- 1Department of Neurology, China-Japan Union Hospital of Jilin University, Changchun, China
- 2Department of Endocrinology, Jilin Province People’s Hospital, Changchun, China
Background: Many clinical studies have shown a correlation between plasma cortisol and neurological disorders. This study explored the causal relationship between plasma cortisol and dementia, epilepsy and multiple sclerosis based on Mendelian randomization (MR) method.
Methods: Data were taken from the summary statistics of a genome-wide association study, FinnGen consortium and United Kingdom Biobank. Dementia, epilepsy, and multiple sclerosis were used as outcomes, and genetic variants associated with plasma cortisol were used as instrumental variables. The main analysis was performed using the inverse variance weighted method, and the results were assessed according to the odds ratio (OR) and 95% confidence interval. Heterogeneity tests, pleiotropy tests, and leave-one-out method were conducted to evaluate the stability and accuracy of the results.
Results: In two-sample MR analysis, the inverse variance weighted method showed that plasma cortisol was associated with Alzheimer’s disease (AD) [odds ratio (95% confidence interval) = 0.99 (0.98-1.00), P = 0.025], vascular dementia (VaD) [odds ratio (95% confidence interval) = 2.02 (1.00-4.05), P = 0.049)], Parkinson’s disease with dementia (PDD) [odds ratio (95% confidence interval) = 0.24 (0.07-0.82), P = 0.023] and epilepsy [odds ratio (95% confidence interval) = 2.00 (1.03-3.91), P = 0.042]. There were no statistically significant associations between plasma cortisol and dementia with Lewy bodies (DLB), frontotemporal dementia (FTD) and multiple sclerosis.
Conclusion: This study demonstrates that plasma cortisol increase the incidence rates of epilepsy and VaD and decrease the incidence rates of AD and PDD. Monitoring plasma cortisol concentrations in clinical practice can help prevent diseases, such as AD, PDD, VaD and epilepsy.
1 Introduction
Dementia, epilepsy and multiple sclerosis (MS)are common neurological disorders for which there are reports of associations with endocrine or immune markers. Hormones are one of the major physiological regulators of brain development, and endocrine and immune system dysfunction may contribute to the development of dementia, epilepsy and MS (1–3).
Approximately 55 million individuals worldwide suffer from dementia, and that number is expected to rise to 78 million by 2030 and 152 million by 2050 (4, 5). In 2019, the number of people with dementia disability globally exceeded 25.27 million (6). Dementia ranks 7th among the top 10 causes of death worldwide, with over 1.62 million deaths from dementia (7). Dementia can be divided into degenerative and nondegenerative categories. The former involves Alzheimer’s disease (AD), dementia with Lewy bodies (DLB), Parkinson’s disease with dementia (PDD) and frontotemporal dementia (FTD), while the latter includes vascular dementia (VaD). AD accounts for 50%–70% of all dementia types (8), DLB is second only to AD in prevalence and accounts for 10%–15% of dementia (9), PDD accounts for approximately 3%-4% of dementia (10), FTD accounts for 5%–10% of dementia (11), and VaD is the most widespread type of dementia caused by a nondegenerative disease, accounting for 15%–20% of patients with dementia (12). Dementia and cognitive impairment are major global issues as the world’s population ages. Many clinical studies have shown that plasma cortisol has a direct relationship with cognitive impairment, and some observational studies have indicated a causal effect between plasma cortisol and dementia (13–15).
Epilepsy is a common neurological disorder worldwide and affects approximately 70 million individuals globally (16). Every year, there are 34 to 76 new cases diagnosed per 100,000 people (17). Epilepsy has high rates of disability and death, and the social and psychological burdens of the disease are severe, which seriously affects the quality of life of individuals with epilepsy (18). In recent years, many scholars have discovered that epilepsy has biological rhythms that are similar to the circadian rhythms of plasma cortisol (19). The circadian rhythms of plasma cortisol concentrations may affect the balance of neuronal excitability and inhibition, and a correlation exists between plasma cortisol and epilepsy susceptibility (20, 21).
Multiple sclerosis is an immune-mediated demyelinating disease of the central nervous system. It primarily affects individuals between the ages of 20 and 40 and is a major cause of disability in young adults, with serious social and economic burdens (22, 23). The Global Burden of Disease Study reports that the age-standardized MS prevalence is highest in high-income regions of North America, at 164.6 per 100,000 people, and is lowest in Asia (24, 25). Some studies have shown that hypothalamus-pituitary-adrenal (HPA) axis dysfunction is associated with the triggering of or increases in MS symptoms (26, 27).
Traditional observational research designs are case−control studies, whose findings are frequently influenced by confounding factors and where causal effects cannot be determined. In addition, randomized clinical trials can be limited by required ethical considerations. Therefore, valid strategies must be developed to identify causal relationships between exposure factors and response outcomes. Mendelian randomization (MR) is based on Mendel’s law of segregation, which states that allele pairs separate or segregate during gamete formation and randomly unite at fertilization (28). The single nucleotide polymorphisms (SNPs) of genes are determined at birth and are not altered by interference from external environmental and behavioral factors; thus, SNPs are considered instrumental variables (IVs), which can be used to enhance the exposure-outcome relationship, prevent reverse causality in the exposure-outcome association and avoid reverse causality. We implemented a two-sample MR analysis using summary statistics and attempted to explore the causal effect of plasma cortisol on dementia, epilepsy, and MS.
2 Methods
2.1 Study design and MR assumptions
MR is based on the following three core assumptions: (i) the association hypothesis: there is a strong association hypothesis between genetic variants and exposure; (ii) the independence hypothesis: genetic variants must be independent of confounding factors; and (iii) the exclusivity hypothesis: genetic variants can only impact the outcome through exposure and not through other pathways (29). The first hypothesis can be directly assessed by examining the strength of the association of genetic variants with risk factors; the second and third hypotheses can also be interpreted as not being influenced by pleiotropy and require sensitivity analyses for exclusion. Genetic pleiotropy means that genes affect more than one phenotypic trait (30). A directed acyclic graph of Mendelian randomization is depicted in Figure 1.
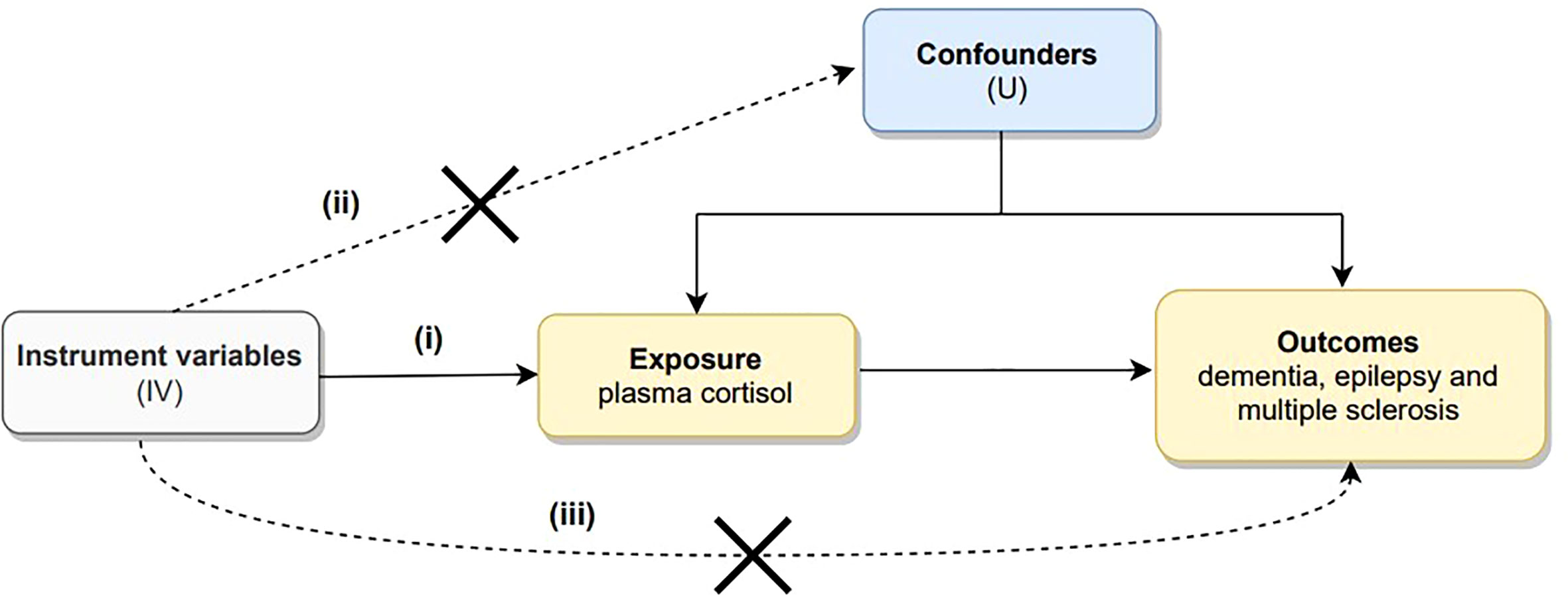
Figure 1 Directed acyclic graph illustrating the core assumptions of Mendelian randomization. There are three core assumptions: (i) there is a strong association hypothesis between genetic variants and exposure; (ii) genetic variants must be independent of confounding factors; and (iii) genetic variants can only impact outcome through exposure but not through other pathways.
2.2 Instrumental variables
The exposure data were obtained from the CORtisol NETwork (CORNET), and included 12,597 participants (mean age 53.5 years, 59.2% female) from 11 Western European population-based cohorts (31). Participants’ blood was collected between 7:00 and 11:00 am, and plasma cortisol concentrations were determined by immunoassay. The z-scores of the plasma cortisol concentrations were log-transformed, and corrected for age, sex, and genetic control (31).
2.3 Outcome data
2.3.1 Dementia
Dementia data were predominantly extracted from the United Kingdom Biobank (UKB) and FinnGen consortium. UKB was a large, detailed, population-based prospective study with over 500,000 participants aged 40–69 years conducted from 2006 to 2010 (32). The research involves medical records from a nationwide longitudinal follow-up and contains information on the phenotypes and genotypes of individuals. The UKB database was principally collected from surveys, sample testing, and genome-wide genotyping. The FinnGen consortium, which involved people over the age of 18 who resided in Finland, was a novel study that integrated genetic information with digital healthcare data (33). This database includes samples from hospitals, disease-based cohorts, and prospective epidemiological cohorts (34).
AD data were derived from the UKB, and a GWAS summary database published by Neale Laboratories in 2017. This database covered 26,757 patients with AD and 283,086 controls, all of whom were of European ancestry (35). In those data, AD was characterized as an anamnestic syndrome of the hippocampal type, with decreased memory, executive function, attention and word finding (36). SNPs associated with VaD were selected from the FinnGen consortium, which included 881 patients with VaD and 211,508 controls of European ancestry. PDD data were derived from the FinnGen consortium and included 267 cases of European ancestry and 216,628 normal subjects of European ancestry. For DLB, we used SNPs from the GWAS, including a sample of 2,591 cases and 4,027 healthy individuals (37). These participants were recruited from 44 institutions/consortia and diagnosed according to established consensus criteria, and all of the above participants were of European ancestry. SNPs associated with FTD were obtained from a GWAS dataset of 515 cases and 2509 controls of European ancestry. Because of the low population prevalence of FTD, the study population was obtained from 45 clinical centers in 11 countries (USA, Canada, UK, Netherlands, Belgium, Spain, Germany, Australia, Finland, France, and Sweden) and members of the International FTD Collaborative with the approval of the Institutional Review Board for cases (38). Detailed information is shown in Table 1.
2.3.2 Epilepsy
SNPs associated with epilepsy were gathered from the FinnGen consortium version R6, including a sample of 929 focal epilepsy cases and 212,532 normal controls of European ancestry. Diagnoses of epilepsy in the FinnGen consortium were based on G40 in the 10th edition of the International Statistical Classification of Diseases and Related Health Problems (ICD), and genotype data were obtained from the Finland Biobank and from digital health record data from the Finland Health Registry.
2.3.3 Multiple sclerosis
We used summary-level data from the International Multiple Sclerosis Genetics Consortium (IMSGC), which included 14,802 patients with MS and 26,703 controls with European ancestry (39). Allele frequencies for SNPs in GWAS data of MS were obtained from 1000 genomic samples of European ancestry (40). Age, sex, and diagnosis criteria for the contributing cohorts were all accessible in the original GWAS.
2.4 Mendelian randomization analyses
We conducted a two-sample MR using the R programming language (Version 4.2.1; R Project for Statistical Computing, Vienna, Austria) (41). We obtained two samples of R programming packages from the MR analysis platform MR-Base.
We obtained the SNPs using the clump module in PLINK software (42). Because the number of SNPs with genome-wide significance is limited, we relaxed the association threshold with P < 5×10−8, a genetic distance of 10 000 kb and a linkage disequilibrium (LD) r2 < 0.3 to obtain the independent SNPs, which is consistent with the study of Larsson et al (43). The method was previously widely used in MR studies (44, 45). To remove confounding factors, we queried each SNPs on the website PhenoScanner (http://www.phenoscanner.medschl.cam.ac.uk/) and excluded SNP related to outcomes and confounders. To evaluate the strength of SNPs in this study F-statistics were calculated for individual SNPs, and F>10 indicated the absence of weak SNP bias. We extracted exposure SNPs from the outcome data and excluded those SNPs associated with outcomes (P < 5×10−8). Harmonization was then performed so that effect allele frequencies for exposure and outcomes correspond to the same effect allele.
To determine the causal effect between plasma cortisol and outcomes, we used inverse variance weighted (IVW) and MR−Egger regression, along with the weighted median, and maximum likelihood. Combining the IVW method with the Wald ratios (ratio of genetic association to outcome versus ratio of genetic association to exposure) of the causal effects of each SNP, where the odds ratio (OR) has a 95% confidence interval (CI) (46–48). The OR and 95% CI represented the risk for outcomes per standard deviation increase in circulating concentrations of plasma cortisol. Statistical significance was set at P < 0.05.
In MR analysis, we mainly used the IVW method to calculate the overall causal effects. To ensure the accuracy of the results, we further combined the inverse variance weighted freedom-effects (IVW-FE) method and the inverse variance weighted random-effects (IVW-RE) method to assess the causal effect between plasma cortisol and outcomes. The accuracy and reliability of the findings were evaluated by comparing the consistency of the findings across the different analytical methods.
We conducted a sensitivity analysis to alleviate the confounding impact of genetic pleiotropy on causal correlations. For the heterogeneity test, we used Cochran’s Q statistic to assess heterogeneity. If P > 0.05 for the result of the heterogeneity test, then there was no heterogeneity, and hypotheses (i) and (iii) in Figure 1 were met, while the contrary suggested the existence of heterogeneity. For the pleiotropy test, pleiotropy test was assessed using the MR−Egger intercept method (49) to identify significant outliers in multiple SNPs. A P-value > 0.05 indicated that there was no horizontal pleiotropy. For the Leave-one-out sensitivity test, SNPs were removed one at a time, and the influence of each remaining SNP on the results was determined. If the results changed dramatically after eliminating a SNP, then SNP was considered an outlier and was removed from the analysis (50). Mendelian Randomization Pleiotropy RESidual Sum and Outlier (MR-PRESSO) regression analyses identified potentially pleiotropic outliers and estimated values after eliminating the outlier SNPs (51).
3 Results
3.1 Basic information on IVs
Summary statistics of instrumental SNPs as genetic IVs for plasma cortisol are presented in Supplementary Table 1. We selected three partially correlated SNPs (rs274952, rs12589136, and rs11621961) that are located in the genes SERPINA6 and SERPINA1. These genes encode proteins that cleave the reactive center loop and release plasma cortisol from corticosteroid-binding globulin (45). Corresponding associations with dementia, epilepsy and MS were extracted. All selected IVs were strongly associated with dementia, epilepsy and MS (P < 5×10-8). IVs had an F-statistic > 10, suggesting that there were no weak effect IVs that would not introduce bias in the MR analysis.
3.2 Mendelian randomization
Supplementary Tables 2–8 show the association of genetically predicted dementia, epilepsy, and MS with plasma cortisol using two-sample MR analysis. Supplementary Figures 1–3 show scatter plots, forest plots, and funnel plots for MR analysis of the causal association between plasma cortisol and outcomes. According to the MR-IVW method, the causal effect between plasma cortisol and AD was statistically significant; the OR of AD per standard deviation decrease in plasma cortisol was 0.99 (95% CI 0.98-1.00), and the OR of VaD was 2.02 (95% CI 1.00-4.05) per standard deviation increase in plasma cortisol, with strength of evidence (P = 0.049). The results of MR are presented in Figure 2. Genetically predicted plasma cortisol was causally associated with PDD, and the OR of PDD per standard deviation decrease in plasma cortisol was 0.24 (95% CI 10.07-0.82, P = 0.023). A one standard deviation increase in genetically proxied plasma cortisol levels corresponded to a 2.00 normalized standard deviation-unit increase in epilepsy (95% CI =1.03-3.91, P = 0.042). There were no correlations between plasma cortisol and DLB, FTD and MS. The corresponding ORs and P-values were 0.65 (95% CI 0.39-1.11) P = 0.115 for DLB, 0.38 (95% CI 0.11-1.27) P = 0.115 for FTD and 0.92 (95% CI 0.73-1.16) P = 0.478 for PDD.
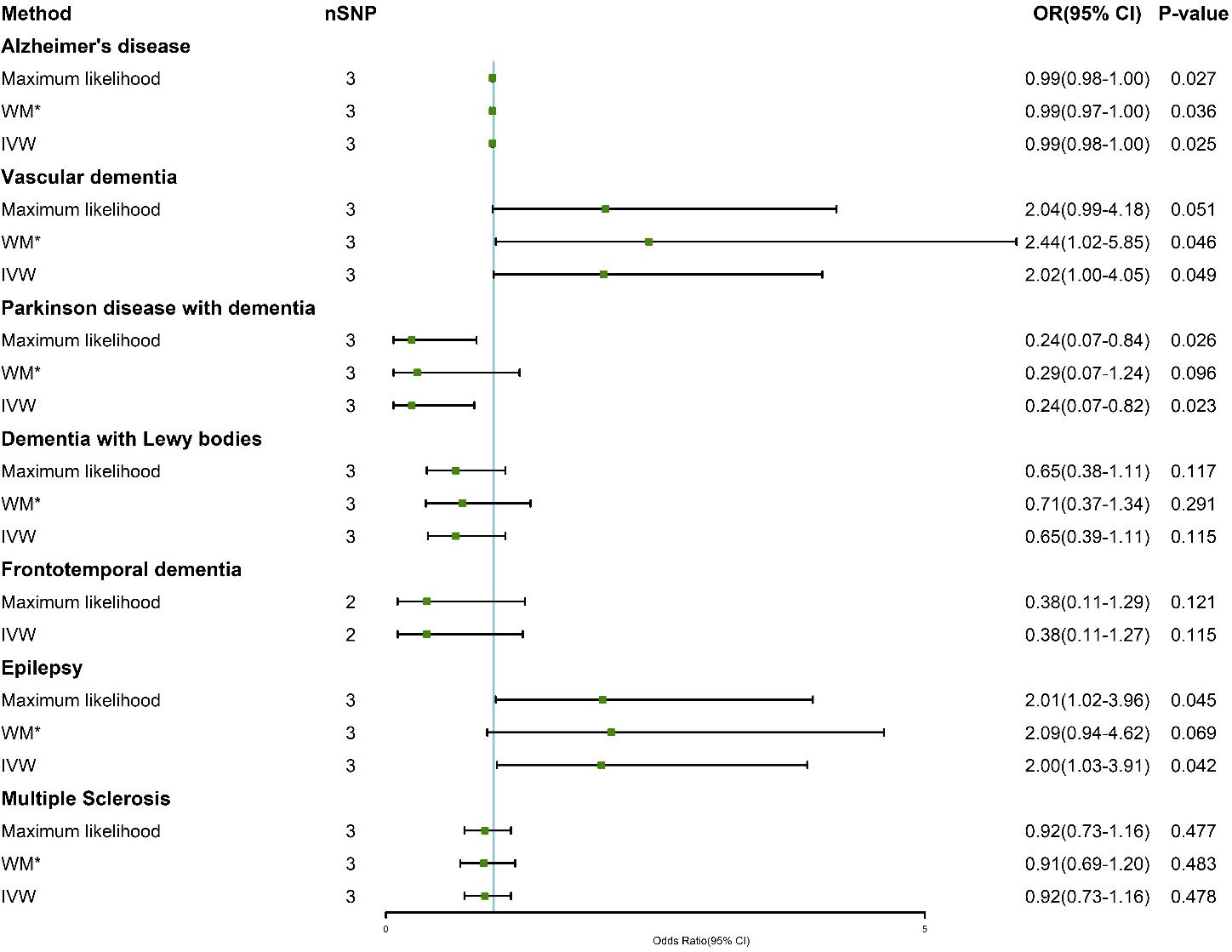
Figure 2 Association of genetically determined plasma cortisol and outcomes using the IVW, weighted median and maximum likelihood methods. IVW, inverse variance weighted; WM*, weighted median; OR, odds ratio; CI, confidence interval; SNP, single nucleotide polymorphism.
The results of the IVW-FE and IVW-RE methods were consistent, all showing that plasma cortisol was an independent risk factor for AD, VaD, PDD, and epilepsy. Figure 3 shows the visualization results for the causal association between exposure and outcomes. We chose a forest plot to depict the results of the study. The corresponding ORs were 0.99 (95% CI 0.98-0.99) for AD, 2.02 (95% CI 1.06-3.84) for VaD and 0.24 (95% CI 0.15-0.37) for PDD using the IVW-RE method, with strength of evidence. A one standard deviation increase in genetically proxied plasma cortisol levels corresponded to a 2.00 normalized standard deviation-unit increase in epilepsy (95% CI =1.54-2.62, P < 0.001). The IVW-FE and IVW-RE methods showed similarities effect for AD, VaD, PDD, and epilepsy.
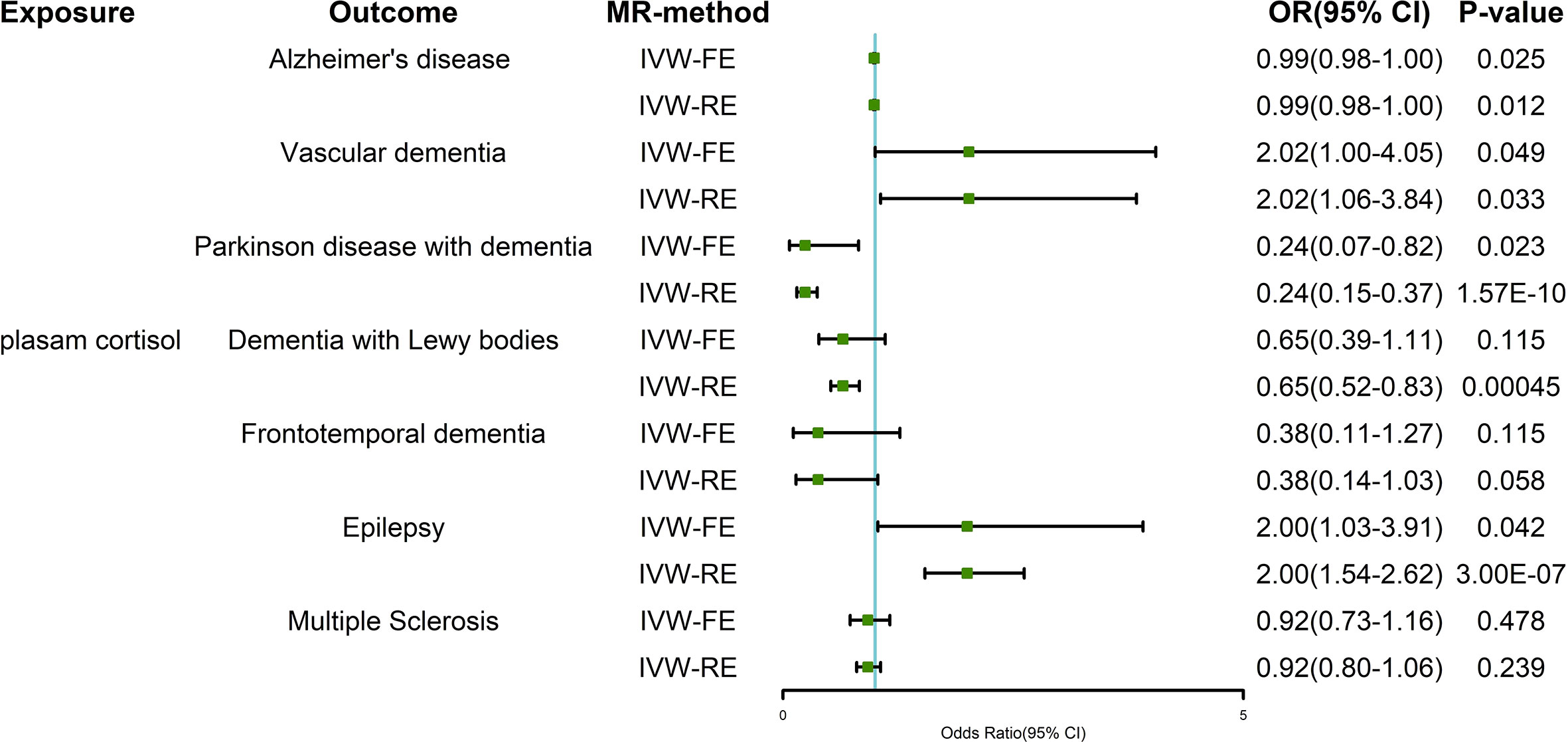
Figure 3 Two-sample Mendelian randomization estimates of the relationship between plasma cortisol and outcomes using IVW-FE and IVW-RE methods. IVW-FE, inverse variance weighted freedom-effects; IVW-RE, inverse variance weighted random-effects; OR, odds ratio; CI, confidence interval.
Genetically predicted plasma cortisol was inversely associated with AD and PDD and positively related to VaD and epilepsy with the IVW method. The study further evaluated the connection between the three IVs and outcomes (Figure 4). The results showed that rs12589136 had a significant role in AD incidence with an OR of 0.98 (95% CI =0.97-1.00, P = 0.039).
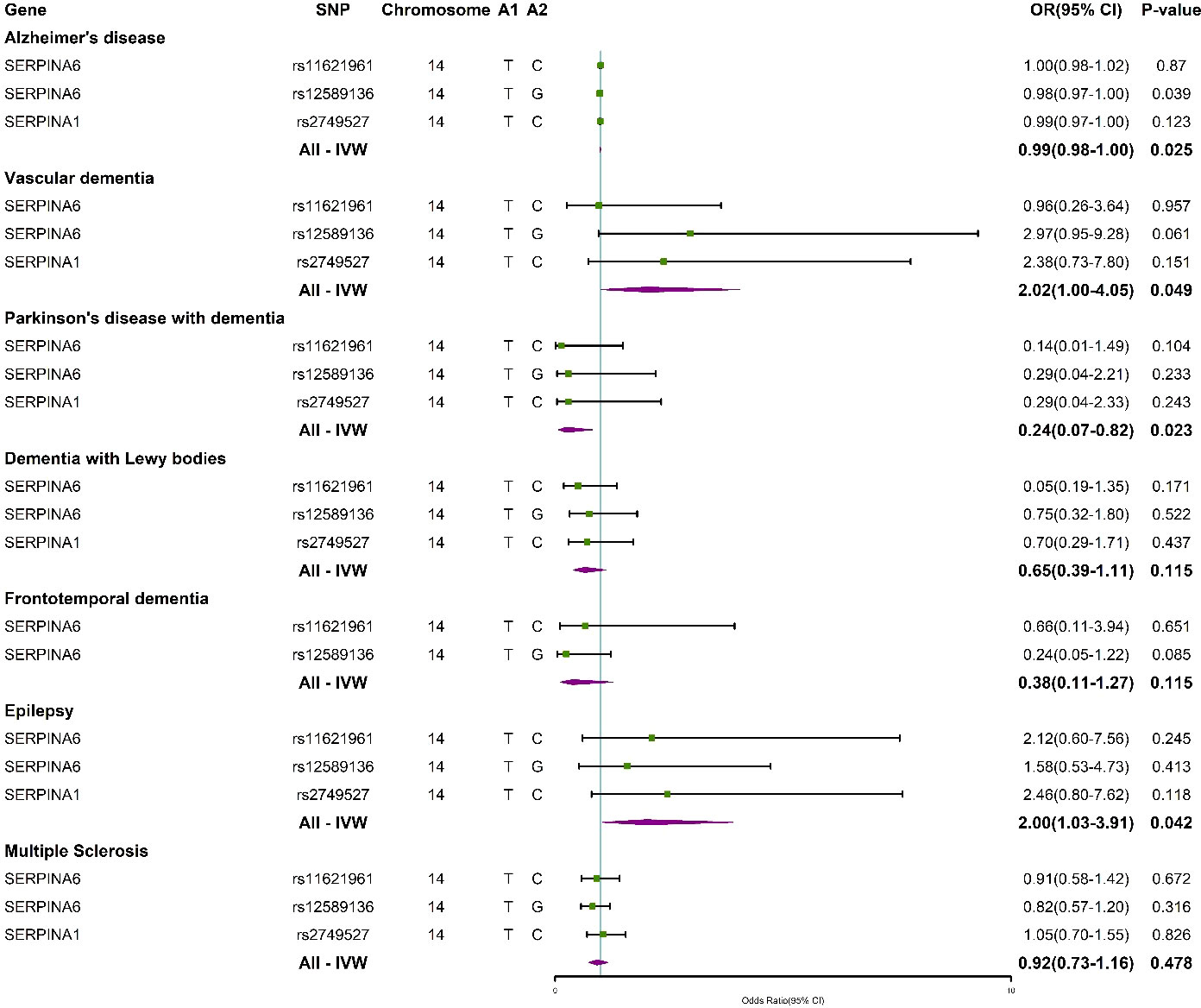
Figure 4 Forest plots summarizing the causal effects of specific plasma cortisol IVs and overall Mendelian randomization estimates associated with outcomes. IVW, inverse variance weighted; SNP, single nucleotide polymorphism; OR, odds ratio; CI, confidence interval.
3.3 Sensitivity analysis
In the MR analysis, we performed a heterogeneity test using both the IVW and MR−Egger methods. The results showed that there was no evidence of heterogeneity (P > 0.05) (Supplementary Table 9). To meet the third core assumption of the MR - the lack of horizontal pleiotropy, the MR−Egger intercept was used to assess the horizontal pleiotropy of IVs, and the results are presented in Supplementary Table 10, with P > 0.05 for the statistical results, suggesting no horizontal pleiotropy effect. In addition, we conducted “leave-one-out” sensitivity analysis. The black dots in each black line and each red line are on the same side of zero and therefore are not influenced by the removal of each IV, showing the robustness and reliability of the results of our MR analysis (Supplementary Figure 4). There were only three IVs in our study, therefore, MR-PRESSO regression analyses could not be performed. Sensitivity analyses using Cochran’s Q statistic, MR−Egger, and leave-one-out showed similar null findings, with no evidence of horizontal pleiotropy from the MR−Egger intercept.
4 Discussion
This two-sample MR study explored the causal relationship between plasma cortisol and dementia, epilepsy, and MS. The results of MR analysis demonstrated that genetic predisposition to higher plasma cortisol is associated with a decreased risk of AD and PDD. There was a positive correlation between plasma cortisol and VaD and epilepsy. Our findings do not suggest that plasma cortisol plays a major role in FTD, DLB, and MS. Neuroactive steroids have the ability to positively or negatively regulate the function of the nervous system (52). Plasma cortisol, one of the stress hormones affected by HPA, is involved in coordinating various processes throughout the body and brain, and is a stress response factor involved in the development of a variety of neuropsychiatric disorders. Many clinical studies have shown that cortisol is associated with anxiety and depression, cognitive impairment, and cerebrovascular disease (53, 54).
4.1 Plasma cortisol and dementia
Our results showed that higher genetically predicted plasma cortisol decreased the risk of AD and PDD, which is inconsistent with previous observational studies (55–59). Observational studies indicated that plasma cortisol levels were significantly higher in AD and PDD patients (58, 60). The outcomes of this investigation are different from previous findings. There are two possibilities for our results. First, there are studies demonstrating that genetically predicted plasma cortisol is associated with a reduced risk of obesity (61), and that plasma cortisol regulates energy metabolism by mobilizing glucose, fatty acids, and amino acids, which eventually reduces obesity (62–64). Obesity is also a risk factor for dementia (65). This indicates that increased cortisol concentrations reduce obesity, which somewhat decreases the incidence of dementia. Second, some limitations must be taken into account. Ferrari, E. et al. (66) showed that cortisol levels were significantly higher during the nighttime in elderly subjects. The GWAS database for plasma cortisol was restricted to samples collected in the morning, which had an impact on the MR analysis. In addition, there was nonrandom selection in the cohort, there were low response rates, and the individuals had a relatively high average education and general health status, which may have affected the results, particularly in the UK Biobank data.
This study demonstrates that higher plasma cortisol is associated with a higher risk of VaD (67). Reports of observational studies on cortisol levels in patients with vascular dementia are disputed, with some studies finding low levels of cortisol following cerebral infarction and others suggesting elevated cortisol concentrations (68, 69). Our investigation offered an important additional source for the evidence that plasma cortisol increased the risk of VaD. The stress response of the body and other neurohumoral factors can also affect the function of the HPA axis (70). Alterations in the HPA axis that result in higher plasma cortisol levels have been linked to corticoid-dependent hippocampal damage and poststroke brain disorders, which can cause cognitive impairment (71). The hippocampus and frontal cortex, which are critical for memory and learning, might suffer damage from elevated plasma cortisol levels (72). This interferes with the development of memory and learning, thus increasing memory loss and cognitive decline. As cerebral infarction proceeds, cerebral edema gradually increases, affecting the blood supply to the pituitary gland and inhibiting the function of the HPA axis, resulting in a decrease in adrenocorticotropic hormone and cortisol secretion (73).
Genetically predicted plasma cortisol was not associated with DLB or FTD, which is consistent with previous observational studies (74, 75). Although Woolley et al. (74) observed significantly lower plasma cortisol levels in patients with FTD, their findings suggested that the reduction in plasma cortisol is probably because of the production of ghrelin in the gastric mucosa after eating (76); thus, there is no clear causal association with FTD, which is consistent with the results of our study. Some researchers have shown that the anticholinergic activity of DLB occurs through reduced plasma cortisol levels, which are caused by severe autonomic parasympathetic dysfunction in DLB (75). Therefore, a bidirectional MR analysis can be undertaken in the future for plasma cortisol and DLB, and the causal association can be further defined.
4.2 Plasma cortisol and epilepsy
Using two-sample MR analysis, genetic variants were causally related to the risk of epilepsy, which is consistent with previous findings (77, 78). Epilepsy is a chronic neurological condition defined by spontaneous recurrent epilepsy (continuous synchronous discharge of neurons). The supraoptic nucleus of the hypothalamus is related to the HPA axis, which directly instructs the rhythm of plasma cortisol secretion. Circadian rhythms of plasma cortisol concentrations may alter several essential homeostatic processes in the body, including the balance between neuronal excitability and inhibition (79). Many researchers believe that plasma cortisol may modify neuronal excitability and epilepsy through GABA levels, which may have a substantial impact on signal transduction and epilepsy susceptibility. Some individuals are more susceptible to having epilepsy, especially when feeling stressed or anxious. Chronic stress decreases the synthesis of GABAergic neurotransmitters, and the loss may impair GABAergic inhibition and generate a neuronal state that is prone to hyperexcitability (21).
4.3 Plasma cortisol and multiple sclerosis
The present study did not find a causal association between plasma cortisol and the risk of developing MS, which is different from many observational studies (80, 81). MS is associated with a variety of neurological symptoms (82), including sensory disturbances, visual problems, fatigue, altered balance, cognitive dysfunction, anxiety and depression (83–88). Many studies have shown that the HPA axis plays an important role in controlling the disease process in MS (89, 90), and researchers have also revealed that anxiety and depression are significantly linked to higher levels of cortisol, especially in patients with relapsing-remitting MS (91, 92). The previous findings differ from the results of our study. This may be because the GWAS data of MS we used did not contain information on MS with depression or anxiety, so we could not clarify whether cortisol is causally associated with depression or anxiety symptoms. In addition, there is a significant sex difference in the incidence of MS, particularly in young women. This study did not classify MS patients by sex, and the causal correlation between sex differences and dysfunction of the HPA could not be clarified. Further research can be conducted l in the future.
This MR study should be confirmed or refuted when larger GWAS databases emerge for plasma cortisol. Nevertheless, our findings may trigger a discussion about the relevance and importance of the endocrine system, especially the HPA axis to the central nervous system.
4.4 Strengths and limitations
This study has several strengths. This was the first study to examine the causal association between plasma cortisol and dementia, epilepsy, and MS using data from GWAS. Compared to observational research, MR analysis minimized the effects of confounding factors. This study also has certain limitations. First, the GWAS data used in our study are limited in number and ancestry, and the relevant SNPs may not have been fully identified. The low sample size of this study may also affect the stability of the results. Second, this study investigated European ancestry for the exposure and outcome, so our results may not be applicable to other populations.
5 Conclusions
This study demonstrated that plasma cortisol increased the incidence rates of VaD and epilepsy and decreased the incidence rates of AD and PDD. Our MR results did not reveal evidence of a causal relationship between plasma cortisol and DLB, FTD or MS. Monitoring plasma cortisol concentrations can help prevent diseases in clinical practice.
Data availability statement
Publicly available datasets were analyzed in this study. This data can be found here: https://gwas.mrcieu.ac.uk/.
Author contributions
HL drafted the manuscript; KC and LY assisted in the preparation of the manuscript and performed the analysis; QW performed the analysis; JZ contributed to the data extraction; and JH designed the study. All authors contributed to the article and approved the submitted version.
Funding
This work was supported by National Natural Science Foundation of China Youth Fund (No.81902292); Jilin Provincial Department of Finance Project (No.2020SCZ10); Jilin Provincial Health Youth Science and Technology Backbone Cultivation Project (No.2019Q018); Bethune Project of Jilin University (No.2020B60); Jilin Provincial Science and Technology Development Project (No.20210101227JC); Jilin Provincial Health Youth Science and Technology Backbone Cultivation Project (No.2019Q035); Jilin Provincial Department of Finance Project (No.2021SCZ08).
Acknowledgments
We are grateful to the International Multiple Sclerosis Genetics Consortium for conducting GWAS and sharing summary-level data on multiple sclerosis. We want to acknowledge the participants and investigators of the FinnGen study and UK Biobank.
Conflict of interest
The authors declare that the research was conducted in the absence of any commercial or financial relationships that could be construed as a potential conflict of interest.
Publisher’s note
All claims expressed in this article are solely those of the authors and do not necessarily represent those of their affiliated organizations, or those of the publisher, the editors and the reviewers. Any product that may be evaluated in this article, or claim that may be made by its manufacturer, is not guaranteed or endorsed by the publisher.
Supplementary material
The Supplementary Material for this article can be found online at: https://www.frontiersin.org/articles/10.3389/fendo.2023.1107780/full#supplementary-material
References
1. Hebda-Bauer EK, Simmons TA, Sugg A, Ural E, Stewart JA, Beals JL, et al. 3xTg-AD mice exhibit an activated central stress axis during early-stage pathology. J Alzheimers Dis (2013) 33(2):407–22. doi: 10.3233/JAD-2012-121438
2. Flak JN, Myers B, Solomon MB, McKlveen JM, Krause EG, Herman JP. Role of paraventricular nucleus-projecting norepinephrine/epinephrine neurons in acute and chronic stress. Eur J Neurosci (2014) 39(11):1903–11. doi: 10.1111/ejn.12587
3. Pieretti S, Di Giannuario A, Loizzo A, Sagratella S, Scotti de Carolis A, Capasso A, et al. Dexamethasone prevents epileptiform activity induced by morphine in in vivo and in vitro experiments. J Pharmacol Exp Ther (1992) 263(2):830–9.
4. McCarron HR, Zmora R, Gaugler JE. A web-based mobile app with a smartwatch to support social engagement in persons with memory loss: Pilot randomized controlled trial. JMIR Aging (2019) 2(1):e13378. doi: 10.2196/13378
5. Pistollato F, Sumalla Cano S, Elio I, Masias Vergara M, Giampieri F, Battino M. Associations between sleep, cortisol regulation, and diet: Possible implications for the risk of Alzheimer disease. Adv Nutr (2016) 7(4):679–89. doi: 10.3945/an.115.011775
6. Ren RJ, Yin P, Wang ZH, Qi JL, Tang R, Wang JT, et al. China Alzheimer's disease report 2021. Diagn Theory Practice (2021) 20(04):317–37. doi: 10.16150/j.1671-2870.2021.04.001
7. Xuelian S, Yiping D, Bilong D. A brief analysis of the key points of dementia and alzheimer's disease progression. Modern Clin Med (2022) 48(04):310–3. doi: 10.11851/j.issn.1673-1557.2022.04.020
8. Hugo J, Ganguli M. Dementia and cognitive impairment: epidemiology, diagnosis, and treatment. Clin Geriatr Med (2014) 30(3):421–42. doi: 10.1016/j.cger.2014.04.001
9. Arnaoutoglou NA, O'Brien JT, Underwood BR. Dementia with lewy bodies - from scientific knowledge to clinical insights. Nat Rev Neurol (2019) 15(2):103–12. doi: 10.1038/s41582-018-0107-7
10. Aarsland D, Kurz MW. The epidemiology of dementia associated with parkinson's disease. Brain Pathol (2010) 20(3):633–9. doi: 10.1111/j.1750-3639.2009.00369.x
11. Bang J, Spina S, Miller BL. Frontotemporal dementia. Lancet (2015) 386(10004):1672–82. doi: 10.1016/S0140-6736(15)00461-4
12. China Dementia and Cognitive Disorders Guideline Writing Group, Cognitive Disorders Committee of the Neurologist Branch of the Chinese Medical Association 2018 China dementia and cognitive disorders diagnosis and treatment guidelines (I): Dementia and its classification and diagnostic criteria. Chin Med J (2018) 98(13):965–70. doi: 10.3760/cma.j.issn.0376-2491.2018.13.003
13. Yeram N, Dalvi S, Mankeshwar R, Patil V, Kale V, Jagiasi K, et al. Relationship between cortisol, interleukin-6 and homocysteine in alzheimer's disease. Qatar Med J (2021) 2021(33):1–10. doi: 10.5339/qmj.2021.33
14. Udeh-Momoh CT, Su B, Evans S, Zheng B, Sindi S, Tzoulaki I, et al. Cortisol, amyloid-beta, and reserve predicts alzheimer's disease progression for cognitively normal older adults. J Alzheimers Dis (2019) 70(2):553–62. doi: 10.3233/JAD-181030
15. Ouanes S, Clark C, Richiardi J, Marechal B, Lewczuk P, Kornhuber J, et al. Cerebrospinal fluid cortisol and dehydroepiandrosterone sulfate, alzheimer's disease pathology, and cognitive decline. Front Aging Neurosci (2022) 14:892754. doi: 10.3389/fnagi.2022.892754
16. Thijs RD, Surges R, O'Brien TJ, Sander JW. Epilepsy in adults. Lancet (2019) 393(10172):689–701. doi: 10.1016/S0140-6736(18)32596-0
17. Weaver DF, Pohlmann-Eden B. Pharmacoresistant epilepsy: unmet needs in solving the puzzle(s). Epilepsia (2013) 54 Suppl 2:80–5. doi: 10.1111/epi.12191
18. Zhang P, Xu Y, Cheng G, Zhou WH. Interpretation of the expert recommendations of the international league against epilepsy task force on neonatal seizure classification (2021). Chin Clin J Pract Pediatrics (2021) 36(20):1534–7. doi: 10.3760/cma.j.cn101070-20210811-00957
19. van Campen JS, Valentijn FA, Jansen FE, Joels M, Braun KP. Seizure occurrence and the circadian rhythm of cortisol: a systematic review. Epilepsy Behav (2015) 47:132–7. doi: 10.1016/j.yebeh.2015.04.071
20. Young EA, Abelson J, Lightman SL. Cortisol pulsatility and its role in stress regulation and health. Front Neuroendocrinol (2004) 25(2):69–76. doi: 10.1016/j.yfrne.2004.07.001
21. Sarabdjitsingh RA, Jezequel J, Pasricha N, Mikasova L, Kerkhofs A, Karst H, et al. Ultradian corticosterone pulses balance glutamatergic transmission and synaptic plasticity. Proc Natl Acad Sci U S A. (2014) 111(39):14265–70. doi: 10.1073/pnas.1411216111
22. Reich DS, Lucchinetti CF, Calabresi PA. Multiple sclerosis. N Engl J Med (2018) 378(2):169–80. doi: 10.1056/NEJMra1401483
23. Stenager E. A global perspective on the burden of multiple sclerosis. Lancet Neurol (2019) 18(3):227–8. doi: 10.1016/S1474-4422(18)30498-8
24. Collaborators GBDMS. Global, regional, and national burden of multiple sclerosis 1990-2016: a systematic analysis for the global burden of disease study 2016. Lancet Neurol (2019) 18(3):269–85. doi: 10.1016/S1474-4422(18)30499-X
25. Ochi H, Fujihara K. Demyelinating diseases in Asia. Curr Opin Neurol (2016) 29(3):222–8. doi: 10.1097/WCO.0000000000000328
26. Heesen C, Gold SM, Raji A, Wiedemann K, Schulz KH. Cognitive impairment correlates with hypothalamo-pituitary-adrenal axis dysregulation in multiple sclerosis. Psychoneuroendocrinology (2002) 27(4):505–17. doi: 10.1016/S0306-4530(01)00071-3
27. Pereira GM, Becker J, Soares NM, de Azeredo LA, Grassi-Oliveira R, Rysdyk A, et al. Hair cortisol concentration, cognitive, behavioral, and motor impairment in multiple sclerosis. J Neural Transm (Vienna) (2019) 126(9):1145–54. doi: 10.1007/s00702-019-02040-w
28. Burgess S, Butterworth A, Thompson SG. Mendelian randomization analysis with multiple genetic variants using summarized data. Genet Epidemiol (2013) 37(7):658–65. doi: 10.1002/gepi.21758
29. Bowden J, Holmes MV. Meta-analysis and mendelian randomization: A review. Res Synth Methods (2019) 10(4):486–96. doi: 10.1002/jrsm.1346
30. Rees JMB, Wood AM, Burgess S. Extending the MR-egger method for multivariable mendelian randomization to correct for both measured and unmeasured pleiotropy. Stat Med (2017) 36(29):4705–18. doi: 10.1002/sim.7492
31. Bolton JL, Hayward C, Direk N, Lewis JG, Hammond GL, Hill LA, et al. Genome wide association identifies common variants at the SERPINA6/SERPINA1 locus influencing plasma cortisol and corticosteroid binding globulin. PloS Genet (2014) 10(7):e1004474. doi: 10.1371/journal.pgen.1004474
32. Sudlow C, Gallacher J, Allen N, Beral V, Burton P, Danesh J, et al. UK Biobank: an open access resource for identifying the causes of a wide range of complex diseases of middle and old age. PloS Med (2015) 12(3):e1001779. doi: 10.1371/journal.pmed.1001779
33. FinnGen-tutkimushanke vie suomalaiset löytöretkelle genomitietoon. FinnGen. Available at: https://www.finngen.fi/fi/finngen_tutkimushanke_vie_suomalaiset_loytoretkelle_genomitietoon.
34. Si S, Li J, Tewara MA, Xue F. Genetically determined chronic low-grade inflammation and hundreds of health outcomes in the UK biobank and the FinnGen population: A phenome-wide mendelian randomization study. Front Immunol (2021) 12:720876. doi: 10.3389/fimmu.2021.720876
35. Sheng J, Liu J, Chan KHK. Evaluating the causal effects of gestational diabetes mellitus, heart disease, and high body mass index on maternal alzheimer's disease and dementia: Multivariable mendelian randomization. Front Genet (2022) 13:833734. doi: 10.3389/fgene.2022.833734
36. Klinedinst BS, Le ST, Larsen B, Pappas C, Hoth NJ, Pollpeter A, et al. Genetic factors of alzheimer's disease modulate how diet is associated with long-term cognitive trajectories: A UK biobank study. J Alzheimers Dis (2020) 78(3):1245–57. doi: 10.3233/JAD-201058
37. Chia R, Sabir MS, Bandres-Ciga S, Saez-Atienzar S, Reynolds RH, Gustavsson E, et al. Genome sequencing analysis identifies new loci associated with lewy body dementia and provides insights into its genetic architecture. Nat Genet (2021) 53(3):294–303. doi: 10.1038/s41588-021-00785-3
38. Van Deerlin VM, Sleiman PM, Martinez-Lage M, Chen-Plotkin A, Wang LS, Graff-Radford NR, et al. Common variants at 7p21 are associated with frontotemporal lobar degeneration with TDP-43 inclusions. Nat Genet (2010) 42(3):234–9. doi: 10.1038/ng.536
39. Harroud A, Mitchell RE, Richardson TG, Morris JA, Forgetta V, Davey Smith G, et al. Childhood obesity and multiple sclerosis: A mendelian randomization study. Mult Scler (2021) 27(14):2150–8. doi: 10.1177/13524585211001781
40. International Multiple Sclerosis Genetics C. Multiple sclerosis genomic map implicates peripheral immune cells and microglia in susceptibility. Science (2019) 365(6460):eaav7188. doi: 10.1126/science.aav7188
41. Hemani G, Zheng J, Elsworth B, Wade KH, Haberland V, Baird D, et al. The MR-base platform supports systematic causal inference across the human phenome. Elife (2018) 7:e34408. doi: 10.7554/eLife.34408
42. Purcell S, Neale B, Todd-Brown K, Thomas L, Ferreira MA, Bender D, et al. PLINK: a tool set for whole-genome association and population-based linkage analyses. Am J Hum Genet (2007) 81(3):559–75. doi: 10.1086/519795
43. Larsson SC, Lee WH, Kar S, Burgess S, Allara E. Assessing the role of cortisol in cancer: a wide-ranged mendelian randomisation study. Br J Cancer (2021) 125(7):1025–9. doi: 10.1038/s41416-021-01505-8
44. Katsuhara S, Yokomoto-Umakoshi M, Umakoshi H, Matsuda Y, Iwahashi N, Kaneko H, et al. Impact of cortisol on reduction in muscle strength and mass: A mendelian randomization study. J Clin Endocrinol Metab (2022) 107(4):e1477–e87. doi: 10.1210/clinem/dgab862
45. Larsson SC, Lee WH, Burgess S, Allara E. Plasma cortisol and risk of atrial fibrillation: A mendelian randomization study. J Clin Endocrinol Metab (2021) 106(7):e2521–e6. doi: 10.1210/clinem/dgab219
46. Bowden J, Del Greco MF, Minelli C, Davey Smith G, Sheehan N, Thompson J. A framework for the investigation of pleiotropy in two-sample summary data mendelian randomization. Stat Med (2017) 36(11):1783–802. doi: 10.1002/sim.7221
47. Burgess S, Small DS, Thompson SG. A review of instrumental variable estimators for mendelian randomization. Stat Methods Med Res (2017) 26(5):2333–55. doi: 10.1177/0962280215597579
48. Burgess S, Thompson SG. Interpreting findings from mendelian randomization using the MR-egger method. Eur J Epidemiol (2017) 32(5):377–89. doi: 10.1007/s10654-017-0255-x
49. Bowden J, Davey Smith G, Burgess S. Mendelian randomization with invalid instruments: effect estimation and bias detection through egger regression. Int J Epidemiol (2015) 44(2):512–25. doi: 10.1093/ije/dyv080
50. Davey Smith G, Hemani G. Mendelian randomization: genetic anchors for causal inference in epidemiological studies. Hum Mol Genet (2014) 23(R1):R89–98. doi: 10.1093/hmg/ddu328
51. Hemani G, Bowden J, Davey Smith G. Evaluating the potential role of pleiotropy in mendelian randomization studies. Hum Mol Genet (2018) 27(R2):R195–208. doi: 10.1093/hmg/ddy163
52. Jia Y, Liu L, Sheng C, Cheng Z, Cui L, Li M, et al. Increased serum levels of cortisol and inflammatory cytokines in people with depression. J Nerv Ment Dis (2019) 207(4):271–6. doi: 10.1097/NMD.0000000000000957
53. Choi KW, Na EJ, Fava M, Mischoulon D, Cho H, Jeon HJ. Increased adrenocorticotropic hormone (ACTH) levels predict severity of depression after six months of follow-up in outpatients with major depressive disorder. Psychiatry Res (2018) 270:246–52. doi: 10.1016/j.psychres.2018.09.047
54. Syafrita Y, Amir D, Susanti R, Fadhilah I. Relationship of brain-derived neurotrophic factor, malondialdehyde, and 8-hydroxy 2-deoxyguanosine with post-ischemic stroke depression. Dement Neuropsychol (2020) 14(1):41–6. doi: 10.1590/1980-57642020dn14-010007
55. Yao Z, Liu B, Wang Y, Dong X. High cortisol and the risk of Alzheimer disease: A protocol for systematic review and meta-analysis. Med (Baltimore) (2021) 100(39):e27319. doi: 10.1097/MD.0000000000027319
56. Zheng B, Tal R, Yang Z, Middleton L, Udeh-Momoh C. Cortisol hypersecretion and the risk of alzheimer's disease: A systematic review and meta-analysis. Ageing Res Rev (2020) 64:101171. doi: 10.1016/j.arr.2020.101171
57. Pena-Bautista C, Baquero M, Ferrer I, Hervas D, Vento M, Garcia-Blanco A, et al. Neuropsychological assessment and cortisol levels in biofluids from early alzheimer's disease patients. Exp Gerontol (2019) 123:10–6. doi: 10.1016/j.exger.2019.05.007
58. Skogar O, Fall PA, Hallgren G, Lokk J, Bringer B, Carlsson M, et al. Diurnal salivary cortisol concentrations in parkinson's disease: increased total secretion and morning cortisol concentrations. Int J Gen Med (2011) 4:561–9. doi: 10.2147/IJGM.S20875
59. Tornhage CJ, Skogar O, Borg A, Larsson B, Robertsson L, Andersson L, et al. Short- and long-term effects of tactile massage on salivary cortisol concentrations in parkinson's disease: a randomised controlled pilot study. BMC Complement Altern Med (2013) 13:357. doi: 10.1186/1472-6882-13-357
60. Hartmann A, Veldhuis JD, Deuschle M, Standhardt H, Heuser I. Twenty-four hour cortisol release profiles in patients with alzheimer's and parkinson's disease compared to normal controls: ultradian secretory pulsatility and diurnal variation. Neurobiol Aging (1997) 18(3):285–9. doi: 10.1016/S0197-4580(97)80309-0
61. Qi X, Cui B, Cao M. The role of morning plasma cortisol in obesity: A bidirectional mendelian randomization study. J Clin Endocrinol Metab (2022) 107(5):e1954–e60. doi: 10.1210/clinem/dgac008
62. Dinneen S, Alzaid A, Miles J, Rizza R. Metabolic effects of the nocturnal rise in cortisol on carbohydrate metabolism in normal humans. J Clin Invest (1993) 92(5):2283–90. doi: 10.1172/JCI116832
63. Djurhuus CB, Gravholt CH, Nielsen S, Mengel A, Christiansen JS, Schmitz OE, et al. Effects of cortisol on lipolysis and regional interstitial glycerol levels in humans. Am J Physiol Endocrinol Metab (2002) 283(1):E172–7. doi: 10.1152/ajpendo.00544.2001
64. Arlt W, Stewart PM. Adrenal corticosteroid biosynthesis, metabolism, and action. Endocrinol Metab Clin North Am (2005) 34(2):293–313, viii. doi: 10.1016/j.ecl.2005.01.002
65. Selman A, Burns S, Reddy AP, Culberson J, Reddy PH. The role of obesity and diabetes in dementia. Int J Mol Sci (2022) 23(16):9267. doi: 10.3390/ijms23169267
66. Ferrari E, Arcaini A, Gornati R, Pelanconi L, Cravello L, Fioravanti M, et al. Pineal and pituitary-adrenocortical function in physiological aging and in senile dementia. Exp Gerontol (2000) 35(9-10):1239–50. doi: 10.1016/S0531-5565(00)00160-1
67. van den Beld AW, Kaufman JM, Zillikens MC, Lamberts SWJ, Egan JM, van der Lely AJ. The physiology of endocrine systems with ageing. Lancet Diabetes Endocrinol (2018) 6(8):647–58. doi: 10.1016/S2213-8587(18)30026-3
68. Terroni L, Amaro E Jr., Iosifescu DV, Mattos P, Yamamoto FI, Tinone G, et al. The association of post-stroke anhedonia with salivary cortisol levels and stroke lesion in hippocampal/parahippocampal region. Neuropsychiatr Dis Treat (2015) 11:233–42. doi: 10.2147/NDT.S73722
69. Cai YY, Liu ZS, Wang SH, Wang QF, Cui XM, Qu L. Effects of electroacupuncture on hypothalamic-pituitary-adrenal axis-related hormones in rats with cerebral ischemia-reperfusion injury. Acupuncture Res (2009) 34(05):297–303. doi: 10.13702/j.1000-0607.2009.05.003
70. Zheng X-M, Huang J-S. Correlation of changes in pituitary-adrenal axis and leptin function in patients with multiple infarct dementia. Chin J Gerontol (2012) 32(23):5132–4. doi: 10.3969/j.issn.1005-9202.2012.23.014
71. Huang S, Liu L, Tang X, Xie S, Li X, Kang X, et al. Research progress on the role of hormones in ischemic stroke. Front Immunol (2022) 13:1062977. doi: 10.3389/fimmu.2022.1062977
72. Zheng ZJ, Yan ZR, Qin F. Correlation of plasma cortisol, brain-derived neurotrophic factor, and nitrite levels with depressive status after acute ischemic stroke. Trauma Acute Crit Care Med (2021) 9(05):331–6. doi: 10.16048/j.issn.2095-5561.2021.05.02
73. Jauch EC, Saver JL, Adams HP Jr., Bruno A, Connors JJ, Demaerschalk BM, et al. Guidelines for the early management of patients with acute ischemic stroke: a guideline for healthcare professionals from the American heart Association/American stroke association. Stroke (2013) 44(3):870–947. doi: 10.1161/STR.0b013e318284056a
74. Woolley JD, Khan BK, Natesan A, Karydas A, Dallman M, Havel P, et al. Satiety-related hormonal dysregulation in behavioral variant frontotemporal dementia. Neurology (2014) 82(6):512–20. doi: 10.1212/WNL.0000000000000106
75. Kitajima Y, Hori K, Konishi K, Tani M, Tomioka H, Akashi N, et al. A review of the role of anticholinergic activity in lewy body disease and delirium. Neurodegener Dis (2015) 15(3):162–7. doi: 10.1159/000381522
76. Geliebter A, Gluck ME, Hashim SA. Plasma ghrelin concentrations are lower in binge-eating disorder. J Nutr (2005) 135(5):1326–30. doi: 10.1093/jn/135.5.1326
77. Durazzo TS, Spencer SS, Duckrow RB, Novotny EJ, Spencer DD, Zaveri HP. Temporal distributions of seizure occurrence from various epileptogenic regions. Neurology (2008) 70(15):1265–71. doi: 10.1212/01.wnl.0000308938.84918.3f
78. den Heijer JM, Otte WM, van Diessen E, van Campen JS, Lorraine Hompe E, Jansen FE, et al. The relation between cortisol and functional connectivity in people with and without stress-sensitive epilepsy. Epilepsia (2018) 59(1):179–89. doi: 10.1111/epi.13947
79. Do MTH. Melanopsin and the intrinsically photosensitive retinal ganglion cells: Biophysics to behavior. Neuron (2019) 104(2):205–26. doi: 10.1016/j.neuron.2019.07.016
80. Kern S, Krause I, Horntrich A, Thomas K, Aderhold J, Ziemssen T. Cortisol awakening response is linked to disease course and progression in multiple sclerosis. PloS One (2013) 8(4):e60647. doi: 10.1371/journal.pone.0060647
81. Trifunovic S, Stevanovic I, Milosevic A, Ristic N, Janjic M, Bjelobaba I, et al. The function of the hypothalamic-Pituitary-Adrenal axis during experimental autoimmune encephalomyelitis: Involvement of oxidative stress mediators. Front Neurosci (2021) 15:649485. doi: 10.3389/fnins.2021.649485
82. Kern S, Schultheiss T, Schneider H, Schrempf W, Reichmann H, Ziemssen T. Circadian cortisol, depressive symptoms and neurological impairment in early multiple sclerosis. Psychoneuroendocrinology (2011) 36(10):1505–12. doi: 10.1016/j.psyneuen.2011.04.004
83. Sakai RE, Feller DJ, Galetta KM, Galetta SL, Balcer LJ. Vision in multiple sclerosis: the story, structure-function correlations, and models for neuroprotection. J Neuroophthalmol (2011) 31(4):362–73. doi: 10.1097/WNO.0b013e318238937f
84. Pilutti LA, Greenlee TA, Motl RW, Nickrent MS, Petruzzello SJ. Effects of exercise training on fatigue in multiple sclerosis: a meta-analysis. Psychosom Med (2013) 75(6):575–80. doi: 10.1097/PSY.0b013e31829b4525
85. Gunn H, Markevics S, Haas B, Marsden J, Freeman J. Systematic review: The effectiveness of interventions to reduce falls and improve balance in adults with multiple sclerosis. Arch Phys Med Rehabil (2015) 96(10):1898–912. doi: 10.1016/j.apmr.2015.05.018
86. Pearson M, Dieberg G, Smart N. Exercise as a therapy for improvement of walking ability in adults with multiple sclerosis: a meta-analysis. Arch Phys Med Rehabil (2015) 96(7):1339–48 e7. doi: 10.1016/j.apmr.2015.02.011
87. Phe V, Chartier-Kastler E, Panicker JN. Management of neurogenic bladder in patients with multiple sclerosis. Nat Rev Urol (2016) 13(5):275–88. doi: 10.1038/nrurol.2016.53
88. Coric D, Balk LJ, Verrijp M, Eijlers A, Schoonheim MM, Killestein J, et al. Cognitive impairment in patients with multiple sclerosis is associated with atrophy of the inner retinal layers. Mult Scler (2018) 24(2):158–66. doi: 10.1177/1352458517694090
89. Biernacki T, Kokas Z, Sandi D, Fuvesi J, Fricska-Nagy Z, Farago P, et al. Emerging biomarkers of multiple sclerosis in the blood and the CSF: A focus on neurofilaments and therapeutic considerations. Int J Mol Sci (2022) 23(6):3383. doi: 10.3390/ijms23063383
90. Chitnis T, Vandercappellen J, King M, Brichetto G. Symptom interconnectivity in multiple sclerosis: A narrative review of potential underlying biological disease processes. Neurol Ther (2022) 11(3):1043–70. doi: 10.1007/s40120-022-00368-2
91. Powell DJ, Moss-Morris R, Liossi C, Schlotz W. Circadian cortisol and fatigue severity in relapsing-remitting multiple sclerosis. Psychoneuroendocrinology (2015) 56:120–31. doi: 10.1016/j.psyneuen.2015.03.010
Keywords: Mendelian randomization, plasma cortisol, dementia, epilepsy, multiple sclerosis
Citation: Li H, Chen K, Yang L, Wang Q, Zhang J and He J (2023) The role of plasma cortisol in dementia, epilepsy, and multiple sclerosis: A Mendelian randomization study. Front. Endocrinol. 14:1107780. doi: 10.3389/fendo.2023.1107780
Received: 25 November 2022; Accepted: 28 February 2023;
Published: 15 March 2023.
Edited by:
Wei Wang, Brigham and Women’s Hospital and Harvard Medical School, United StatesReviewed by:
Gaoyang Chen, Jinan University, ChinaHecheng Ma, Qilu Hospital, Shandong University, China
Lingling Wang, Beihua University Hospital, China
Jiahao Cai, Guangzhou Medical University, China
Copyright © 2023 Li, Chen, Yang, Wang, Zhang and He. This is an open-access article distributed under the terms of the Creative Commons Attribution License (CC BY). The use, distribution or reproduction in other forums is permitted, provided the original author(s) and the copyright owner(s) are credited and that the original publication in this journal is cited, in accordance with accepted academic practice. No use, distribution or reproduction is permitted which does not comply with these terms.
*Correspondence: Jinting He, aGVqdEBqbHUuZWR1LmNu
†These authors have contributed equally to this work and share first authorship