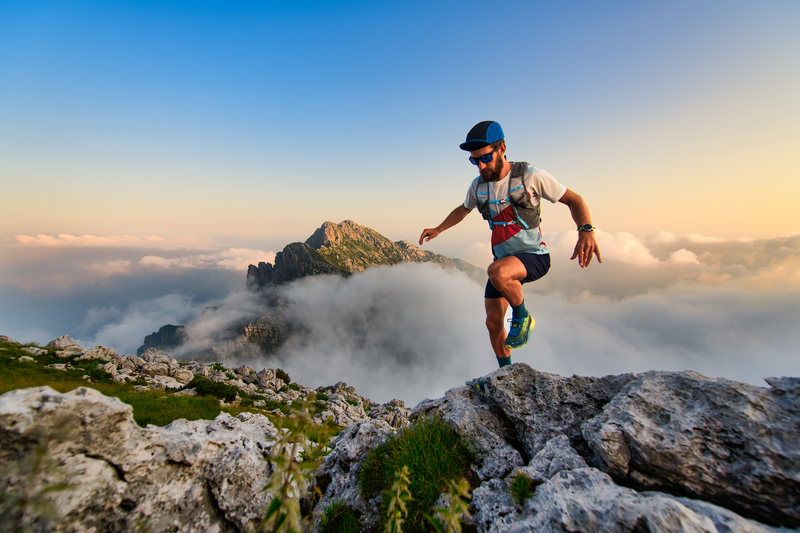
94% of researchers rate our articles as excellent or good
Learn more about the work of our research integrity team to safeguard the quality of each article we publish.
Find out more
ORIGINAL RESEARCH article
Front. Endocrinol. , 20 April 2023
Sec. Bone Research
Volume 14 - 2023 | https://doi.org/10.3389/fendo.2023.1125427
This article is part of the Research Topic Epilepsy and Endocrine Function View all 5 articles
Introduction: Osteoporosis (OP) is primarily diagnosed through bone mineral density (BMD) measurements, and it often leads to fracture. Observational studies suggest that several mental diseases (MDs) may be linked to OP, but the causal direction of these associations remain unclear. This study aims to explore the potential causal association between five MDs (Schizophrenia, Depression, Alzheimer's disease, Parkinson's disease, and Epilepsy) and the risk of OP.
Methods: First, single-nucleotide polymorphisms (SNPs) were filtered from summary-level genome-wide association studies using quality control measures. Subsequently, we employed two-sample Mendelian randomization (MR) analysis to indirectly analyze the causal effect of MDs on the risk of OP through bone mineral density (in total body, femoral neck, lumbar spine, forearm, and heel) and fractures (in leg, arm, heel, spine, and osteoporotic fractures). Lastly, the causal effect of the MDs on the risk of OP was evaluated directly through OP. MR analysis was performed using several methods, including inverse variance weighting (IVW)-random effects, IVW-fixed effects, maximum likelihood, weighted median, MR-Egger regression, and penalized weighted median.
Results: The results did not show any evidence of a causal relationship between MDs and the risk of OP (with almost all P values > 0.05). The robustness of the above results was proved to be good.
Discussion: In conclusion, this study did not find evidence supporting the claim that MDs have a definitive impact on the risk of OP, which contradicts many existing observational reports. Further studies are needed to determine the potential mechanisms of the associations observed in observational studies.
Osteoporosis (OP) is the most common systemic bone disease, characterized by a decrease in bone mineral density (BMD) and brittle fractures caused by deterioration of bone microstructure (1), which can easily lead to disability or even death in elderly patients (2). The standard diagnostic method for OP involves measuring BMD through dual-energy x-ray absorptiometry at the same skeletal site from childhood to old age. The femoral neck, lumbar spine, and forearm are the most commonly used skeletal sites for diagnosing OP (3, 4). Recently, the heel site has also been used to estimate OP (5). Moreover, total body BMD (TB-BMD) measurement is also an appropriate method for an unbiased assessment of BMD. Fractures are another feature of OP (6–8), with the leg, arm, heel, and spine being the most representative. According to the latest report of the International Osteoporosis Foundation, one in three women and one in five men over the age of 50 will experience OP worldwide (6–9). This disease not only impacts the patient’s quality of life but also poses a significant burden on public health and the national economy.
Mental diseases (MDs) are becoming increasingly prevalent in modern populations and can be classified into primary and secondary psychosis. Primary psychosis includes schizophrenia (SCH), depression (MDD), mood disorders, split personality, and other related conditions. Secondary psychosis, on the other hand, is caused by somatic organ diseases, neurological diseases, or substance abuse, and includes conditions such as Alzheimer’s disease (AD), stroke, Parkinson’s disease (PD), and epilepsy (EP) (10). As chronic diseases, MDs have been linked to abnormal bone metabolism, with patients suffering from some of the most common psychiatric disorders such as SCH (9), MDD (11), AD (12), PD (13), or EP (14) being more likely to have lower BMD and fracture risk, including OP, compared to the general population. However, most of these reports are observational in nature and may be subject to confounding factors, making it difficult to establish a definitive etiological link between MDs and OP.
Mendelian randomization (MR) analysis, the mimic design of randomized control trials, is an epidemiological research method that uses genetic variants (typically single-nucleotide polymorphisms, SNPs) to assess the causal association between modifiable exposures (or risk factors) and outcome (15, 16). MR analysis has advantages over clinical trials in terms of financial resources, material resources, and time. It is extensively applied in various studies (17, 18), particularly in research related to COVID-19 (19–21).
The aim of this study is to explore the potential causal relationship between MDs and the risk of OP by leveraging genetic variation through the use of two-sample MR analysis (22). Our investigation seeks to contribute novel insights and empirical evidence to the field of research on the association between MDs and OP.
In this study, we estimated the causal effect of MDs on BMD using the five genome-wide association studies (GWAS) summary data (TB-BMD; femoral neck-BMD, FN-BMD; lumbar spine-BMD, LS-BMD; forearm-BMD, FA-BMD; and heel-BMD, eBMD), as well as the causal effect of MDs on fracture using an additional five GWAS summary data (leg fracture, LF; arm fracture, AF; heel fracture, HF; spine fracture, SF; and osteoporotic fractures, OPF). These data indirectly evaluated the causal impact of MDs on the risk of OP via MR analysis. At the end of those parts, we directly assessed the causal effect of MDs on the risk of OP by utilizing GWAS summary data of OP.
GWAS summary statistics for BMDs were downloaded from the GEnetic Factors for osteoporosis Consortium website (GEFOS, http://www.gefos.org/). GWAS summary statistics for fracture were downloaded from the Medical Research Council Integrative Epidemiology Unit website (MRC-IEU, http://www.bristol.ac.uk/integrative-epidemiology/). GWAS summary statistics for OPF and OP were downloaded from the FinnGen website (https://www.finngen.fi/en/access_results). In addition, GWAS summary statistics for MDs, BMD, fractures, and OP were downloaded from the GWAS catalog website (https://www.ebi.ac.uk/gwas/downloads/summary-statistics). All study participants were of European descent. More detailed information can be found in Table 1.
Table 1 The detailed data information of the Mendelian randomization study on the association of MDs with the risk of OP.
To derive a reliable and valid inference regarding the correlation between MDs and OP, we opted for the most substantial GWAS database of MDs, encompassing SCH (N = 35,476 cases; 46,839 controls) (23) from the Psychiatric Genomics Consortium, MDD (N = 113,769 cases; 208,811 controls) (24) from the UK Biobank Psychiatric Genetics Group, AD (N = 25,580 cases; 48,466 controls) (25) from the French National Foundation on Alzheimer’s Disease and Related Disorder, PD (N = 1,713 cases; 3,978 controls) (26) from US National Institute of Neurological Disorders and Stroke (NINDS), and EP (N = 3,769 cases; 29,677 controls) (27) from The International League Against Epilepsy Consortium on Complex Epilepsies.
The GWAS database of TB-BMD (N = 66,628), FN-BMD (N = 32,735), LS-BMD (N = 28,498), and FA-BMD (N = 8,143) were downloaded from GEFOS (28, 29). Five separate GWAS summary statistics of eBMD (N = 265,627), AF (N = 4,714 cases; 455,626 controls), SF (N = 1,036 cases; 459,304 controls), LF (N = 2,988 cases; 457,352 controls), and HF (N = 306,379) were downloaded from MRC-IEU. The data of separate GWAS summary statistics of OPF (N = 173,619) and OP (N = 3,203 cases; 209,575 controls) were downloaded from the FinnGen consortium.
We employed stringent criteria to select SNPs as the genetic instrumental variables from the GWAS summary data of MDs, including SCH, MDD, AD, PD, and EP. Initially, SNPs with genome-wide significance (p < 5 × 10−8, R2 < 0.001, kb = 10,000) of MDs were selected. Subsequently, the clumping process (R2 > 0.001, window size = 10,000 kb) was executed to ensure that all the SNPs were not in linkage disequilibrium (LD) with the clump data function. Thirdly, if an SNP was not present in the outcome GWAS during the R calculation process, it would also be excluded. Fourthly, any ambiguous or palindromic SNPs that were ambiguous with non-concordant alleles (e.g., A/G vs. A/C) or with an ambiguous strand (i.e., A/T or G/C) were excluded. Finally, using the PhenoScanner tool (http://www.phenoscanner.medschl.cam.ac.uk/) (30–32), we excluded any SNPs associated with the confounding factor of the outcome, and we used the F-statistic to indicate the strength of the genetic instrumental variants.
The instrumental SNPs were utilized to carry out a two-sample MR analysis for the purpose of evaluating the causal effect of MDs on the risk of OP. The summary statistics (OR and standard error) of BMD and fracture enabled the indirect assessment of the causal association between MDs and the risk of OP, whereas the summary statistics of OP facilitated a direct evaluation. Detailed methods of MR analysis included inverse variance weighting (IVW)-random effects, IVW-fixed meta-analysis, maximum likelihood, weighted median (WM), and MR-Egger regression, and penalized weighted median was applied to estimate the effects. Bonferroni correction (p-value = 0.05/11 outcomes) was used to adjust for multiple testing (p = 0.0045) in this MR. All of these analyses were conducted in R V.4.2.0 by using R packages of “Two-Sample MR” (https://mrcieu.github.io/TwoSampleMR/reference/clump_data.html) (33) and p-values < 0.05 were considered statistically significant. The detailed steps are shown in the flowchart (Figure 1).
Figure 1 A step-by-step illustration of the study design and workflow. SNP, single-nucleotide polymorphism; MDs, mental diseases; SCH, schizophrenia; MDD, depression; AD, Alzheimer’s disease; PD, Parkinson’s disease; EP, epilepsy; GWAS, genome-wide association studies; BMD, bone mineral density; MR analysis, Mendelian randomization analysis; IVX, inverse variance weighting.
IVW (random effect and fixed effect) and MR Egger regression were used to assess the potential horizontal pleiotropic effects of the SNPs. Cochran Q-test statistics were used to quantify heterogeneities. Furthermore, we performed a “leave-one-out” sensitivity analysis to identify potentially influential SNPs. In this method, we excluded each SNP in turn and checked whether it was responsible for the association. We also applied the MR Steiger filtering method to verify the causality between MDs and OP.
We obtained 83 SNPs in SHC, 14 SNPs in MDD, 20 SNPs in AD, 23 SNPs in PD, and 11 SNPs in EP, which met the generally accepted genome-wide significance threshold (p < 5 × 10−8, r2 < 0.001, kb = 10,000) for exposure. Some SNPs strongly associated with confounding factors such as alcohol (34), glucocorticoid use (35), BMD (3, 4), and age (36) were eliminated. The removed SNPs are as follows: seven SNPs (rs11210892, rs2909457, rs2851447, rs13217619, rs7405404, rs4129585, and rs12887734) in SHC, one SNP (rs9530139) in MDD, two SNPs (rs10838725 and rs983392) in AD, two SNPs (rs35265698 and rs58879558) in PD, and one SNP (rs1402398) in EP. Moreover, all F-statistics > 10 indicated no weak instrument bias, the Bonferroni test verified the multiple testing p-value precision (p-value > 0.0045), and the directionality test conducted by MR Steiger confirmed our estimation of potential causal direction (p < 0.001). The detailed information is listed in Supplementary Material 1.
The MR analysis did not reveal any significant causal association between MDs and the risk of OP, whether analyzed indirectly from the causal effect of MDs on BMD (TB-BMD, FN-BMD, LS-BMD, FA-BMD, and eBMD) and fractures (LF, AF, SF, HF, and OPF), or directly from the causal effect of the MDs on OP (all p > 0.05) (Figure 2). The other methods including IVW-fixed effects, maximum likelihood, WM, MR-Egger regression, and penalized weighted median also verified that there was no significant association between MDs and the risk of OP (all p > 0.05). The results of those verified methods were consistent with the result in the MR analysis (Supplementary Material 2). The effect size of each SNP on the BMD, fracture, and OP is shown in Supplementary Material 3-7.
Figure 2 Associations of genetically predicted MDs with the risk of OP. The Forest plot of causal relationship between MDs and the risk of OP using the IVX. All p-values > 0.05 in the IVW random method. OR, odds ratio; IVX, inverse variance weighting; CI, confidence interval.
The MR analysis presented in Supplementary Material 2 estimated the causal effect of SCH on the risk of OP through indirect means such as BMD and fractures, as well as direct means such as OP. In the primary IVW analyses, SCH showed no MR association with BMD [FA-BMD: OR (95% CI) 1.002 (0.942–1.066), p = 0.944; LS-BMD: OR (95% CI) 1.019 (0.982–1.057), p = 0.327; FN-BMD: OR (95% CI) 1.002 (0.972–1.033), p = 0.913; eBMD: OR (95% CI) 1.002 (0.987–1.018), p = 0.765; and TB-BMD: OR (95% CI) 1.020 (0.993–1.047), p = 0.152], fracture [AF: OR (95% CI) 1.000 (0.999–1.001), p = 0.636; SF: OR (95% CI) 1.000 (0.999–1.001), p = 0.983; LF: OR (95% CI) 1.000 (1.000–1.001), p = 0.513; HF: OR (95% CI) 1.000 (1.000–1.001), p = 0.348; and OPF: OR (95% CI) 1.010 (0.826–1.237), p = 0.920], and OP (OP: OR (95% CI) 1.029 (0.919–1.152), p = 0.622] (Figure 2). These results were corroborated by other methods, indicating that SCH had no MR association with the risk of OP through either indirect (BMD and fractures) or direct (OP) pathways, with all p-values greater than 0.05 (Supplemental Materials 2, 3).
The MR analysis conducted to estimate the causal effect of MDD on the risk of OP is outlined in Supplementary Material 2. In the primary IVW analyses, MDD showed no MR association with BMD [FA-BMD: OR (95% CI) 2.108 (0.473–9.395), p = 0.328; LS-BMD: OR (95% CI) 0.988 (0.484–2.017), p = 0.975; FN-BMD: OR (95% CI) 1.023 (0.557–1.879), p = 0.941; eBMD: OR (95% CI) 1.031 (0.636–1.671), p = 0.902; and TB-BMD: OR (95% CI) 0.844 (0.474–1.504), p = 0.565], fracture [AF: OR (95% CI) 1.012 (0.990–1.034), p = 0.298; SF: OR (95% CI) 1.012 (0.997–1.026), p = 0.120; LF: OR (95% CI) 1.007 (0.994–1.021), p = 0.277; HF: OR (95% CI) 1.010 (0.997–1.024), p = 0.142; and OPF: OR (95% CI) 3.078 (0.039–243.226), p = 0.614], and OP [OP: OR (95% CI) 2.121 (0.280–16.084), p = 0.476] (Figure 2). Additional methods also confirmed that MDD was not associated with the risk of OP using both indirect (BMD and fractures) and direct (OP) approaches (all p > 0.05) (Supplementary Material 2, 4).
Supplementary Material 2, which includes MR estimates obtained from various methods of assessing the causal effect of AD on the risk of OP, indicated that AD demonstrated no association with the risk of OP. In the primary IVW analyses, AD exhibited no MR association with BMD [FA-BMD: OR (95% CI) 1.012 (0.931–1.100), p = 0.777; LS-BMD: OR (95% CI) 1.016 (0.967–1.067), p = 0.538; FN-BMD: OR (95% CI) 1.020 (0.980–1.060), p = 0.334; eBMD: OR (95% CI) 1.013 (0.997–1.029), p = 0.109, and TB-BMD: OR (95% CI) 0.975 (0.943–1.008), p = 0.140], fracture [AF: OR (95% CI) 1.000 (0.999–1.001), p = 0.371; SF: OR (95% CI) 1.000 (0.999–1.001), p = 0.854; LF: OR (95% CI) 1.000 (0.999–1.001), p = 0.487; HF: OR (95% CI) 1.000 (1.000–1.001), p = 0.361; and OPF: OR (95% CI) 1.214 (0.950–1.552), p = 0.121], and OP [OP: OR (95% CI) 0.918 (0.805–1.047), p = 0.201] (Figure 2). The results from other methods also supported the null association between AD and the risk of OP (all p > 0.05) (Supplementary Material 2, 5).
In the primary IVW analyses, PD was found to have no association with the risk of OP. In terms of its indirect aspect, PD was also found to have no MR association with BMD [FA-BMD: OR (95% CI) 1.013 (0.954–1.075), p = 0.673; LS-BMD: OR (95% CI) 1.034 (0.998–1.071), p = 0.067; FN-BMD: OR (95% CI) 1.011 (0.982–1.041), p = 0.462; eBMD: OR (95% CI) 1.009 (0.997–1.022), p = 0.154; and TB-BMD: OR (95% CI) 1.010 (0.990–1.031), p = 0.321] and fracture [AF: OR (95% CI) 1.000 (0.999–1.000), p = 0.353; SF: OR (95% CI) 1.000 (0.999–1.000), p = 0.867; LF: OR (95% CI) 1.000 (0.999–1.000), p = 0.930; HF: OR (95% CI) 1.000 (0.999–1.000), p = 0.726; and OPF: OR (95% CI) 1.056 (0.871–1.280), p = 0.580]. In the direct aspect, PD showed no MR association with OP [OP: OR (95% CI) 1.081 (0.982–1.189), p = 0.112] (Figure 2). Additional methods also demonstrated that PD had no MR association with the risk of OP (all p > 0.05) (Supplementary Material 2, 6).
We estimated the EP’s causal effect on the risk of OP. In the primary IVW analyses, EP showed no MR association with BMD [FA-BMD: OR (95% CI) 1.106 (0.957–1.277), p = 0.171; LS-BMD: OR (95% CI) 0.971 (0.897–1.053), p = 0.480; FN-BMD: OR (95% CI) 0.973 (0.908–1.042), p = 0.430; eBMD: OR (95% CI) 1.016 (0.987–1.045), p = 0.291; and TB-BMD: OR (95% CI) 0.990 (0.924–1.060), p = 0.765], fracture [AF: OR (95% CI) 1.000 (0.998–1.001), p = 0.631; SF: OR (95% CI) 1.000 (0.998–1.002), p = 0.868; LF: OR (95% CI) 1.001 (0.999–1.002), p = 0.283; HF: OR (95% CI) 0.999 (0.998–1.001), p = 0.342; and OPF: OR (95% CI) 2.260 (1.141–4.477), p = 0.137], and OP [OP: OR (95% CI) 1.238 (0.935–1.641), p = 0.136] (Figure 2). Other methods also indicated that EP had no MR association with the risk of OP (all p > 0.05) (Supplementary Material 2, 7).
Cochran’s Q-test did not reveal any sign of heterogeneity during the sensitivity analyses (all p > 0.05) (Supplementary Material 8). The MR-Egger regression method examined the possibility of horizontal pleiotropy between SNPs and outcome, and the results indicated no evidence of such pleiotropy (all p > 0.05) (Supplementary Material 8). Additionally, the funnel plots suggested no observable horizontal pleiotropy for any of the outcomes (Supplementary Material 3-7). Furthermore, the leave-one-out sensitivity analysis plots demonstrated that no single SNP was likely to have influenced the causal association and that our conclusions were therefore robust (Supplementary Material 3-7). Taken together, all of the findings suggest that the null association between genetic predisposition to MDs and the risk of OP was not significantly impacted by any individual SNP (Supplementary Material 3-7).
To our knowledge, this is the first study to evaluate the causal association between five kinds of MDs and the risk of OP using two-sample MR analysis. This study extensively mined the largest database, GWAS, and other relevant databases to investigate the causal association between the five most prevalent MDs (SCH, MDD, AD, PD, and EP) and the risk of OP, as well as its clinical manifestations (BMD and fracture). Our findings suggest that there is no clear causal relationship between mental disorders and the risk of OP.
Existing reports are limited to observational studies. These studies have consistently found that MDs increase the risk of OP (11, 13, 37–39). Specifically, Gomez and Stubbs discovered that individuals with SCH and MDD tend to have lower BMD at the hip and lumbar spine compared to healthy individuals (37, 40). Liu et al. studied PD and observed that PD patients have lower BMD at the total body, arm, and femur neck (13). Zhao et al. investigated the link between MDs and OP, and found that individuals with AD have a greater propensity for hip fractures (38). It is important to note, however, that these were all observational studies.
The aforementioned findings are in contrast to our own results, which may be attributed to the limitations of observational studies. Firstly, the studies cited were all based on case–control and cross-sectional designs, leaving it uncertain whether MDs are prospectively linked to an increased risk of OP. Secondly, many patients with MDs take long-term medications such as corticosteroids, which could potentially introduce bias in the results. Thirdly, some of the original studies lacked access to raw data, which could affect the accuracy of the findings. Lastly, certain assessment criteria may lower the reliability of the results, and BMD and fracture risk of multiple body parts should be taken into account in the investigation.
Due to the limitations of conventional observational study (41), we used “two-sample MR analysis” (16, 42) to ascertain the causal effects between MDs and the risk of OP, proceeding from the indirect aspect (BMD and fracture) to the direct aspect (OP). As we all know, MDs are frequently accompanied by other illnesses and substance abuse, resulting in the traditional misconception that psychiatric disorders can result in OP. However, which returns to the genetic level of analysis, indicates that MDs cannot lead to OP directly. Meanwhile, the randomness and fixedness of alleles preclude the reverse causation bias (43). The use of heterogeneity and sensitivity analysis in our analysis, with various Mendelian tools, augments the result’s stability, and the extensive sample size and singular population distribution diminish the bias of population stratification, ensuring an accurate causal effect between MDs and the risk of OP.
Undoubtedly, this study is not exempt from some constraints. First and foremost, the study’s participants are exclusively of European descent, necessitating further data collection and analysis to determine whether the findings apply to other populations. Second, the absence of publicly available source data precludes us from determining the potential sample overlap bias. Finally, even though we took measures to eliminate any confounding factors, we cannot fully rule out the potential influence of horizontal pleiotropy on our findings.
Moreover, given the constraints of our current research, our findings should be viewed as preliminary and warrant further investigation. Additionally, given the intricate nature of confounding factors, it is still advisable to consider various intervention methods and prevention strategies (44) for patients with MDs. These methods could include modifications to their lifestyle, such as promoting physical activity and improving their diet and exercise regime, increasing their intake of calcium and vitamin D (45), and minimizing the risk of falls.
The results of this study did not provide conclusive evidence to support the notion that MDs (including SCH, MDD, AD, PD, and EP) have a direct causal effect on the risk of OP. This is in contrast to the findings of numerous observational studies. It is possible that the relationship between MDs and OP risk reported in these observational studies is confounded by other risk factors. With the availability of more sophisticated approaches and a larger sample size of OP patients, the estimates can become less biased and the results can be more accurate. It is important to acknowledge that further research is needed to fully understand the relationship between MDs and OP risk.
The original contributions presented in the study are included in the article/Supplementary Material. Further inquiries can be directed to the corresponding authors. The R code to perform the MR analysis is detailed in the presentation 1 (Supplementary Material 9).
This study used publicly available GWAS summary databases. Thus, no ethical committee approval was required.
XD, FT, DX, SW, and HZ conceived the idea for the study. FT obtained the genetic data and performed the data analyses. FT, DX, SW, and HZ interpreted the results of the data analyses. All authors contributed to the article and approved the submitted version.
This work was supported by the National Key R&D Program of China (No. 2021YFC2100201).
The authors acknowledged PGC (the Psychiatric Genomics Consortium), UK Biobank (the UK Biobank Psychiatric Genetics Group), IGAP (the French National Foundation on Alzheimer’s Disease and Related Disorder), LNG [US National Institute of Neurological Disorders and Stroke (NINDS) Human Genetics Resource Center DNA and Cell Line Repository], ILNE (The International League Against Epilepsy Consortium on Complex Epilepsies), GEFOS (the Genetic Factors for osteoporosis Consortium), MRC-IEU (The Medical Research Council Integrative Epidemiology Unit), and The FinnGen consortium for contributing the data used in this work. We thank all the genetics consortiums for making the GWAS summary data publicly available, and we also thank the guidance and help from Dr. Kaiwen Wu and Dr. Ke Bi.
The authors declare that the research was conducted in the absence of any commercial or financial relationships that could be construed as a potential conflict of interest.
All claims expressed in this article are solely those of the authors and do not necessarily represent those of their affiliated organizations, or those of the publisher, the editors and the reviewers. Any product that may be evaluated in this article, or claim that may be made by its manufacturer, is not guaranteed or endorsed by the publisher.
The Supplementary Material for this article can be found online at: https://www.frontiersin.org/articles/10.3389/fendo.2023.1125427/full#supplementary-material
Supplementary Material 1 | Instrumental variables SNPs.
Supplementary Material 2 | Other methods to assess the link of MDs with the risk of OP.
Supplementary Material 8 | Heterogeneity, level pleiotropy test and the power of Mendelian randomization analyses.
Supplementary Material 9 | The detailed R code perform the MR analysis.
1. Kanis JA, Melton LJ, Christiansen 3C, Johnston CC, Khaltaev N. The diagnosis of osteoporosis. J Bone Miner Res (1994) 9:1137–41. doi: 10.1002/jbmr.5650090802
2. Borgstrom F, Karlsson L, Ortsater G, Norton N, Halbout P, Cooper C, et al. Fragility fractures in Europe: burden, management and opportunities. Arch Osteoporos (2020) 15:59. doi: 10.1007/s11657-020-0706-y
3. Kanis JA. Diagnosis of osteoporosis and assessment of fracture risk. Lancet (2002) 359:1929–36. doi: 10.1016/S0140-6736(02)08761-5
4. Kanis JA. Diagnosis of osteoporosis. Osteoporos Int (1997) 7(Suppl 3):S108–16. doi: 10.1007/BF03194355
5. Bai WY, Wang L, Ying ZM, Hu B, Xu L, Zhang GQ, et al. Identification of PIEZO1 polymorphisms for human bone mineral density. Bone (2020) 133:115247. doi: 10.1016/j.bone.2020.115247
6. Liu J, Curtis EM, Cooper C, Harvey NC. State of the art in osteoporosis risk assessment and treatment. J Endocrinol Invest (2019) 42:1149–64. doi: 10.1007/s40618-019-01041-6
7. Black DM, Geiger EJ, Eastell R, Vittinghoff E, Li BH, Ryan DS, et al. Atypical femur fracture risk versus fragility fracture prevention with bisphosphonates. N Engl J Med (2020) 383:743–53. doi: 10.1056/NEJMoa1916525
8. Leder BZ, Mitlak B, Hu MY, Hattersley G, Bockman RS. Effect of abaloparatide vs alendronate on fracture risk reduction in postmenopausal women with osteoporosis. J Clin Endocrinol Metab 105 (2020) 119:e2111537119. doi: 10.1210/clinem/dgz162
9. Cui J, Liu H, Shao J, Xu DM, Wang Y, Fei Z, et al. Prevalence, risk factors and clinical characteristics of osteoporosis in Chinese inpatients with schizophrenia. Schizophr Res (2018) 195:488–94. doi: 10.1016/j.schres.2017.10.027
11. Schweiger JU, Schweiger U, Huppe M, Kahl KG, Greggersen W, Fassbinder E. Bone density and depressive disorder: a meta-analysis. Brain Behav (2016) 6:e00489. doi: 10.1002/brb3.489
12. Baker NL, Cook MN, Arrighi HM, Bullock R. Hip fracture risk and subsequent mortality among alzheimer's disease patients in the united kingdom, 1988-2007. Age Ageing (2011) 40:49–54. doi: 10.1093/ageing/afq146
13. Liu B, Chen G, Yu Z, Ji C, Liang T, He J, et al. Bone mineral density and related scores in parkinson's disease: A systematic review and meta-analysis. World Neurosurg (2021) 146:e1202–18. doi: 10.1016/j.wneu.2020.11.132
14. Fraser LA, Burneo JG, Fraser JA. Enzyme-inducing antiepileptic drugs and fractures in people with epilepsy: A systematic review. Epilepsy Res (2015) 116:59–66. doi: 10.1016/j.eplepsyres.2015.07.003
15. Davies NM, Holmes MV, Davey Smith G. Reading mendelian randomisation studies: a guide, glossary, and checklist for clinicians. BMJ (2018) 362:k601. doi: 10.1136/bmj.k601
16. Smith GD, Ebrahim S. 'Mendelian randomization': can genetic epidemiology contribute to understanding environmental determinants of disease? Int J Epidemiol (2003) 32:1–22. doi: 10.1093/ije/dyg070
17. Yarmolinsky J, Wade KH, Richmond RC, Langdon RJ, Bull CJ, Tilling KM, et al. Causal inference in cancer epidemiology: What is the role of mendelian randomization? Cancer Epidemiol Biomarkers Prev (2018) 27:995–1010. doi: 10.1158/1055-9965.EPI-17-1177
18. Chen H, Zhang H, Zheng L. No causal association between coffee consumption and risk of migraine: A mendelian randomization study. Front Genet (2022) 13:792313. doi: 10.3389/fgene.2022.792313
19. Gaziano L, Giambartolomei C, Pereira AC, Gaulton A, Posner DC, Swanson SA, et al. Actionable druggable genome-wide mendelian randomization identifies repurposing opportunities for COVID-19. Nat Med (2021) 27:668–76. doi: 10.1038/s41591-021-01310-z
20. Zhou S, Butler-Laporte G, Nakanishi T, Morrison DR, Afilalo J, Afilalo M, et al. A Neanderthal OAS1 isoform protects individuals of European ancestry against COVID-19 susceptibility and severity. Nat Med (2021) 27:659–67. doi: 10.1038/s41591-021-01281-1
21. Li GH, Lam SK, Wong IC, Chu JK, Cheung CL. Education attainment, intelligence and COVID-19: A mendelian randomization study. J Clin Med (2021) 101529–1535. doi: 10.3390/jcm10214870
22. Trajanoska K, Rivadeneira F. Using mendelian randomization to decipher mechanisms of bone disease. Curr Osteoporos Rep (2018) 16:531–40. doi: 10.1007/s11914-018-0467-3
23. C. Schizophrenia Working Group of the Psychiatric Genomics. Biological insights from 108 schizophrenia-associated genetic loci. Nature (2014) 511:421–7. doi: 10.1038/nature13595
24. Howard DM, Adams MJ, Shirali M, Clarke TK, Marioni RE, Davies G, et al. Genome-wide association study of depression phenotypes in UK biobank identifies variants in excitatory synaptic pathways. Nat Commun (2018) 9:1470. doi: 10.1038/s41467-018-03819-3
25. Lambert JC, Ibrahim-Verbaas CA, Harold D, Naj AC, Sims R, Bellenguez C, et al. Meta-analysis of 74,046 individuals identifies 11 new susceptibility loci for alzheimer's disease. Nat Genet (2013) 45:1452–8. doi: 10.1038/ng.2802
26. Simon-Sanchez J, Schulte C, Bras JM, Sharma M, Gibbs JR, Berg D, et al. Genome-wide association study reveals genetic risk underlying parkinson's disease. Nat Genet (2009) 41:1308–12. doi: 10.1038/ng.487
27. International League Against Epilepsy Consortium on Complex. Genome-wide mega-analysis identifies 16 loci and highlights diverse biological mechanisms in the common epilepsies. Nat Commun (2018) 9:5269.
28. Medina-Gomez C, Kemp JP, Trajanoska K, Luan J, Chesi A, Ahluwalia TS, et al. Life-course genome-wide association study meta-analysis of total body BMD and assessment of age-specific effects. Am J Hum Genet (2018) 102:88–102. doi: 10.1016/j.ajhg.2017.12.005
29. Zheng HF, Forgetta V, Hsu YH, Estrada K, Rosello-Diez A, Leo PJ, et al. Whole-genome sequencing identifies EN1 as a determinant of bone density and fracture. Nature (2015) 526:112–7. doi: 10.1038/nature14878
30. Yoshikawa M, Asaba K, Nakayama T. Estimating causal effects of atherogenic lipid-related traits on COVID-19 susceptibility and severity using a two-sample mendelian randomization approach. BMC Med Genomics (2021) 14:269. doi: 10.1186/s12920-021-01127-2
31. Cui Z, Tian Y. Using genetic variants to evaluate the causal effect of serum vitamin d concentration on COVID-19 susceptibility, severity and hospitalization traits: a mendelian randomization study. J Transl Med (2021) 19:300. doi: 10.1186/s12967-021-02973-5
32. He Q, Yang Z, Sun Y, Qu Z, Jia X, Li J, et al. The impact of homocysteine on the risk of hormone-related cancers: A mendelian randomization study. Front Nutr (2021) 8:645371. doi: 10.3389/fnut.2021.645371
33. Hemani G, Zheng J, Elsworth B, Wade KH, Haberland V, Baird D, et al. The MR-base platform supports systematic causal inference across the human phenome. Elife (2018) 7. doi: 10.7554/eLife.34408
34. Luo Z, Liu Y, Liu Y, Chen H, Shi S, Liu Y. Cellular and molecular mechanisms of alcohol-induced osteopenia. Cell Mol Life Sci (2017) 74:4443–53. doi: 10.1007/s00018-017-2585-y
35. Rizzoli R, Biver E. Glucocorticoid-induced osteoporosis: who to treat with what agent? Nat Rev Rheumatol (2015) 11:98–109. doi: 10.1038/nrrheum.2014.188
36. Khosla S. Pathogenesis of age-related bone loss in humans. J Gerontol A Biol Sci Med Sci (2013) 68:1226–35. doi: 10.1093/gerona/gls163
37. Gomez L, Stubbs B, Shirazi A, Vancampfort D, Gaughran F, Lally J. Lower bone mineral density at the hip and lumbar spine in people with psychosis versus controls: a comprehensive review and skeletal site-specific meta-analysis. Curr Osteoporos Rep (2016) 14:249–59. doi: 10.1007/s11914-016-0325-0
38. Zhao Y, Shen L, Ji HF. Alzheimer's disease and risk of hip fracture: a meta-analysis study. ScientificWorldJournal (2012) 2012:872173. doi: 10.1100/2012/872173
39. Min L, Chunyan W, Biaoxue R. Effects of valproic acid on skeletal metabolism in children with epilepsy: a systematic evaluation and meta-analysis based on 14 studies. BMC Pediatr (2020) 20:97. doi: 10.1186/s12887-020-1984-7
40. Stubbs B, Brefka S, Dallmeier D, Stubbs J, Vancampfort D, Denkinger MD. Depression and reduced bone mineral density at the hip and lumbar spine: A comparative meta-analysis of studies in adults 60 years and older. Psychosom Med (2016) 78:492–500. doi: 10.1097/PSY.0000000000000292
41. Boyko EJ. Observational research–opportunities and limitations. J Diabetes Complications (2013) 27:642–8. doi: 10.1016/j.jdiacomp.2013.07.007
42. Skrivankova VW, Richmond RC, Woolf BAR, Yarmolinsky J, Davies NM, Swanson SA, et al. Strengthening the reporting of observational studies in epidemiology using mendelian randomization: The STROBE-MR statement. JAMA (2021) 326:1614–21. doi: 10.1001/jama.2021.18236
43. Fu Y, Xu F, Jiang L, Miao Z, Liang X, Yang J, et al. Circulating vitamin c concentration and risk of cancers: a mendelian randomization study. BMC Med (2021) 19:171. doi: 10.1186/s12916-021-02041-1
44. Miller MJ, Ambrose DM. The problem of missed mental healthcare appointments. Clin Schizophr Relat Psychoses (2019) 12:177–84. doi: 10.3371/CSRP.MIAM.112316
Keywords: mental diseases, osteoporosis, osteoporosis fracture, Mendelian randomization, genome-wide association study
Citation: Tang F, Wang S, Zhao H, Xia D and Dong X (2023) Mendelian randomization analysis does not reveal a causal influence of mental diseases on osteoporosis. Front. Endocrinol. 14:1125427. doi: 10.3389/fendo.2023.1125427
Received: 16 December 2022; Accepted: 28 February 2023;
Published: 20 April 2023.
Edited by:
Laura Gianotti, Azienda Sanitaria Ospedaliera S.Croce e Carle Cuneo, ItalyReviewed by:
Ouyang Chen, Duke University, United StatesCopyright © 2023 Tang, Wang, Zhao, Xia and Dong. This is an open-access article distributed under the terms of the Creative Commons Attribution License (CC BY). The use, distribution or reproduction in other forums is permitted, provided the original author(s) and the copyright owner(s) are credited and that the original publication in this journal is cited, in accordance with accepted academic practice. No use, distribution or reproduction is permitted which does not comply with these terms.
*Correspondence: Xin Dong, ZG9uZ3hpbnNtbXVAMTI2LmNvbQ==; Demeng Xia, ZGVtZW5neGlhQDE2My5jb20=
†These authors have contributed equally to this work
Disclaimer: All claims expressed in this article are solely those of the authors and do not necessarily represent those of their affiliated organizations, or those of the publisher, the editors and the reviewers. Any product that may be evaluated in this article or claim that may be made by its manufacturer is not guaranteed or endorsed by the publisher.
Research integrity at Frontiers
Learn more about the work of our research integrity team to safeguard the quality of each article we publish.