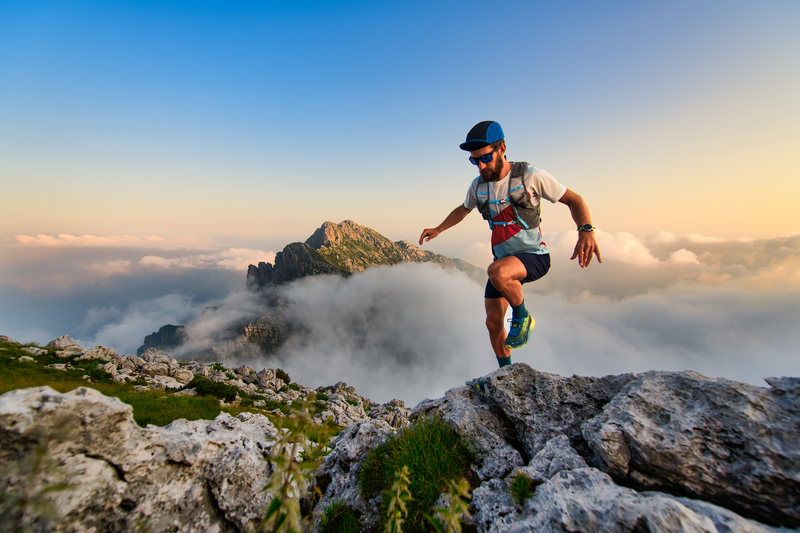
95% of researchers rate our articles as excellent or good
Learn more about the work of our research integrity team to safeguard the quality of each article we publish.
Find out more
ORIGINAL RESEARCH article
Front. Endocrinol. , 12 April 2023
Sec. Gut Endocrinology
Volume 14 - 2023 | https://doi.org/10.3389/fendo.2023.1128767
This article is part of the Research Topic Emerging Roles of the Gut Microbiota in the Pathogenesis of Metabolic Disorders - Volume II View all 8 articles
Introduction: The human gut microbiota (GM) is a dynamic system which ecological interactions among the community members affect the host metabolism. Understanding the principles that rule the bidirectional communication between GM and its host, is one of the most valuable enterprise for uncovering how bacterial ecology influences the clinical variables in the host.
Methods: Here, we used SparCC to infer association networks in 16S rRNA gene amplicon data from the GM of a cohort of Mexican patients with type 2 diabetes (T2D) in different stages: NG (normoglycemic), IFG (impaired fasting glucose), IGT (impaired glucose tolerance), IFG + IGT (impaired fasting glucose plus impaired glucose tolerance), T2D and T2D treated (T2D with a 5-year ongoing treatment).
Results: By exploring the network topology from the different stages of T2D, we observed that, as the disease progress, the networks lose the association between bacteria. It suggests that the microbial community becomes highly sensitive to perturbations in individuals with T2D. With the purpose to identify those genera that guide this transition, we computationally found keystone taxa (driver nodes) and core genera for a Mexican T2D cohort. Altogether, we suggest a set of genera driving the progress of the T2D in a Mexican cohort, among them Ruminococcaceae NK4A214 group, Ruminococcaceae UCG-010, Ruminococcaceae UCG-002, Ruminococcaceae UCG-005, Alistipes, Anaerostipes, and Terrisporobacter.
Discussion: Based on a network approach, this study suggests a set of genera that can serve as a potential biomarker to distinguish the distinct degree of advances in T2D for a Mexican cohort of patients. Beyond limiting our conclusion to one population, we present a computational pipeline to link ecological networks and clinical stages in T2D, and desirable aim to advance in the field of precision medicine.
Type 2 Diabetes Mellitus (T2D) is considered a global epidemic with a constant increase in new cases and negative economic and social impact on public health (1, 2). T2D is preceded by prediabetes (PreT2D), a condition characterized by intermediate hyperglycemia, insulin resistance (IR), and β-cell dysfunction that predisposes individuals to the development of T2D (3, 4). PreT2D can be prevented or delayed through lifestyle modifications, drugs, or bariatric surgery; however, up to 70% of people with preT2D will eventually evolve into T2D (4, 5). Current strategies for successfully diagnosing and managing preT2D are limited in part by an incomplete understanding of its pathophysiology (2).
Recently, human gut microbiota (GM) alterations were proposed as an important factor in the progression of T2D (6). Several cohorts and cross-sectional studies worldwide reported associations between the GM composition to preT2D and T2D (7). These studies indicated that several gut microorganisms (e.g., Escherichia/Shigella, Ruminococcus, Dorea, and Veillonella) (8) increase the absorption of energy from food, cause chronic low-grade inflammation, regulate fatty acid metabolism, secrete derived peptides, and increase the metabolic endotoxins production (lipopolysaccharides), which conduct into insulin resistance (IR). Furthermore, long-term IR leads to a constant raised level of systemic glucose concentration (9). According to these studies, a clear association between GM composition and T2D has been observed. However, bacterial genera associated with preTD2 and T2D differ when different populations are compared (8). For example, a Danish preT2D population suffers dysbiosis in the GM characterized by a decreased abundance of Clostridium genus and Akkermansia muciniphila strain (10). European and Chinese studies have demonstrated that the quantity of Firmicutes, Bifidobacteria, and Clostridia was significantly lower in T2D patients compared to healthy individuals, while the number of Bacteroidetes and beta Proteobacteria was markedly higher in both populations (11, 12). Likewise, in the Chinese cohort, the Bacteroidetes/Firmicutes ratio in T2D was positively and significantly correlated with plasma glucose concentration. Still, it appeared independent of body weight, confirming its association with reduced glucose tolerance (12). Moreover, a cross-sectional study from two Dutch population-based cohorts: the Rotterdam Study and the Lifelines DEEP study, reported associations among α diversity, β diversity, and taxa with the Homeostatic Model Assessment of Insulin Resistance (HOMA-IR) and with T2D. They reported 12 bacterial genera (butyrate producers) associated with HOMA-IR or T2D. (i.e., Christensenellaceae, Christensenellaceae R7 group, Marvinbryantia, Ruminococcaceae UCG-005, Ruminococcaceae UCG-008, Ruminococcaceae UCG-010, Ruminococcaceae NK4A214 group, Clostridiaceae 1, Peptostreptococcaceae, Clostridium sensu stricto 1, Intestinibacter and Romboutsia) (13).
The composition and structure of GM can be analyzed with specialized bioinformatics tools such as Sparse Correlations for Compositional data (SparCC), Sparse and Compositionally Robust Inference of Microbial Ecological Networks (SPEIC-EASI), and Bayesian Analysis of Compositional Covariance (BAnOCC) (14). Due to the high data complexity, these algorithms must be able to model the complex interactions and nonlinear effects between microbial communities (15). In a Mexican population with preT2D and T2D, Diener and collaborators studied 16S rRNA gene amplicon data from a cohort of 405 participants. They reported that Escherichia and Veillonella were associated with T2D progression, along with biochemical measures of blood glucose and insulin-related measures. Furthermore, Blautia and Anaerostipes were related to improved β-cell function and insulin efficiency, and these genera decreased with T2D development. Besides, the authors argue remarkable evidence that GM can alter intestinal inflammation (8).
A Chinese Cohort of 450 T2D subjects was exposed to two clinical interventions: metformin and AMC (Chinese herbal formula from Rhizoma Anemarrhenae, Momordica charantia, Coptis Chinensis, aloe vera, and red yeast rice). Authors reported GM alterations through SparCC association networks in the group treated with metformin. They observed a notable increase in Blautia spp (producer of short-chain fatty acids SCFA). Moreover, AMC treatment increased the abundance of two genera related to butyrate production (i.e., Faecalibacterium and Roseburia) (16, 17). All these results have contributed to a better understanding of the role of GM and T2D. However, these contributions remained at the association studies level without a deeper analysis of the ecological insights of gut microbial communities. Subsequently, medicine has emphasized reductionist ways, where the subunits of a system are analyzed separately, ignoring their complex non-linear interactions (18).
To understand the interactions and insights between GM and T2D patients, we studied the behavior of GM based on the concept of “the medical ecology of the human gut microbiome”. This concept relays on the need for new ecological perspectives and dynamical systems theory to advocate personalized medicine (19, 20). Furthermore, precision medicine has emerged with remarkable results in treating T2D Sommer and collaborators. (21) and collaborators reported differences in hemoglobin A1c (HbA1c) reductions between three T2D drugs (sitagliptin, pioglitazone, and canagliflozin) (21). According to their results, using simple clinical measures to identify the drug class most likely to deliver the greatest glycemic reduction for a given patient (22).
Bioinformatics, omics sciences, and systems biology have paved the way for the development of new strategies, to characterize a healthy and unhealthy microbiota composition and its relationship with the host (23). For example, Ezzamouri and collaborators used metagenomics data from a metformin study with genome-scale metabolic modelling of the key bacteria (e.g. Akkermansia municiphila, Intestinibacter bartlettii, Clostridium saudiense, Romboutsia timonensis) to research the mechanistic role of the GM in response to metformin in a Spanish T2D cohort (24). However, the GM is a complex ecological system that involves interactions between hundreds of bacterial species. Thus, scientific research should focus on studying complex networks of nonlinear interactions between many entities. Efforts to develop this field of medical ecology of the human GM have been reported (25, 26). Although specifically for Mexican T2D patients, our work represents the first attempt from a medical ecology perspective to understand the interactions between the GM and its host. For this reason, we used GM 16S rRNA gene amplicon data from a Mexican T2D cohort to perform systems biology and bioinformatics analysis between study groups (i.e., NG (Normoglycemic) IFG (Impaired Fasting Glucose), IGT (Impaired Glucose Tolerance), IFG+IGT (both conditions of preT2D), T2D (Type 2 diabetes), and T2D (Type 2 diabetes with 5 years ongoing treatment). Our specific objectives were to accomplish a: 1) GM ecological analysis (α-β diversity), 2) evaluation and analysis of association networks focused on their topological characteristics and their association with the clinical status, 3) differential abundance analysis at the genus level, and 4) supervised machine learning analysis to denoise GM data and improve the identification of keystone-taxa pattern shifts in Mexican T2D subjects.
Our results shed light on the role of several genera as driver nodes to explain the changes in the community structure from one stage to another, along with the development of preT2D and T2D within a Mexican cohort.
We performed an association network analysis with GM 16S rRNA gene amplicon data from a Mexican cohort of T2D patients (8, 27) (Figure 1). Specifically, we used variants.csv file (16S rRNA gene amplicon sequence variants ASV and their abundances in samples) and taxa.csv file (taxonomy assignment for each variant) from the GitHub repository https://github.com/resendislab/mext2d/tree/master/data. Then, we stratified all samples based on their T2D status as follows: 1) NG, 2) IFG, 3) IGT, 4) IFG+IGT, 5) T2D, and 6) T2D treated.
Figure 1 Experimental and Bioinformatics for taxonomic and ecological analyses, inference networks, network analyses, differential abundance analyses, microbial structure analyses, and supervised machine learning analysis. Bioinformatic tools are added at the bottom of the figure.
With the taxonomy assignment for amplicon sequence variants (ASVs), we prepared Krona plots (RRID: SCR_012785) to explore the microbial diversity of all samples (28). We constructed rarefaction curves using the R library vegan v2.4-6 (RRID: SCR_011950) (29) from R (version 4.0.4). A phyloseq object with ASV data was used to calculate α diversity indexes (i.e., Observed, Chao 1, Fisher, Simpson, Inv Simpson, and Shannon indexes), which were computed by R Phyloseq (RRID: SCR_013080) library 1.34.0 (30).
We used EdgeR (RRID: SCR_012802) (31) to assess differential abundance changes of the GM along different T2D stages. First, we filtered out OTUs for which the variance across all samples is very low (1e-5) and did this before ever passing the data to edgeR. Also, we estimated a series of log-linear generalized linear models predicting each ASV abundance. ASVs were considered differentially abundant at a false discovery rate (FDR) < 0.01. To examine potential keystone taxa at the genus level, we analyzed differentially abundant bacteria between two stages of T2D: NG vs IFG, IFG vs IGT, IFG+IGT vs T2D, and T2D vs T2D treated. Moreover, these pairwise comparisons were prepared to evaluate the changes in the GM composition in the subsequent stages, that is, step by step, until the frank diagnosis of T2D (27, 32). Finally, we performed an upset plot graph to describe intersections of differentially abundant genera between comparisons.
We used the SparCC network inference approach (RRID: SCR_022734) (33) to infer underlying interactions from each group (i.e., NG, IFG, IGT, IFG+IGT, T2D, and T2D treated). Then, we utilized the non-normalized taxonomic abundance at the genus level to compute associations and prepared one network for each group. SparCC was run based on in-house scripts and adaptations from Netherlands Bioinformatics and Systems Biology Research School. First, we computed correlations (compositionality-robust) as the median of ten iterations, where SparCC averages its results over several estimates of the true fractions, with the Dirichlet distribution. Second, we calculated bootstraps and prepared one correlation matrix from one resampled dataset (n=100 iterations). Third, we computed p-values, based on bootstrapped correlation scores. Fourth, we selected nodes and edges based on the determined cut point of correlation level (0.60). The results from SparCC were plotted in an interactive network with VisNetwork R library (34) and were uploaded to Github (https://github.com/resendislab/MEXT2D_Networks).
To further evaluate the topological features of each network, we used Netshift software (RRID: SCR_022733) to identify driver nodes between case-control association networks (https://web.rniapps.net/netshift/) (35). We focused on identifying key attributes (e.g., nodes, clusters, and edges) based on a two conditions approach. NG vs IFG, IFG vs IGT, IFG+IGT vs T2D, T2D vs T2D treated. Then, we detected driver nodes from all comparisons.
Absence/presence plots were generated using the Upset R library (RRID: SCR_022731) (36) from the nodes network for each group (i.e., NG, IFG, IGT, IFG+IGT, T2D, and T2D treated).
We denoised ASV data from the GM of a Mexican T2D cohort (https://github.com/resendislab/mext2d/tree/master/data) through a supervised denoising-machine learning approach named mb-PHENIX (37). We performed this analysis to observe the driver nodes involved in the transition of T2D states (37) and because of the high rate of missing information of microbiome data (38) that do not let find cluster structure with traditional unsupervised methods. The mb-PHENIX method consists of mapping different classes (here T2D stages) in the low dimensional space as far apart as possible, while maintaining the internal class structure and the inter-class relationships. Then, the missing taxa data is recovered (denoising) by sharing taxa information among the nearest neighbors.
We preprocessed the ASV table for imputation with the following steps: 1) We filtered the ASV by the number of counts above 20 which detection is deemed non-negligible and at least appears in 5 samples. 2) We performed L1 normalization. 3) Root squared transformation with the parameters of mb-PHENIX as follows: for PCA (n_components=100,random_state=1), then for the PCA space we applied supervised embedding with UMAP (n_components=2, verbose=True, metric=‘cosine’, n_epochs=1000, min_dist=0.1, random_state=1, n_neighbors=500, target_weight=0.5), and last the imputation via diffusion (t=5, decay=50, metric=‘euclidean’, knn=17). After imputation, we calculated the most significant taxa for each T2D stage within the imputed ASV data with the earth mover’s distance metric (EMD) (39). Particularly, we compared each T2D stage against the rest of the groups to quantify the differences between distributions among T2D stages. Then, the EMD was multiplied by the sign of the mean difference of each cluster to denote the overall direction of the shift (EMD score). Lastly, the imputed ASV table was collapsed to identify their taxonomical annotation at the genus level. We used EMD (40), a nonparametric measure of the distance between two distributions that quantifies the flow required to morph one distribution to another. It is defined as the L1 norm of the cumulative density functions, DEMD = \\CDF1 -CDF2\\1; and has successfully been used to quantify gene expression differences in single-cell data and microbiome data (39). Also, the EMD metric was used for imputed data via diffusion (41). Additionally, the EMD metric does not make parametric assumptions about their underlying distributions (41). The details of the mb-PHENIX software can be found in (https://github.com/resendislab/mb-PHENIX).
Linear discriminant analysis effect size (LEfSe) (RRID: SCR_014609), is a method that combines non-parametric Kruskal-Wallis and Wilcoxon rank sum test with linear discriminant analysis (LDA) (42). It was employed to detect the features in terms of bacterial genera to discriminate communities in each group (NG, IFG, IGT, IFG+IGT, T2D, and T2D treated). To run this analysis, we used the galaxy server from Hutlab (https://huttenhower.sph.harvard.edu/galaxy/) with the following parameters: 1) Alpha values for the factorial Kruskal-Wallis and pairwise Wilcoxon tests among classes were 0.05, 2) Threshold on the logarithmic LDA score for discriminative features was set to 2, and 3) Set the strategy for multi-class analysis was set to one against all.
Classical approaches to analyzing microbiomes are based on taxonomic profiling and ecological diversity studies (e.g., phyloseq, edgeR, krona). Thus, we started with these analyses to describe the GM of a Mexican T2D cohort. To disentangle the complexity of the GM structure and ecological interactions on the progress of T2D in a Mexican cohort, we used a multidimensional approach based on bioinformatics tools such as SparCC, Netshift, and mb-PHENIX.
Gut bacteria communities were highly diverse in all T2D samples, independently of their disease stage. The rarefaction curves (Figure S1 A-F) reach asymptotes in a range of 100-600 species (set size= 50) in all samples. We did not set up a threshold based on the lowest number of sequences found in a sample, because this would artificially mask the diversity of the community (43). As seen in the rarefaction curves, the NG patients had greater diversity across GM samples than the disease groups.
Related to GM taxonomy, the Krona graphs (https://github.com/resendislab/MEXT2D_Networks/tree/main/results) for NG patients showed that Firmicutes and Bacteroidetes ranged 40-80% and 20-60%, respectively. For IFG patients, Firmicutes ranged in 37-91% and 2-50% for Bacteroidetes. For IGT patients, Firmicutes ranged in 40-80% and 10-50% for Bacteroidetes. For IFG+IGT patients, Firmicutes ranged 30-70% and 10-35% for Bacteroidetes. For T2D patients, Firmicutes ranged from 40-60% and Bacteroidetes 5-30%. Lastly, T2D patients with treatment Firmicutes ranged 50-80% and Bacteroidetes 1-20%.
The Chao1, ACE, Shannon, and Fisher indexes (Figure S2) were calculated to estimate the α-diversity. No significant differences were found between the five groups, but IGT subjects showed a slightly increased diversity compared with all other stages. This result indicated that IGT patients had higher microbial diversity than in other stages. With this result, we can hypothesize that this diversity increase may be because of an internal feedback mechanism between the GM and its host as a last effort to stabilize the evolving microbial community due to the progression of T2D. This hypothesis is supported by the latest reports on the functional redundancy of microbial communities in the human gut and the holobiont theory as a framework of analysis for microbial communities associated with their host (44–46).
A previous study from our group reported that our Mexican T2D cohort had some degree of obesity (8, 27). For example, groups with IGT and T2D have the highest values of body mass index (BMI) compared with other groups such as NG and IFG (8). This behavior was also observed in other computational modeling studies (Flux Balance Analysis FBA) related to T2D cohorts (47).
To estimate β-diversity, we used non-phylogenetic methods such as Jaccard distances and Non-Metric Multidimensional Scaling (NMDS) plotting (48). The results showed undefined clustering patterns (Figure S3A). Moreover, we made an additional NMDS plot at phylum level to observe the differences among clustering groups. We observed the vast amplitude of members of Firmicutes phylum and reinforced the remarkable abundance of these members in the GM of humans (49) (Figure S3B). However, we did not observe clustering of the groups based on the T2D stage. Then, we established that more complex nonlinear (unsupervised dimensionality) reduction methods did not present separation patterns of samples based on T2D stages groups (Figure S5A UMAP). These results are likely due to technical noise, high-dimensionality, sparsity, and intrinsic ecological community heterogeneity (50). Therefore, it is necessary to use supervised methods to reveal the underlying topological information on these noisy and highly heterogeneous systems (38).
Numerous taxa were differentially abundant among T2D stages. Figure 2 displays log-2 fold change (logFC). The logFC can be interpreted as the log-base-2 ratio of relative abundance compared to the reference group. For example, Blautia was found to be 32 (25) times more abundant in IFG subjects compared with NG subjects, but in another comparison was found to be 36.75 (25.2) times less abundant in IGT subjects compared with IFG subjects (Figure 2).
Figure 2 LogFC differential abundance (or coefficient from edgeR log-linear models for each comparison group and all significant ASVs) of GM data of Mexican T2D patients. Colors refer to the phyla taxonomy level of the plotted genera.
Prevotella 9 genus is another example of notable variations along preT2D stages. It was 36.75 (22.5) more abundant in IFG patients compared with NG patients. Similar behavior was observed in other stages of preT2D, where it was 39.39 (25.3) more abundant in IGT patients compared with IFG and 5.27 (22.4) more abundant in T2D patients compared with IFG+IGT patients. However, it was 16 (24) less abundant in IFG+IGT subjects compared with IGT patients (Figure 2). Notably, this is the first report where a genus-level cluster of Prevotella 9 emerges in a T2D cohort.
Modeling interactions by association networks is an effective computational tool for analyzing the structure and stability of microbial communities. Also, possible keystone taxa (driver taxa) related to the dynamic equilibria of GM and its host can be inferred. We performed and analyzed association networks for each stage of T2D (i.e., NG, IFG, IGT, IFG+IGT, T2D, and T2D treated). Then, we used Netshift to identify the driver nodes in each case-control association as those accomplished in the differential abundance analysis (NG vs. IFG, IFG vs. IGT, etc.) (Figure S4A). In each comparison, we built and compared the network association for each state and obtained some topological parameters, such as the network density, cluster coefficient, and average path length. Together, they are called global graph properties because they provide insights into the overall organization of the network and enable the assessment of its modularity (35).
In a microbial community, density corresponds to the proportion of observed microbial associations (edges) out of all theoretically possible associations (all the nodes in the network). Therefore, a greater density value indicates higher crosstalk among the resident microbes represented in the network nodes (35). Moreover, network density shows how quickly perturbations may spread (51). Thus, the small network density (Figure S4A) indicated microbial communities composed of scarcely connected groups. This behavior was expected due to the scarce resilience of the system since a poorly connected network is less robust to changes than high-density networks (52).
Complementary, it has been suggested that communities with the modular organization of the type “small world” are more stable at facing perturbations. The modular arrangement allows different groups of nodes to perform different functions with some degree of independence (53). In this way, the clustering coefficient quantifies the tendency of the graph to be divided into subunits. In other words, a microbial network with a higher number of independent units of associated microbes is expected to have a higher clustering coefficient value (35). Our study showed slight changes in this parameter between comparisons, particularly IGT vs. IFG+IGT and IFG+IGT vs. T2D had remarkable changes (almost double the previous value) (Figure S4A).
Average path-length indicates the average number of steps that would be required to reach from one node to another in the network. This parameter represents to what extent the microbial community structure is compacted. In almost all cases, we detected an increase in the path length from 1.5 to 2 in IGT vs. IFG+IGT, from 1.2 to 1.5 in NG vs. IFG, and IFG+IGT vs. T2D, for example (Figure S4A). However, we detected a large change in the T2D vs. T2D treated. Based on the previous results, low density and lower average path length in the network indicated lower information transport which might suggest a decolonization activity in the GM.
Figure S4B showed that the number of total nodes decreased on every pairwise comparison (i.e., IFG vs. IGT, IGT vs. IFG_IGT, IFG_IGT vs. T2D, T2D vs. T2D treated), except in NG vs. IFG where only remains the same number of total nodes (Figure S4B). These results agree with our previous results, where we suggested a possible decolonization activity in the GM along with T2D development and progression.
Regarding the total number of edges, we detected several exciting patterns (Figure S4B). For example, we observed that the number of total edges decreased for NG vs. IFG, IFG vs. IGT, and IGT vs. IFG_IGT, while for IFG_IGT vs. T2D and T2D vs. T2D treated increased. As expected, the comparison of T2D vs. T2D treated had a remarkable difference as the GM composition changed within T2D subjects treated with antidiabetic drugs such as metformin (54). In terms of exclusive edges, we detected patterns such as NG vs. IFG, IFG_IGT vs. T2D, and T2D vs. T2D treated with an increase in this value. From the other side, we detected a decrease in terms of exclusive edges for IFG vs. IGT and IGT vs. IFG_IGT. This behavior is in agreement with our results of density and average path length (Figure S4A). Moreover, it was previously observed in some pathologies related to GM, such as IBD (55).
Although the exclusive edge count between two networks is an indicator of rewiring, it is also valuable to consider the Jaccard Edge Index (JEI) of the compared networks as it can quantify changes in the interacting partners for each node between two graphs (each T2D stage) (Figure S4C). According to Figure S4C and in the first stage of the disease, we observed in the pairwise comparisons, NG vs. IFG and IFG vs. IGT, the value of JEI is near to 1 and indicated that the edges in the association networks are practically the same. But when the disease evolved to other stages such as IGT, IFG_IGT, T2D, and T2D treated, JEI decreased dramatically to zero. These results can be interpreted as a remarkable change owing to a different stage of T2D disease (56). In short, all these topological properties of the association networks offer insights into the overall differences in the community structure between a pairwise comparison network.
In many diseases, a set of key microbial groups are likely to act as ‘drivers’ for facilitating several changes in the microbial community structure and hence become an essential factor for understanding the microbial basis of the disease (35, 55–59). With this idea in mind, we obtained the driver nodes for each pairwise comparison with the software Netshift (Figure 3). Figure 3 shows our results of driver nodes for the pairwise comparisons analyzed along with this study. These keystone nodes belong to several phyla: 1) Anaerococcus, Anaerostipes, Christensenellaceae, Dorea, Ezakiella, Erysipelotrichaceae, Roseburia, Terrisporobacter, and Ruminococcaceae belonging to Firmicutes phylum. 2) Alistipes belonging to Bacteroidetes phylum, and 3) Eggerthella belonging to Actinobacteria phylum. Our results fall into the most representative genera and phyla belonging to GM (49) and add more evidence to our previous hypothesis, which proposes that the changes in the human gut enterotypes are associated with some keystone or driver taxa along the different stages of preT2D and T2D in a Mexican cohort. Later, we prepared an Upset Plot graph with all the nodes obtained with SparCC and the driver nodes from NetShift to determine specific genera that belong to a particular group (Figure 4). As seen in Figure 4, Ruminococcaceae_NK4A214_group, Anaerostipes, Alistipes, Ruminococcaceae_UCG-002, Ruminococcaceae_UCG-005, Ruminococcaceae_UCG-010, and Terrisporobacter were the core community in all analyzed groups. This finding is highly relevant as it is the first microbial core reported for the GM of Mexicans with T2D.
Figure 3 Driver nodes were obtained with NetShift. Driver nodes were colored red, edges present in both states were colored with blue, edges colored with green were exclusive to control data, and edges colored with red were exclusive to case data. All comparisons are based on a control-case order. Heatmap of the key drivers shifts among T2D states based on the denoised data, the earth-mover distance (EMD) is used to quantify distribution shifts among the T2D states clusters.
Figure 4 Core nodes for the Mexican T2D cohort. Each node represents a node in the SparCC association networks. The red color indicates “driver nodes” obtained from NetShift Analysis. Below, the black dots represent the presence of the core in the corresponding study group (e.g. T2D treated has 70 unique genera along with the cohort).
To analyze in-depth all these data from networks, we compared the results of differentially abundant analysis of all groups in the cohort (Figure 2) with the results from NetShift (Figure 3) to get coincidences between these two approaches (Figure 5). Alistipes can be a potential SCFA producer, and their decrease contributes to the development of inflammatory diseases related to inadequate modulation of the immune system by SCFA in the gut (e.g., CRC, IBD, cardiovascular disease (CVD), etc.) (60, 61). We detected a remarkable effect of Alistipes through two different approaches: 1) Association networks with SparCC and Netshift analysis and 2) Differential Abundance for Microbiome Data with EdgeR. Based on these results, Alistipes seems to play a role in the development of preT2D (IFG) as with other inflammatory diseases such as IBD, CRC, CVD, and hypertension (62).
Figure 5 A multidimensional approach to interlace nodes information obtained with the differentially abundance tool (EdgeR) and driver nodes (Netshift). For both EdgeR and Netshift, the data was obtained from pairwise comparisons along with the cohort. NG vs IFG, IFG vs IGT, IGT vs IFG_IGT, IFG_IGT vs T2D and T2D vs T2D treated. *Note: In red were marked genera that had “driver node” characteristic from NetShift Analysis.
To reinforce the results obtained with Netshift about the taxa-drivers in each T2D stage (Figures 5, 6), we denoised microbiota data (ASV table) through mb-PHENIX to remark the potential driver nodes involved in the transition of T2D states (37). Specifically, we used this approach because data lacks a well-defined cluster structure (Figures S3A, B (NMDS), Figure S5A, (UMAP)) due to the high rate of missing information (38). Then, we quantified the differences of the recovered (denoised) taxa between different T2D stages with EMD (63). We observed statistically relevant changes in the abundance of a determined driver or keystone taxa (Figure 3 EMD Score) and confirmed our previous results with EdgeR, SparCC, and Netshift. Hence, we used several tools to prove the existence of keystone taxa pattern shifts among T2D stages. Figure S5B shows that the changes along the T2D stages were mediated by a group of microbial interactions independently of whether their abundance is significantly different or not in a specific T2D group (Figure S5C). Therefore, this work showed the complexity of intrinsic non-linearity and the potential taxa differentiating the different groups of T2D states.
Figure 6 LEfSe analysis results. (A) Taxonomic cladogram. Differences are represented by the color of the most abundant genera at each group (NG, IFG, IGT, IFG+IGT, T2D and T2D treated). The diameter of each circle is proportional to the taxon's abundance. (B) The linear discriminant analysis (LDA) value histogram of the GM at genus level and cohort groups (NG, IFG, IGT, IFG+IGT, T2D and T2D treated).
To further explore differences in the GM among our groups (NG, IFG, IGT, IFG+IGT, T2D, and T2D treated), we used LEfSe to recognize the specific altered bacterial phenotype at genus level. These differences are shown in Figure 6. For the T2D treated group, we detected four bacterial genera that changed significantly among the groups with LDA score log 10 > 2, Cetobacterium, Lactonifactor, Coprococcus_1, and Erysipelatoclostridium. For the IGT group, we detected two bacterial genera with the same LDA score, Parabacteroides and Fournierella, while for the NG and the IFG+IGT groups, we detected Intestinibacter and Prevotellaceae_UCG003, respectively.
T2D is an expanding global health problem closely linked to obesity, and hypertension (9). Changes in the structure and composition of GM in T2D patients represent a key step to understanding the GM dysbiosis that accompanies the progression of IR in T2D. In this way, the GM is a complex system that needs to be analyzed in a holistic way, with several approaches such as bioinformatics and systems biology altogether under the concept of medical ecology of the human gut microbiota.
In terms of ecological indexes (α and β), we detected some variations related to the weight of the patients and their group (i.e. NG, IFG, IGT, IFG+IGT, T2D, and T2D_treated). This phenomenon is probably connected with the role of GM in T2D and its related disorders, including obesity. In agreement with this observation, some studies in murine obesity models indicated that altered GM had an increased capacity to harvest energy from the diet (64, 65) and suggested that changes in the diversity of GM should be considered a contributing factor to the pathophysiology of obesity and T2D.
Furthermore, the keystone roles of Prevotella 9 and Blautia with T2D progression were remarkable. In the specific case of Blautia, several studies have reported similar roles in other human diseases: fewer Blautia in T2D/obesity patients, fewer in colorectal cancer (CRC) and Inflammatory bowel disease (IBD) (66). This is relevant because, as far as we know, this is the first report of differential abundance changes of Blautia in IFG and IGT. Specifically, Prevotella (Bacteroidetes) and Blautia (Firmicutes) are remarkable examples in this analysis, which both suggest a subject-specific microbiome type led to the concept of human gut enterotypes that may change among NG and T2D groups of our Mexican cohort (67, 68). Overall, the study of Prevotella and Blautia has been hampered by their intrinsic difficulty in cultivating In vitro (they are anaerobic) (66, 69).
Network analysis is a powerful tool to understand the structure and ecological functions of the GM in patients with T2D. In the Netshift analysis, we detected a core of genera present in our Mexican T2D cohort. This core captures several ecological insights that will be explained next. Ruminococcaceae_NK4A214_group genus has been related to fiber degradation (58). In a murine model, this genus was correlated with alleviating colitis in casein-fed mice (70). However, another study with rats showed this genus with positive correlations with levels of blood glucose, HOMA-IR, and lipopolysaccharides (LPS) (71). Also, other studies showed increased abundance in a cohort of obese adults with elevated fasting glucose levels subjected to an almond consumption diet; interestingly, this genus was identified as one of the principal drivers of microbiota-level changes in our cohort (56).
In agreement with our findings, Alistipes (SCFA producer) was also increased in a T2D Austrian Cohort (72). Several authors reported that high levels of Alistipes and low levels of Blautia were found in patients with T2D (73). Moreover, Alistipes abundance was increased in diabetic mice with a potential association with high sucrose and a high-fat diet (74, 75). Whereas, in hypertension, it seems that Alistipes contributes to inflammation and epithelium alterations as A. finegoldii had an increased number and functional genes in the high blood pressure cohort (62). These results indicate that the composition of the GM is closely related to the levels of blood glucose and pro-inflammatory cytokines, which cause low-grade inflammation and contribute to the T2D progression. In this scenery, IR could be a consequence of dysregulation of bacterial production of butyrate, SCFA, and other metabolites (73).
The genus Anaerostipes (SCFA producer) was found significantly decreased in T2D cases compared to controls in an African cohort, further, the GM of T2D patients had decreased butyrate-producing bacteria and consequently reduced butyrate production, previously associated with IR (76). Several studies showed that Anaerostipes could interact with other microbes with diverse catabolic capacities to produce lactate (77). To this extent, some species of Anaerostipes (e.g., A. caccae, A. rhamnosivorans, A. hadrus, and A. butyraticus) are not only able to use a broad range of carbohydrates but also lactate and acetate for butyrogenesis (78). Nevertheless, to disentangle the metabolic differences between species and strains, we need to obtain broad genomic information through shotgun metagenomics.
Following up with the core microorganisms, a study in obesity and fasting plasma insulin (FPI) status in Mexican children reported a negative association of obesity with Ruminococcaceae UCG-002 and a positive association between FPI and Ruminococcaceae UCG-002 (79). For Ruminococcaceae UCG-005, some authors reported a positive correlation with Christensenellaceae R-7-group genus abundances and HDL cholesterol but a negative correlation with triglyceride levels (21). As mentioned before, the genera Ruminococcaceae UCG-010 showed a positive correlation with the levels of blood glucose, HOMA-IR, and LPS in diabetic (T2D) rats (71). Bacterial members of this family predominantly utilize fibers and polysaccharides as energy substrates and are SCFA producers. SCFAs are linked to improved colonic health and are known mainly for their anti-inflammatory properties (80). However, the relevance of Ruminococcaceae to health outcomes has not been fully elucidated. Conflicting data show that these genera are both positively and negatively correlated with lipid metabolites such as VLDL and HDL and are also associated with higher BMI (81).
Terrisporobacter was related to inflammation and oxidative stress in a study of NG Danish young men. This genus decreased immediately after metformin treatment initiation and remained low throughout the intervention period (82). In a similar report with Egyptian patients, authors reported that Terrisporobacter and Turicibacter were significantly more abundant in the control group (NG) as compared to either Type 1 or Type 2 Diabetes groups (83).
Dorea also appeared as a core for patients in all stages of the disease (i.e., IFG, IGT, IFG_IGT, T2D, and T2D treated). A Spanish cross-sectional study reported that body weight, waist circumference, and BMI showed a positive association with Dorea formicigenerans and Dorea longicatena with increased abundances in the overweight/obese group. They proposed these species as microbiota biomarkers of obesity in the Spanish population (84). These results agree with another study in a Chinese population, where the abundance of Dorea was significantly increased in T2D individuals and negatively correlated with the abundance of butyrate-producing bacteria. The authors argued that increases in Dorea could play a role in the development of T2D (85). Supporting this, a cohort study of Danish patients with preT2D (IFG) and NG individuals reported a differentially significant increase of Dorea in the IFG patients versus NG individuals (10).
Roseburia is known to be a butyrate-producing genus, dependent on fermentable carbohydrates from the diet (82). In our results, Roseburia appeared as a core and driver node of all stages of T2D, except for the IGT group. Thus, it is plausible that Roseburia could affect T2D development. Still, its specific role is unclear since in our study appeared in the groups with T2D or preT2D and in other studies appeared as a health biomarker (6, 86).
Blautia is a particular case because it was not identified as a driver genus in Netshift but appeared as a core genus in the cohort. This behavior can be explained by its increase in disease groups in three of four cross-sectional studies of T2D and its reduction after bariatric surgery (6). Disagreeing with these reports, Blautia spp. was increased after metformin treatment (17). Notably, 87 results are concordant with our genus-level analysis, demonstrating positive associations between T2D and several OTUs of all three of these genera (87, 88).
In accordance with the LEfSe analysis, all identified bacteria belong to genera with previous reports linked to the progression of insulin resistance in T2D (Figure 6). However, Coprococcus_1, found in the T2D treated group, was already characterized by a high insulin sensitivity (β = 0.14; P = 0.002) and disposition index (β = 0.12; P = 0.012); for this reason, we hypothesized that its effect was mostly related to the changes in the constant metformin consumption in T2D patients (89). Furthermore, this is the first association of Fournierella with T2D patients and metformin treatment. Since this genus has reports with other low-grade inflammatory processes (i.e., obesity, insulin resistance, and others) that are part of the metabolic syndrome diagnostic (90–92). Another potential biomarker for the NG group was Intestinibacter genus. Early reports showed that a decrease in this bacteria abundance was described in patients with T2D (13).
According to our multidimensional analysis, Alistipes can be a potential biomarker of the development of T2D. Still, further studies will be necessary in the near future to better define the role of this genus when T2D is accompanied by other comorbidities (93). For instance, it has been reported that T2D patients have higher levels of depression incidence (94). There are several studies on mental diseases that reported an increase of Alistipes abundance (almost 4-fold) in Norwegian patients with chronic fatigue syndrome (95). These findings correspond with the evidence of an increase in T2D patients with depression, who typically struggle with fatigue and stress (96). An increase in Alistipes disrupts the gut-brain axis because it is an indole-positive organism and thus decreases serotonin availability. Tryptophan (indole ring) is a precursor of serotonin, and a decrease in serotonin is associated with depression. Moreover, Alistipes express glutamate decarboxylase in chickens, an enzyme that metabolizes glutamate into γ-aminobutyric acid (GABA). Therefore, an increase in Alistipes abundance can be related to a GABA increase with a potential link to depression (62). To confirm the role that Alistipes and other microorganisms have in comorbidities associated with T2D, additional studies should be addressed shortly.
We conclude that using bioinformatics, systems biology, and supervised machine learning tools, we obtained a complete ecological perspective of GM dysbiosis in a Mexican T2D cohort. Ruminococcaceae NK4A214 group, Ruminococcaceae UCG-010, Ruminococcaceae UCG-002, Ruminococcaceae UCG-005, Alistipes, Anaerostipes, and Terrisporobacter appear to have a distinctive ecological role in the preT2D group. However, more high throughput sequencing methods are needed to determine which species and strains are involved. Our arguments and results are important advances in the medical ecology of the human gut microbiota from a Mexican T2D cohort, as an effort to provide cutting-edge interventions for personalized medicine in the near future.
Raw sequencing data along with metadata is provided on the sequence read archive (SRA) under the Bioproject PRJNA541332 at https://www.ncbi.nlm.nih.gov/. The datasets generated and analyzed during the current study, and the customized scripts used in this study are available in the GitHub repositories: [https://github.com/resendislab/MEXT2D_Networks], [https://github.com/resendislab/mb-PHENIX],[https://github.com/resendislab/mext2d].
The studies involving human participants were reviewed and approved by Comité de Etica, Universidad de Guanajuato. The patients/participants provided their written informed consent to participate in this study.
DE-H and OR-A conceived and designed the study. DE-H executed the experiments, analyzed the data, performed statistical tests, and drafted/revised the manuscript. YL-M, JS-C, DN-R, and CP-M analyzed the data, performed statistical tests, and contributed to the design of the research. DG-V and CM-O performed statistical tests, executed complementation experiments, and contributed to the implementation of bioinformatics analysis. All authors contributed to the writing of the manuscript and approved the final version.
OR-A thanks the financial support from CONACYT (Grant Ciencia de Frontera 2019, FORDECYT-PRONACES/425859/2020), PAPIIT-UNAM (IA202720), and an internal grant from the National Institute of Genomic Medicine (INMEGEN, México).
DE-H is a postdoctoral research associate at INMEGEN and received a CONACyT fellowship to CVU 420693. YM-L is a doctoral student from the Doctor in Science program in Ciencias Médicas, Odontológicas y de la Salud by Universidad Nacional Autónoma de México (UNAM) and received CONACyT fellowship 629384. JPS-C is a student from the Master in Sciences program in Ciencias Bioquímicas, UNAM and received CONACyT fellowship to CVU 1005702. DN-R is a student from the Master in Sciences program in Ciencias Bioquímicas, UNAM and received CONACyT fellowship to CVU 1083211. CP-M is a doctoral student from the Doctor in Science program in Ciencias Biomédicas, UNAM and received CONACyT fellowship 855825. DG-V is a student from the Master in Sciences program in Ciencias Bioquímicas, UNAM and received CONACyT fellowship to CVU 1083058. CM-O is a student from the Master in Sciences program in Ciencias Bioquímicas, UNAM and received CONACyT fellowship to CVU 1103002.
The authors declare that the research was conducted in the absence of any commercial or financial relationships that could be construed as a potential conflict of interest.
All claims expressed in this article are solely those of the authors and do not necessarily represent those of their affiliated organizations, or those of the publisher, the editors and the reviewers. Any product that may be evaluated in this article, or claim that may be made by its manufacturer, is not guaranteed or endorsed by the publisher.
The Supplementary Material for this article can be found online at: https://www.frontiersin.org/articles/10.3389/fendo.2023.1128767/full#supplementary-material
1. Reed J, Bain S, Kanamarlapudi V. A review of current trends with type 2 diabetes epidemiology, aetiology, pathogenesis, treatments and future perspectives. Diabetes Metab Syndrome Obesity: Targets Ther Volume (2021) 14:3567–602. doi: 10.2147/dmso.s319895
2. Martínez-López YE, Esquivel-Hernández DA, Sánchez-Castañeda JP, Neri-Rosario D, Guardado-Mendoza R, Resendis-Antonio O. Type 2 diabetes, gut microbiome, and systems biology: A novel perspective for a new era. Gut Microbes (2022) 14(1):2111952. doi: 10.1080/19490976.2022.2111952
3. Dorcely B, Katz K, Jagannathan R, Chiang SS, Oluwadare B, Goldberg IJ, et al. Novel biomarkers for prediabetes, diabetes, and associated complications. Diabetes Metab syndrome obesity: Targets Ther (2017) 10:345. doi: 10.2147/DMSO.S100074
4. Wu H, Tremaroli V, Schmidt C, Lundqvist A, Olsson LM, Krämer M, et al. The gut microbiota in prediabetes and diabetes: A population-based cross-sectional study. Cell Metab (2020) 32(3):379–390.e373. doi: 10.1016/j.cmet.2020.06.011
5. Tuso P. Prediabetes and lifestyle modification: time to prevent a preventable disease. Permanente J (2014) 18(3):88. doi: 10.7812/TPP/14-002
6. Gurung M, Li Z, You H, Rodrigues R, Jump DB, Morgun A, et al. Role of gut microbiota in type 2 diabetes pathophysiology. EBioMedicine (2020) 51:102590. doi: 10.1016/j.ebiom.2019.11.051
7. Sharma S, Tripathi P. Gut microbiome and type 2 diabetes: where we are and where to go? J Nutr Biochem (2019) 63:101–8. doi: 10.1016/j.jnutbio.2018.10.003
8. Diener C, Reyes-Escogido M, Jimenez-Ceja LM, Matus M, Gomez-Navarro CM, Chu ND, et al. Progressive shifts in the gut microbiome reflect prediabetes and diabetes development in a treatment-naive Mexican cohort. Front Endocrinol (2021) 11:602326. doi: 10.3389/fendo.2020.602326
9. Boulangé CL, Neves AL, Chilloux J, Nicholson JK, Dumas M-E. Impact of the gut microbiota on inflammation, obesity, and metabolic disease. Genome Med (2016) 8(1):1–12. doi: 10.1186/s13073-016-0303-2
10. Allin KH, Tremaroli V, Caesar R, Jensen BA, Damgaard MT, Bahl MI, et al. Aberrant intestinal microbiota in individuals with prediabetes. Diabetologia (2018) 61(4):810–20. doi: 10.1007/s00125-018-4550-1
11. Qiu J, Zhou H, Jing Y, Dong C. Association between blood microbiome and type 2 diabetes mellitus: A nested case-control study. J Clin Lab Anal (2019) 33(4):e22842. doi: 10.1002/jcla.22842
12. Zhang Z, Tian T, Chen Z, Liu L, Luo T, Dai J. Characteristics of the gut microbiome in patients with prediabetes and type 2 diabetes. PeerJ (2021) 9:e10952. doi: 10.7717/peerj.10952
13. Chen Z, Radjabzadeh D, Chen L, Kurilshikov A, Kavousi M, Ahmadizar F, et al. Association of insulin resistance and type 2 diabetes with gut microbial diversity: A microbiome-wide analysis from population studies. JAMA network Open (2021) 4(7):e2118811–e2118811. doi: 10.1001/jamanetworkopen.2021.18811
14. Qian G, Ho JW. Challenges and emerging systems biology approaches to discover how the human gut microbiome impact host physiology. Biophys Rev (2020) 12(4):851–63. doi: 10.1007/s12551-020-00724-2
15. Jiang D, Armour CR, Hu C, Mei M, Tian C, Sharpton TJ, et al. Microbiome multi-omics network analysis: Statistical considerations, limitations, and opportunities. Front Genet (2019) 10:995. doi: 10.3389/fgene.2019.00995
16. Quévrain E, Maubert M, Michon C, Chain F, Marquant R, Tailhades J, et al. Identification of an anti-inflammatory protein from faecalibacterium prausnitzii, a commensal bacterium deficient in crohn’s disease. Gut (2016) 65(3):415–25. doi: 10.1136/gutjnl-2014-307649
17. Tong X, Xu J, Lian F, Yu X, Zhao Y, Xu L, et al. Structural alteration of gut microbiota during the amelioration of human type 2 diabetes with hyperlipidemia by metformin and a traditional Chinese herbal formula: A multicenter, randomized, open label clinical trial. MBio (2018) 9(3):e02392–02317. doi: 10.1128/mBio.02392-17
18. Ramaswami R, Bayer R, Galea S. Precision medicine from a public health perspective. Annu Rev Public Health (2018) 39:153–68. doi: 10.1146/annurev-publhealth-040617-014158
19. Pepper JW, Rosenfeld S. The emerging medical ecology of the human gut microbiome. Trends Ecol Evol (2012) 27(7):381–4. doi: 10.1016/j.tree.2012.03.002
20. Angulo MT, Moog CH, Liu Y-Y. A theoretical framework for controlling complex microbial communities. Nat Commun (2019) 10(1):1–12. doi: 10.1038/s41467-019-08890-y
21. Sommer AJ, Peters A, Rommel M, Cyrys J, Grallert H, Haller D, et al. A randomization-based causal inference framework for uncovering environmental exposure effects on human gut microbiota. PloS Comput Biol (2022) 18(5):e1010044. doi: 10.1371/journal.pcbi.1010044
22. Shields BM, Dennis JM, Angwin CD, Warren F, Henley WE, Farmer AJ, et al. Patient stratification for determining optimal second-line and third-line therapy for type 2 diabetes: The TriMaster study. Nat Med (2023) 29, 376–83. doi: 10.1038/s41591-022-02120-7
23. Van Ommen B, Wopereis S, Van Empelen P, Van Keulen HM, Otten W, Kasteleyn M, et al. From diabetes care to diabetes cure–the integration of systems biology, eHealth, and behavioral change. Front Endocrinol (2018) 8:381. doi: 10.3389/fendo.2017.00381
24. Ezzamouri B, Rosario D, Bidkhori G, Lee S, Uhlen M, Shoaie S. Metabolic modelling of the human gut microbiome in type 2 diabetes patients in response to metformin treatment. NPJ Syst Biol Appl (2023) 9(1):1–8. doi: 10.1038/s41540-022-00261-6
25. Ma ZS. Critical network structures and medical ecology mechanisms underlying human microbiome-associated diseases. Iscience (2020) 23(6):101195. doi: 10.1016/j.isci.2020.101195
26. Bayer G, Ganobis CM, Allen-Vercoe E, Philpott DJ. Defined gut microbial communities: Promising tools to understand and combat disease. Microbes Infection (2021) 23(6-7):104816. doi: 10.1016/j.micinf.2021.104816
27. Guardado-Mendoza R, Salazar-López SS, Álvarez-Canales M, Farfán-Vázquez D, Martínez-López YE, Jiménez-Ceja LM, et al. The combination of linagliptin, metformin and lifestyle modification to prevent type 2 diabetes (PRELLIM). a randomized clinical trial. Metabolism (2020) 104:154054. doi: 10.1016/j.metabol.2019.154054
28. Ondov BD, Bergman NH, Phillippy AM. Interactive metagenomic visualization in a web browser. BMC Bioinf (2011) 12(1):1–10. doi: 10.1186/1471-2105-12-385
29. Dixon P. VEGAN, a package of r functions for community ecology. J Vegetation Sci (2003) 14(6):927–30. doi: 10.1111/j.1654-1103.2003.tb02228.x
30. McMurdie PJ, Holmes S. Phyloseq: an r package for reproducible interactive analysis and graphics of microbiome census data. PloS One (2013) 8(4):e61217. doi: 10.1371/journal.pone.0061217
31. Robinson MD, McCarthy DJ, Smyth GK. edgeR: A bioconductor package for differential expression analysis of digital gene expression data. bioinformatics (2010) 26(1):139–40. doi: 10.1093/bioinformatics/btp616
32. Abdul-Ghani MA, DeFronzo RA. Pathophysiology of prediabetes. Curr Diabetes Rep (2009) 9(3):193–9. doi: 10.1007/s11892-009-0032-7
33. Friedman J, Alm EJ. Inferring correlation networks from genomic survey data. PLOS Computational Biology (2012) 8(9):e1002687. doi: 10.1371/journal.pcbi.1002687
34. Kassambara A. Network Analysis and Visualization in R: Quick Start Guide: Create Space Independent Publishing Platform. (2017a) Marseille Cedex 9 France. Available at: https://www.sthda.com/english/.
35. Kuntal BK, Chandrakar P, Sadhu S, Mande SS. ‘NetShift’: A methodology for understanding ‘driver microbes’ from healthy and disease microbiome datasets. ISME J (2019) 13(2):442–54. doi: 10.1038/s41396-018-0291-x
36. Lex A, Gehlenborg N, Strobelt H, Vuillemot R, Pfister H. UpSet: visualization of intersecting sets. IEEE Trans Visualization Comput Graphics (2014) 20(12):1983–92. doi: 10.1109/TVCG.2014.2346248
37. Padron-Manrique C, Vazquez-Jimenez A, Esquivel-Hernandez DA, Martinez-Lopez YE, Neri-Rosario D, Sanchez JP, et al. Mb-PHENIX: Diffusion and supervised uniform manifold approximation for denoising microbiota data. bioRxiv (2022). doi: 10.1101/2022.06.23.497285
38. Ghannam RB, Techtmann SM. Machine learning applications in microbial ecology, human microbiome studies, and environmental monitoring. Comput Struct Biotechnol J (2021) 19:1092–107. doi: 10.1016/j.csbj.2021.01.028
39. McClelland J, Koslicki D. EMDUniFrac: Exact linear time computation of the UniFrac metric and identification of differentially abundant organisms. J Math Biol (2018) 77(4):935–49. doi: 10.1007/s00285-018-1235-9
40. Levina E, Bickel P. The earth mover’s distance is the mallows distance: Some insights from statistics. In: Proceedings eighth IEEE international conference on computer vision. ICCV 2001. IEEE (2001) 2:251–6. doi: 10.1109/ICCV.2001.937632
41. Ramdas A, García Trillos N, Cuturi M. On wasserstein two-sample testing and related families of nonparametric tests. Entropy (2017) 19(2):47. doi: 10.3390/e19020047
42. Segata N, Izard J, Waldron L, Gevers D, Miropolsky L, Garrett WS, et al. Metagenomic biomarker discovery and explanation. Genome Biol (2011) 12:1–18. doi: 10.1186/gb-2011-12-6-r60
43. Knight R, Vrbanac A, Taylor BC, Aksenov A, Callewaert C, Debelius J, et al. Best practices for analysing microbiomes. Nat Rev Microbiol (2018) 16(7):410–22. doi: 10.1038/s41579-018-0029-9
44. Dethlefsen L, Eckburg PB, Bik EM, Relman DA. Assembly of the human intestinal microbiota. Trends Ecol Evol (2006) 21(9):517–23. doi: 10.1016/j.tree.2006.06.013
45. Moya A, Ferrer M. Functional redundancy-induced stability of gut microbiota subjected to disturbance. Trends Microbiol (2016) 24(5):402–13. doi: 10.1016/j.tim.2016.02.002
46. Van de Guchte M, Blottière HM, Doré J. Humans as holobionts: implications for prevention and therapy. Microbiome (2018) 6(1):1–6. doi: 10.1186/s40168-018-0466-8
47. Diener C, Gibbons SM, Resendis-Antonio O. MICOM: metagenome-scale modeling to infer metabolic interactions in the gut microbiota. Msystems (2020) 5(1). doi: 10.1128/mSystems.00606-19
48. Kuczynski J, Liu Z, Lozupone C, McDonald D, Fierer N, Knight R. Microbial community resemblance methods differ in their ability to detect biologically relevant patterns. Nat Methods (2010) 7(10):813–9. doi: 10.1038/nmeth.1499
49. Qin J, Li R, Raes J, Arumugam M, Burgdorf KS, Manichanh C, et al. A human gut microbial gene catalogue established by metagenomic sequencing. nature (2010) 464(7285):59–65. doi: 10.1038/nature08821
50. Pan AY. Statistical analysis of microbiome data: The challenge of sparsity. Curr Opin Endocrine Metab Res (2021) 19:35–40. doi: 10.1016/j.coemr.2021.05.005
51. Esquivel-Hernández DA, García-Pérez JS, Xu X, Metha S, Maldonado J, Xia S, et al. Microbial ecology in selenate-reducing biofilm communities: Rare biosphere and their interactions with abundant phylotypes. Biotechnol bioengineering (2021) 118(7):2460–71. doi: 10.1002/bit.27754
52. Sun MY, Dafforn KA, Johnston EL, Brown MV. Core sediment bacteria drive community response to anthropogenic contamination over multiple environmental gradients. Environ Microbiol (2013) 15(9):2517–31. doi: 10.1111/1462-2920.12133
53. Newman ME, Girvan M. Finding and evaluating community structure in networks. Phys Rev E (2004) 69(2):026113. doi: 10.1103/PhysRevE.69.026113
54. Lee H, Ko G. Effect of metformin on metabolic improvement and gut microbiota. Appl Environ Microbiol (2014) 80(19):5935–43. doi: 10.1128/AEM.01357-14
55. Greenblum S, Turnbaugh PJ, Borenstein E. Metagenomic systems biology of the human gut microbiome reveals topological shifts associated with obesity and inflammatory bowel disease. Proc Natl Acad Sci (2012) 109(2):594–9. doi: 10.1073/pnas.1116053109
56. Ley RE, Bäckhed F, Turnbaugh P, Lozupone CA, Knight RD, Gordon JI. Obesity alters gut microbial ecology. Proc Natl Acad Sci (2005) 102(31):11070–5. doi: 10.1073/pnas.0504978102
57. Trosvik P, de Muinck EJ. Ecology of bacteria in the human gastrointestinal tract–identification of keystone and foundation taxa. Microbiome (2015) 3(1):1–12. doi: 10.1186/s40168-015-0107-4
58. Baxter NT, Schmidt AW, Venkataraman A, Kim KS, Waldron C, Schmidt TM. Dynamics of human gut microbiota and short-chain fatty acids in response to dietary interventions with three fermentable fibers. MBio (2019) 10(1):e02566–02518. doi: 10.1128/mBio.02566-18
59. Gibbons SM. Keystone taxa indispensable for microbiome recovery. Nat Microbiol (2020) 5(9):1067–8. doi: 10.1038/s41564-020-0783-0
60. Radka CD, Frank MW, Rock CO, Yao J. Fatty acid activation and utilization by alistipes finegoldii, a representative bacteroidetes resident of the human gut microbiome. Mol Microbiol (2020) 113(4):807–25. doi: 10.1111/mmi.14445
61. Houtman TA, Eckermann HA, Smidt H, de Weerth C. Gut microbiota and BMI throughout childhood: the role of firmicutes, bacteroidetes, and short-chain fatty acid producers. Sci Rep (2022) 12(1):3140. doi: 10.1038/s41598-022-07176-6
62. Parker BJ, Wearsch PA, Veloo AC, Rodriguez-Palacios A. The genus alistipes: gut bacteria with emerging implications to inflammation, cancer, and mental health. Front Immunol (2020) 11:906. doi: 10.3389/fimmu.2020.00906
63. Czech L, Stamatakis A, Dunthorn M, Barbera P. Metagenomic analysis using phylogenetic placement–a review of the first decade. arXiv preprint arXiv:2202.03534. (2022) 2. doi: 10.3389/fbinf.2022.871393
64. Turnbaugh PJ, Ley RE, Mahowald MA, Magrini V, Mardis ER, Gordon JI. An obesity-associated gut microbiome with increased capacity for energy harvest. nature (2006) 444(7122):1027–31. doi: 10.1038/nature05414
65. Kashani A, Brejnrod AD, Jin C, Kern T, Madsen AN, Holm LA, et al. Impaired glucose metabolism and altered gut microbiome despite calorie restriction of ob/ob mice. Anim microbiome (2019) 1(1):1–16. doi: 10.1186/s42523-019-0007-1
66. Liu X, Mao B, Gu J, Wu J, Cui S, Wang G, et al. Blautia–a new functional genus with potential probiotic properties? Gut Microbes (2021) 13(1):1875796. doi: 10.1080/19490976.2021.1875796
67. Cani PD. Human gut microbiome: Hopes, threats and promises. Gut (2018) 67(9):1716–25. doi: 10.1136/gutjnl-2018-316723
68. Costea PI, Hildebrand F, Arumugam M, Bäckhed F, Blaser MJ, Bushman FD, et al. Enterotypes in the landscape of gut microbial community composition. Nat Microbiol (2018) 3(1):8–16. doi: 10.1038/s41564-017-0072-8
69. Ley RE. Prevotella in the gut: choose carefully. Nat Rev Gastroenterol Hepatol (2016) 13(2):69–70. doi: 10.1038/nrgastro.2016.4
70. Yu L, Zhao D, Nian Y, Li C. Casein-fed mice showed faster recovery from DSS-induced colitis than chicken-protein-fed mice. Food Funct (2021) 12(13):5806–20. doi: 10.1039/d1fo00659b
71. Zhang B, Sun W, Yu N, Sun J, Yu X, Li X, et al. Anti-diabetic effect of baicalein is associated with the modulation of gut microbiota in streptozotocin and high-fat-diet induced diabetic rats. J Funct Foods (2018) 46:256–67. doi: 10.1016/j.jff.2018.04.070
72. Remely M, Hippe B, Zanner J, Aumueller E, Brath H, G Haslberger A. Gut microbiota of obese, type 2 diabetic individuals is enriched in faecalibacterium prausnitzii, akkermansia muciniphila and peptostreptococcus anaerobius after weight loss. Endocrine Metab Immune Disorders-Drug Targets (Formerly Curr Drug Targets-Immune Endocrine Metab Disorders) (2016) 16(2):99–106. doi: 10.2174/1871530316666160831093813
73. Yuan X, Chen R, Zhang Y, Lin X, Yang X, McCormick KL. Gut microbiota of Chinese obese children and adolescents with and without insulin resistance. Front Endocrinol (2021) 12:636272. doi: 10.3389/fendo.2021.636272
74. Zhao C, Yang C, Chen M, Lv X, Liu B, Yi L, et al. Regulatory efficacy of brown seaweed lessonia nigrescens extract on the gene expression profile and intestinal microflora in type 2 diabetic mice. Mol Nutr Food Res (2018) 62(4):1700730. doi: 10.1002/mnfr.201700730
75. Wu C, Tian Y, Yu J, Zhang R, Zhang X, Guo P. The pandanus tectorius fruit extract (PTF) modulates the gut microbiota and exerts anti-hyperlipidaemic effects. Phytomedicine (2019) 58:152863. doi: 10.1016/j.phymed.2019.152863
76. Doumatey AP, Adeyemo A, Zhou J, Lei L, Adebamowo SN, Adebamowo C, et al. Gut microbiome profiles are associated with type 2 diabetes in urban africans. Front Cell infection Microbiol (2020) 63. doi: 10.3389/fcimb.2020.00063
77. Shetty SA, Boeren S, Bui TP, Smidt H, de Vos WM. Unravelling lactate-acetate and sugar conversion into butyrate by intestinal anaerobutyricum and anaerostipes species by comparative proteogenomics. Environ Microbiol (2020) 22(11):4863–75. doi: 10.1111/1462-2920.15269
78. Bui TPN, Mannerås-Holm L, Puschmann R, Wu H, Troise AD, Nijsse B, et al. Conversion of dietary inositol into propionate and acetate by commensal anaerostipes associates with host health. Nat Commun (2021) 12(1):1–16. doi: 10.1038/s41467-021-25081-w
79. Vazquez-Moreno M, Perez-Herrera A, Locia-Morales D, Dizzel S, Meyre D, Stearns JC, et al. Association of gut microbiome with fasting triglycerides, fasting insulin and obesity status in Mexican children. Pediatr Obes (2021) 16(5):e12748. doi: 10.1111/ijpo.12748
80. Shang Q, Shan X, Cai C, Hao J, Li G, Yu G. Dietary fucoidan modulates the gut microbiota in mice by increasing the abundance of lactobacillus and ruminococcaceae. Food Funct (2016) 7(7):3224–32. doi: 10.1039/C6FO00309E
81. Aslam H, Collier F, Davis JA, Quinn TP, O’Hely M, Pasco JA, et al. Gut microbiome diversity and composition are associated with habitual dairy intakes: A cross-sectional study in men. J Nutr (2021) 151(11):3400–12. doi: 10.1093/jn/nxab252
82. Bryrup T, Thomsen CW, Kern T, Allin KH, Brandslund I, Jørgensen NR, et al. Metformin-induced changes of the gut microbiota in healthy young men: results of a non-blinded, one-armed intervention study. Diabetologia (2019) 62(6):1024–35. doi: 10.1007/s00125-019-4848-7
83. Radwan S, Gilfillan D, Eklund B, Radwan HM, El Menofy NG, Lee J, et al. A comparative study of the gut microbiome in Egyptian patients with type I and type II diabetes. PloS One (2020) 15(9):e0238764. doi: 10.1371/journal.pone.0238764
84. Companys J, Gosalbes MJ, Pla-Pagà L, Calderón-Pérez L, Llauradó E, Pedret A, et al. Gut microbiota profile and its association with clinical variables and dietary intake in overweight/obese and lean subjects: a cross-sectional study. Nutrients (2021) 13(6):2032. doi: 10.3390/nu13062032
85. Li Q, Chang Y, Zhang K, Chen H, Tao S, Zhang Z. Implication of the gut microbiome composition of type 2 diabetic patients from northern China. Sci Rep (2020) 10(1):1–8. doi: 10.1038/s41598-020-62224-3
86. Tamanai-Shacoori Z, Smida I, Bousarghin L, Loreal O, Meuric V, Fong SB, et al. Roseburia spp.: a marker of health? Future Microbiol (2017) 12(2):157–70. doi: 10.2217/fmb-2016-0130
87. He Y, Wu W, Zheng H-M, Li P, McDonald D, Sheng H-F, et al. Regional variation limits applications of healthy gut microbiome reference ranges and disease models. Nat Med (2018) 24(10):1532–5. doi: 10.1038/s41591-018-0164-x
88. Al Bander Z, Nitert MD, Mousa A, Naderpoor N. The gut microbiota and inflammation: an overview. Int J Environ Res Public Health (2020) 17(20):7618. doi: 10.3390/ijerph17207618
89. Cui J, Ramesh G, Wu M, Jensen ET, Crago O, Bertoni AG, et al. Butyrate-producing bacteria and insulin homeostasis: The microbiome and insulin longitudinal evaluation study (MILES). Diabetes (2022) 71(11):2438–46. doi: 10.2337/db22-0168
90. Yang Y-CS, Chang H-W, Lin I, Chien L-N, Wu M-J, Liu Y-R, et al. Long-term proton pump inhibitor administration caused physiological and microbiota changes in rats. Sci Rep (2020) 10(1):1–11. doi: 10.1038/s41598-020-57612-8
91. Low D, Tee KX, Meldrum O, D’Agostino G, Kim HJ, Kang A, et al. Diet-associated variability in the elderly gut microbiome. Curr Developments Nutr (2022) 6(Supplement_1):1020–0. doi: 10.1093/cdn/nzac069.025
92. Taladrid D, de Celis M, Belda I, Bartolomé B, Moreno-Arribas MV. Hypertension-and glycaemia-lowering effects of a grape-pomace-derived seasoning in high-cardiovascular risk and healthy subjects. interplay with the gut microbiome. Food Funct (2022) 13(4):2068–82. doi: 10.1039/D1FO03942C
93. Subba R, Sandhir R, Singh SP, Mallick BN, Mondal AC. Pathophysiology linking depression and type 2 diabetes: psychotherapy, physical exercise, and fecal microbiome transplantation as damage control. Eur J Neurosci (2021) 53(8):2870–900. doi: 10.1111/ejn.15136
94. Farzi A, Hassan AM, Zenz G, Holzer P. Diabesity and mood disorders: Multiple links through the microbiota-gut-brain axis. Mol aspects Med (2019) 66:80–93. doi: 10.1016/j.mam.2018.11.003
95. Frémont M, Coomans D, Massart S, De Meirleir K. High-throughput 16S rRNA gene sequencing reveals alterations of intestinal microbiota in myalgic encephalomyelitis/chronic fatigue syndrome patients. Anaerobe (2013) 22:50–6. doi: 10.1016/j.anaerobe.2013.06.002
Keywords: gut microbiota, microbial ecology, systems biology, type 2 diabetes, network analysis
Citation: Esquivel-Hernández DA, Martínez-López YE, Sánchez-Castañeda JP, Neri-Rosario D, Padrón-Manrique C, Giron-Villalobos D, Mendoza-Ortíz C and Resendis-Antonio O (2023) A network perspective on the ecology of gut microbiota and progression of type 2 diabetes: Linkages to keystone taxa in a Mexican cohort. Front. Endocrinol. 14:1128767. doi: 10.3389/fendo.2023.1128767
Received: 21 December 2022; Accepted: 21 March 2023;
Published: 12 April 2023.
Edited by:
Ma. Cecilia Opazo, Universidad de Las Américas, ChileReviewed by:
Sidharth Prasad Mishra, University of South Florida, United StatesCopyright © 2023 Esquivel-Hernández, Martínez-López, Sánchez-Castañeda, Neri-Rosario, Padrón-Manrique, Giron-Villalobos, Mendoza-Ortíz and Resendis-Antonio. This is an open-access article distributed under the terms of the Creative Commons Attribution License (CC BY). The use, distribution or reproduction in other forums is permitted, provided the original author(s) and the copyright owner(s) are credited and that the original publication in this journal is cited, in accordance with accepted academic practice. No use, distribution or reproduction is permitted which does not comply with these terms.
*Correspondence: Osbaldo Resendis-Antonio, b3Jlc2VuZGlzQGlubWVnZW4uZ29iLm14
Disclaimer: All claims expressed in this article are solely those of the authors and do not necessarily represent those of their affiliated organizations, or those of the publisher, the editors and the reviewers. Any product that may be evaluated in this article or claim that may be made by its manufacturer is not guaranteed or endorsed by the publisher.
Research integrity at Frontiers
Learn more about the work of our research integrity team to safeguard the quality of each article we publish.