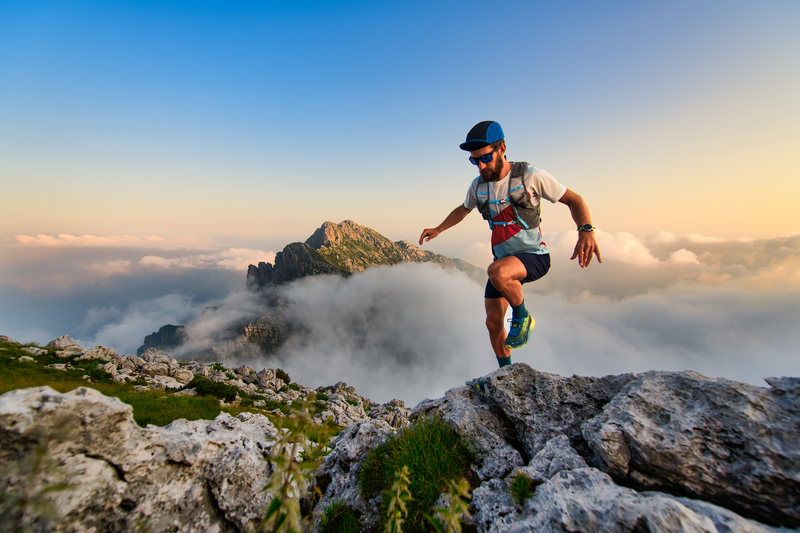
95% of researchers rate our articles as excellent or good
Learn more about the work of our research integrity team to safeguard the quality of each article we publish.
Find out more
SYSTEMATIC REVIEW article
Front. Endocrinol. , 03 February 2023
Sec. Clinical Diabetes
Volume 14 - 2023 | https://doi.org/10.3389/fendo.2023.1117076
Aim: Progression to type 1 diabetes (T1D) is defined in stages and clinical disease is preceded by a period of silent autoimmunity. Improved prediction of the risk and rate of progression to T1D is needed to reduce the prevalence of diabetic ketoacidosis at presentation as well as for staging participants for clinical trials. This systematic review evaluates novel circulating biomarkers associated with future progression to T1D.
Methods: PubMed, Ovid, and EBSCO databases were used to identify a comprehensive list of articles. The eligibility criteria included observational studies that evaluated the usefulness of circulating markers in predicting T1D progression in at-risk subjects <20 years old.
Results: Twenty-six studies were identified, seventeen were cohort studies and ten were case control studies. From the 26 studies, 5 found evidence for protein and lipid dysregulation, 11 identified molecular markers while 12 reported on changes in immune parameters during progression to T1D. An increased risk of T1D progression was associated with the presence of altered gene expression, immune markers including regulatory T cell dysfunction and higher short-lived effector CD8+ T cells in progressors.
Discussion: Several circulating biomarkers are dysregulated before T1D diagnosis and may be useful in predicting either the risk or rate of progression to T1D. Further studies are required to validate these biomarkers and assess their predictive accuracy before translation into broader use.
Systematic review registration: https://www.crd.york.ac.uk/prospero, identifier (CRD42020166830).
Type 1 diabetes (T1D) is a multifactorial, heterogeneous, and polygenic autoimmune disorder characterised by the destruction of pancreatic beta cells resulting in loss of insulin secretion and consequent hyperglycaemia. Presently, the global incidence and prevalence of T1D are increasing with an estimated incidence rate of 15 per 100,000 people and prevalence of 9.5 per 10,000 (1). T1D is diagnosed predominantly in children younger than 15 years (2), however, it may also occur in adulthood (3). TID is a clinical diagnosis based on blood glucose concentration, HbA1c, C-peptide concentrations and islet-specific autoantibodies in combination with typical symptoms (4). However, these symptoms are preceded by a period of ongoing autoimmunity involving immune activation and destruction of insulin secreting beta cells. While this phase of autoimmunity is clinically silent, it represents an opportunity to identify individuals at the greatest risk of developing T1D (5). Identification of these individuals would have multiple benefits including reducing the risk of diabetic ketoacidosis at diagnosis and allowing identification of subjects for new preventative interventions.
The risk factors for T1D are both genetic and environmental. Specific human leukocyte antigen (HLA) class II alleles are associated with increased risk, for example about 30-50% of children with T1D have the combined DR3-DQ2 and DR4-DQ8 haplotype (6). Environmental risk factors for T1D have not been clearly defined but may include nutrition, gut microbiota, antibiotic use, viral infections, route of birth, and the intrauterine and maternal environment (7). These genetic and environmental risk factors initiate a sequence of events that activate autoreactive T cells and subsequent destruction of beta cells, with eventual loss of insulin secretion (8). Prior to diagnosis, autoimmune reactivity is currently identified by the emergence of autoantibodies against proteins linked to the beta cell secretory pathway (9). The presence of islet autoantibodies and high-risk HLA genotypes are well described risk factors for T1D (10) and individuals possessing high-risk HLA genotypes advance to autoantibodies more often compared with individuals with moderate-risk genotypes (11).
Recently, the progression to a clinical diagnosis of T1D has been classified into three stages: Stage 1- a presymptomatic phase characterised by seroconversion (≥ 2 islet autoantibodies), and normoglycaemia; Stage 2- presymptomatic phase characterised by seroconversion and dysglycaemia; and Stage 3 - symptomatic stage characterised by islet autoimmunity and hyperglycaemia (12). The time spent in the presymptomatic stages of T1D varies extensively. Although autoantibodies have proven reliable predictors of T1D, additional biomarkers linked to the rate of progression through these presymptomatic stages of T1D would help identify underlying mechanistic pathways. Accurate circulating biomarkers are highly useful for disease prediction, being relatively easy to access (13). The identification of predictors of clinical T1D may help to elucidate disease pathogenesis and inform the use of preventative therapy. This systematic review evaluates identified circulating predictors of progression from presymptomatic stages to the clinical diagnosis of T1D.
This systematic review was registered on PROSPERO (CRD42020166830) and reported in accordance with the Preferred Reporting Items for Systematic Reviews and Meta-Analyses (PRISMA). The study inclusion dates were amended to 1 January 2010 – 31 August 2022.
Original research articles selected from PubMed, Ovid and EBSCO and published between 2010 and 2022 have been included in this study. Articles were selected using the following search terms: (type 1 diabetes) AND (prediction/predicting/predict/predictor/progression/biomarker/islet autoimmunity). The term ‘Type 1 diabetes’ was searched in the title only. Other search terms including prediction, predicting, predict, predictor, progression, progressor(s), biomarker(s), disease risk, indicator(s), susceptibility, and prognosis were searched individually in combination with Type 1 diabetes in the search field tags title and text word (Supplementary methods).
The full text of titles and abstracts were reviewed to determine eligibility. Studies were deemed eligible using the following inclusion criteria: (1) observational studies (2) analysis of circulating markers predicting onset or progression to T1D or islet autoimmunity; (3) population size of at least 20 participants; (4) participants younger than 20 years old; and (5) participants with HLA DR or/and DQ haplotypes associated with T1D and one or more of the following; a family history of T1D, confirmed islet autoantibodies, abnormalities in glucose metabolism, deficient beta cell function, and T1D. The following studies were excluded (1) reviews, systematic reviews, meta-analyses, studies primarily reporting outcomes from randomized controlled clinical trials, case reports, case series, conference papers or editorials; (2) abstracts where full text components were not available; (3) animal models of T1D; (4) participants older than 20 years; (5) participants with type 2 diabetes; (6) pregnant participants; (7) studies focussed solely on autoantibodies and (8) non-English articles. The search and screening process was objectively performed by one reviewer (EWB) and reviewed by another (EHW). Randomised controlled trials were excluded from this current study as this study focuses on the natural progression of disease and not the response to interventions. Studies with more than 20 participants were chosen as they had more statistical power. Given that T1D is a heterogeneous disease, we chose to focus on participants aged <20 years to minimise the effects of other comorbidities and to allow for identification of markers that may be present early in the disease process prior to adulthood.
All selected articles were assessed for quality of research using the Critical Appraisal Skills Programme (CASP) for cohort studies and case control studies (CASP, 2018). Markers of quality included study design, methods, and duration of study (Supplemental Tables S1, S2).
For all studies meeting the inclusion criteria the data extracted was transferred into an excel spreadsheet. The following information was retrieved from each study: first author, date of publication (year), country or region, diagnostic criteria for T1D, number of cases and controls, type of cases, age at diagnosis or age at sample collection, presence or absence of autoantibodies, male to female ratio and results. The primary outcome was predictors of progression to T1D, where measures of prediction were defined as: (1) gene expression; (2) proteins and lipids; and (3) and immune markers (cells and cytokines). Data extraction from eligible full text articles was performed by one reviewer and reviewed by two reviewers (EHW and MH). A meta-analysis was not possible due to the high degree of heterogeneity between the studies.
Initially, 26546 articles were identified following evaluation of titles and text words (Figure 1). Following the inspection process outlined in Figure 1, 26 articles were chosen for the systematic review. These articles comprised 17 cohort and 9 case control studies (10, 14–36). The characteristics of the selected studies and study participants are presented in Table 1. Among the 26 studies, four did not report on the age at seroconversion or age at the time of sample collection. The 17 cohort studies, included the TrialNet pathway to prevention study (TrialNet), Diabetes prediction and prevention study (DIPP), Diabetes Autoimmunity study in the young (DAISY), Primary Prevention of Type 1 Diabetes in Relatives at Increased Genetic Risk (BABYDIET), German BabyDiab study (BABYDIAB), Pathogenesis of type 1 diabetes-testing the hygiene hypothesis (DIABIMMUNE) and the environmental determinants of diabetes in the Young (TEDDY) cohort studies (10, 20–23, 25–29, 31, 32, 34–36). The 26 selected studies used in the current systematic review were categorised according to marker type with 5 focused on protein and lipid markers, 12 on immunological markers and 11 on molecular markers.
Twelve studies identified alterations in the function and phenotype of different immune cell populations linked to progression to T1D or seroconversion (Table 2). Most of the immune studies focused on T cell subsets and their phenotype, with the changes observed varying depending on the disease stage (Figure 2).
Seroconverted participants had decreased frequencies of mucosal associated invariant T cell (MAIT) and CD127dimCD4+ regulatory T cells (Tregs) compared with islet antibody negative (Ab-) participants. An increased ratio of short-lived effector CD8+ T cells (SLEC) T cells to MAIT cells as well as SLEC : CD127dim cells was found in all seroconverted individuals (progressors and non-progressors) compared with Ab- participants (20). The increase in SLEC was highest amongst seroconverted individuals that later progressed to T1D (20). The SLEC (CD127-, CD27-, CD57+, CD28-) phenotype indicates short-lived, terminally differentiated cells undergoing chronic stimulation with cytotoxic potential. Non-progressors showed reduced CD45RA+CCR7dim memory CD8+T cells and reduced CD127bright memory CD4+T cells compared with Ab- participants (20).
In recent-onset T1D (ROT1D), Glisic et al., reported no change in the number of Tregs but increased Treg apoptosis and reduced suppressive function compared with healthy controls, particularly in those with high-HLA risk (17). Similarly, during the first year after diagnosis Th17 and activated Tregs were elevated in some children compared with those with early beta cell autoimmunity (28, 38). Viisanen et al. found that ROT1D individuals had increased CD25+FOXP3low, CD25lowCD127lowFOXP3+ and total Tregs, due to an increase in naïve Tregs in comparison to healthy subjects (34). There was also a decrease in IFN-γ+ memory Tregs and Ki67+ proliferative Tregs at disease onset compared with controls (34). In another study, both follicular helper Tregs (CXCR5+FOXP3+) and conventional Tregs (CXCR5-FOXP3+) were high in ROT1D compared with Ab- controls, owing to high expression levels of FOXP3+ in CD4+ cells (33). However, in the same study, programmed cell death-1 (PD-1) expression, an immunoregulatory marker linked to T cell tolerance (39), was significantly diminished in Tregs from ROT1D and at-risk individuals compared with Ab- controls (33). Recently, Starosz et al. reported an increase in both Treg frequency and in the ratio of Treg to Th17 cells (38). While Tregs populations were either increased or unchanged in frequency in several studies (17, 33, 34, 38), effector T cells were found to be more resistant to Treg suppression in ROT1D when compared with multiple autoantibody positive subjects (21). Together, these data suggest an important role for a diminishment in Treg suppression of effector T cells at the time of T1D onset.
Dysregulation in proteins and lipids were also observed in individuals progressing to T1D (Table 3). Islet autoantibody positive children had higher serum lipids compared to those children without islet autoantibodies (27). Three metabolic peptides were found to predict future progression to T1D including, complement factor H, hepatocyte growth factor activator and ceruloplasmin (35). Lipids such as sphingomyelins, triacylglycerols and phosphatidylcholines as well as methionine and hydroxyproline were reduced in peripheral blood mononuclear cells (PBMC) or plasma from individuals who progressed to T1D compared with controls (23, 30). These studies suggest that multiple factors may exert a differential effect on lipid levels during disease progression.
Differential expression of genes in blood cell populations may provide insights into the function of immune cells and metabolic pathways as autoimmunity develops. Several genes were observed to be differentially expressed in ROT1D, in individuals progressing to T1D and even very early prior to seroconversion (Table 4; Figure 2) (10, 15, 18, 19, 22, 24, 25, 31, 32, 37). A signature of 56 genes inducible with type-1-interferon including SIGLEC-1 was found to be upregulated prior to the development of autoantibodies in at-risk children compared with controls (15). As islet autoimmunity progressed, reduced expression of several transcription factors involved in T-cell differentiation were observed in whole blood RNA from individuals with multiple islet autoantibodies (T-bet, CTLA-4 and GATA3) compared with Ab- control children (18). Higher expression of BACH2, TXNDC5 and CDC20, genes that regulate lymphocytes and cell cycle progression, were found in islet autoantibody+ children that later progressed to T1D compared with non-progressors (22). This suggests that altered gene expression profiles occur amongst blood immune cells prior to T1D onset and these may be useful for prediction of future diagnosis.
In ROT1D, the expression of T cell specific transcription factors including RORC, FOXP3, T-bet and GATA3 were reduced compared with Ab- control children (18). However, mRNA levels of several genes (IL-17A, IL-17F, IFN-γ, IL-9 and Foxp3) linked to T cell effector function or regulation were increased in PBMC stimulated with anti-CD3 and anti-CD28 from individuals with ROT1D compared with Ab- controls (28). Cytokine genes were also upregulated in non-activated PBMCs from ROT1D compared to islet autoantibody+ individuals (IFN-γ, IL-4 and IL-10) (19). Of interest, higher unmethylated INS DNA, a marker of beta cell death, was observed in serum from ROT1D participants compared with islet autoantibody+ participants (31). The above biomarkers suggest that multiple physiological processes are disrupted during progression to T1D.
Differential expression of microRNAs (miRNA) with varying roles in metabolic and immune function were observed in several studies. Importantly, 11 miRNAs were upregulated and 6 were down regulated in islet autoantibody+ participants compared with healthy controls (37). In contrast, only 4 miRNA were upregulated and one downregulated in participants with ROT1D. Increased expression of miR-23a-3p, miR-23b-3p, miR-24-3p, miR-27a-3p, miR-27b-3p, miR-21-3p, miR-29a-3p and miR-424-5p were observed in islet autoantibody+ participants who progressed to T1D compared with healthy controls (16, 32). A downregulation of miR-375 was observed in individuals with ROT1D compared with healthy controls (24), while miR-152, miR-30a-5p, miR-181a, miR-24, miR-148, miR-200, miR-210a, miR-27a, miR-29a, miR-27b, miR-26a and miR-25 were upregulated in ROTID participants compared with healthy controls (25). Of interest, mir-200a-3p was consistently upregulated in both islet autoantibody+ and ROT1D and miR-16 was reduced in both groups (37). Several of these miRNAs have been linked to beta cell apoptosis and damage (40, 41), suggesting an important role of miRNAs in the pathogenesis of T1D.
Biomarkers predicting progression to T1D are urgently needed and represent an emerging area of research interest. This systematic review of case control and cohort studies summarises important circulating biomarkers in individuals progressing to clinical T1D (Figure 2). These circulating biomarkers comprise immunological, metabolic, and molecular factors. Biomarkers with concordant evidence from multiple studies included increased Treg numbers but decreased functionality, while other markers such as cytokine expression patterns and lipid levels were discordant. Collectively, these biomarkers suggest that prior to diagnosis immune dysfunction accelerates with increased effector cell function and lack of immune suppression, coinciding with beta cell functional decline. However, there are still unknown mechanistic pathways involved in this critical period.
Several markers of beta cell destruction were identified in the current study. Beta cells are the main producers of unmethylated INS and a higher unmethylated: methylated INS is observed during progression to T1D (31). The presence of circulating unmethylated INS DNA is a marker for beta cell death and reduction in insulin which accelerates at the symptomatic stage of the disease (42). MiR-375 is highly expressed in islet cells and regulates development of islet cell mass (alpha and beta) (43). In animal models, deficiency in miR-375 islet cells results in persistent hyperglycaemia due to alteration of pancreatic α-cell function, including elevated α cell mass (24) and a concomitant decrease in beta cell mass due to upregulation of destructive growth factor genes including apoptosis-inducing factor, mitochondrion associated 1 (Aifm1), caveolin1 (Cav1), complement component 1 q subcomponent binding protein (C1qbp), cell adhesion molecule 1 (Cadm1), eukaryotic elongation factor 1 epsilon 1 (Eef1e1), HuD antigen (HuD), inhibitor of DNA binding 3 (Id3), Ras-dexamethasone-induced-1 (Rasd1), and regulator of G protein signalling 16 (Rgs16) (43, 44). Increased expression of miR-29 and miR-200a-3p in beta cells promotes destruction of beta cells and progression to T1D (41, 45). Circulating miR-25 increases residual beta cell function and glycaemic control (25) and more recently it has been shown to suppress translation of INS gene and decreased insulin production (46).
Importantly, Tregs are among the key cells implicated in the pathogenesis of T1D and polymorphisms in IL2RA, CTLA4, PTPN2 and PTPN22 have been shown to contribute to alterations in Treg function in T1D (8). In addition, CD8+T cells and other effector cells are involved in pancreatic insulitis prior to clinical diagnosis (47). The presence of these cells and SLEC cells suggest increased effector cell activity, and changes in effector cell to Treg ratios prior to disease onset. Differential expression of genes involved in the regulation of immune cell biology, are likely to explain the alterations in immune function in T1D (48). Notably, dysregulation in T cell transcription factors affects multiple T cell functional pathways. The increase in Treg numbers after T1D onset seems counter-intuitive but may reflect resolution of inflammatory stimuli that may have been present at clinical onset or a down-regulation of the islet-specific response in general once islet antigens are lost.
In the present review, other circulating miRNAs were associated with progression to T1D. Dysregulation in miRNA pathways including miR-142-3p, miR-181a, mirR-181c, miR-193, miR-200, miR-27a, miR-29a, miR-330-3p and miR-326 may contribute to the loss of Treg function (49–52). In addition, miR-181a-5p from activated CD4+ T cells targets SHP-2 and PTPN22, two genes implicated in the progression to T1D (53). Despite the many unanswered questions relating to circulating miRNAs, they are likely to provide important clues in understanding T1D progression and may be important future therapeutic targets. Interestingly, the expression of transcription factors and cytokine data reported in the various studies included in this review were often discordant, possibly due to differences in methodology.
This review is not without limitations, as selected studies were restricted to participants less than 20 years of age, and therefore does not directly address progression to T1D in adult subjects. Most of the studies in this review involved participants with high genetic risk factors and this may not be representative of the current population of individuals diagnosed with T1D in the clinical setting. Several molecular studies were excluded due to the small sample size and these articles may have provided further insight into the molecular mechanisms underlying islet autoimmunity and progression to T1D. As previously mentioned, subject selection differed for the various studies, potentially contributing to some of the discrepant findings. Variation in the attributes of the at-risk populations studied including family history of T1D and the presence or absence of certain HLAs significantly influences the risk profile.
This review highlights a number of knowledge gaps and suggests five main areas of future research. Firstly, additional research is required in the stratifying individuals at high risk of progressing to T1D. Secondly, the discrepancy in some studies suggests that the exact mechanistic pathways underlying to progression to T1D may vary. Thirdly, numerous miRNAs were identified in the review but the exact role of many of these miRNAs is yet to be determined and further studies specifically focused on circulating or cell derived miRNA may be useful in validating these molecules in other cohorts. Fourthly, given that there were only a handful of studies on the role of proteins and lipids in progression to T1D further research is required in this area. A focus on molecules likely perform better as biomarkers and be adaptable to clinical assays such as proteins or simple gene expression on whole blood should be prioritised. Lastly, the pathways responsible for progressing to early and late onset T1D are needed.
In conclusion, the included studies confirm disturbances in the prediabetes stage of T1D, particularly at the immunological and molecular level. A greater understanding of these parameters may provide future therapeutic opportunities. Future research should consider and address specific applications of these biomarkers using animal models and large samples of participants at risk of and progressing to T1D. Such research should be sufficiently powered, focused on specific cell types, and consider incorporating multiple reported biomarkers in developing prediction algorithms.
The original contributions presented in the study are included in the article/Supplementary Material. Further inquiries can be directed to the corresponding author.
EH-W, MH and EB designed the study. EB managed data collection. EB and EH-W conducted the analysis. EH-W, MH and EB contributed to the interpretation of the results. EB wrote the initial draft of the manuscript, with revisions by all authors. The final manuscript was approved by all authors. EB, EH-W and MH are the guarantors of this work. All authors contributed to the article and approved the submitted version.
EH-W is supported by grants from the Juvenile Diabetes Research Foundation (3-SRA-2019-730-S-B, 201308399), Children’s Hospital Foundation and National Health and Medical Research Council (APP2002917). Part of this research was carried out at the Translational Research Institute, Woolloongabba, 4102 Australia. The Translational Research Institute is supported by a grant from the Australian Government.
Figure 2 made using BioRender.
The authors declare that the research was conducted in the absence of any commercial or financial relationships that could be construed as a potential conflict of interest.
All claims expressed in this article are solely those of the authors and do not necessarily represent those of their affiliated organizations, or those of the publisher, the editors and the reviewers. Any product that may be evaluated in this article, or claim that may be made by its manufacturer, is not guaranteed or endorsed by the publisher.
The Supplementary Material for this article can be found online at: https://www.frontiersin.org/articles/10.3389/fendo.2023.1117076/full#supplementary-material
1. Mobasseri M, Shirmohammadi M, Amiri T, Vahed N, Hosseini Fard H, Ghojazadeh M. Prevalence and incidence of type 1 diabetes in the world: a systematic review and meta-analysis. Health Promot Perspect (2020) 10(2):98–115. doi: 10.34172/hpp.2020.18
2. Chobot A, Polanska J, Brandt A, Deja G, Glowinska-Olszewska B, Pilecki O, et al. Updated 24-year trend of type 1 diabetes incidence in children in Poland reveals a sinusoidal pattern and sustained increase. Diabetes Med (2017) 34(9):1252–8. doi: 10.1111/dme.13345
3. DiMeglio LA, Evans-Molina C, Oram RA. Type 1 diabetes. Lancet (2018) 391(10138):2449–62. doi: 10.1016/S0140-6736(18)31320-5
4. Use of glycated haemoglobin (HbA1c) in the diagnosis of diabetes mellitus: Abbreviated report of a WHO consultation. Geneva: WHO Guidelines Approved by the Guidelines Review Committee (2011).
5. Insel R, Dutta S, Hedrick J. Type 1 diabetes: Disease stratification. BioMed Hub (2017) 2(Suppl 1):111–26. doi: 10.1159/000481131
6. Pociot F, Lernmark A. Genetic risk factors for type 1 diabetes. Lancet (2016) 387(10035):2331–9. doi: 10.1016/S0140-6736(16)30582-7
7. Craig ME, Kim KW, Isaacs SR, Penno MA, Hamilton-Williams EE, Couper JJ, et al. Early-life factors contributing to type 1 diabetes. Diabetologia (2019) 62(10):1823–34. doi: 10.1007/s00125-019-4942-x
8. Ilonen J, Lempainen J, Veijola R. The heterogeneous pathogenesis of type 1 diabetes mellitus. Nat Rev Endocrinol (2019) 15(11):635–50. doi: 10.1038/s41574-019-0254-y
9. Fousteri G, Ippolito E, Ahmed R, Hamad ARA. Beta-cell specific autoantibodies: Are they just an indicator of type 1 diabetes? Curr Diabetes Rev (2017) 13(3):322–9. doi: 10.2174/1573399812666160427104157
10. Mehdi AM, Hamilton-Williams EE, Cristino A, Ziegler A, Bonifacio E, Le Cao KA, et al. A peripheral blood transcriptomic signature predicts autoantibody development in infants at risk of type 1 diabetes. JCI Insight (2018) 3(5):e98212. doi: 10.1172/jci.insight.98212
11. Pollanen PM, Ryhanen SJ, Toppari J, Ilonen J, Vahasalo P, Veijola R, et al. Dynamics of islet autoantibodies during prospective follow-up from birth to age 15 years. J Clin Endocrinol Metab (2020) 105(12):e4638–e4651. doi: 10.1210/clinem/dgaa624
12. Couper JJ, Haller MJ, Greenbaum CJ, Ziegler AG, Wherrett DK, Knip M, et al. ISPAD clinical practice consensus guidelines 2018: Stages of type 1 diabetes in children and adolescents. Pediatr Diabetes (2018) 19 Suppl 27:20–7. doi: 10.1111/pedi.12734
13. Yi L, Swensen AC, Qian WJ. Serum biomarkers for diagnosis and prediction of type 1 diabetes. Transl Res (2018) 201:13–25. doi: 10.1016/j.trsl.2018.07.009
14. Arif S, Leete P, Nguyen V, Marks K, Nor NM, Estorninho M, et al. Blood and islet phenotypes indicate immunological heterogeneity in type 1 diabetes. Diabetes (2014) 63(11):3835–45. doi: 10.2337/db14-0365
15. Ferreira RC, Guo H, Coulson RM, Smyth DJ, Pekalski ML, Burren OS, et al. A type I interferon transcriptional signature precedes autoimmunity in children genetically at risk for type 1 diabetes. Diabetes (2014) 63(7):2538–50. doi: 10.2337/db13-1777
16. Garavelli S, Bruzzaniti S, Tagliabue E, Di Silvestre D, Prattichizzo F, Mozzillo E, et al. Plasma circulating miR-23~27~24 clusters correlate with the immunometabolic derangement and predict c-peptide loss in children with type 1 diabetes. Diabetologia (2020) 63(12):2699–712. doi: 10.1007/s00125-020-05237-x
17. Glisic S, Jailwala P. Interaction between treg apoptosis pathways, treg function and HLA risk evolves during type 1 diabetes pathogenesis. PloS One (2012) 7(4):e36040. doi: 10.1371/journal.pone.0036040
18. Hamari S, Kirveskoski T, Glumoff V, Kulmala P, Simell O, Knip M, et al. Analyses of regulatory CD4+ CD25+ FOXP3+ T cells and observations from peripheral T cell subpopulation markers during the development of type 1 diabetes in children. Scand J Immunol (2016) 83(4):279–87. doi: 10.1111/sji.12418
19. Han D, Leyva CA, Matheson D, Mineo D, Messinger S, Blomberg BB, et al. Immune profiling by multiple gene expression analysis in patients at-risk and with type 1 diabetes. Clin Immunol (2011) 139(3):290–301. doi: 10.1016/j.clim.2011.02.016
20. Harms RZ, Lorenzo-Arteaga KM, Ostlund KR, Smith VB, Smith LM, Gottlieb P, et al. Abnormal T cell frequencies, including cytomegalovirus-associated expansions, distinguish seroconverted subjects at risk for type 1 diabetes. Front Immunol (2018) 9:2332. doi: 10.3389/fimmu.2018.02332
21. Ihantola EL, Viisanen T, Gazali AM, Nanto-Salonen K, Juutilainen A, Moilanen L, et al. Effector T cell resistance to suppression and STAT3 signaling during the development of human type 1 diabetes. J Immunol (2018) 201(4):1144–53. doi: 10.4049/jimmunol.1701199
22. Jin Y, Sharma A, Bai S, Davis C, Liu H, Hopkins D, et al. Risk of type 1 diabetes progression in islet autoantibody-positive children can be further stratified using expression patterns of multiple genes implicated in peripheral blood lymphocyte activation and function. Diabetes (2014) 63(7):2506–15. doi: 10.2337/db13-1716
23. Lamichhane S, Ahonen L, Dyrlund TS, Kemppainen E, Siljander H, Hyoty H, et al. Dynamics of plasma lipidome in progression to islet autoimmunity and type 1 diabetes - type 1 diabetes prediction and prevention study (DIPP). Sci Rep (2018) 8(1):10635. doi: 10.1038/s41598-018-28907-8
24. Marchand L, Jalabert A, Meugnier E, Van den Hende K, Fabien N, Nicolino M, et al. miRNA-375 a sensor of glucotoxicity is altered in the serum of children with newly diagnosed type 1 diabetes. J Diabetes Res (2016) 2016:1869082. doi: 10.1155/2016/1869082
25. Nielsen LB, Wang C, Sorensen K, Bang-Berthelsen CH, Hansen L, Andersen ML, et al. Circulating levels of microRNA from children with newly diagnosed type 1 diabetes and healthy controls: evidence that miR-25 associates to residual beta-cell function and glycaemic control during disease progression. Exp Diabetes Res (2012) 2012:896362. doi: 10.1155/2012/896362
26. Oling V, Reijonen H, Simell O, Knip M, Ilonen J. Autoantigen-specific memory CD4+ T cells are prevalent early in progression to type 1 diabetes. Cell Immunol (2012) 273(2):133–9. doi: 10.1016/j.cellimm.2011.12.008
27. Pflueger M, Seppanen-Laakso T, Suortti T, Hyotylainen T, Achenbach P, Bonifacio E, et al. Age- and islet autoimmunity-associated differences in amino acid and lipid metabolites in children at risk for type 1 diabetes. Diabetes (2011) 60(11):2740–7. doi: 10.2337/db10-1652
28. Reinert-Hartwall L, Honkanen J, Salo HM, Nieminen JK, Luopajarvi K, Harkonen T, et al. Th1/Th17 plasticity is a marker of advanced beta cell autoimmunity and impaired glucose tolerance in humans. J Immunol (2015) 194(1):68–75. doi: 10.4049/jimmunol.1401653
29. Salami F, Lee HS, Freyhult E, Elding Larsson H, Lernmark A, Torn C, et al. Reduction in white blood cell, neutrophil, and red blood cell counts related to sex, HLA, and islet autoantibodies in Swedish TEDDY children at increased risk for type 1 diabetes. Diabetes (2018) 67(11):2329–36. doi: 10.2337/db18-0355
30. Sen P, Dickens AM, Lopez-Bascon MA, Lindeman T, Kemppainen E, Lamichhane S, et al. Metabolic alterations in immune cells associate with progression to type 1 diabetes. Diabetologia (2020) 63(5):1017–31. doi: 10.1007/s00125-020-05107-6
31. Simmons KM, Fouts A, Pyle L, Clark P, Dong F, Yu L, et al. Unmethylated insulin as an adjunctive marker of beta cell death and progression to type 1 diabetes in participants at risk for diabetes. Int J Mol Sci (2019) 20(16):3857. doi: 10.3390/ijms20163857
32. Snowhite IV, Allende G, Sosenko J, Pastori RL, Messinger Cayetano S, Pugliese A. Association of serum microRNAs with islet autoimmunity, disease progression and metabolic impairment in relatives at risk of type 1 diabetes. Diabetologia (2017) 60(8):1409–22. doi: 10.1007/s00125-017-4294-3
33. Vecchione A, Di Fonte R, Gerosa J, Jofra T, Cicalese MP, Napoleone V, et al. Reduced PD-1 expression on circulating follicular and conventional FOXP3(+) treg cells in children with new onset type 1 diabetes and autoantibody-positive at-risk children. Clin Immunol (2020) 211:108319. doi: 10.1016/j.clim.2019.108319
34. Viisanen T, Gazali AM, Ihantola EL, Ekman I, Nanto-Salonen K, Veijola R, et al. FOXP3+ regulatory T cell compartment is altered in children with newly diagnosed type 1 diabetes but not in autoantibody-positive at-risk children. Front Immunol (2019) 10:19. doi: 10.3389/fimmu.2019.00019
35. von Toerne C, Laimighofer M, Achenbach P, Beyerlein A, de Las Heras Gala T, Krumsiek J, et al. Peptide serum markers in islet autoantibody-positive children. Diabetologia (2017) 60(2):287–95. doi: 10.1007/s00125-016-4150-x
36. Waugh K, Snell-Bergeon J, Michels A, Dong F, Steck AK, Frohnert BI, et al. Increased inflammation is associated with islet autoimmunity and type 1 diabetes in the diabetes autoimmunity study in the young (DAISY). PloS One (2017) 12(4):e0174840. doi: 10.1371/journal.pone.0174840
37. Santos AS, Ferreira LRP, da Silva AC, Alves LI, Damasceno JG, Kulikowski L, et al. Progression of type 1 diabetes: Circulating MicroRNA expression profiles changes from preclinical to overt disease. J Immunol Res (2022) 2022:2734490. doi: 10.1155/2022/2734490
38. Starosz A, Jamiolkowska-Sztabkowska M, Glowinska-Olszewska B, Moniuszko M, Bossowski A, Grubczak K. Immunological balance between treg and Th17 lymphocytes as a key element of type 1 diabetes progression in children. Front Immunol (2022) 13:958430. doi: 10.3389/fimmu.2022.958430
39. Dai S, Jia R, Zhang X, Fang Q, Huang L. The PD-1/PD-Ls pathway and autoimmune diseases. Cell Immunol (2014) 290(1):72–9. doi: 10.1016/j.cellimm.2014.05.006
40. Klein D, Misawa R, Bravo-Egana V, Vargas N, Rosero S, Piroso J, et al. MicroRNA expression in alpha and beta cells of human pancreatic islets. PloS One (2013) 8(1):e55064. doi: 10.1371/journal.pone.0055064
41. Filios SR, Xu G, Chen J, Hong K, Jing G, Shalev A. MicroRNA-200 is induced by thioredoxin-interacting protein and regulates Zeb1 protein signaling and beta cell apoptosis. J Biol Chem (2014) 289(52):36275–83. doi: 10.1074/jbc.M114.592360
42. Fisher MM, Watkins RA, Blum J, Evans-Molina C, Chalasani N, DiMeglio LA, et al. Elevations in circulating methylated and unmethylated preproinsulin DNA in new-onset type 1 diabetes. Diabetes (2015) 64(11):3867–72. doi: 10.2337/db15-0430
43. Poy MN, Hausser J, Trajkovski M, Braun M, Collins S, Rorsman P, et al. miR-375 maintains normal pancreatic alpha- and beta-cell mass. Proc Natl Acad Sci U S A (2009) 106(14):5813–8. doi: 10.1073/pnas.0810550106
44. Assmann TS, Recamonde-Mendoza M, De Souza BM, Crispim D. MicroRNA expression profiles and type 1 diabetes mellitus: systematic review and bioinformatic analysis. Endocr Connect (2017) 6(8):773–90. doi: 10.1530/EC-17-0248
45. Slusarz A, Pulakat L. The two faces of miR-29. J Cardiovasc Med (Hagerstown) (2015) 16(7):480–90. doi: 10.2459/JCM.0000000000000246
46. Margaritis K, Margioula-Siarkou G, Giza S, Kotanidou EP, Tsinopoulou VR, Christoforidis A, et al. Micro-RNA implications in type-1 diabetes mellitus: A review of literature. Int J Mol Sci (2021) 22(22):12165. doi: 10.3390/ijms222212165
47. Pugliese A. Insulitis in the pathogenesis of type 1 diabetes. Pediatr Diabetes (2016) 17 Suppl 22:31–6. doi: 10.1111/pedi.12388
48. Redondo MJ, Steck AK, Pugliese A. Genetics of type 1 diabetes. Pediatr Diabetes (2018) 19(3):346–53. doi: 10.1111/pedi.12597
49. Soltanzadeh-Yamchi M, Shahbazi M, Aslani S, Mohammadnia-Afrouzi M. MicroRNA signature of regulatory T cells in health and autoimmunity. BioMed Pharmacother (2018) 100:316–23. doi: 10.1016/j.biopha.2018.02.030
50. Li QJ, Chau J, Ebert PJ, Sylvester G, Min H, Liu G, et al. miR-181a is an intrinsic modulator of T cell sensitivity and selection. Cell (2007) 129(1):147–61. doi: 10.1016/j.cell.2007.03.008
51. Scherm MG, Daniel C. miRNA regulation of T cells in islet autoimmunity and type 1 diabetes. Curr Diabetes Rep (2020) 20(9):41. doi: 10.1007/s11892-020-01325-9
52. Scherm MG, Serr I, Kaestner KH, Daniel C. The role of T cell miRNAs for regulatory T cell induction in islet autoimmunity. Mol Metab (2019) 27S:S122–S8. doi: 10.1016/j.molmet.2019.06.009
Keywords: biomarkers, islet autoimmunity, type 1 diabetes, disease progression, miRNA, systematic review, immune markers
Citation: Brenu EW, Harris M and Hamilton-Williams EE (2023) Circulating biomarkers during progression to type 1 diabetes: A systematic review. Front. Endocrinol. 14:1117076. doi: 10.3389/fendo.2023.1117076
Received: 06 December 2022; Accepted: 25 January 2023;
Published: 03 February 2023.
Edited by:
Ping Wang, Department of Radiology, Michigan State University, United StatesReviewed by:
Arnaud Zaldumbide, Leiden University Medical Center (LUMC), NetherlandsCopyright © 2023 Brenu, Harris and Hamilton-Williams. This is an open-access article distributed under the terms of the Creative Commons Attribution License (CC BY). The use, distribution or reproduction in other forums is permitted, provided the original author(s) and the copyright owner(s) are credited and that the original publication in this journal is cited, in accordance with accepted academic practice. No use, distribution or reproduction is permitted which does not comply with these terms.
*Correspondence: Emma E. Hamilton-Williams, ZS5oYW1pbHRvbndpbGxpYW1zQHVxLmVkdS5hdQ==
†ORCID: Emma E. Hamilton-Williams, orcid.org/0000-0003-4892-3691
Disclaimer: All claims expressed in this article are solely those of the authors and do not necessarily represent those of their affiliated organizations, or those of the publisher, the editors and the reviewers. Any product that may be evaluated in this article or claim that may be made by its manufacturer is not guaranteed or endorsed by the publisher.
Research integrity at Frontiers
Learn more about the work of our research integrity team to safeguard the quality of each article we publish.