- 1The Key Laboratory of Geriatrics, Beijing Institute of Geriatrics, Institute of Geriatric Medicine, Chinese Academy of Medical Science, Beijing Hospital/National Center of Gerontology of National Health Commission, Beijing, China
- 2Shenzhen Institute of Synthetic Biology, Shenzhen Institute of Advanced Technology, Chinese Academy of Sciences, Shenzhen, China
- 3Department of Laboratory Medicine, Beijing Hospital, National Center of Gerontology, National Health Commission, Institute of Geriatric Medicine, Chinese Academy of Medical Sciences, Beijing, China
Background: Diabetes mellitus (DM), a metabolic disease that has attracted significant research and clinical attention over the years, can affect the eye structure and induce cataract in patients diagnosed with DM. Recent studies have indicated the relationship between glycoprotein non-metastatic melanoma protein B (GPNMB) and DM and DM-related renal dysfunction. However, the role of circulating GPNMB in DM-associated cataract is still unknown. In this study, we explored the potential of serum GPNMB as a biomarker for DM and DM-associated cataract.
Methods: A total of 406 subjects were enrolled, including 60 and 346 subjects with and without DM, respectively. The presence of cataract was evaluated and serum GPNMB levels were measured using a commercial enzyme-linked immunosorbent assay kit.
Results: Serum GPNMB levels were higher in diabetic individuals and subjects with cataract than in those without DM or cataract. Subjects in the highest GPNMB tertile group were more likely to have metabolic disorder, cataract, and DM. Analysis performed in subjects with DM elucidated the correlation between serum GPNMB levels and cataract. Receiver operating characteristic (ROC) curve analysis also indicated that GPNMB could be used to diagnose DM and cataract. Multivariable logistic regression analysis illustrated that GPNMB levels were independently associated with DM and cataract. DM was also found to be an independent risk factor for cataract. Further surveys revealed the combination of serum GPNMB levels and presence of DM was associated with a more precise identification of cataract than either factor alone.
Conclusions: Increased circulating GPNMB levels are associated with DM and cataract and can be used as a biomarker of DM-associated cataract.
Introduction
Diabetes mellitus (DM) is a metabolic disease characterized by high blood glucose levels. Owing to the rapidly increasing numbers of diabetic patients worldwide, it has become one of the most common and insidious chronic diseases with an estimated 4.2 million deaths among 20–79-year-old adults in 2019 (1, 2). In the Middle East and North Africa region, 16.2% of all-cause deaths are attributable to DM. In the South-East Asia and Western Pacific regions, the number of DM-related deaths in 2019 was 1,150,344 and 1,265,051, respectively (2). In addition, another study in 2021 showed that almost a half of all 20–79-year-old adults with DM were unaware of their diabetic status (3). Thus, advances in the study of biomarkers and mechanism are urgently needed for the prediction and therapy for DM.
The progression of DM are often accompanied by several complications such as acute kidney injury, cardiovascular diseases, heart failure, muscle infarction, and cognitive dysfunction (4–8). DM also affects ocular structures, contributes to the pathogenesis of cataract, and causes visual impairment in diabetic patients (9). According to the World Health Organization, cataract is an opacity of the lens with corrected visual acuity less than 0.7. Reports have regarded cataract as the leading cause of blindness globally and indicate that most cases of blindness cases from cataract occur in low- and middle-income countries (10–12). Globally, cost utility values for intraocular lens (IOL) implant surgery, one of the cost-effective treatments for cataract, remain considerable and cause a heavy public health burden (13). However, except for ocular examination, effective molecular biomarkers from blood indices are rarely discovered for the detection of cataract.
Glycoprotein non-metastatic melanoma protein B (GPNMB), also known as osteoactivin given its role in osteopetrosis in rats (14), is widely expressed in various types of cells such as macrophages (15), dendritic cells (16), osteoblasts (17), melanocytes (18), neurons (19) and hepatocytes (20). GPNMB contains an integrin-binding domain and extracellular heparin which contributes to binding to several types of cells such as vascular endothelial cells, keratinocytes, melanoma cells, fibroblasts and T cells (21–24). Study has identified the role of liver-secreted GPNMB in exacerbating obesity and insulin resistance by promoting lipogenesis (20). Cao C et al.’s (25) research has revealed the correlation of circulating levels of GPNMB with gestational DM. Moreover, the expression level of GPNMB is reportedly associated with type 1 DM-related renal function decline (26). Nevertheless, little is known about the role of GPNMB in diabetic cataract.
We investigated the role of GPNMB as a potential biomarker for DM-related cataract based on the data and samples collected from an ongoing cohort study—China Aging Longitudinal Study (CALS)—that enrolled a total of 26,000 healthy Chinese residents from seven geographic areas with the aim to investigate health and aging trends in China. The serum concentrations of GPNMB were measured, and some indicators of physical examination were analyzed.
Materials and methods
Study population
Subjects aged ≥25 years and without psychiatric disorders and alcohol or drug abuse were enrolled in CALS. From this existing cohort, we enrolled subjects from Long Tanhu Community in North China (426 participants) and excluded the following: subjects who required acute medical treatment or hospitalization within the first 3 months of GPNMB measurement (3 participants); those with severe diseases including cardiac, hepatic, or renal disease, and respiratory failure (10 participants); those unable to walk independently (2 participants); and those previously diagnosed with dementia (2 participants) and cancers (3 participants).
Finally, a total of 406 participants (155 men and 251 women; with DM=60, without DM=346) were included in this study. All subjects signed the informed consent form. Among these, 21.43% participants were diagnosed with cataract.
Clinical and biochemical measurements
Participants who had an opacity in the lens or were previously diagnosed with cataract were defined as the cataract group. Diabetes mellitus was defined by fasting serum glucose ≥126 mg/dL and/or glycosylated hemoglobin (HbA1c) ≥6.5% or those requiring treatment with anti-diabetes medication. All subjects were required to fast for 8 h before screening and filled in questionnaires regarding medical history. Clinical characteristics such as height, weight, body mass index (BMI), and total body fat mass were obtained. Total body fat mass was detected with the body composition analyzer (TsingHua Tong Fang, BCA-2A). Body fat percentage (Fat%) was defined as total body fat mass divided by body weight. Fat mass index (FMI) was defined as total body fat mass divided by the height squared.
Concentrations of fasting blood glucose (GLU), total cholesterol (TC), total triglyceride (TG), high density lipoprotein-C (HDL-C), and low density lipoprotein-C (LDL-C) were assayed using enzymatic methods and detected by Hitachi Automatic Analyzer ((LABOSPECT 008 AS, Japan). Concentrations of insulin and folic acid (FOL) were assayed using a solid-phase enzyme-linked chemiluminescent immunoassay and detected by IMMULITE2000 Automatic Immune Analyzer (Siemens Healthcare Diagnostics, Inc.). HbA1c was measured by nitroblue tetrazolium method and detected by AU680 Automated Biochemical Instrument (Beckman Coulter, Inc.). Insulin resistance status was assessed using the homeostasis model assessment of insulin resistance (HOMA-IR) according to the following formula: fasting serum insulin (μU/mL) × fasting serum glucose (mmol/L)/22.5 (27, 28).
Serum samples preparation and measurement
After 8 h of overnight fasting, blood samples were obtained via venipuncture from the median cubital vein, left in vacutainer tubes with coagulant at room temperature to clot for 15-30 min, centrifuged to collect the supernatant, and finally stored at -80°C until further analysis. Serum GPNMB concentrations were determined with an enzyme-linked immunosorbent assay kit (ELH-Osteoactivin, RayBiotech, Inc, Norcross, GA, USA). The assay had a sensitivity of 45 ng/mL to human GPNMB. The intra-assay and inter-assay coefficients of variation were <10% and <12%, respectively. The assay detection range was 49.15–12000 pg/mL. Serum samples were diluted 10 times before detection and measured according to the manufacturer’s instructions.
Statistical analysis
Statistical analysis was performed using IBM SPSS Statistics for Mac (version 26.0; IBM Corporation, Armonk, NY, USA) and R script 4.1.0. Continuous variables were presented as mean ± SD or median (interquartile range), while categorical variables were expressed as percentages. Differences between the two groups were analyzed by Wilcoxon rank sum test or Student’s t-test. Comparison among three or more groups was performed using one-way analysis of variance (ANOVA) or Kruskal–Wallis H test. Categorical variables were compared using chi-squared test or Fisher’s exact test. The association of study variables with DM or cataract was analyzed by univariable and multivariable logistic regression. The area under the receiver-operating characteristic (ROC) curve was calculated to test its predictive discrimination of DM and cataract. The optimal cut-off value was determined using the maximum sum of sensitivity and specificity based on the Youden index. Two-sided values of P<0.05 were considered to indicate statistically significant differences.
Results
The clinical and biochemical characteristics of subjects with or without DM are shown in Table 1. Subjects with DM were older and had higher BMI, FMI, Fat%, HOMA-IR, HbA1c, TG, FOL, INS, blood glucose and serum GPNMB levels than those without DM. Further, the DM group had a higher proportion of subjects diagnosed with cataract than the non-DM group. HDL-C levels were also lower in the DM than non-DM group. Circulating serum GPNMB levels were higher in subjects with DM than those without DM (Figure 1). We further plotted ROC curves based on GPNMB levels which showed a predictive ability of 0.734 to identify DM (asymptotic significance: <0.001) (Figure 2). The optimal cut-off value of GPNMB was 12,820.057 pg/mL (88.3% sensitivity and 53.2% specificity) to detect DM.
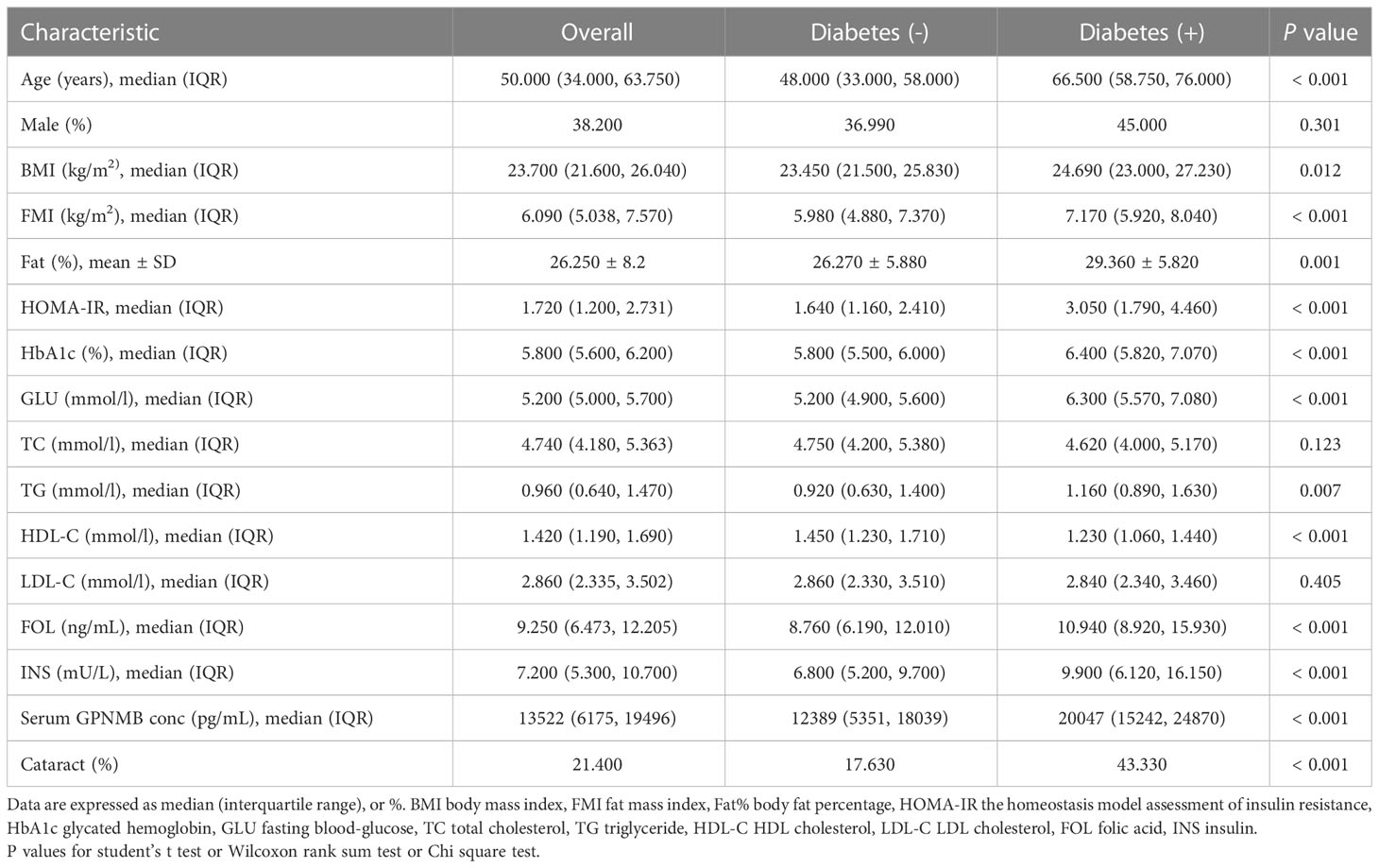
Table 1 Clinical and biochemical characteristics of study participants classified according to diabetes.
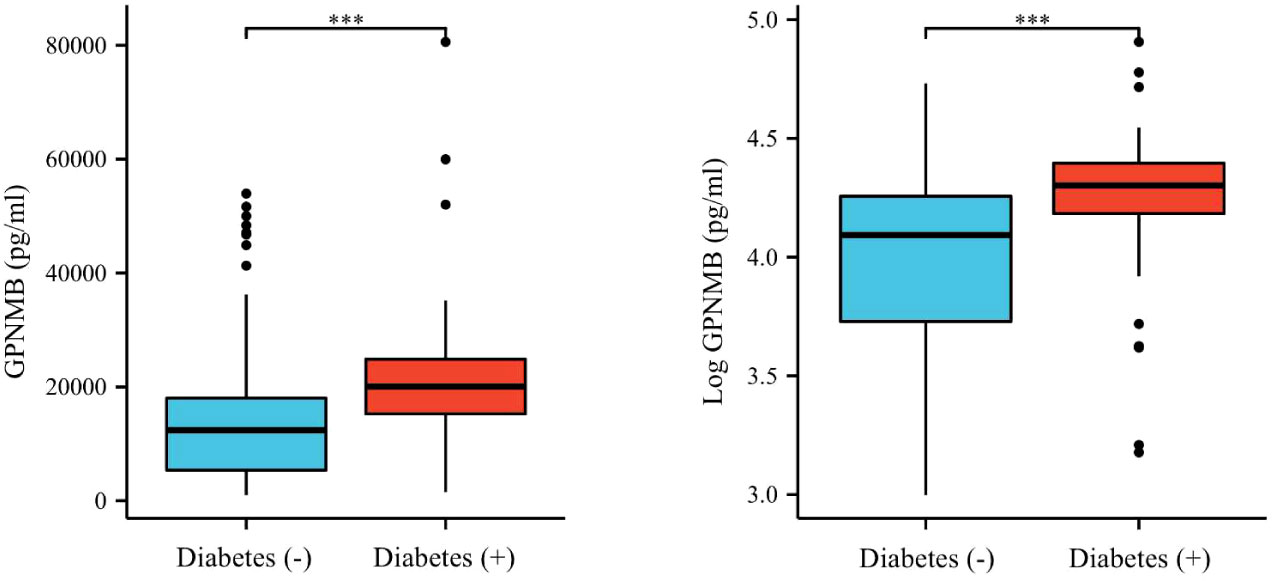
Figure 1 Plasma GPNMB levels depending on the existence of diabetes. ***P < 0.001 using a Wilcoxon rank sum test.
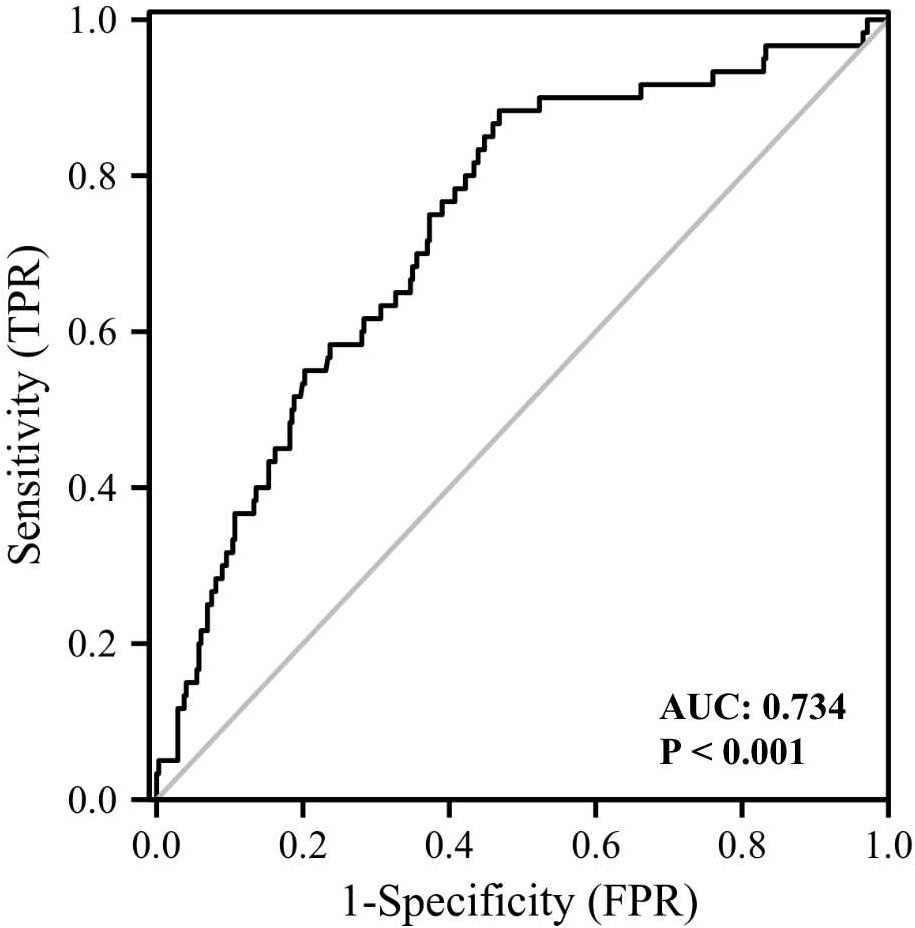
Figure 2 ROC curve analysis of the ability of plasma GPNMB to predict the presence of diabetes. AUC, area under curve.
We then compared the variables in subjects with or without cataract (Table 2). Those with cataract were older and had higher FMI, Fat%, HOMA-IR, HbA1c, TC, TG, FOL, INS, blood glucose and serum GPNMB levels than those without cataract. A higher proportion of subjects with DM were detected in the cataract group than the cataract-free group. Furthermore, we performed analysis in subjects with DM and found that DM-bearing participants tended to be older and had a higher level of TC and serum GPNMB (Table 3). Circulating serum GPNMB levels were higher in subjects with cataract than those without cataract (Figure 3). The ability of serum GPNMB levels to predict the presence of cataract was 0.783, based on ROC curve analysis (asymptotic significance: <0.001) (Figure 4). The optimal cut-off value of GPNMB was 16,630.675 pg/mL (73.6% sensitivity and 73.0% specificity) to detect DM.
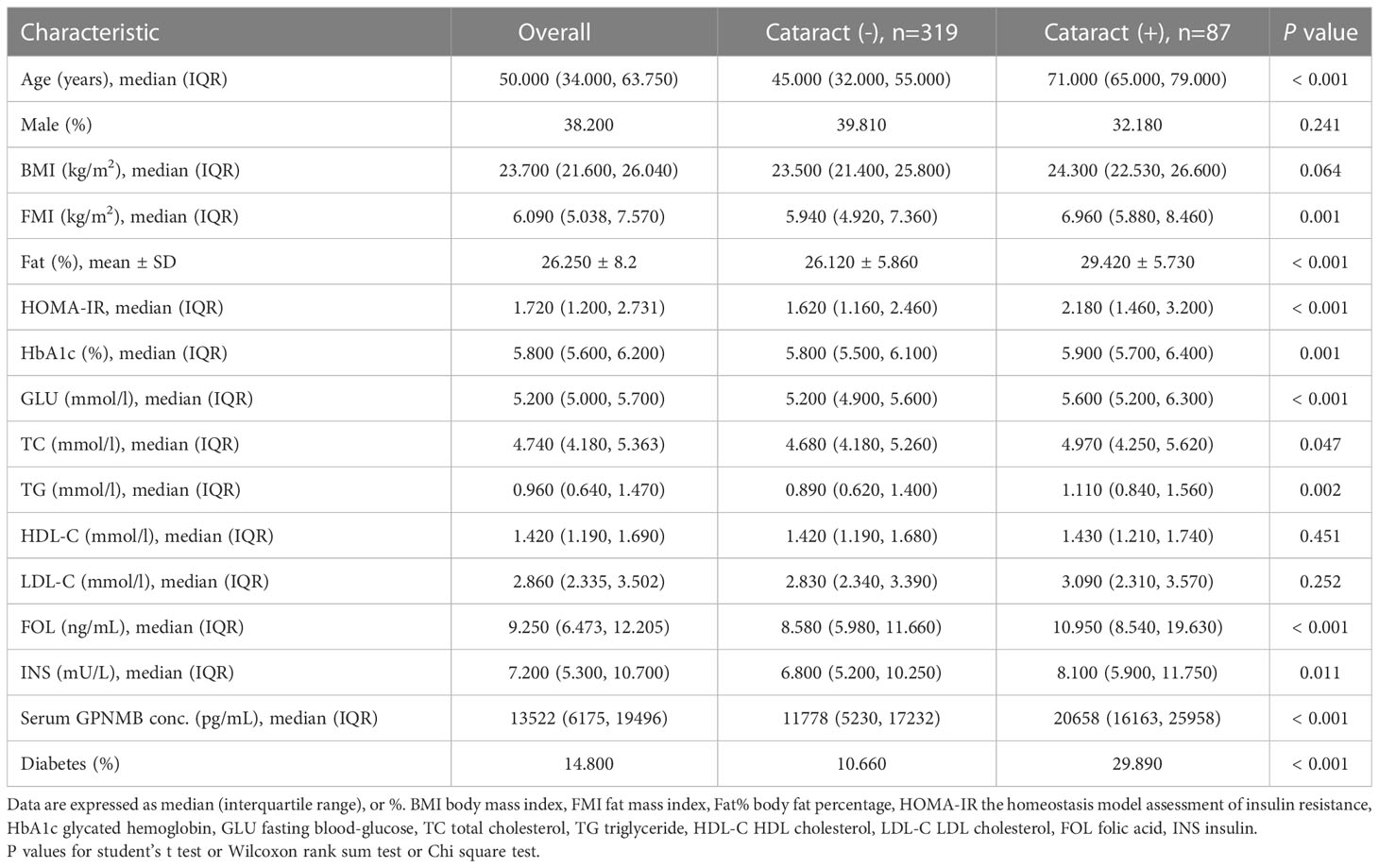
Table 2 Clinical and biochemical characteristics of study participants classified according to cataract.
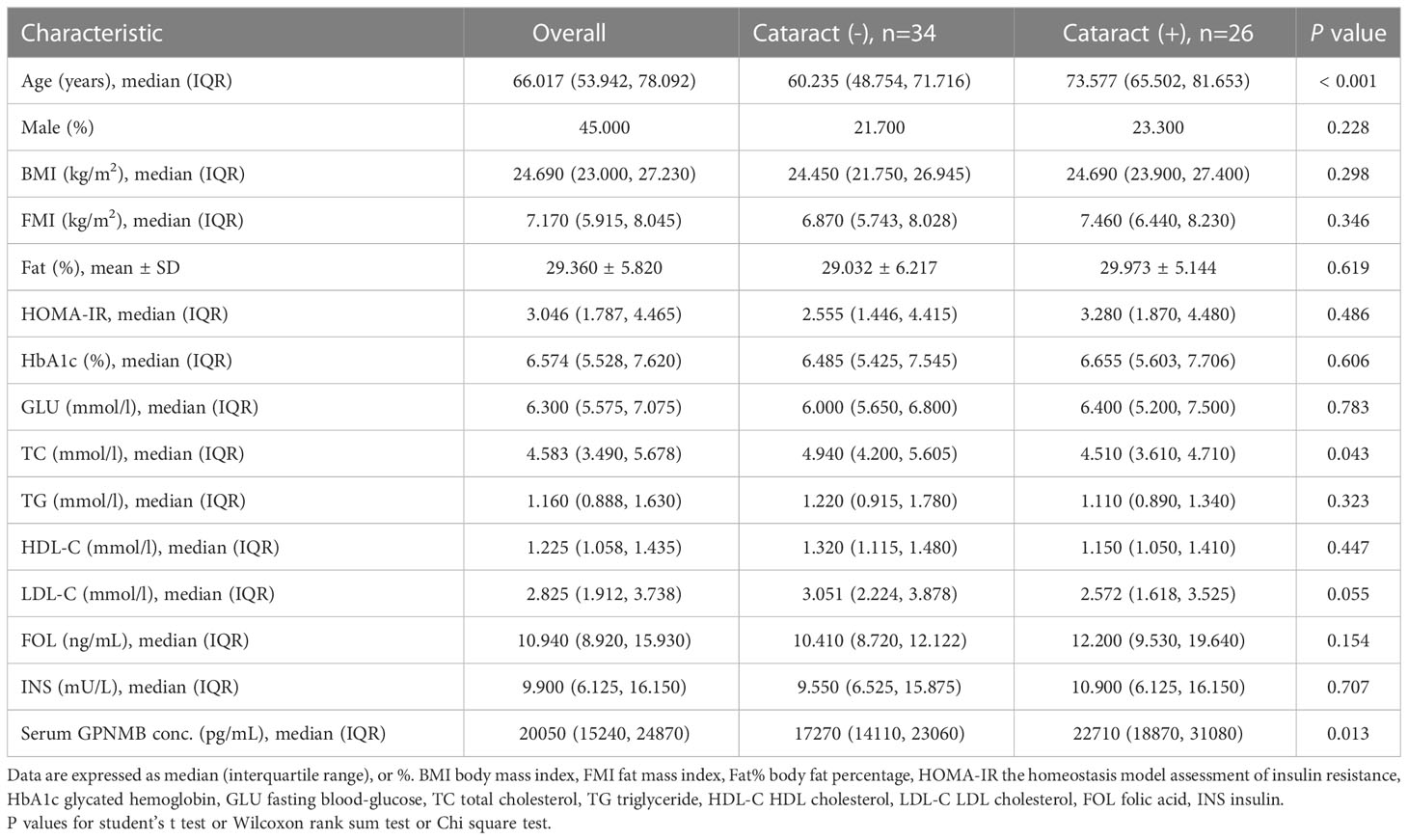
Table 3 Clinical and biochemical characteristics of study participants with diabetes classified according to cataract.
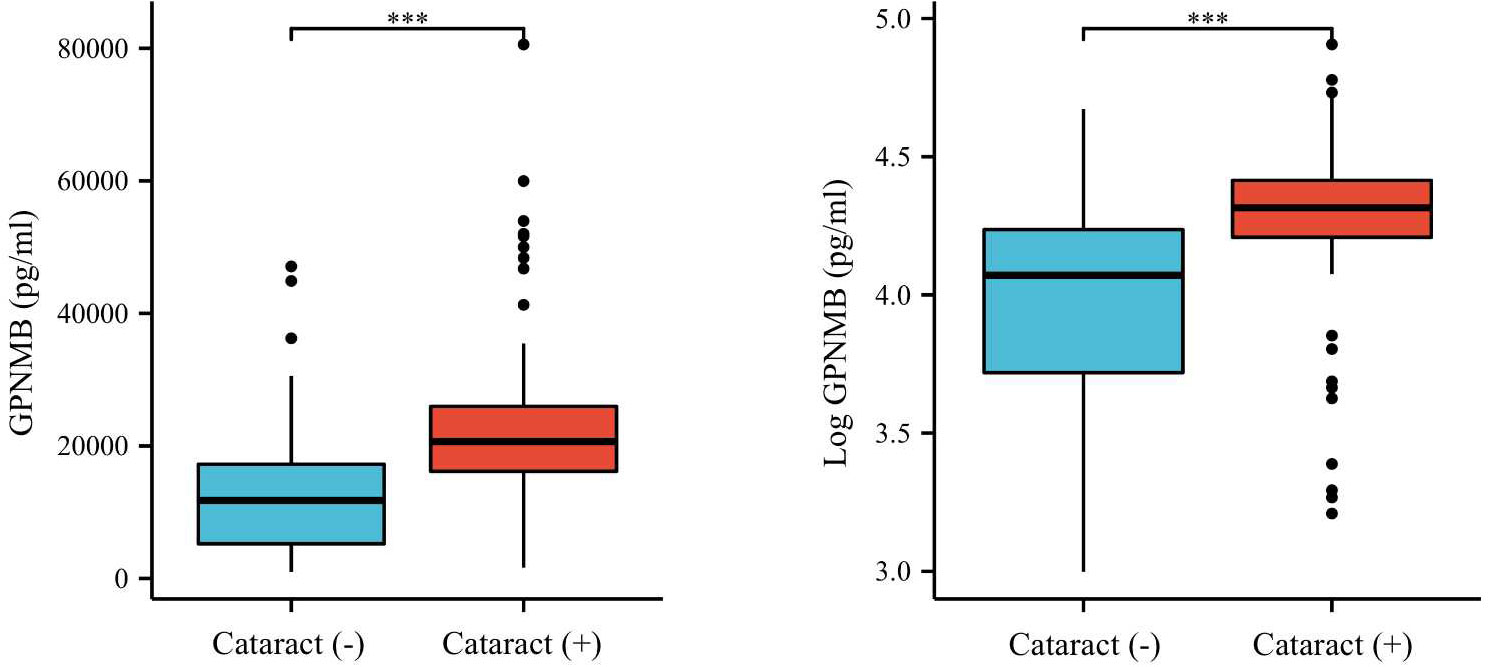
Figure 3 Plasma GPNMB levels depending on the existence of cataract. ***P < 0.001 using a Wilcoxon rank sum test.
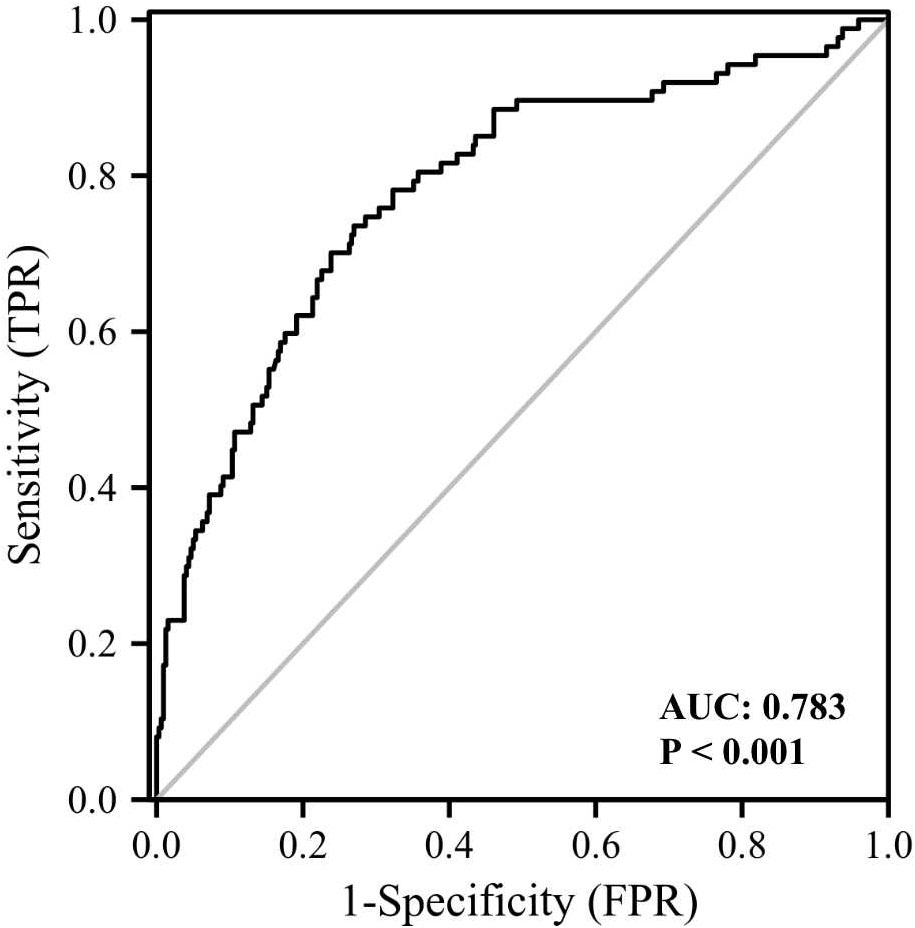
Figure 4 ROC curve analysis of the ability of plasma GPNMB to predict the presence of cataract. AUC, area under curve.
Table 4 shows the general characteristics of enrolled individuals grouped according to GPNMB tertiles. Lower age, Fat%, TC, TG, LDL-C, FOL levels, and more men were detected in tertile 1 than in tertiles 2 and 3. Nevertheless, the median levels of TC and LDL-C of individuals in tertile 2 were higher than those in tertile 3. Notably, a higher proportion of participants in tertile 3 were diagnosed as having DM and cataract than in tertiles 2 and 1.
As shown in Table 5, univariable logistic regression analysis revealed that age, BMI, FMI, Fat%, HOMA-IR, HbA1c, GLU, INS, TC, HDL-C, FOL, and log GPNMB were associated with DM. We included these variables in the multivariable logistic regression analysis. To avoid collinearity, we explored the linear correlation of log GPNMB with age, Fat%, GLU, TC, and FOL through Pearson’s correlation coefficient analysis (Supplementary Figure 1) and finally excluded those five variables from the multivariable logistic regression analysis. It was indicated that HOMA-IR, HDL-C, and log GPNMB were independently associated with the presence of DM.
We also performed univariable and multivariable logistic regression analysis to investigate potential biomarkers for cataract (Table 6). Age, FMI, Fat%, HbA1c, GLU, TC, FOL, and log GPNMB were found to be associated with cataract by univariable logistic regression analysis. Multivariable logistic regression analysis showed that log GPNMB was an independent predictor of cataract. To gain further understanding of the relationship between serum GPNMB and DM-related cataract, we utilized ROC curve analysis based on serum GPNMB levels and the diabetic status (Figure 5). The area under the ROC curve was 0.789 with an asymptotic significance <0.001.
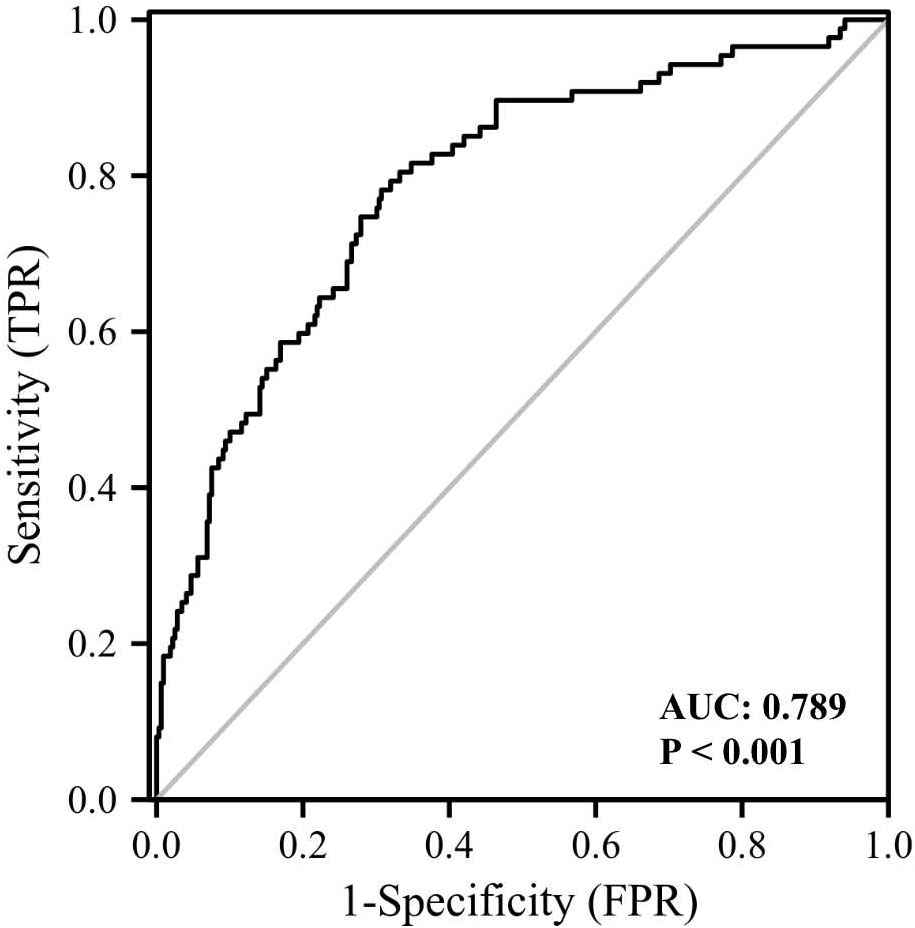
Figure 5 ROC curve analysis of the ability of plasma GPNMB and the presence of diabetes together to predict the presence of cataract. AUC, area under curve.
Discussion
To our best knowledge, our study is the first to demonstrate that serum GPNMB levels correlate with both DM and cataract. The results showed higher GPNMB levels in subjects with DM or cataract than in control subjects. In addition, serum GPNMB levels were independently correlated with both DM and cataract. ROC curve analysis also proved the potential of serum GPNMB levels as an independent biomarker for DM and cataract. Notably, the ability of GPNMB levels to diagnose cataract was improved with the presence of DM. Further research is required to validate these results.
GPNMB possesses an extracellular N-terminal signal peptide, a transmembrane domain, and a short cytoplasmic tail (21) and functions both as an inflammatory mediator and a circulating cytokine. Referring to its role in inflammatory response, a previous study detected the prevailing expression of GPNMB in macrophages, and showed that the expression level of GPNMB can be induced by interferon gamma (IFN-γ) and lipopolysaccharide and is elevated in inflammatory macrophages (15). In addition, overexpression of GPNMB in RAW264.7 cells, a macrophage cell line derived from mice, causes significant decrease of interleukin (IL)-6 and IL-12p40, inflammatory cytokines, as well as the inflammatory regulator nitric oxide (NO) (15). Thus, GPNMB is considered as a negative regulator of macrophage inflammatory capacity. Furthermore, macrophage inflammatory response is closely related to metabolic disorders (29). and DM is one of the most common metabolic diseases and can cause aberrant metabolism of blood glucose and induce the inflammatory response. By utilizing mouse models fed with high-fat diet, Prabata et al. (30) detected attenuated insulin and glucose tolerance in GPNMB-knock-out (KO) mice, and observed high levels of inflammatory cytokines produced by macrophages derived from GPNMB KO mice. In addition, the increase in inflammatory cytokines secreted by macrophages could be abrogated by added GPNMB extracellular domain. Prabata et al. (30) also found that GPNMB could bind to CD44 to prohibit nuclear factor kappa-B (NF-κB), thus abate the inflammatory response of macrophages.
GPNMB can also be cleaved into a soluble form that contains an ectodomain (ECD) and functions as a secreted cytokine (31). Gong et al. (20) revealed that GPNMB-ECD could interact with CD44 to trigger AKT signaling and further contributed to lipogenesis in adipocytes of withe adipose tissues. They further showed that GPNMB was capable of inducing obesity and insulin resistance in mice and this phenotype could be rescued by an anti-GPNMB antibody. Gong et al. (20) also performed a population-based study and ascertained that serum levels of GPNMB were correlated with human obesity. Research also determined the positive association between serum levels of GPNMB and disease presence and severity of Parkinson’s Disease (32). Another study that concentrated on diabetic retinopathy—a microvascular complication characterized by aberrant angiogenesis—found that GPNMB knockdown attenuated retinal angiogenesis stimulated by high glucose both in vivo and in vitro (33).
In this study, elevated serum levels of GPNMB were determined in subjects with DM and cataract. Based on the results of previous research, it was quite possible that the increased circulating levels of GPNMB caused abnormal phosphorylation of AKT via binding to CD44 and then stimulated anomalous activation of AKT/PI3K signaling to promote lipogenesis and provoke diabetes. Moreover, activation of AKT signaling followed by downregulation of connexin 43 is required for transforming growth factor-beta 2 (TGF-β2)-induced epithelial mesenchymal transition (EMT) of HLE B-3 cells—a human lens epithelial cell line (34). It has been reported that lens epithelial cells that undergo EMT can result in posterior capsule opacification, which is the main cause and symptom of cataract (35). Yao et al. (36) demonstrated that integrin beta-1, a target protein of GPNMB, was essential for TGF-β2-mediated migration of lens epithelial cells. Thus, the raised serum levels of GPNMB may abate the expression of connexin 43 and stimulate the upregulation of integrin beta-1 to promote cataract formation. Notably, high glucose levels due to diabetes-induced insulin resistance also contributes to the pathogenesis of cataract (37). Accordingly, we concluded that the way in which GPNMB promoted DM might enhance the development of cataract. Further investigations are needed to reveal the underlying molecular mechanisms.
To minimize probable bias, we excluded subjects with alcohol and drug abuse, severe diseases, and those that underwent recent medical treatment. We aimed to only enroll subjects with a relatively steady metabolism, because DM and cataract are both metabolic diseases. Moreover, recruited individuals were all required to undergo 8h fasting before screening to maintain stable levels of serum cytokines. We reviewed the medical history of included individuals and performed ocular examination to avoid excluding those who had undergone cataract surgery before or were unaware of their cataract. Furthermore, we utilized different statistical methods to analyze the data, and found that the results were consistent. These strategies added to the strengths of our study. A limitation of the study is the relatively small number of individuals in the subgroups. Further mechanistic studies are required to investigate the mechanism underlying GPNMB’s effect on the pathogenesis of DM-related cataract.
Data availability statement
The raw data supporting the conclusions of this article will be made available by the authors, without undue reservation.
Ethics statement
The studies involving human participants were reviewed and approved by the Research Ethics Committee of Beijing Hospital (2019BJYYEC-054-02). The patients/participants provided their written informed consent to participate in this study.
Author contributions
Conceptualization: JC, J-PC, T-MZ. Methodology: JC, DH, Y-YL. Samples collection: DH, Y-YL, C-BL, L-TZ, G-QF, YW, L-QZ, JP, C-BL. Software Formal analysis: DH, Y-YL, CZ, X-FL. Data curation: JC, DH, Y-YL. Writing—original draft preparation: DH, Y-YL. Writing—review and editing: JC, J-PC, T-MZ, JP, L-QZ, TS. Supervision: JC, J-PC. All authors contributed to the article and approved the submitted version.
Funding
This work was supported by the National Key Research and Development Program of China (2018YFC2000304, 2020YFC2002700); the National Natural Science Foundation of China (82073264, 81770228); the CAMS Innovation Fund for Medical Sciences (No. 2021-I2M-1–050); Dongcheng District Outstanding Talent Nurturing Program (2022-dchrcpyzz-66); Shenzhen Institute of Synthetic Biology Scientific Research Program (DWKF20210009).
Conflict of interest
The authors declare that the research was conducted in the absence of any commercial or financial relationships that could be construed as a potential conflict of interest.
Publisher’s note
All claims expressed in this article are solely those of the authors and do not necessarily represent those of their affiliated organizations, or those of the publisher, the editors and the reviewers. Any product that may be evaluated in this article, or claim that may be made by its manufacturer, is not guaranteed or endorsed by the publisher.
Supplementary material
The Supplementary Material for this article can be found online at: https://www.frontiersin.org/articles/10.3389/fendo.2023.1110337/full#supplementary-material
Supplementary Figure 1 | The linear correlation of log GPNMB with different variables through Pearson’s correlation coefficient analysis. BMI body mass index, FMI fat mass index, Fat% body fat percentage, HOMA-IR the homeostasis model assessment of insulin resistance, HbA1c glycated hemoglobin, GLU fasting blood-glucose, TC total cholesterol, HDL-C HDL cholesterol, FOL folic acid, INS insulin.
References
1. Heald AH, Stedman M, Davies M, Livingston M, Alshames R, Lunt M, et al. Estimating life years lost to diabetes: Outcomes from analysis of national diabetes audit and office of national statistics data. Cardiovasc Endocrinol Metab (2020) 9(4):183–5. doi: 10.1097/XCE.0000000000000210
2. Saeedi P, Salpea P, Karuranga S, Petersohn I, Malanda B, Gregg EW, et al. Mortality attributable to diabetes in 20-79 years old adults, 2019 estimates: Results from the international diabetes federation diabetes atlas, 9(th) edition. Diabetes Res Clin Pract (2020) 162:108086. doi: 10.1016/j.diabres.2020.108086
3. Ogurtsova K, Guariguata L, Barengo NC, Ruiz PL, Sacre JW, Karuranga S, et al. IDF diabetes atlas: Global estimates of undiagnosed diabetes in adults for 2021. Diabetes Res Clin Pract (2022) 183:109118. doi: 10.1016/j.diabres.2021.109118
4. Advani A. Acute kidney injury: A bona fide complication of diabetes. Diabetes (2020) 69(11):2229–37. doi: 10.2337/db20-0604
5. Alvarez CA, Lingvay I, Vuylsteke V, Koffarnus RL, McGuire DK. Cardiovascular risk in diabetes mellitus: Complication of the disease or of antihyperglycemic medications. Clin Pharmacol Ther (2015) 98(2):145–61. doi: 10.1002/cpt.143
6. Bell DS. Heart failure: The frequent, forgotten, and often fatal complication of diabetes. Diabetes Care (2003) 26(8):2433–41. doi: 10.2337/diacare.26.8.2433
7. Trujillo-Santos AJ. Diabetic muscle infarction: an underdiagnosed complication of long-standing diabetes. Diabetes Care (2003) 26(1):211–5. doi: 10.2337/diacare.26.1.211
8. Luo A, Xie Z, Wang Y, Wang X, Li S, Yan J, et al. Type 2 diabetes mellitus-associated cognitive dysfunction: Advances in potential mechanisms and therapies. Neurosci Biobehav Rev (2022) 137:104642. doi: 10.1016/j.neubiorev.2022.104642
9. Drinkwater JJ, Davis WA, Davis TME. A systematic review of risk factors for cataract in type 2 diabetes. Diabetes Metab Res Rev (2019) 35(1):e3073. doi: 10.1002/dmrr.3073
10. Pascolini D, Mariotti SP. Global estimates of visual impairment: 2010. Br J Ophthalmol (2012) 96(5):614–8. doi: 10.1136/bjophthalmol-2011-300539
11. Foster A, Gilbert C, Johnson G. Changing patterns in global blindness: 1988-2008. Community Eye Health (2008) 21(67):37–9.
12. Resnikoff S, Pascolini D, Etya'ale D, Kocur I, Pararajasegaram R, Pokharel GP, et al. Global data on visual impairment in the year 2002. Bull World Health Organ (2004) 82(11):844–51.
13. Lansingh VC, Carter MJ, Martens M. Global cost-effectiveness of cataract surgery. Ophthalmology (2007) 114(9):1670–8. doi: 10.1016/j.ophtha.2006.12.013
14. Marks SC Jr, Popoff SN. Osteoclast biology in the osteopetrotic (op) rat. Am J Anat (1989) 186(4):325–34. doi: 10.1002/aja.1001860402
15. Ripoll VM, Irvine KM, Ravasi T, Sweet MJ, Hume DA. Gpnmb is induced in macrophages by IFN-gamma and lipopolysaccharide and acts as a feedback regulator of proinflammatory responses. J Immunol (2007) 178(10):6557–66. doi: 10.4049/jimmunol.178.10.6557
16. Ahn JH, Lee Y, Jeon C, Lee SJ, Lee BH, Choi KD, et al. Identification of the genes differentially expressed in human dendritic cell subsets by cDNA subtraction and microarray analysis. Blood (2002) 100(5):1742–54. doi: 10.1182/blood.V100.5.1742.h81702001742_1742_1754
17. Abdelmagid SM, Barbe MF, Rico MC, Salihoglu S, Arango-Hisijara I, Selim AH, et al. Osteoactivin, an anabolic factor that regulates osteoblast differentiation and function. Exp Cell Res (2008) 314(13):2334–51. doi: 10.1016/j.yexcr.2008.02.006
18. Hoashi T, Sato S, Yamaguchi Y, Passeron T, Tamaki K, Hearing VJ. Glycoprotein nonmetastatic melanoma protein b, a melanocytic cell marker, is a melanosome-specific and proteolytically released protein. FASEB J (2010) 24(5):1616–29. doi: 10.1096/fj.09-151019
19. Huang JJ, Ma WJ, Yokoyama S. Expression and immunolocalization of gpnmb, a glioma-associated glycoprotein, in normal and inflamed central nervous systems of adult rats. Brain Behav (2012) 2(2):85–96. doi: 10.1002/brb3.39
20. Gong XM, Li YF, Luo J, Wang JQ, Wei J, Wang JQ, et al. Gpnmb secreted from liver promotes lipogenesis in white adipose tissue and aggravates obesity and insulin resistance. Nat Metab (2019) 1(5):570–83. doi: 10.1038/s42255-019-0065-4
21. Selim AA. Osteoactivin bioinformatic analysis: prediction of novel functions, structural features, and modes of action. Med Sci Monit (2009) 15(2):Mt19–33.
22. Shikano S, Bonkobara M, Zukas PK, Ariizumi K. Molecular cloning of a dendritic cell-associated transmembrane protein, DC-HIL, that promotes RGD-dependent adhesion of endothelial cells through recognition of heparan sulfate proteoglycans. J Biol Chem (2001) 276(11):8125–34. doi: 10.1074/jbc.M008539200
23. Tomihari M, Hwang SH, Chung JS, Cruz PD Jr., Ariizumi K. Gpnmb is a melanosome-associated glycoprotein that contributes to melanocyte/keratinocyte adhesion in a RGD-dependent fashion. Exp Dermatol (2009) 18(7):586–95. doi: 10.1111/j.1600-0625.2008.00830.x
24. Chung JS, Sato K, Dougherty II, Cruz PD Jr, Ariizumi K. DC-HIL is a negative regulator of T lymphocyte activation. Blood (2007) 109(10):4320–7. doi: 10.1182/blood-2006-11-053769
25. Cao C, Wei L, Han Y, Wang Y, Zhang J, Zhang L, et al. Elevated circulating levels of glycoprotein non-metastatic melanoma protein b as a predictor of gestational diabetes mellitus. Clin Lab (2021) 67(9):1989–94. doi: 10.7754/Clin.Lab.2020.201047
26. Monteiro MB, Pelaes TS, Santos-Bezerra DP, Thieme K, Lerario AM, Oba-Shinjo SM, et al. Urinary sediment transcriptomic and longitudinal data to investigate renal function decline in type 1 diabetes. Front Endocrinol (Lausanne). (2020) 11:238. doi: 10.3389/fendo.2020.00238
27. Matthews DR, Hosker JP, Rudenski AS, Naylor BA, Treacher DF, Turner RC. Homeostasis model assessment: Insulin resistance and beta-cell function from fasting plasma glucose and insulin concentrations in man. Diabetologia (1985) 28(7):412–9. doi: 10.1007/BF00280883
28. Wallace TM, Levy JC, Matthews DR. Use and abuse of HOMA modeling. Diabetes Care (2004) 27(6):1487–95. doi: 10.2337/diacare.27.6.1487
29. Saltiel AR, Olefsky JM. Inflammatory mechanisms linking obesity and metabolic disease. J Clin Invest. (2017) 127(1):1–4. doi: 10.1172/JCI92035
30. Prabata A, Ikeda K, Rahardini EP, Hirata KI, Emoto N. GPNMB plays a protective role against obesity-related metabolic disorders by reducing macrophage inflammatory capacity. J Biol Chem (2021) 297(5):101232. doi: 10.1016/j.jbc.2021.101232
31. Rose AA, Annis MG, Dong Z, Pepin F, Hallett M, Park M, et al. ADAM10 releases a soluble form of the GPNMB/Osteoactivin extracellular domain with angiogenic properties. PloS One (2010) 5(8):e12093. doi: 10.1371/journal.pone.0012093
32. Diaz-Ortiz ME, Seo Y, Posavi M, Carceles Cordon M, Clark E, Jain N, et al. GPNMB confers risk for parkinson's disease through interaction with α-synuclein. Science (2022) 377(6608):eabk0637. doi: 10.1126/science.abk0637
33. Qin T, Xi X, Wu Z. Downregulation of glycoprotein non-metastatic melanoma protein b prevents high glucose-induced angiogenesis in diabetic retinopathy. Mol Cell Biochem (2022). doi: 10.1007/s11010-022-04537-7
34. Yao K, Ye PP, Tan J, Tang XJ, Shen Tu XC. Involvement of PI3K/Akt pathway in TGF-beta2-mediated epithelial mesenchymal transition in human lens epithelial cells. Ophthalmic Res (2008) 40(2):69–76. doi: 10.1159/000113884
35. Marcantonio JM, Syam PP, Liu CS, Duncan G. Epithelial transdifferentiation and cataract in the human lens. Exp Eye Res (2003) 77(3):339–46. doi: 10.1016/S0014-4835(03)00125-8
36. Hu H, Li Z, Lu M, Yun X, Li W, Liu C, et al. Osteoactivin inhibits dexamethasone-induced osteoporosis through up-regulating integrin β1 and activate ERK pathway. BioMed Pharmacother (2018) 105:66–72. doi: 10.1016/j.biopha.2018.05.051
37. Jain AK, Lim G, Langford M, Jain SK. Effect of high-glucose levels on protein oxidation in cultured lens cells, and in crystalline and albumin solution and its inhibition by vitamin B6 and n-acetylcysteine: Its possible relevance to cataract formation in diabetes. Free Radic Biol Med (2002) 33(12):1615–21. doi: 10.1016/S0891-5849(02)01109-7
Keywords: diabetes mellitus, cataract, GPNMB, biomarker, serum
Citation: Huo D, Liu Y-Y, Zhang C, Zeng L-T, Fan G-Q, Zhang L-Q, Pang J, Wang Y, Shen T, Li X-F, Li C-B, Zhang T-M, Cai J-P and Cui J (2023) Serum glycoprotein non-metastatic melanoma protein B (GPNMB) level as a potential biomarker for diabetes mellitus-related cataract: A cross-sectional study. Front. Endocrinol. 14:1110337. doi: 10.3389/fendo.2023.1110337
Received: 28 November 2022; Accepted: 06 February 2023;
Published: 16 February 2023.
Edited by:
Maurizio Delvecchio, Giovanni XXIII Children’s Hospital, ItalyReviewed by:
Liangcai Zhao, Wenzhou Medical University, ChinaBaohua Liu, Shenzhen University, China
Qin Zhang, Zhejiang University, China
Copyright © 2023 Huo, Liu, Zhang, Zeng, Fan, Zhang, Pang, Wang, Shen, Li, Li, Zhang, Cai and Cui. This is an open-access article distributed under the terms of the Creative Commons Attribution License (CC BY). The use, distribution or reproduction in other forums is permitted, provided the original author(s) and the copyright owner(s) are credited and that the original publication in this journal is cited, in accordance with accepted academic practice. No use, distribution or reproduction is permitted which does not comply with these terms.
*Correspondence: Ju Cui, anV6aS5jdWlAZ21haWwuY29t; Y3VpanU0MzY2QGJqaG1vaC5jbg==; Jian-Ping Cai, Y2FpanA2MUB2aXAuc2luYS5jb20=
†These authors have contributed equally to this work