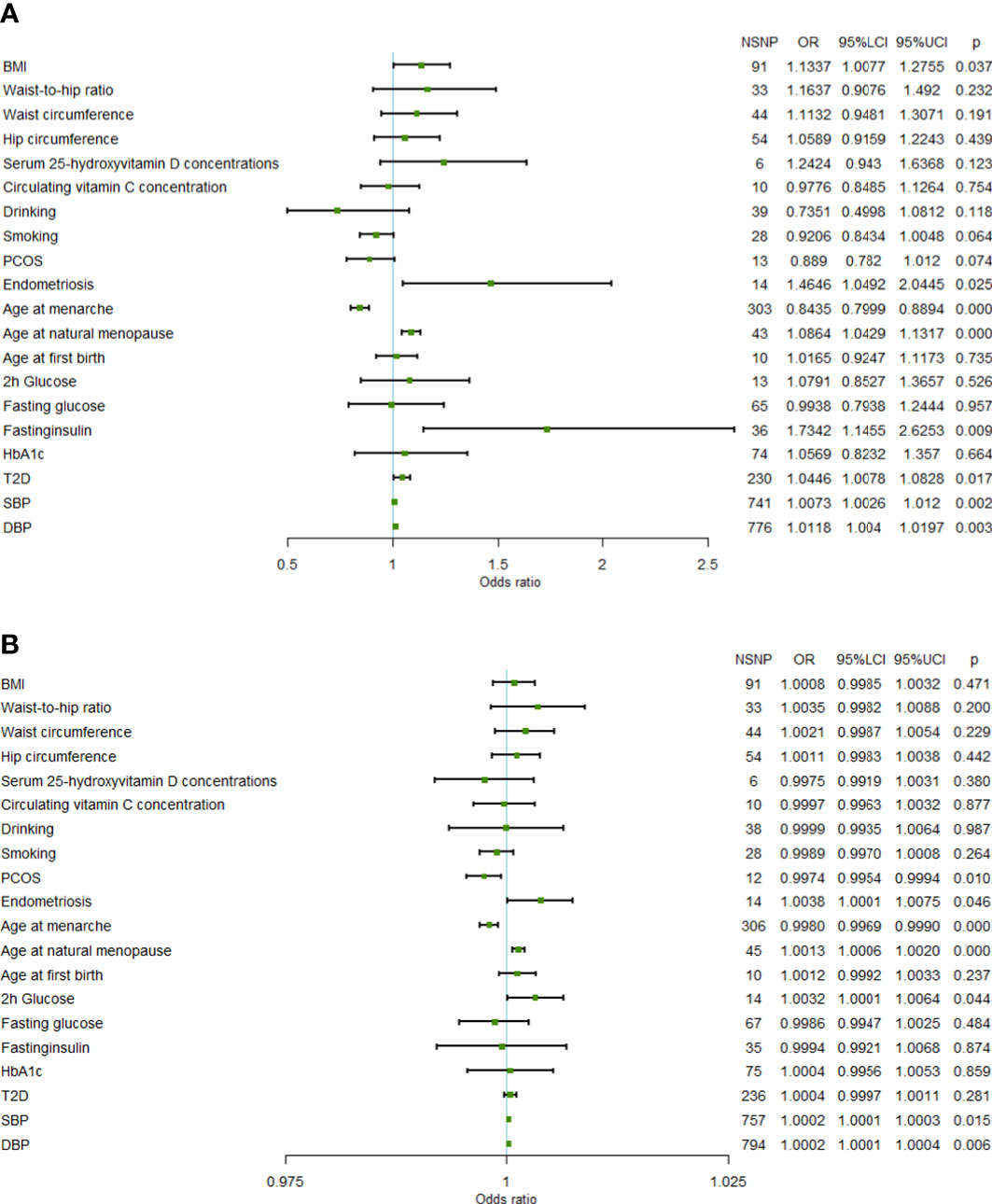
Figure 1 Forest plot of Mendelian randomization results. (A) Mendelian randomization results in the FinnGen data set. (B) Mendelian randomization results in the UKB data set (95%LCI, lower limit of 95% CI; 95%UCI, upper limit of 95% CI; BMI, body mass index; PCOS, polycystic ovary syndrome; 2h glucose, 2-hour glucose after oral glucose tolerance test; HbA1c, glycated hemoglobin; T2D, Type 2 diabetes; SBP, systolic blood pressure; DBP, diastolic blood pressure; NSNP, number of single nucleotide polymorphisms).
In addition, the results of the validation set showed that genetic liability to PCOS (OR=0.9974, 95%CI=0.9954-0.9994, P=1.09×10-2), 2-hour glucose level (OR=1.0032, 95%CI=1.0001-1.0064, P=4.57×10-2), and endometriosis (OR=1.0038, 95%CI=1.0001-1.0075, P=4.34×10-2) were also influential factors for uterine fibroids.
It is worth noting that the statistical power of the UKB results was not sufficient (<50%). The reason may be that UKB data set has fewer cases than the FinnGen data set, resulting in lower statistical power.
3.4 Combined result of uterine leiomyoma from meta-analysis
The results of the meta-analysis further confirmed the previous findings that a higher age at natural menopause (OR=1.0013, 95%CI=1.0006-1.0020, P=2.94×10-4), SBP (OR=1.0002, 95%CI=1.0001-1.0003, P=1.01×10-4), and DBP (OR=1.0002, 95%CI=1.0001-1.0003,P=8.95×10-3) are risk factors for uterine fibroids, and a higher age at menarche (OR=0.9979, 95%CI=0.9969-0.9990, P=1.02×10-4) is a protective factor for uterine leiomyoma (Figure 2).
In addition, the results of meta-analysis suggested that uterine leiomyoma may also be affected by PCOS (OR=0.9974, 95%CI=0.9954-0.9994, P=1.09×10-2), endometriosis (OR=1.0038, 95%CI=1.0002-1.0076, P=4.33×10-2), and 2-hour glucose levels (OR=1.0032, 95%CI=1.0001-1.0064, P=4.57×10-2). In fact, PCOS and endometriosis were also significant in the FinnGen results, although they failed to pass FDR correction. Therefore, the results of analysis of the FinnGen and UKB data sets and the meta-analysis of PCOS and endometriosis were consistent. The difference between the results of the FinnGen and UKB data sets for 2-hour glucose level may be related to the different number of SNPs in the instrumental variables.
4 Discussion
Our MR study found that a genetically predicted higher age at natural menopause, SBP, DBP, endometriosis, and elevated 2-hour glucose level were risk factors for uterine leiomyoma, and a higher age at menarche and PCOS were protective factors for uterine leiomyoma.
An earlier age at menarche is thought to be associated with an increased risk of uterine leiomyoma (40, 41), and this finding is further supported by our study. Women with an earlier age at menarche had higher levels of estradiol and estrone and lower levels of sex hormone-binding globulin in their hormonal milieu than women with a later age at menarche (42, 43). Fibroids were found to have more estrogen receptors, lower estradiol metabolism, and a stronger transcriptional response to estrogen than myometrium (44). Therefore, higher estrogen and progesterone levels may increase the risk of fibroids. Animal models have also confirmed that hormonal stimulation can increase tumor proliferation and decrease apoptosis (45). There are few studies on the effect of menopausal age on uterine leiomyoma risk. Our results suggest that a later age at menopause is associated with an increased risk of uterine leiomyoma. A prospective study of female teachers also found a reduced risk of fibroids in postmenopausal women compared to premenopausal women (13). The National Institute of Environmental Health Sciences (NIEHS) Fibroid Growth Study found that the growth rate of uterine leiomyoma in white women was related to age (46). Rapid growth of uterine leiomyoma after the age of 30, especially in premenopause, is consistent with age-related changes in estrogen and progesterone (47). Therefore, the effect of menopausal age on fibroids may also be related to hormone levels. In addition, mitotic activity in the myometrium is greatest during the luteal phase of the menstrual cycle, and prolonged exposure to the menstrual cycle may increase the risk of uterine leiomyoma (48). This also suggests that earlier menstruation age and later menopause age can increase the risk of uterine leiomyoma.
Several studies have found a significant positive association between hypertension and uterine leiomyoma, but most of these studies are retrospective studies, cross-sectional studies, or prospective studies with small sample sizes and have not successfully established a causal association (49–51). Our results suggest that higher SBP and DBP are causally associated with an increased risk of uterine leiomyoma. During the onset of hypertension, angiotensin is hydrolyzed to angiotensin I, which is then converted to angiotensin II by the angiotensin converting enzyme (ACE) (52). Angiotensin II has been reported to significantly increase the number of uterine leiomyoma cells in a dose-dependent manner (53). Hsieh et al. found that mutations in angiotensin-converting enzyme activation genes were significantly associated with leiomyoma susceptibility (54). A recent study reported a 31.8% reduction in clinically diagnosed uterine leiomyoma in hypertensive adult women who had previously used angiotensin-converting enzyme inhibitors (ACEis) compared with those who had not used ACEis (55). Therefore, hypertension may cause uterine leiomyoma through production of angiotensin II. In addition, hypertension can induce fibroid proliferation and fibrogenesis by inducing smooth muscle cell injury through mechanical shear stress, which may also lead to uterine leiomyoma (56).
The relationship between diabetes mellitus and uterine leiomyoma has been controversial for many years. On the one hand, some observational studies have found a lower incidence of fibroids in diabetic patients and hypothesized that diabetes may inhibit tumor development by causing vascular dysfunction (57). On the other hand, diabetes is often accompanied by obesity and hypertension, which may increase the risk of uterine leiomyoma. Since it is not possible to assess the relationship between diabetes and fibroids in untreated diabetic populations, observational estimates may capture both the effect of disease and treatment effects on fibroids. A study that indirectly examined the relationship between diabetes and treatment found that the protective effects of diabetes was only present in diabetic patients receiving the drug (15). Elevated 2-hour glucose post-challenge, an indicator of diabetes, was found in our study to be associated with an increased risk of uterine leiomyoma. However, fasting glucose, another indicator of diabetes, was not causally associated with uterine leiomyoma in either the FinnGen or the UKB data sets. In addition, elevated fasting insulin levels and type 2 diabetes were found to be associated with an increased risk of uterine leiomyoma in the FinnGen data, but this association was not found in the UKB data. Due to the low power of the UKB results, we were unable to determine the relationship between diabetes and uterine leiomyoma. To be sure, the risk of uterine leiomyoma should be considered when 2-hour glucose post-challenge is elevated.
Endometriosis polycystic ovary syndrome (PCOS) and uterine leiomyoma are common non-cancerous gynecological diseases in women. Our study found that endometriosis was associated with an increased risk of uterine leiomyoma, while PCOS was associated with a reduced risk of uterine leiomyoma. Although there is no direct evidence that endometriosis is a influence factor of uterine leiomyomas, Uimari et al. found that 20% of patients with symptomatic fibroids had endometriosis, and 26% of patients with symptomatic endometriosis had fibroids (58). Hemmings et al. (59) also reported that patients with endometriosis were more likely to develop uterine leiomyoma than patients without endometriosis. Physiologically, endometriosis tissues have been shown to express aromatase and produce estrogen independently of the ovary (60), which is a major cause of uterine leiomyoma. Therefore, aromatase inhibitors for endometriosis may reduce the risk of uterine leiomyoma. There are few studies on the relationship between PCOS and uterine leiomyoma, and existing studies are controversial (15, 16). Our study found that PCOS was associated with a reduced risk of uterine leiomyoma. Our results are consistent with a large later study using data from PPCOS I (National Institute of Child Health and Human Development Cooperative Reproductive Medicine Network Pregnancy in Polycystic Ovary Syndrome I), PPCOS II (Pregnancy in Polycystic Ovary Syndrome II), and AMIGOS (Assessing Multiple Intrauterine Gestations from Ovarian Stimulation) and found PCOS patients had a reduced risk of uterine leiomyoma compared to unexplained infertility patients (61). The results of this large study using ultrasound diagnosis were more reliable than those of a small sample using self-reported data. PCOS patients are anovulatory and have limited exposure of myometrium to progesterone, which has been shown to stimulate leiomyoma growth through a group of key genes that regulate apoptosis and proliferation, and may be the cause of this association (62–64).
Obesity has been consistently recognized as a risk factor for uterine leiomyoma. Given the insufficient power of the UKB database and the fact that a recent Mendelian randomization study (65) using different instrumental variables found that a higher BMI slightly increased the risk of uterine fibroids in the UKB data set, we concluded that obesity is unquestionably a risk factor for uterine leiomyomas.
Several studies have reported a negative association between age at first birth and uterine leiomyoma risk (40, 41, 66), while our study found no such association. Pregnancy may lead to decreased estrogen receptor levels in myometrium (67). Postpartum reduction of collagen content and smooth muscle cytoplasm can eliminate or shrink uterine leiomyoma (68). In addition, the vascular distribution of uterine leiomyoma is different from that of the myometrium, and delivery ischemia and uterine remodeling can give priority to the elimination of uterine leiomyoma (69, 70). But the relationship between age at first birth and uterine leiomyoma may be non-linear. Donnaet al. (71) reported that the effect of age at first birth on uterine leiomyoma was not linear, and mid-reproductive (25-29 years) delivery appeared to be most protective against fibroids development. Larger fibroids are more common in women over the age of 40. If women give birth at a young age, the disease may not develop. But there may be no benefit if the first pregnancy is too late, as some tumors may have grown too large. Our study was unable to determine whether there is a non-linear relationship between age and uterine leiomyoma risk, so more research is needed.
There are some advantages to our study: (1) This was a Mendelian randomized study that could find causal associations. (2) Our study found that some previously unknown factors, such as uterine leiomyoma and menopausal age, were associated with uterine leiomyoma. In addition, the influence of previously controversial factors such as PCOS, smoking, and diabetes on uterine leiomyoma were identified. And (3), participants in all GWAS studies were of predominantly European ancestry, with less racial bias. Discovery data sets, validation sets, and meta-analysis were used to increase the reliability of the results. Our research also has some shortcomings: (1) The influence of pleiotropy in the MR design, including horizontal pleiotropic and vertical pleiotropic; we used two sensitivity analysis methods to detect pluripotency, including MR-Egger intercept and MR-PRESSO, in the hope of minimizing bias. (2) The power of the results verified in the UKB data set was lower, resulting in several factors that were found to be significant in the FinnGen data set but not in the UKB. (3) The fact that the GWAS studies were mainly Europeans may have influenced the extrapolation of the results. In addition, the non-linear relationships could not be detected in this study. And (4), the genetic instruments were variants identified through GWAS analyses with p-values < 5x10-8. As a result, the estimates of these genetic effects tend to be upwardly biased due to a phenomenon known as the “winner’s curse”.
In conclusion, our MR study confirmed that earlier menstrual age, hypertension, obesity, and elevated 2-hour glucose post-challenge were risk factors for uterine leiomyoma, and ruled out the causal relationship between smoking and uterine leiomyoma. In addition, a later age of menopause and endometriosis were found to increase the risk of uterine leiomyoma, while PCOS was found to decrease the risk.
Data availability statement
The datasets presented in this study can be found in online repositories. The names of the repository/repositories and accession number(s) can be found below: ReproGen: (http://www.reprogen.org/); GIANT: (http://portals.broadinstitute.org/collaboration/giant/index.php/GIANT_consortium_data_files); MAGIC: (https://magicinvestigators.org/downloads/); GLGC: (http://lipidgenetics.org/#data-downloads-title); UKB: (http://www.nealelab.is/uk-biobank); FinnGen: (https://r4.finngen.fi/).
Ethics statement
Ethical review and approval was not required for the study on human participants in accordance with the local legislation and institutional requirements. The patients/participants provided their written informed consent to participate in this study. All the data used were from the GWAS studies, specific ethical and consent statements for each GWAS in this study can be found in the original GWAS publications.
Author contributions
HW and YQ conceptualized and designed the study, drafted the initial manuscript, and reviewed and revised the manuscript. YQ and LC collected the data and carried out the initial analyses. SG and YL reviewed and revised the manuscript. All authors approved the final manuscript as submitted and agree to be accountable for all aspects of the work.
Conflict of interest
The authors declare that the research was conducted in the absence of any commercial or financial relationships that could be construed as a potential conflict of interest.
Publisher’s note
All claims expressed in this article are solely those of the authors and do not necessarily represent those of their affiliated organizations, or those of the publisher, the editors and the reviewers. Any product that may be evaluated in this article, or claim that may be made by its manufacturer, is not guaranteed or endorsed by the publisher.
Supplementary material
The Supplementary Material for this article can be found online at: https://www.frontiersin.org/articles/10.3389/fendo.2023.1133260/full#supplementary-material
Supplementary Figure 1 | Main design of this study.
References
1. Millien C, Manzi A, Katz AM, Gilbert H, Smith Fawzi MC, Farmer PE, et al. Assessing burden, risk factors, and perceived impact of uterine fibroids on women's lives in rural Haiti: implications for advancing a health equity agenda, a mixed methods study. Int J Equity Health (2021) 20:1. doi: 10.1186/s12939-020-01327-9
3. Johnson G, MacLehose RF, Baird DD, Laughlin-Tommaso SK, Hartmann KE. Uterine leiomyomata and fecundability in the right from the start study. Hum Reprod (2012) 27:2991–7. doi: 10.1093/humrep/des263
4. Whiteman MK, Kuklina E, Jamieson DJ, Hillis SD, Marchbanks PA. Inpatient hospitalization for gynecologic disorders in the united states. Am J Obstet Gynecol (2010) 202:541. doi: 10.1016/j.ajog.2009.12.013
5. Pavone D, Clemenza S, Sorbi F, Fambrini M, Petraglia F. Epidemiology and risk factors of uterine fibroids. Best Pract Res Clin Obstet Gynaecol (2018) 46:3–11. doi: 10.1016/j.bpobgyn.2017.09.004
6. Kho PF, Mortlock S, Rogers PAW, Nyholt DR, Montgomery GW, Spurdle AB, et al. Genetic analyses of gynecological disease identify genetic relationships between uterine fibroids and endometrial cancer, and a novel endometrial cancer genetic risk region at the WNT4 1p36. 12 locus. Hum Genet (2021) 140:1353–65. doi: 10.1007/s00439-021-02312-0
7. Stewart EA, Cookson CL, Gandolfo RA, Schulze-Rath R. Epidemiology of uterine fibroids: a systematic review. Bjog (2017) 124:1501–12. doi: 10.1111/1471-0528.14640
8. Stewart EA, Laughlin-Tommaso SK, Catherino WH, Lalitkumar S, Gupta D, Vollenhoven B. Uterine fibroids. Nat Rev Dis Primers (2016) 2:16043. doi: 10.1038/nrdp.2016.43
9. Anneveldt KJ, van 't Oever HJ, Nijholt IM, Dijkstra JR, Hehenkamp WJ, Veersema S, et al. Systematic review of reproductive outcomes after high intensity focused ultrasound treatment of uterine fibroids. Eur J Radiol (2021) 141:109801. doi: 10.1016/j.ejrad.2021.109801
10. Donnez J, Dolmans MM. Uterine fibroid management: from the present to the future. Hum Reprod Update (2016) 22:665–86. doi: 10.1093/humupd/dmw023
11. Flake GP, Andersen J, Dixon D. Etiology and pathogenesis of uterine leiomyomas: a review. Environ Health Perspect (2003) 111:1037–54. doi: 10.1289/ehp.5787
12. Parazzini F, Negri E, La Vecchia C, Rabaiotti M, Luchini L, Villa A, et al. Uterine myomas and smoking. Results an Ital study. J Reprod Med (1996) 41:316–20.
13. Templeman C, Marshall SF, Clarke CA, DeLellis Henderson K, Largent J, Neuhausen S, et al. Risk factors for surgically removed fibroids in a large cohort of teachers. Fertil Steril (2009) 92:1436–46. doi: 10.1016/j.fertnstert.2008.08.074
14. Dragomir AD, Schroeder JC, Connolly A, Kupper LL, Hill MC, Olshan AF, et al. Potential risk factors associated with subtypes of uterine leiomyomata. Reprod Sci (2010) 17:1029–35. doi: 10.1177/1933719110376979
15. Wise LA, Palmer JR, Stewart EA, Rosenberg L. Polycystic ovary syndrome and risk of uterine leiomyomata. Fertil Steril (2007) 87:1108–15. doi: 10.1016/j.fertnstert.2006.11.012
16. Abdel-Gadir A, Oyawoye OO, Chander BP. Coexistence of polycystic ovaries and uterine fibroids and their combined effect on the uterine artery blood flow in relation to age and parity. J Reprod Med (2009) 54:347–52.
17. Baird DD, Travlos G, Wilson R, Dunson DB, Hill MC, D'Aloisio AA, et al. Uterine leiomyomata in relation to insulin-like growth factor-I, insulin, and diabetes. Epidemiology (2009) 20:604–10. doi: 10.1097/EDE.0b013e31819d8d3f
18. Poretsky L, Kalin MF. The gonadotropic function of insulin. Endocr Rev (1987) 8:132–41. doi: 10.1210/edrv-8-2-132
19. Okolo S. Incidence, aetiology and epidemiology of uterine fibroids. Best Pract Res Clin Obstet Gynaecol (2008) 22:571–88. doi: 10.1016/j.bpobgyn.2008.04.002
20. Emdin CA, Khera AV, Kathiresan S. Mendelian randomization. Jama (2017) 318:1925–6. doi: 10.1001/jama.2017.17219
21. Locke AE, Kahali B, Berndt SI, Justice AE, Pers TH, Day FR, et al. Genetic studies of body mass index yield new insights for obesity biology. Nature (2015) 518:197–206. doi: 10.1038/nature14177
22. Shungin D, Winkler TW, Croteau-Chonka DC, Ferreira T, Locke AE, Mägi R, et al. New genetic loci link adipose and insulin biology to body fat distribution. Nature (2015) 518:187–96. doi: 10.1038/nature14132
23. Evangelou E, Warren HR, Mosen-Ansorena D, Mifsud B, Pazoki R, Gao H, et al. Genetic analysis of over 1 million people identifies 535 new loci associated with blood pressure traits. Nat Genet (2018) 50:1412–25. doi: 10.1038/s41588-018-0205-x
24. Jiang X, O'Reilly PF, Aschard H, Hsu YH, Richards JB, Dupuis J, et al. Genome-wide association study in 79,366 European-ancestry individuals informs the genetic architecture of 25-hydroxyvitamin d levels. Nat Commun (2018) 9:260. doi: 10.1038/s41467-017-02662-2
25. Zheng JS, Luan J, Sofianopoulou E, Imamura F, Stewart ID, Day FR, et al. And type 2 diabetes: genome-wide association study and mendelian randomization analysis in European populations. Diabetes Care (2021) 44:98–106. doi: 10.2337/dc20-1328
26. Liu M, Jiang Y, Wedow R, Li Y, Brazel DM, Chen F, et al. Association studies of up to 1.2 million individuals yield new insights into the genetic etiology of tobacco and alcohol use. Nat Genet (2019) 51:237–44. doi: 10.1038/s41588-018-0307-5
27. Day FR, Thompson DJ, Helgason H, Chasman DI, Finucane H, Sulem P, et al. Genomic analyses identify hundreds of variants associated with age at menarche and support a role for puberty timing in cancer risk. Nat Genet (2017) 49:834–41. doi: 10.1038/ng.3841
28. Day FR, Ruth KS, Thompson DJ, Lunetta KL, Pervjakova N, Chasman DI, et al. Large-Scale genomic analyses link reproductive aging to hypothalamic signaling, breast cancer susceptibility and BRCA1-mediated DNA repair. Nat Genet (2015) 47:1294–303. doi: 10.1038/ng.3412
29. Barban N, Jansen R, de Vlaming R, Vaez A, Mandemakers JJ, Tropf FC, et al. Genome-wide analysis identifies 12 loci influencing human reproductive behavior. Nat Genet (2016) 48:1462–72. doi: 10.1038/ng.3698
30. Day F, Karaderi T, Jones MR, Meun C, He C, Drong A, et al. Large-Scale genome-wide meta-analysis of polycystic ovary syndrome suggests shared genetic architecture for different diagnosis criteria. PloS Genet (2018) 14:e1007813. doi: 10.1371/journal.pgen.1007813
31. Sapkota Y, Steinthorsdottir V, Morris AP, Fassbender A, Rahmioglu N, De Vivo I, et al. Meta-analysis identifies five novel loci associated with endometriosis highlighting key genes involved in hormone metabolism. Nat Commun (2017) 8:15539. doi: 10.1038/ncomms15539
32. Mahajan A, Taliun D, Thurner M, Robertson NR, Torres JM, Rayner NW, et al. Fine-mapping type 2 diabetes loci to single-variant resolution using high-density imputation and islet-specific epigenome maps. Nat Genet (2018) 50:1505–13. doi: 10.1038/s41588-018-0241-6
33. Chen J, Spracklen CN, Marenne G, Varshney A, Corbin LJ, Luan J, et al. The trans-ancestral genomic architecture of glycemic traits. Nat Genet (2021) 53:840–60. doi: 10.1038/s41588-021-00852-9
34. Chen L, Yang H, Li H, He C, Yang L, Lv G. Insights into modifiable risk factors of cholelithiasis: a mendelian randomization study. Hepatology (2022) 75:785–96. doi: 10.1002/hep.32183
35. Hemani G, Zheng J, Elsworth B, Wade KH, Haberland V, Baird D, et al. The MR-base platform supports systematic causal inference across the human phenome. Elife (2018) 7:e34408. doi: 10.7554/eLife.34408
36. Bowden J, Davey Smith G, Burgess S. Mendelian randomization with invalid instruments: effect estimation and bias detection through egger regression. Int J Epidemiol (2015) 44:512–25. doi: 10.1093/ije/dyv080
37. Bowden J, Davey Smith G, Haycock PC, Burgess S. Consistent estimation in mendelian randomization with some invalid instruments using a weighted median estimator. Genet Epidemiol (2016) 40:304–14. doi: 10.1002/gepi.21965
38. Verbanck M, Chen CY, Neale B, Do R. Detection of widespread horizontal pleiotropy in causal relationships inferred from mendelian randomization between complex traits and diseases. Nat Genet (2018) 50:693–8. doi: 10.1038/s41588-018-0099-7
39. Brion MJ, Shakhbazov K, Visscher PM. Calculating statistical power in mendelian randomization studies. Int J Epidemiol (2013) 42:1497–501. doi: 10.1093/ije/dyt179
40. Wise LA, Palmer JR, Harlow BL, Spiegelman D, Stewart EA, Adams-Campbell LL, et al. Reproductive factors, hormonal contraception, and risk of uterine leiomyomata in African-American women: a prospective study. Am J Epidemiol (2004) 159:113–23. doi: 10.1093/aje/kwh016
41. Terry KL, De Vivo I, Hankinson SE, Missmer SA. Reproductive characteristics and risk of uterine leiomyomata. Fertil Steril (2010) 94:2703–7. doi: 10.1016/j.fertnstert.2010.04.065
42. Abetew DF, Enquobahrie DA, Dishi M, Rudra CB, Miller RS, Williams MA. Age at menarche, menstrual characteristics, and risk of preeclampsia. ISRN Obstet Gynecol (2011) 2011:472083. doi: 10.5402/2011/472083
43. Emaus A, Espetvedt S, Veierød MB, Ballard-Barbash R, Furberg AS, Ellison PT, et al. 17-beta-estradiol in relation to age at menarche and adult obesity in premenopausal women. Hum Reprod (2008) 23:919–27. doi: 10.1093/humrep/dem432
44. Velez Edwards DR, Baird DD, Hartmann KE. Association of age at menarche with increasing number of fibroids in a cohort of women who underwent standardized ultrasound assessment. Am J Epidemiol (2013) 178:426–33. doi: 10.1093/aje/kws585
45. Hunter DS, Hodges LC, Eagon PK, Vonier PM, Fuchs-Young R, Bergerson JS, et al. Influence of exogenous estrogen receptor ligands on uterine leiomyoma: evidence from an in vitro/in vivo animal model for uterine fibroids. Environ Health Perspect (2000) 108 Suppl 5:829–34. doi: 10.1289/ehp.00108s5829
46. Peddada SD, Laughlin SK, Miner K, Guyon JP, Haneke K, Vahdat HL, et al. Growth of uterine leiomyomata among premenopausal black and white women. Proc Natl Acad Sci USA (2008) 105:19887–92. doi: 10.1073/pnas.0808188105
47. Baird DD, Kesner JS, Dunson DB. Luteinizing hormone in premenopausal women may stimulate uterine leiomyomata development. J Soc Gynecol Investig (2006) 13:130–5. doi: 10.1016/j.jsgi.2005.12.001
48. Wise LA, Laughlin-Tommaso SK. Epidemiology of uterine fibroids: from menarche to menopause. Clin Obstet Gynecol (2016) 59:2–24. doi: 10.1097/GRF.0000000000000164
49. Silver MA, Raghuvir R, Fedirko B, Elser D. Systemic hypertension among women with uterine leiomyomata: potential final common pathways of target end-organ remodeling. J Clin Hypertens (Greenwich) (2005) 7:664–8. doi: 10.1111/j.1524-6175.2005.04384.x
50. Takeda T, Sakata M, Isobe A, Miyake A, Nishimoto F, Ota Y, et al. Relationship between metabolic syndrome and uterine leiomyomas: a case-control study. Gynecol Obstet Invest (2008) 66:14–7. doi: 10.1159/000114250
51. Haan YC, Diemer FS, van der Woude L, Van Montfrans GA, Oehlers GP, Brewster LM. The risk of hypertension and cardiovascular disease in women with uterine fibroids. J Clin Hypertens (Greenwich) (2018) 20:718–26. doi: 10.1111/jch.13253
52. Narkiewicz K. Diagnosis and management of hypertension in obesity. Obes Rev (2006) 7:155–62. doi: 10.1111/j.1467-789X.2006.00226.x
53. Isobe A, Takeda T, Sakata M, Miyake A, Yamamoto T, Minekawa R, et al. Dual repressive effect of angiotensin II-type 1 receptor blocker telmisartan on angiotensin II-induced and estradiol-induced uterine leiomyoma cell proliferation. Hum Reprod (2008) 23:440–6. doi: 10.1093/humrep/dem247
54. Hsieh YY, Lee CC, Chang CC, Wang YK, Yeh LS, Lin CS. Angiotensin I-converting enzyme insertion-related genotypes and allele are associated with higher susceptibility of endometriosis and leiomyoma. Mol Reprod Dev (2007) 74:808–14. doi: 10.1002/mrd.20474
55. Fischer NM, Nieuwenhuis TO, Singh B, Yenokyan G, Segars JH. Angiotensin-converting enzyme inhibitors reduce uterine fibroid incidence in hypertensive women. J Clin Endocrinol Metab (2021) 106:e650–9. doi: 10.1210/clinem/dgaa718
56. Humphrey JD. Mechanisms of arterial remodeling in hypertension: coupled roles of wall shear and intramural stress. Hypertension (2008) 52:195–200. doi: 10.1161/HYPERTENSIONAHA.107.103440
57. Walocha JA, Litwin JA, Miodoński AJ. Vascular system of intramural leiomyomata revealed by corrosion casting and scanning electron microscopy. Hum Reprod (2003) 18:1088–93. doi: 10.1093/humrep/deg213
58. Uimari O, Järvelä I, Ryynänen M. Do symptomatic endometriosis and uterine fibroids appear together? J Hum Reprod Sci (2011) 4:34–8. doi: 10.4103/0974-1208.82358
59. Hemmings R, Rivard M, Olive DL, Poliquin-Fleury J, Gagné D, Hugo P, et al. Evaluation of risk factors associated with endometriosis. Fertil Steril (2004) 81:1513–21. doi: 10.1016/j.fertnstert.2003.10.038
60. Bulun SE, Lin Z, Imir G, Amin S, Demura M, Yilmaz B, et al. Regulation of aromatase expression in estrogen-responsive breast and uterine disease: from bench to treatment. Pharmacol Rev (2005) 57:359–83. doi: 10.1124/pr.57.3.6
61. Huang H, Kuang H, Sun F, Diamond MP, Legro RS, Coutifaris C, et al. Lower prevalence of non-cavity-distorting uterine fibroids in patients with polycystic ovary syndrome than in those with unexplained infertility. Fertil Steril (2019) 111:1011–1019.e1. doi: 10.1016/j.fertnstert.2019.01.020
62. Moravek MB, Yin P, Ono M, J.S.t. Coon, Dyson MT, Navarro A, et al. Ovarian steroids, stem cells and uterine leiomyoma: therapeutic implications. Hum Reprod Update (2015) 21:1–12. doi: 10.1093/humupd/dmu048
63. Blake RE. Leiomyomata uteri: hormonal and molecular determinants of growth. J Natl Med Assoc (2007) 99:1170–84.
64. Rein MS, Barbieri RL, Friedman AJ. Progesterone: a critical role in the pathogenesis of uterine myomas. Am J Obstet Gynecol (1995) 172:14–8. doi: 10.1016/0002-9378(95)90077-2
65. Venkatesh SS, Ferreira T, Benonisdottir S, Rahmioglu N, Becker CM, Granne I, et al. Obesity and risk of female reproductive conditions: a mendelian randomisation study. PloS Med (2022) 19:e1003679. doi: 10.1371/journal.pmed.1003679
66. Marshall LM, Spiegelman D, Goldman MB, Manson JE, Colditz GA, Barbieri RL, et al. A prospective study of reproductive factors and oral contraceptive use in relation to the risk of uterine leiomyomata. Fertil Steril (1998) 70:432–9. doi: 10.1016/S0015-0282(98)00208-8
67. Kawaguchi K, Fujii S, Konishi I, Iwai T, Nanbu Y, Nonogaki H, et al. Immunohistochemical analysis of oestrogen receptors, progesterone receptors and ki-67 in leiomyoma and myometrium during the menstrual cycle and pregnancy. Virchows Arch A Pathol Anat Histopathol (1991) 419:309–15. doi: 10.1007/BF01606522
68. Schwartz SM, Marshall LM, Baird DD. Epidemiologic contributions to understanding the etiology of uterine leiomyomata. Environ Health Perspect (2000) 108 Suppl 5:821–7. doi: 10.1289/ehp.00108s5821
69. Burbank F. Childbirth and myoma treatment by uterine artery occlusion: do they share a common biology? J Am Assoc Gynecol Laparosc (2004) 11:138–52. doi: 10.1016/S1074-3804(05)60189-2
70. Laughlin SK, Schroeder JC, Baird DD. New directions in the epidemiology of uterine fibroids. Semin Reprod Med (2010) 28:204–17. doi: 10.1055/s-0030-1251477
Keywords: uterine leiomyoma, risk factor, Mendelian randomization, influence, study
Citation: Qu Y, Chen L, Guo S, Liu Y and Wu H (2023) Genetic liability to multiple factors and uterine leiomyoma risk: a Mendelian randomization study. Front. Endocrinol. 14:1133260. doi: 10.3389/fendo.2023.1133260
Received: 03 January 2023; Accepted: 06 June 2023;
Published: 27 July 2023.
Edited by:
Kavita Jadhav, Epic-Bio, United StatesReviewed by:
Yuan Shi, Children’s Hospital of Chongqing Medical University, ChinaBo Li, Jilin University, China
Copyright © 2023 Qu, Chen, Guo, Liu and Wu. This is an open-access article distributed under the terms of the Creative Commons Attribution License (CC BY). The use, distribution or reproduction in other forums is permitted, provided the original author(s) and the copyright owner(s) are credited and that the original publication in this journal is cited, in accordance with accepted academic practice. No use, distribution or reproduction is permitted which does not comply with these terms.
*Correspondence: Hui Wu, d3VodWlAamx1LmVkdS5jbg==