- 1Department of Clinical Laboratory, The Affiliated People’s Hospital of Shandong First Medical University, Jinan, China
- 2Department of Endocrinology, The Affiliated People’s Hospital of Shandong First Medical University, Jinan, China
- 3Department of Endocrinology, The Third People’s Hospital of Jinan, Jinan, China
- 4Department of Nephrology, The Affiliated People’s Hospital of Shandong First Medical University, Jinan, China
Objective: Pyroptosis, a lytic and inflammatory programmed cell death, has been implicated in type 2 diabetes mellitus (T2DM) and its complications. Nonetheless, it remains elusive exactly which pyroptosis molecule exerts an essential role in T2DM, and this study aims to solve such issue.
Methods: Transcriptional profiling datasets of T2DM, i.e., GSE20966, GSE95849, and GSE26168, were acquired. Four machine learning models, namely, random forest, support vector machine, extreme gradient boosting, and generalized linear modeling, were built based on pyroptosis genes. A nomogram of key pyroptosis genes was also generated, and the clinical value was appraised via calibration curves and decision curve analysis. Immune infiltration was inferred utilizing CIBERSORT. Drug–druggable target relationships were acquired from the Drug Gene Interaction Database. Through WGCNA, key pyroptosis-relevant genes were selected.
Results: Most pyroptosis genes exhibited upregulation in T2DM relative to controls, indicating the activity of pyroptosis in T2DM. The SVM model composed of BAK1, CHMP2B, NLRP6, PLCG1, and TIRAP exhibited the best performance in T2DM diagnosis, with AUC = 1. The nomogram can predict the risk of T2DM for clinical practice. NK cells resting exhibited a lower abundance in T2DM versus normal specimens, with a higher abundance of neutrophils. NLRP6 was positively linked with neutrophils. Drugs (keracyanin, 9,10-phenanthrenequinone, diclofenac, phosphomethylphosphonic acid adenosyl ester, acetaminophen, cefixime, aspirin, ustekinumab) potentially targeted the key pyroptosis genes. Additionally, CHMP2B-relevant genes were determined.
Conclusion: Altogether, this work proposes the key pyroptosis genes in T2DM, which might become possible molecules for the management and treatment of T2DM and its complications.
Introduction
Diabetes mellitus (DM) is a chronic metabolic disorder with the characteristics of high blood glucose level, which results from definitive insulin function and/or decreased insulin generation (1). In both type 1 DM (T1DM) and type 2 DM (T2DM), a variety of genetic and environmental factors may lead to progressive loss of β-cell number and/or function, clinically manifested as hyperglycemia (2–4). When hyperglycemia occurs, diabetic individuals are at risk of developing the same chronic complications, though the rate of progression may be different (5). DM is connected with acute and chronic complications, which can be restrained or delayed through intensive glycemic management (6). An in-depth understanding of the pathogenesis of T2DM allows us to better predict the outcome and choose the more precise treatment.
Pyroptosis is a form of programmed cell death characterized by rapid membrane rupture, cell swelling with large bubbles, and the release of proinflammatory cell ingredients (7). The main role of pyroptosis is to drive a strong inflammatory response and protect the host from microbial infection (8). Accumulated evidence suggests the connections of pyroptosis with DM and its complications. For instance, CD74 ablation can rescue T2DM-driven cardiac remodeling and contractile dysfunction via pyroptosis-induced modulation of ferroptosis (9). HECTD3 facilitates NLRP3 inflammasome and pyroptosis for exacerbating DM-relevant cognitive impairment through stabilizing MALT1 (10). Mitochondrial injury and activation of the cytosolic DNA sensor cGAS-STING signaling result in cardiac pyroptosis and hypertrophy in diabetic cardiomyopathy (11). ManNAc exerts a protective effect on podocyte pyroptosis in diabetic renal injury through inhibition of mitochondrial injury and ROS/NLRP3 signaling (12). Schisandrin A mitigates ferroptosis and NLRP3 inflammasome-driven pyroptosis in diabetic nephropathy via mitochondrial damage through AdipoR1 ubiquitination (13). Nonetheless, it is still elusive exactly which pyroptosis molecule exerts a crucial function in T2DM pathogenesis. Herein, diverse machine learning algorithms were adopted for the selection of key pyroptosis molecules, which might have the potential as therapeutic targets of T2DM.
Materials and methods
Datasets
Transcriptional profiling of DM was acquired from the Gene Expression Omnibus. The GSE20966 dataset (https://www.ncbi.nlm.nih.gov/geo/query/acc.cgi?acc=GSE20966) was composed of pancreatic tissues from 10 non-diabetic controls and 10 T2DM patients on the GPL1352 platform (14). The GSE95849 dataset (https://www.ncbi.nlm.nih.gov/geo/query/acc.cgi?acc=GSE95849) comprised peripheral blood samples from six T2DM patients and six healthy participants on the GPL22448 platform (15). The GSE20966 and GSE95849 datasets were merged as the discovery set, and batch effects were removed using the sva package, which was visualized into the principal component analysis (PCA) (16). The GSE26168 dataset (https://www.ncbi.nlm.nih.gov/geo/query/acc.cgi?acc=GSE26168) contained peripheral blood specimens from eight healthy subjects and nine T2DM patients on the GPL6883 platform for external verification (17).
Collection of pyroptosis genes
Pyroptosis genes were gathered from prior research (18–20). RCircos package was adopted for the visualization of the genomic position of pyroptosis genes (21).
Machine learning models
Four machine learning approaches composed of random forest (RF), support vector machine (SVM), extreme gradient boosting (XGB), and generalized linear modeling (GLM) were conducted for selecting the characteristic pyroptosis genes. Receiver operating characteristic curves (ROCs) were plotted for computing the area under the curve (AUC) on each established model or key pyroptosis gene.
Nomogram establishment
A nomogram was generated through the integration of key pyroptosis genes utilizing the rms package. Calibration curves were drawn for the visualization and evaluation of the consistency between the actual observations and the nomogram-predicted results. Decision curve analysis (DCA) was conducted for quantifying the net benefit at distinct threshold probabilities.
Gene set enrichment analysis
Gene set enrichment analysis (GSEA) was conducted for identifying the possible functions of the selected genes (22). The reference gene sets were acquired from the Molecular Signature Database (23), with p <0.05 as the threshold.
Immune infiltration estimation
Through the CIBERSORT approach (24), the normalized transcriptional profiling of T2DM and normal specimens was transformed to the immune components based upon the LM22 reference signature matrix set at 1,000 permutations.
Drug–druggable target network
From the Drug Gene Interaction Database (DGIdb; www.dgidb.org) (25), the interactions of drugs with key pyroptosis genes were acquired. Afterward, a drug–target network was built by using the Cytoscape software (26).
Weighted correlation network analysis
The weighted correlation network analysis (WGCNA) package was adopted for building co-expression modules (27). The optimal soft thresholding value was selected through the pickSoftThreshold function. By using the dynamic tree cut method, highly connected genes were merged into one co-expression module. The structure of the co-expression modules was visualized through a heatmap plot via the TOMplot function. The interactions of modules with key pyroptosis genes were then estimated via Pearson’s test, followed by the evaluation of the module membership versus gene significance.
Protein–protein interaction
Module genes were imported onto the STRING website (28), and protein–protein interaction pairs were acquired. The key genes were selected by using molecular complex detection (MCODE) (a plugin in Cytoscape).
Functional enrichment analysis
By using the clusterProfiler method (29), Gene Ontology (GO) enrichment analysis was carried out, which comprised the biological process (BP), cellular component (CC), and molecular function (MF). Afterward, enrichment of the Kyoto Encyclopedia of Genes and Genomes (KEGG) pathways was implemented. Results with p <0.05 were indicative of significant enrichment.
Statistical analysis
All the analyses were implemented using the R package (version 3.5.3; https://www.r-project.org/). Two groups were compared by adopting the Wilcoxon test. Pearson’s test was employed for correlation analysis. p <0.05 was indicative of statistical significance.
Results
Aberrant expression of pyroptosis genes in T2DM
Figure 1 depicts the workflow of this study. This work combined two T2DM datasets, namely, GSE20966 and GSE95849, for expanding the sample size as much as possible (Figure 2A). The removal of batch effects was then implemented (Figure 2B). Figure 2C illustrates the genomic position of pyroptosis genes. The detailed information is listed in Table 1. Next, the expression differences in pyroptosis genes were estimated in T2DM and controls. Most pyroptosis genes including CHMP4A, CHMP6, GSDMD, IL1B, IRF2, TP53, CASP9, NLRC4, NOD1, NOD2, and PYCARD exhibited notable upregulation in T2DM relative to normal specimens (Figures 2D, E), indicating the activation of pyroptosis in T2DM. Both in T2DM and control tissues, pyroptosis genes closely interacted (Figure 2F).
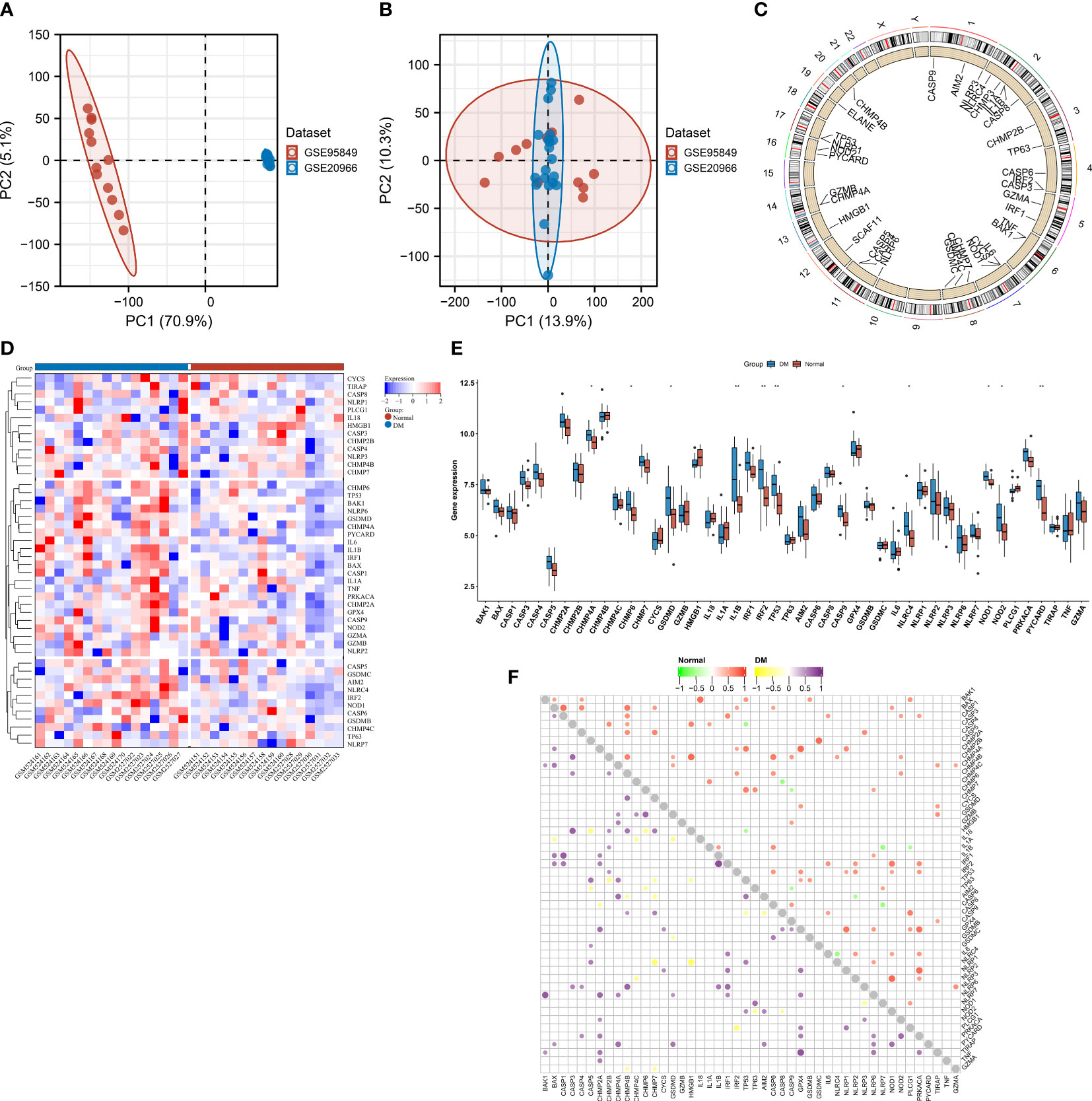
Figure 2 Aberrant expression of pyroptosis genes in type 2 diabetes mellitus (T2DM). (A, B) PCA plots of transcriptional profiling of T2DM and control specimens in the GSE20966 and GSE95849 datasets (A) before and (B) after the removal of batch effects. Each dot denotes one specimen. (C) Genomic location of pyroptosis genes. (D) Heatmap of the transcript levels of pyroptosis genes across T2DM and controls. (E) Comparing the levels of pyroptosis genes in T2DM relative to normal specimens. *p < 0.05; **p < 0.01. (F) Relationships between pyroptosis genes in T2DM or normal tissues.
Establishment of multiple machine learning models of T2DM based upon pyroptosis genes
Four machine learning algorithms—RF, SVM, XGB, and GLM—were implemented for establishing pyroptosis-relevant models for T2DM diagnosis. The selected characteristic pyroptosis genes of each model were as follows: RF (CASP3, TP53, TIRAP, CHMP2B, and NLRP6), SVM (PLCG1, CHMP2B, TIRAP, BAK1, and NLRP6), XGB (CASP1, IRF1, CHMP4A, NLRP7, and CHMP2B), and GLM (CASP4, IRF1, CHMP4A, HMGB1, and TP53). Among the four models, the SVM model exhibited the lowest “residual” (Figures 3A, B). We also summarized the feature importance distribution of pyroptosis genes in each machine learning algorithm (Figure 3C). The ROCs demonstrated excellent diagnostic efficacy of the SVM model with AUC = 1 (Figure 3D). The sensitivity of the RF, SVM, XGB, and GLM models was 1, 1, 1, and 0.75, respectively. The specificity of the four models was 0.75, 1, 0.5, and 0.25, respectively. Thus, the SVM was the best model, and the genes selected by the SVM were considered the key pyroptosis genes.
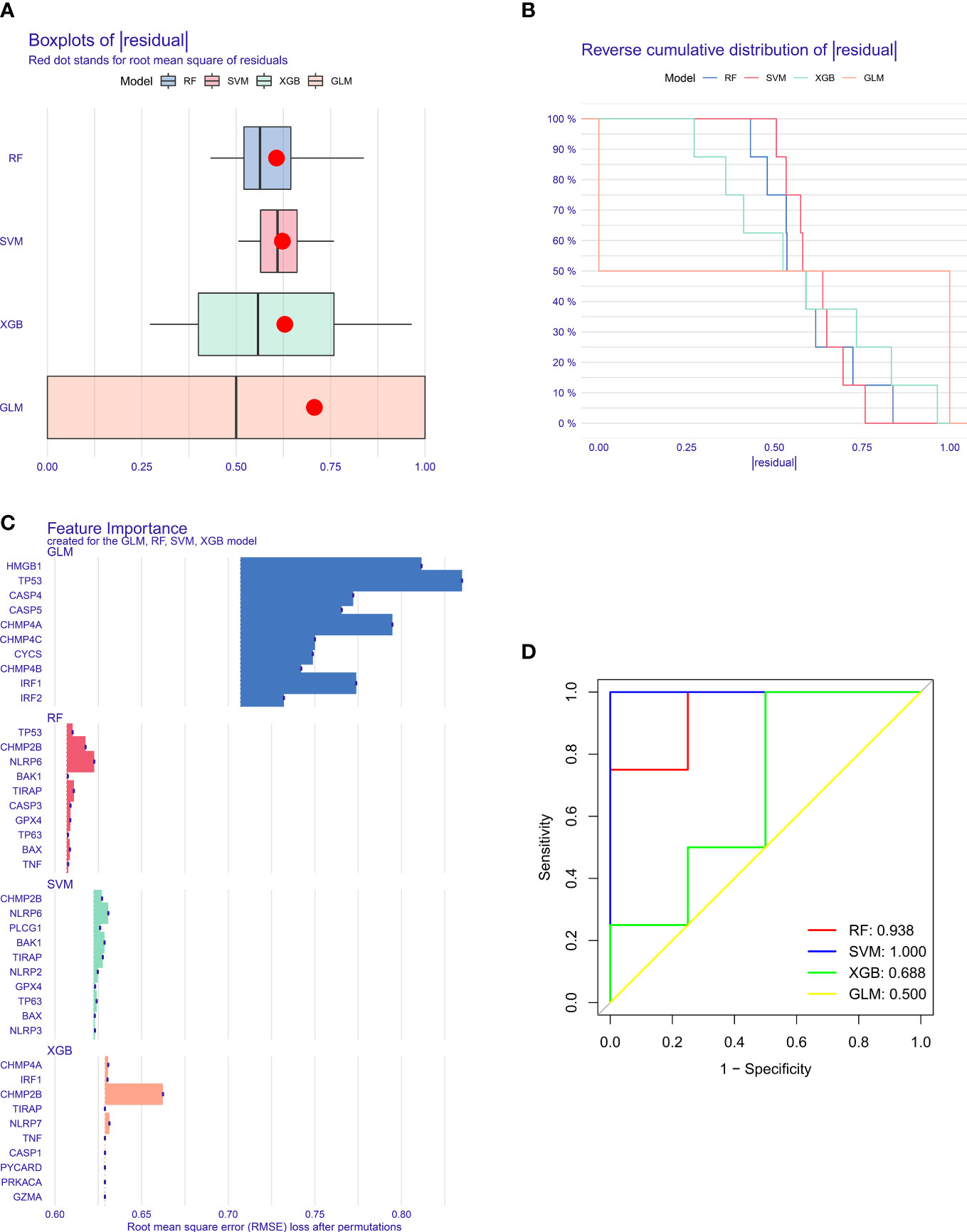
Figure 3 Selection of key pyroptosis genes through multiple machine learning approaches. (A) Box plots showing the “residual” of each machine learning method. (B) Reverse cumulative distribution of the “residual” for diverse machine learning approaches. (C) Feature importance of the selected pyroptosis genes by different machine learning approaches. (D) ROCs for the assessment of the diagnostic efficacy of distinct machine learning models.
Generation of a nomogram based upon key pyroptosis genes for T2DM risk
Five key pyroptosis genes were eventually selected for establishing the nomogram, composed of BAK1, CHMP2B, NLRP6, PLCG1, and TIRAP (Figure 4A). Calibration curves proved the good consistency between the nomogram-predictive results and the actual observations (Figure 4B). For determining the clinical significance of the nomogram in daily clinical practice, we plotted DCA curves. As depicted in Figure 4C, in comparison to all of the patients or none of them, the application of the nomogram to predict the risk of T2DM might be reasonable and have more clinical net benefit in accordance with the predicted possibilities computed by the nomogram and threshold probabilities.
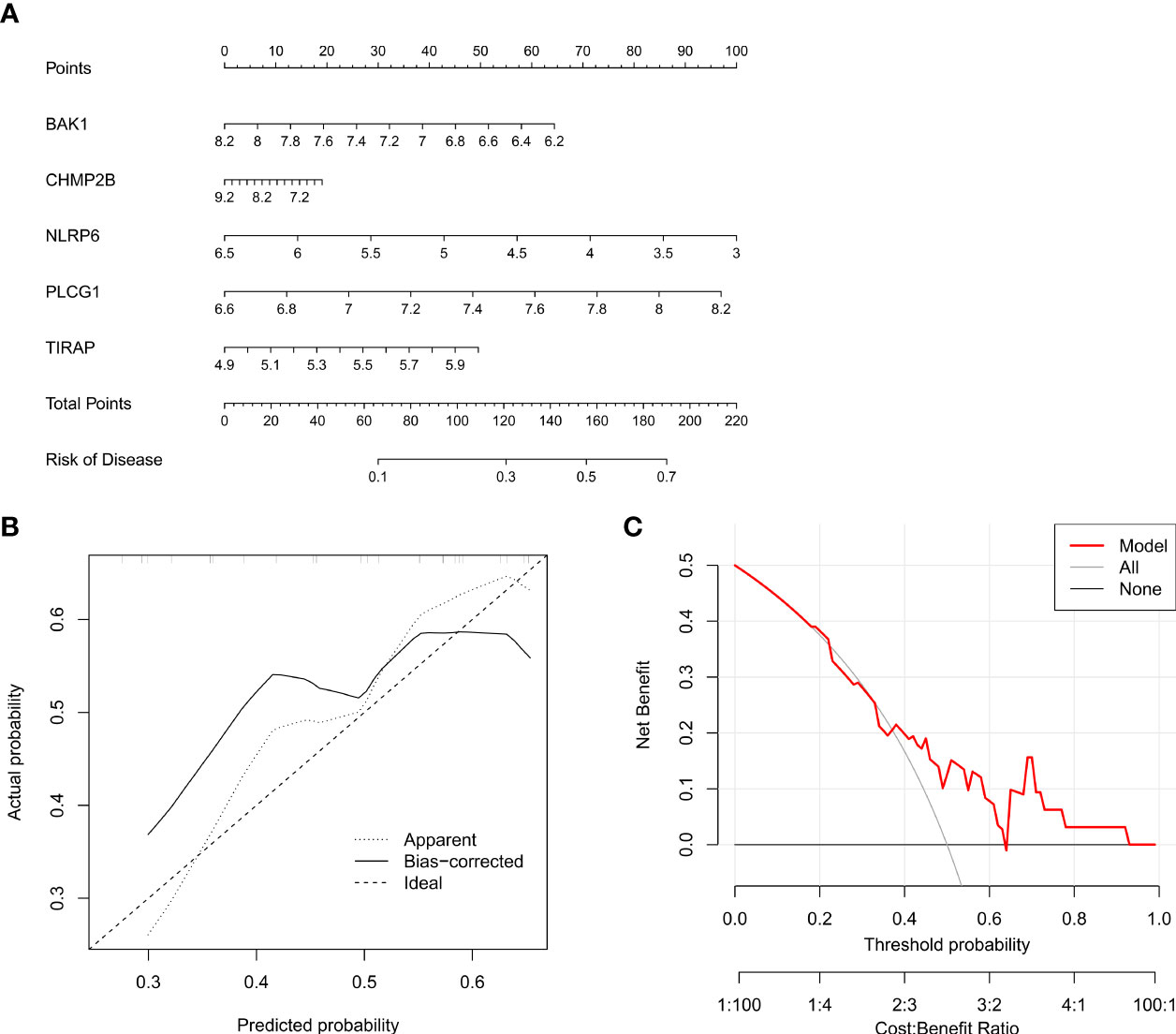
Figure 4 Generation of a nomogram for T2DM based upon key pyroptosis genes. (A) The nomogram comprising BAK1, CHMP2B, NLRP6, PLCG1, and TIRAP. (B) Calibration curves showing the consistency between the nomogram-predicted results and actual observations. (C) DCA curves for the evaluation of the clinical net benefit.
Verification of the diagnostic efficacy of the SVM model
The excellent diagnostic efficacy of the SVM model was also proven in the GSE26168 dataset (AUC = 1; Figure 5A). In addition, this work investigated the diagnostic performance of each key pyroptosis gene. It was demonstrated that BAK1, CHMP2B, NLRP6, PLCG1, and TIRAP can individually diagnose T2DM with relatively high AUC values (Figures 5B–F). This demonstrated the crucial significance of key pyroptosis genes in T2DM.
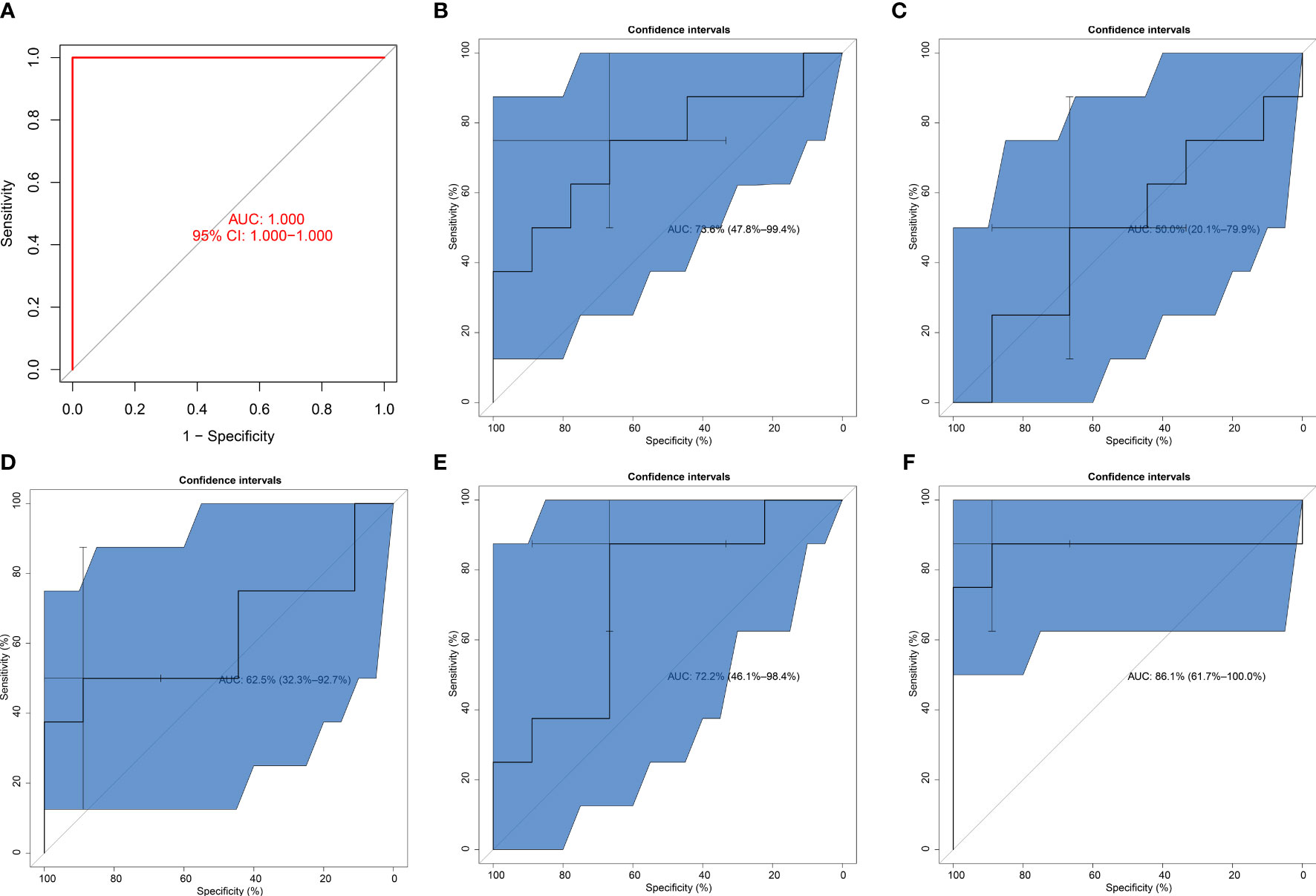
Figure 5 Verification of the diagnostic efficacy of the SVM model. (A) External verification of the diagnostic performance of the SVM model in the GSE26168 dataset. (B–F) ROCs of (B) BAK1, (C) CHMP2B, (D) NLRP6, (E) PLCG1, and (F) TIRAP in diagnosing T2DM.
Molecular mechanisms underlying key pyroptosis genes and their correlations with clinical features
GSEA unveiled that BAK1 was negatively connected with basal cell carcinoma, taurine and hypotaurine metabolism, epithelial cell signaling in Helicobacter pylori infection, and maturity-onset diabetes of the young (Figure 6A). CHMP2B was negatively related to the mTOR signaling pathway, renal cell carcinoma, progesterone-mediated oocyte maturation, and glycosphingolipid biosynthesis of lacto- and neolacto-series (Figure 6B). NLRP6 exhibited positive interactions with hematopoietic cell lineage, basal cell carcinoma, galactose metabolism, hedgehog signaling pathway, epithelial cell signaling in H. pylori infection, and oxidative phosphorylation (Figure 6C). PLCG1 was negatively linked with peroxisome, fatty acid metabolism, primary bile acid biosynthesis, propanoate metabolism, O-glycan biosynthesis, and PPAR signaling pathway (Figure 6D). TIRAP presented positive connections with Vibrio cholerae infection, long-term potentiation, and hedgehog signaling pathway (Figure 6E).
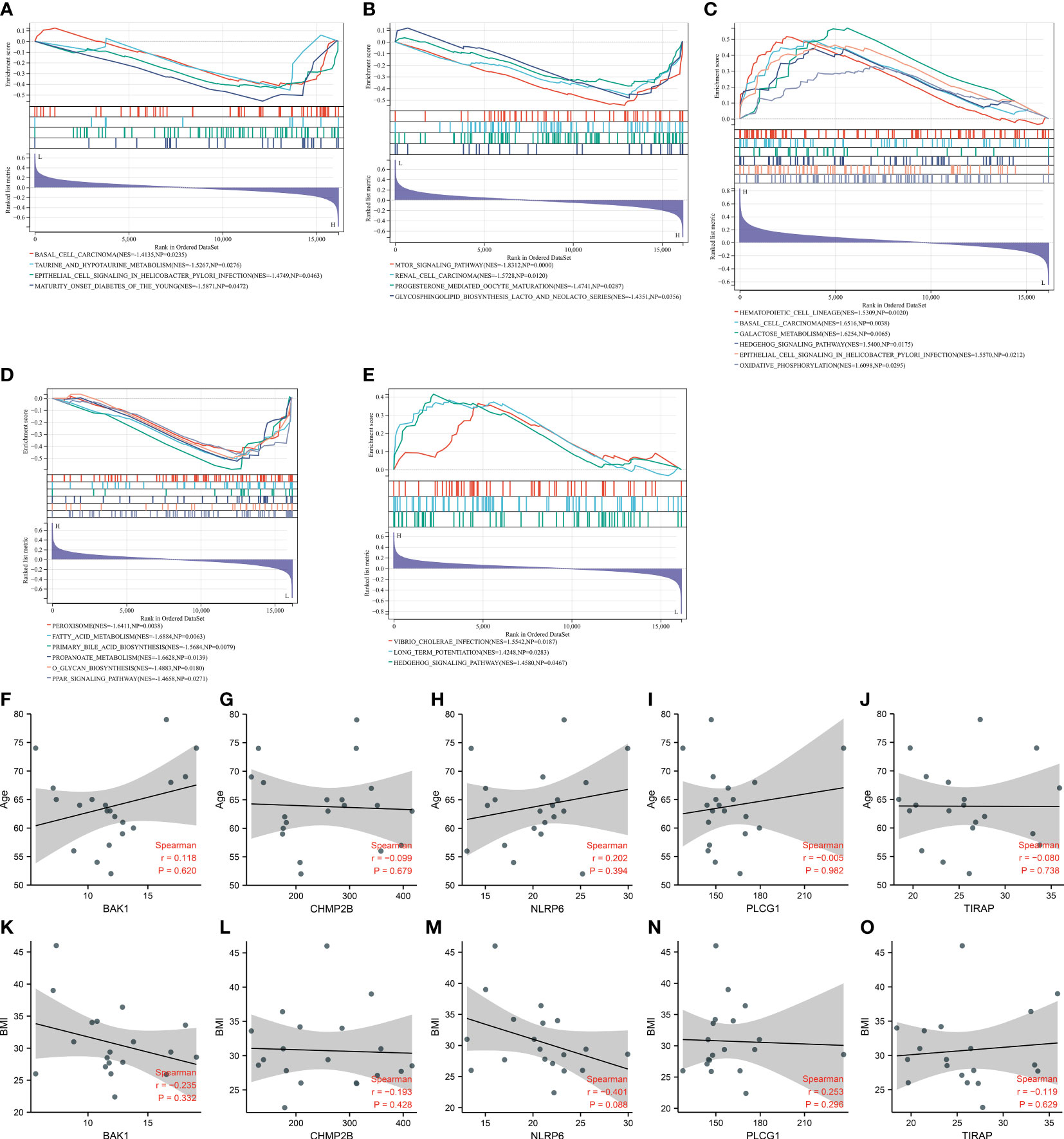
Figure 6 Molecular mechanisms underlying key pyroptosis genes. (A–E) GSEA for the difference in enriched KEGG pathways between low and high expression of (A) BAK1, (B) CHMP2B, (C) NLRP6, (D) PLCG1, and (E) TIRAP. (F–J) Correlation analysis on (F) BAK1, (G) CHMP2B, (H) NLRP6, (I) PLCG1, and (J) TIRAP with age. (K–O) Correlation analysis on (K) BAK1, (L) CHMP2B, (M) NLRP6, (N) PLCG1, and (O) TIRAP with BMI.
We also evaluated the correlations between the key pyroptosis genes and clinical features (age and BMI). Nevertheless, no significant associations between BAK1, CHMP2B, NLRP6, PLCG1, and TIRAP and age and BMI were observed among T2DM patients (Figures 6F–O).
Interactions of key pyroptosis genes with immune infiltration
Through the implementation of CIBERSORT, the fraction of diverse immune cell types was estimated across T2DM and control tissues (Figure 7A; Supplementary Table 1). Their difference was also investigated between the two groups. As illustrated in Figure 7B, natural killer (NK) cells resting exhibited lower abundance in T2DM relative to the normal specimens. In contrast, the higher abundance of neutrophils was investigated in T2DM. Among the key pyroptosis genes, NLRP6 was positively connected with mast cells activated and neutrophils (Figure 7C). In addition, CHMP2B had a positive interaction with B cells naive. The assessment of the interactions between key pyroptosis genes was also conducted. In Figure 7D, CHMP2B negatively interacted with BAK1 and PLCG1, while the other genes presented positive interactions.
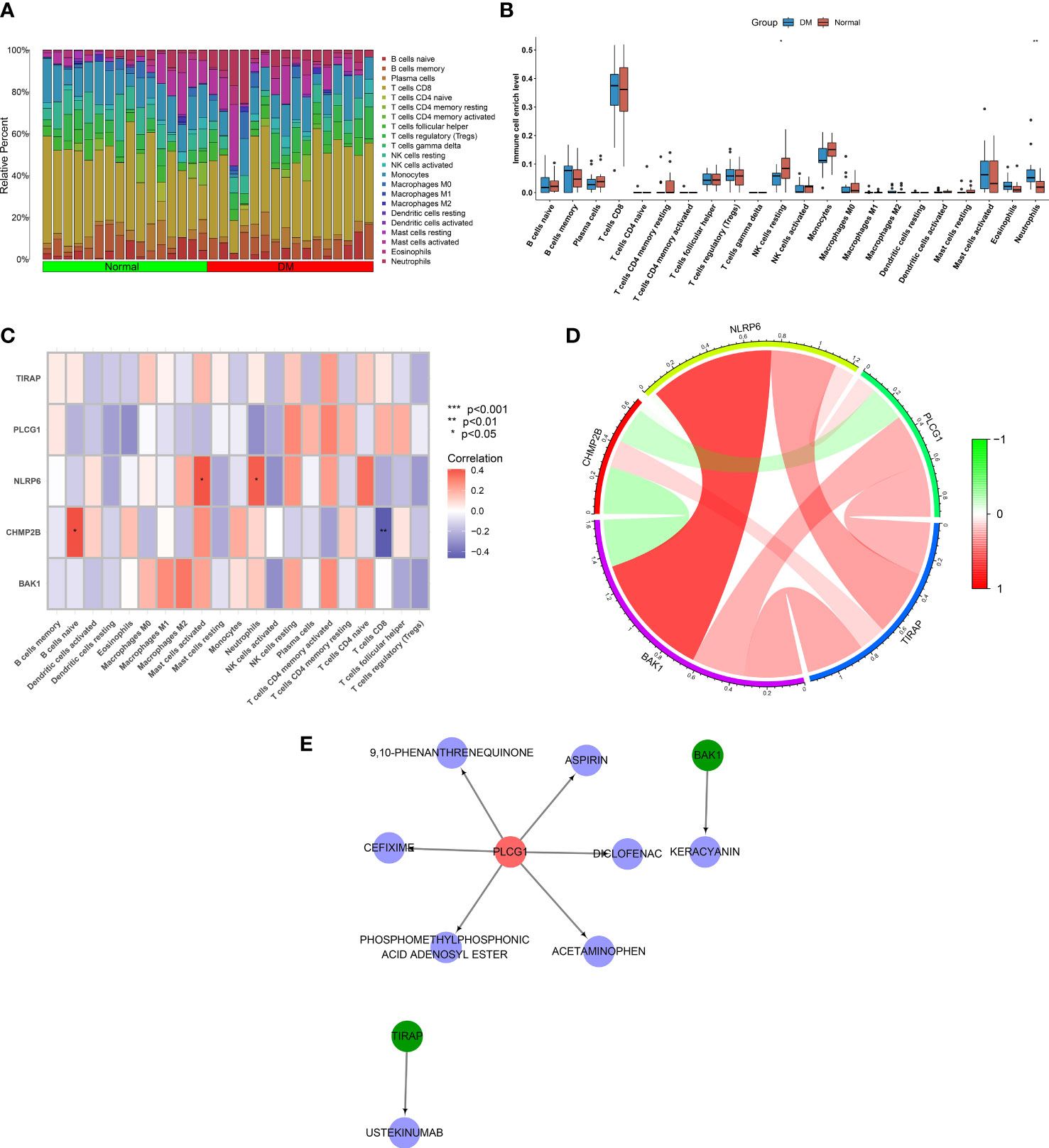
Figure 7 Associations of key pyroptosis genes with immune infiltration and generation of a drug–druggable target network. (A) Landscape of the fraction of immune components across T2DM and normal specimens. (B) Comparing the fraction of immune components in T2DM relative to controls. (C) Correlation analysis on key pyroptosis genes with immune infiltration. (D) Interactions between key pyroptosis genes. (E) A network of key pyroptosis genes with matched compounds. *p < 0.05; **p < 0.01.
Drug–druggable target network
Drugs that potentially targeted key pyroptosis genes were inferred utilizing the DGIdb. As a result, BAK1 was a druggable target of keracyanin; PLCG1 was a druggable target of 9,10-phenanthrenequinone, diclofenac, phosphomethylphosphonic acid adenosyl ester, acetaminophen, cefixime, and aspirin; and TIRAP was a druggable target of ustekinumab (Figure 7E).
Establishment of the key pyroptosis gene-relevant co-expression modules
To select the key pyroptosis gene-relevant co-expression modules, this work adopted the WGCNA (Figure 8A). The appropriate soft thresholding value was set to 13 based on the scale independence as well as mean connectivity (Figure 8B). By using the dynamic tree cut method, eight co-expression modules were built (Figures 8C, D). Among them, the turquoise module exhibited the strongest interaction with the key pyroptosis gene CHMP2B (Figure 8E). The genes in the turquoise module were regarded as CHMP2B-relevant genes (Figure 8F; Supplementary Table 2).
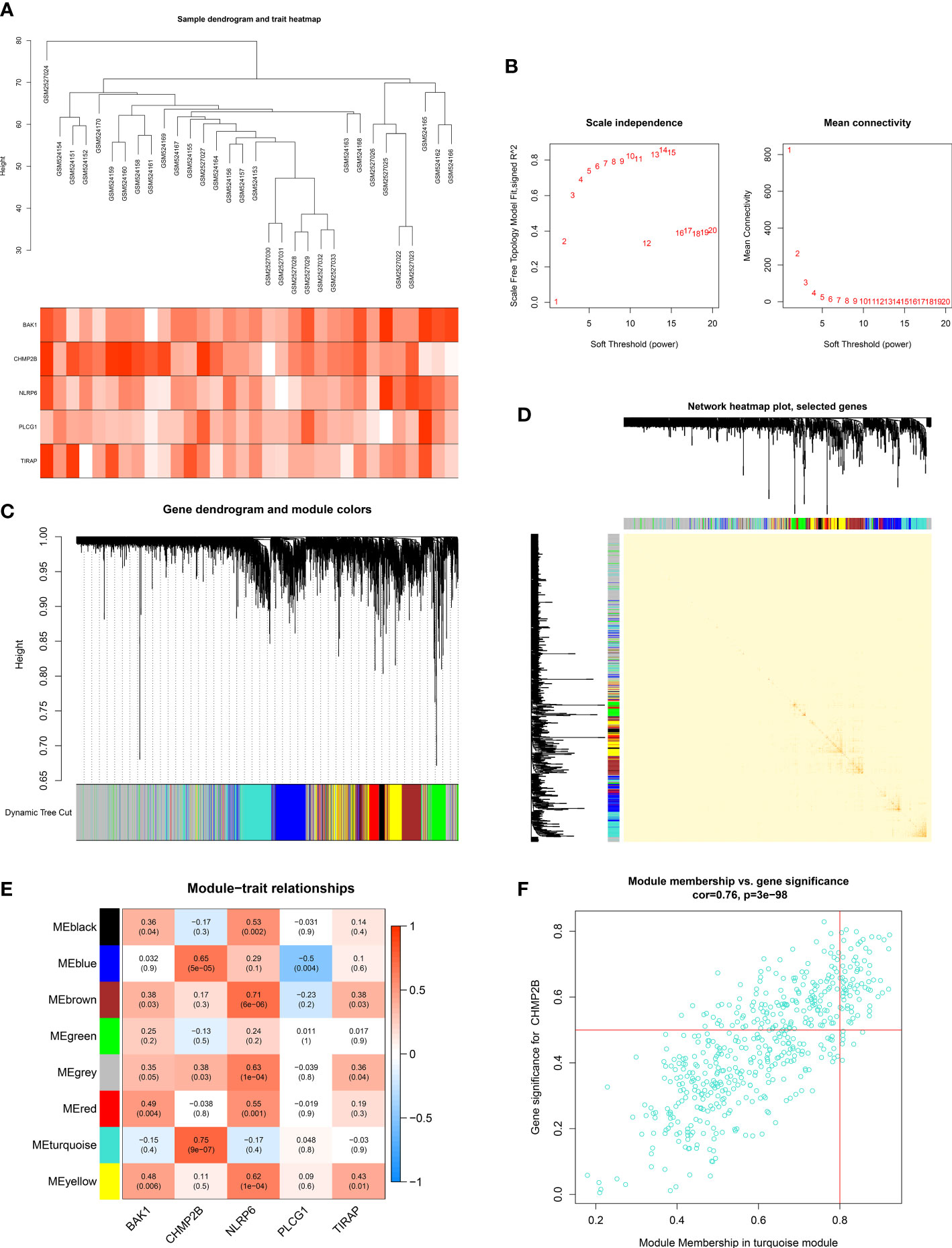
Figure 8 Establishment of key pyroptosis gene-relevant co-expression modules. (A) Sample dendrogram (upper) and heatmap of key pyroptosis genes (below). (B) Selection of the appropriate soft thresholding value in accordance with scale independence as well as mean connectivity. (C) Gene dendrogram and merged modules. (D) The network heatmap plot. (E) Pearson correlation on the established modules with key pyroptosis genes. Red, positive interaction; blue, negative interaction. (F) Scatter plot of the module membership in the turquoise module versus gene significance for CHMP2B.
Interactions between key CHMP2B-relevant genes and their biological implications
To determine the key CHMP2B-relevant genes, the MCODE method was adopted (Supplementary Table 3). As a result, 10 key genes were acquired as follows: KNTC1, NCAPG, KIAA0101, DLGAP5, GMNN, CEP55, KIF20B, ZWILCH, MCM6, and MAD2L1 (Figure 9A). Most of the genes presented differential expression in T2DM relative to the normal specimens (Figure 9B). The biological significance of CHMP2B-relevant genes was further probed. It was noted that they were notably connected with proteasome-mediated ubiquitin-dependent protein catabolic process, proteasomal protein catabolic process, etc. (Figure 9C; Table 2). In addition, RNA transport, cell cycle, T-cell receptor pathway, etc. were remarkably enriched by CHMP2B-relevant genes (Figure 9D; Table 3).
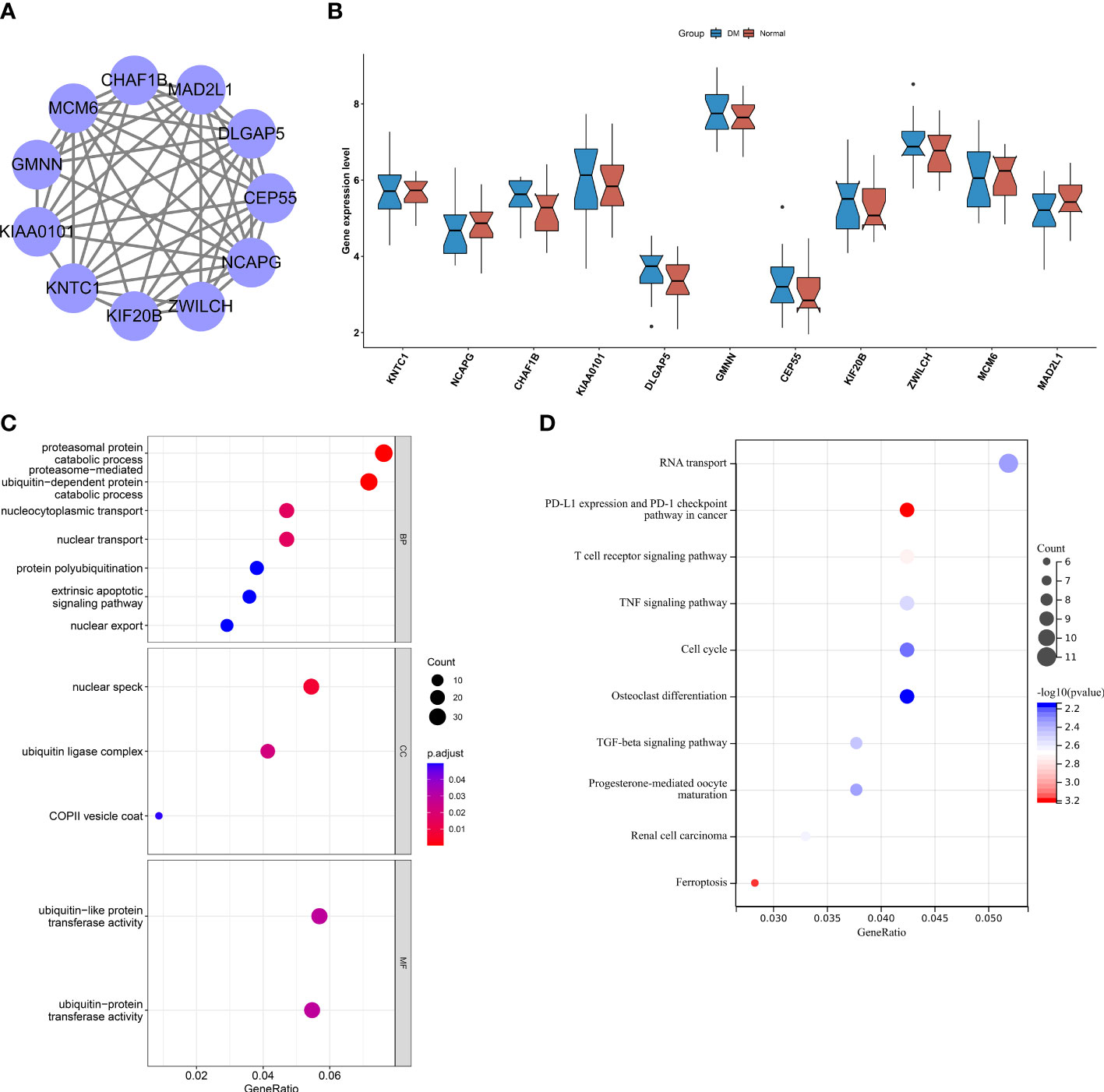
Figure 9 Interactions between key CHMP2B-relevant genes and their biological implications. (A) Protein interactions between key CHMP2B-relevant genes. (B) Transcript level of key CHMP2B-relevant genes in T2DM and control tissues. (C) GO enrichment results of key CHMP2B-relevant genes. (D) KEGG pathways enriched by CHMP2B-relevant genes.
Discussion
Pyroptosis, a proinflammatory form of programmed cell death, has the features of cellular swelling, lysis, and the release of proinflammatory cytokines (30). In the present work, most pyroptosis molecules containing CHMP4A, CHMP6, GSDMD, IL1B, IRF2, TP53, CASP9, NLRC4, NOD1, NOD2, and PYCARD presented remarkable upregulation in T2DM relative to controls, which was indicative of the activation of the pyroptosis process in T2DM, similar to prior research (31).
To select the key pyroptosis molecules exerting essential functions in T2DM, four machine learning algorithms—RF, SVM, XGB, and GLM—were applied. Among them, the SVM model presented the best efficacy in T2DM prediction. Therefore, SVM-selected genes were considered key pyroptosis molecules, including BAK1, CHMP2B, NLRP6, PLCG1, and TIRAP. The nomogram built had clinical superiority in risk prediction. Experimental research has demonstrated that the key pyroptosis genes are involved in DM. Targeting BAK1 alleviates diabetic cardiomyopathy (32). In addition, inhibition of PLCG1 mitigates diabetic retinopathy (33). TIRAP is associated with T2DM and insulin resistance (34).
T2DM is associated with increased systemic inflammation that results in insulin resistance, hyperglycemia, and risk of diabetic complications (35). Herein, it was found that T2DM displayed a lower level of NK cells resting as well as a higher level of neutrophils in comparison to normal specimens, consistent with prior research (36). It has been proven that NK cells correlate to DM by relieving systemic inflammation and enhancing cellular insulin sensitivity (37). Neutrophils are probably the dominating leukocytes in the innate arm of the immune system considering the response to damage and danger signals, which are the first leukocytes reacting to and accumulating inside the target tissues of DM (38). NLRP6 presented a positive connection with neutrophils as previously reported (39).
Based upon the DGIdb, possible compounds potentially targeting key pyroptosis genes were determined, comprising keracyanin, 9,10-phenanthrenequinone, diclofenac, phosphomethylphosphonic acid adenosyl ester, acetaminophen, cefixime, aspirin, and ustekinumab. Prior research has proposed that aspirin pretreatment mitigates inflammasome-driven pyroptosis through downregulating NF-κB/NLRP3 signaling in ischemic stroke (40). Nevertheless, experimental verification needs to be carried out for the interactions of these compounds with druggable pyroptosis molecules in T2DM.
This work determined 10 key CHMP2B-relevant genes utilizing the WGCNA along with the MCODE, namely, KNTC1, NCAPG, KIAA0101, DLGAP5, GMNN, CEP55, KIF20B, ZWILCH, MCM6, and MAD2L1. NCAPG and MAD2L1 have been demonstrated to be associated with DM and HCV-related hepatocellular carcinoma (41). DLGAP5 is connected with T2DM-attributed end-stage kidney disease among African Americans (42). Hypermethylation of KIF20B is found in the proximal tubules of diabetic kidney disease (43). Thus, prior research has unveiled the functions of key CHMP2B-relevant genes in T2DM.
Nevertheless, the limitations of this study should be pointed out. Firstly, the performance of the key pyroptosis gene-based nomogram in predicting the risk of T2DM should be validated in prospective cohorts. Secondly, the biological roles of the key pyroptosis genes in T2DM pathogenesis require to be further investigated through more experiments. Thirdly, the interactions between CHMP2B and its relevant genes should be further analyzed in T2DM.
Conclusion
In summary, this work proposed the key pyroptosis genes in T2DM by comparing distinct machine learning approaches, composed of BAK1, CHMP2B, NLRP6, PLCG1, and TIRAP. The key pyroptosis gene-based nomogram enabled to predict the risk of T2DM for clinical application. Possible compounds that targeted the key pyroptosis genes were screened. In addition, the key CHMP2B-relevant genes were KNTC1, NCAPG, KIAA0101, DLGAP5, GMNN, CEP55, KIF20B, ZWILCH, MCM6, and MAD2L1, which might interact with CHMP2B during T2DM. Altogether, our findings offered promising molecules for the management and therapy of T2DM and its complications.
Data availability statement
The datasets presented in this study can be found in online repositories. The names of the repository/repositories and accession number(s) can be found in the article/Supplementary Material.
Author contributions
MW conceived and designed the study. HW and RW conducted most of the experiments and data analysis and wrote the manuscript. YT and QC participated in collecting the data and helped to draft the manuscript. All authors contributed to the article and approved the submitted version.
Conflict of interest
The authors declare that the research was conducted in the absence of any commercial or financial relationships that could be construed as a potential conflict of interest.
Publisher’s note
All claims expressed in this article are solely those of the authors and do not necessarily represent those of their affiliated organizations, or those of the publisher, the editors and the reviewers. Any product that may be evaluated in this article, or claim that may be made by its manufacturer, is not guaranteed or endorsed by the publisher.
Supplementary material
The Supplementary Material for this article can be found online at: https://www.frontiersin.org/articles/10.3389/fendo.2023.1112507/full#supplementary-material
Supplementary Table 1 | Landscape of the fraction of immune cell types across T2DM and control specimens.
Supplementary Table 2 | The list of CHMP2B-relevant genes.
Supplementary Table 3 | Selection of key CHMP2B-relevant genes via MCODE method.
Abbreviations
DM, diabetes mellitus; T1DM, type 1 DM; TIDM, type 2 DM; PCA, principal component analysis; RF, random forest; SVM, support vector machine; XGB, extreme gradient boosting; GLM, generalized linear modeling; ROCs, receiver operating characteristic curves; AUC, area under the curve; DCA, decision curve analysis; GSEA, gene set enrichment analysis; DGIdb, Drug Gene Interaction Database; WGCNA, weighted correlation network analysis; MCODE, molecular complex detection; GO, Gene Ontology; BP, biological process; CC, cellular component; MF, molecular function; KEGG, Kyoto Encyclopedia of Genes and Genomes; NK, natural killer.
References
1. Zimmet P, Alberti KG, Magliano DJ, Bennett PH. Diabetes mellitus statistics on prevalence and mortality: facts and fallacies. Nat Rev Endocrinol (2016) 12(10):616–22. doi: 10.1038/nrendo.2016.105
2. Gardner CD, Landry MJ, Perelman D, Petlura C, Durand LR, Aronica L, et al. Effect of a ketogenic diet versus Mediterranean diet on glycated hemoglobin in individuals with prediabetes and type 2 diabetes mellitus: the interventional keto-med randomized crossover trial. Am J Clin Nutr (2022) 116(3):640–52. doi: 10.1093/ajcn/nqac154
3. Kadowaki T, Isendahl J, Khalid U, Lee SY, Nishida T, Ogawa W, et al. Semaglutide once a week in adults with overweight or obesity, with or without type 2 diabetes in an east Asian population (STEP 6): a randomised, double-blind, double-dummy, placebo-controlled, phase 3a trial. Lancet Diabetes Endocrinol (2022) 10(3):193–206. doi: 10.1016/s2213-8587(22)00008-0
4. Lam CSP, Ramasundarahettige C, Branch KRH, Sattar N, Rosenstock J, Pratley R, et al. Efpeglenatide and clinical outcomes with and without concomitant sodium-glucose cotransporter-2 inhibition use in type 2 diabetes: exploratory analysis of the AMPLITUDE-O trial. Circulation (2022) 145(8):565–74. doi: 10.1161/circulationaha.121.057934
5. Takeshita Y, Honda M, Harada K, Kita Y, Takata N, Tsujiguchi H, et al. Comparison of tofogliflozin and glimepiride effects on nonalcoholic fatty liver disease in participants with type 2 diabetes: a randomized, 48-week, open-label, active-controlled trial. Diabetes Care (2022) 45(9):2064–75. doi: 10.2337/dc21-2049
6. Wharton W, Anderson A, Hayden KM, Carmichael OT, Clark JM, Luchsinger JA, et al. Effect of renin-angiotensin system antihypertensive medication use on cognitive function in diabetes mellitus with obesity or overweight: an ancillary study to the action for health in diabetes (Look AHEAD) trial. Diabetes Obes Metab (2022) 24(12):2443–53. doi: 10.1111/dom.14838
7. Chen B, Yan Y, Yang Y, Cao G, Wang X, Wang Y, et al. A pyroptosis nanotuner for cancer therapy. Nat Nanotechnol (2022) 17(7):788–98. doi: 10.1038/s41565-022-01125-0
8. Niu X, Chen L, Li Y, Hu Z, He F. Ferroptosis, necroptosis, and pyroptosis in the tumor microenvironment: perspectives for immunotherapy of SCLC. Semin Cancer Biol (2022) 86(Pt 3):273–85. doi: 10.1016/j.semcancer.2022.03.009
9. Chen L, Yin Z, Qin X, Zhu X, Chen X, Ding G, et al. CD74 ablation rescues type 2 diabetes mellitus-induced cardiac remodeling and contractile dysfunction through pyroptosis-evoked regulation of ferroptosis. Pharmacol Res (2022) 176:106086. doi: 10.1016/j.phrs.2022.106086
10. Ruan Z, Li Y, Chen Y. HECTD3 promotes NLRP3 inflammasome and pyroptosis to exacerbate diabetes-related cognitive impairment by stabilising MALT1 to regulate JNK pathway. Arch Physiol Biochem (2022), 1–12. doi: 10.1080/13813455.2022.2093377
11. Yan M, Li Y, Luo Q, Zeng W, Shao X, Li L, et al. Mitochondrial damage and activation of the cytosolic DNA sensor cGAS-STING pathway lead to cardiac pyroptosis and hypertrophy in diabetic cardiomyopathy mice. Cell Death Discovery (2022) 8(1):258. doi: 10.1038/s41420-022-01046-w
12. Gao Y, Ma Y, Xie D, Jiang H. ManNAc protects against podocyte pyroptosis via inhibiting mitochondrial damage and ROS/NLRP3 signaling pathway in diabetic kidney injury model. Int Immunopharmacol (2022) 107:108711. doi: 10.1016/j.intimp.2022.108711
13. Wang X, Li Q, Sui B, Xu M, Pu Z, Qiu T. Schisandrin a from schisandra chinensis attenuates ferroptosis and NLRP3 inflammasome-mediated pyroptosis in diabetic nephropathy through mitochondrial damage by AdipoR1 ubiquitination. Oxid Med Cell Longev (2022) 2022:5411462. doi: 10.1155/2022/5411462
14. Marselli L, Thorne J, Dahiya S, Sgroi DC, Sharma A, Bonner-Weir S, et al. Gene expression profiles of beta-cell enriched tissue obtained by laser capture microdissection from subjects with type 2 diabetes. PloS One (2010) 5(7):e11499. doi: 10.1371/journal.pone.0011499
15. Luo L, Zhou WH, Cai JJ, Feng M, Zhou M, Hu SP, et al. Gene expression profiling identifies downregulation of the neurotrophin-MAPK signaling pathway in female diabetic peripheral neuropathy patients. J Diabetes Res (2017) 2017:8103904. doi: 10.1155/2017/8103904
16. Leek JT, Johnson WE, Parker HS, Jaffe AE, Storey JD. The sva package for removing batch effects and other unwanted variation in high-throughput experiments. Bioinformatics (2012) 28(6):882–3. doi: 10.1093/bioinformatics/bts034
17. Karolina DS, Armugam A, Tavintharan S, Wong MT, Lim SC, Sum CF, et al. MicroRNA 144 impairs insulin signaling by inhibiting the expression of insulin receptor substrate 1 in type 2 diabetes mellitus. PloS One (2011) 6(8):e22839. doi: 10.1371/journal.pone.0022839
18. Deng M, Sun S, Zhao R, Guan R, Zhang Z, Li S, et al. The pyroptosis-related gene signature predicts prognosis and indicates immune activity in hepatocellular carcinoma. Mol Med (2022) 28(1):16. doi: 10.1186/s10020-022-00445-0
19. Lu H, Wu J, Liang L, Wang X, Cai H. Identifying a novel defined pyroptosis-associated long noncoding RNA signature contributes to predicting prognosis and tumor microenvironment of bladder cancer. Front Immunol (2022) 13:803355. doi: 10.3389/fimmu.2022.803355
20. Wang Z, Cao L, Zhou S, Lyu J, Gao Y, Yang R. Construction and validation of a novel pyroptosis-related four-lncRNA prognostic signature related to gastric cancer and immune infiltration. Front Immunol (2022) 13:854785. doi: 10.3389/fimmu.2022.854785
21. Chen L, Niu X, Qiao X, Liu S, Ma H, Shi X, et al. Characterization of interplay between autophagy and ferroptosis and their synergistical roles on manipulating immunological tumor microenvironment in squamous cell carcinomas. Front Immunol (2021) 12:739039. doi: 10.3389/fimmu.2021.739039
22. Subramanian A, Tamayo P, Mootha VK, Mukherjee S, Ebert BL, Gillette MA, et al. Gene set enrichment analysis: a knowledge-based approach for interpreting genome-wide expression profiles. Proc Natl Acad Sci U.S.A. (2005) 102(43):15545–50. doi: 10.1073/pnas.0506580102
23. Liberzon A, Birger C, Thorvaldsdóttir H, Ghandi M, Mesirov JP, Tamayo P. The molecular signatures database (MSigDB) hallmark gene set collection. Cell Syst (2015) 1(6):417–25. doi: 10.1016/j.cels.2015.12.004
24. Newman AM, Liu CL, Green MR, Gentles AJ, Feng W, Xu Y, et al. Robust enumeration of cell subsets from tissue expression profiles. Nat Methods (2015) 12(5):453–7. doi: 10.1038/nmeth.3337
25. Freshour SL, Kiwala S, Cotto KC, Coffman AC, McMichael JF, Song JJ, et al. Integration of the drug-gene interaction database (DGIdb 4.0) with open crowdsource efforts. Nucleic Acids Res (2021) 49(D1):D1144–d1151. doi: 10.1093/nar/gkaa1084
26. Shannon P, Markiel A, Ozier O, Baliga NS, Wang JT, Ramage D, et al. Cytoscape: a software environment for integrated models of biomolecular interaction networks. Genome Res (2003) 13(11):2498–504. doi: 10.1101/gr.1239303
27. Langfelder P, Horvath S. WGCNA: an r package for weighted correlation network analysis. BMC Bioinf (2008) 9:559. doi: 10.1186/1471-2105-9-559
28. Szklarczyk D, Gable AL, Nastou KC, Lyon D, Kirsch R, Pyysalo S, et al. The STRING database in 2021: customizable protein-protein networks, and functional characterization of user-uploaded gene/measurement sets. Nucleic Acids Res (2021) 49(D1):D605–d612. doi: 10.1093/nar/gkaa1074
29. Yu G, Wang LG, Han Y, He QY. clusterProfiler: an r package for comparing biological themes among gene clusters. Omics (2012) 16(5):284–7. doi: 10.1089/omi.2011.0118
30. Liu X, Zhang M, Liu H, Zhu R, He H, Zhou Y, et al. Bone marrow mesenchymal stem cell-derived exosomes attenuate cerebral ischemia-reperfusion injury-induced neuroinflammation and pyroptosis by modulating microglia M1/M2 phenotypes. Exp Neurol (2021) 341:113700. doi: 10.1016/j.expneurol.2021.113700
31. Liu BH, Tu Y, Ni GX, Yan J, Yue L, Li ZL, et al. Total flavones of abelmoschus manihot ameliorates podocyte pyroptosis and injury in high glucose conditions by targeting METTL3-dependent m(6)A modification-mediated NLRP3-inflammasome activation and PTEN/PI3K/Akt signaling. Front Pharmacol (2021) 12:667644. doi: 10.3389/fphar.2021.667644
32. Wang X, Zhang Z, Wang M. MiR-29a regulates cardiomyocyte apoptosis by targeting Bak1 in diabetic cardiomyopathy. J Biochem (2022) 171(6):663–71. doi: 10.1093/jb/mvac025
33. He Y, Zhang Z, Yao T, Huang L, Gan J, Lv H, et al. Extracellular vesicles derived from human umbilical cord mesenchymal stem cells relieves diabetic retinopathy through a microRNA-30c-5p-dependent mechanism. Diabetes Res Clin Pract (2022) 190:109861. doi: 10.1016/j.diabres.2022.109861
34. Degirmenci I, Ozbayer C, Kebapci MN, Kurt H, Colak E, Gunes HV. Common variants of genes encoding TLR4 and TLR4 pathway members TIRAP and IRAK1 are effective on MCP1, IL6, IL1β, and TNFα levels in type 2 diabetes and insulin resistance. Inflammation Res (2019) 68(9):801–14. doi: 10.1007/s00011-019-01263-7
35. Kimball A, Schaller M, Joshi A, Davis FM, denDekker A, Boniakowski A, et al. Ly6C(Hi) blood Monocyte/Macrophage drive chronic inflammation and impair wound healing in diabetes mellitus. Arterioscler Thromb Vasc Biol (2018) 38(5):1102–14. doi: 10.1161/atvbaha.118.310703
36. Lin J, Lu Y, Wang B, Jiao P, Ma J. Analysis of immune cell components and immune-related gene expression profiles in peripheral blood of patients with type 1 diabetes mellitus. J Transl Med (2021) 19(1):319. doi: 10.1186/s12967-021-02991-3
37. Wang Y, Li M, Chen L, Bian H, Chen X, Zheng H, et al. Natural killer cell-derived exosomal miR-1249-3p attenuates insulin resistance and inflammation in mouse models of type 2 diabetes. Signal Transduct Target Ther (2021) 6(1):409. doi: 10.1038/s41392-021-00805-y
38. Giovenzana A, Carnovale D, Phillips B, Petrelli A, Giannoukakis N. Neutrophils and their role in the aetiopathogenesis of type 1 and type 2 diabetes. Diabetes Metab Res Rev (2022) 38(1):e3483. doi: 10.1002/dmrr.3483
39. Lima C, Falcao MAP, Andrade-Barros AI, Seni-Silva AC, Grund LZ, Balogh E, et al. Natterin an aerolysin-like fish toxin drives IL-1β-dependent neutrophilic inflammation mediated by caspase-1 and caspase-11 activated by the inflammasome sensor NLRP6. Int Immunopharmacol (2021) 91:107287. doi: 10.1016/j.intimp.2020.107287
40. Li F, Xu D, Hou K, Gou X, Lv N, Fang W, et al. Pretreatment of indobufen and aspirin and their combinations with clopidogrel or ticagrelor alleviates inflammasome mediated pyroptosis Via inhibiting NF-κB/NLRP3 pathway in ischemic stroke. J Neuroimmune Pharmacol (2021) 16(4):835–53. doi: 10.1007/s11481-020-09978-9
41. Sufyan M, Ali Ashfaq U, Ahmad S, Noor F, Hamzah Saleem M, Farhan Aslam M, et al. Identifying key genes and screening therapeutic agents associated with diabetes mellitus and HCV-related hepatocellular carcinoma by bioinformatics analysis. Saudi J Biol Sci (2021) 28(10):5518–25. doi: 10.1016/j.sjbs.2021.07.068
42. Guan M, Keaton JM, Dimitrov L, Hicks PJ, Xu J, Palmer ND, et al. An exome-wide association study for type 2 diabetes-attributed end-stage kidney disease in African americans. Kidney Int Rep (2018) 3(4):867–78. doi: 10.1016/j.ekir.2018.03.002
Keywords: type 2 diabetes mellitus, pyroptosis genes, machine learning, support vector machine, immune infiltration, druggable targets, risk
Citation: Wang M, Wu H, Wu R, Tan Y and Chang Q (2023) Application of multiple machine learning approaches to determine key pyroptosis molecules in type 2 diabetes mellitus. Front. Endocrinol. 14:1112507. doi: 10.3389/fendo.2023.1112507
Received: 05 December 2022; Accepted: 15 June 2023;
Published: 19 July 2023.
Edited by:
Jiandong Zhou, University of Oxford, United KingdomReviewed by:
Elena Rampanelli, Amsterdam University Medical Center, NetherlandsUsharani Thirunavukkarasu, Saveetha University, India
Vijander Singh, Netaji Subhas University of Technology, India
Copyright © 2023 Wang, Wu, Wu, Tan and Chang. This is an open-access article distributed under the terms of the Creative Commons Attribution License (CC BY). The use, distribution or reproduction in other forums is permitted, provided the original author(s) and the copyright owner(s) are credited and that the original publication in this journal is cited, in accordance with accepted academic practice. No use, distribution or reproduction is permitted which does not comply with these terms.
*Correspondence: Min Wang, lwyytys@163.com