- 1Department of Endocrinology, Institute of Geriatric Medicine, Liyuan Hospital, Tongji Medical College, Huazhong University of Science and Technology, Wuhan, China
- 2Geriatric Medicine Center, Key Laboratory of Endocrine Gland Diseases of Zhejiang Province, Department of Endocrinology, Zhejiang Provincial People’s Hospital, Affiliated People’s Hospital, Hangzhou Medical College, Hangzhou, China
Background: Neutrophil/high-density lipoprotein (HDL) ratio (NHR), monocyte/HDL ratio (MHR), lymphocyte/HDL ratio (LHR), platelet/HDL ratio (PHR), systemic immune-inflammation index (SII), system inflammation response index (SIRI), and aggregate index of systemic inflammation (AISI) have been recently investigated as novel inflammatory markers. Herein, the correlation was investigated between these inflammatory biomarkers and peripheral arterial disease (PAD) in type 2 diabetes mellitus (T2DM) patients.
Methods: In this retrospective observational study, the hematological parameter data of 216 T2DM patients without PAD (T2DM-WPAD) and 218 T2DM patients with PAD (T2DM-PAD) at Fontaine stages II, III or IV stage had been collected. Differences in NHR, MHR, LHR, PHR, SII, SIRI, and AISI were analyzed, and receiver operating characteristic (ROC) curves were used to analyze the diagnostic potential of these parameters.
Results: The levels of NHR, MHR, PHR, SII, SIRI and AISI in T2DM-PAD patients were significantly higher than in T2DM-WPAD patients (P < 0.001). They were correlated with disease severity. Further, multifactorial logistic regression analyses showed that higher NHR, MHR, PHR, SII, SIRI, and AISI might be independent risk factors for T2DM-PAD (P < 0.001). The areas under the curve (AUCs) of the NHR, MHR, PHR, SII, SIRI, and AISI for T2DM-PAD patients was 0.703, 0.685, 0.606, 0.648, 0.711, and 0.670, respectively. The AUC of the NHR and SIRI combined model was 0.733.
Conclusion: The levels of NHR, MHR, PHR, SII, SIRI, and AISI were higher in T2DM-PAD patients, and they were independently linked with its clinical severity. The combination model of NHR and SIRI was most valuable for predicting T2DM – PAD.
Introduction
Patients with T2DM and PAD have a cardiovascular mortality risk five times higher than patients with only one disease (1, 2). Therefore, early recognition and intervention of PAD in diabetic patients are necessary to lower the risk of major adverse limb events (MALEs) (3). The ankle-brachial index (ABI) is currently recommended as the primary screening tool for PAD in diabetic patients and those with multiple risk factors (4). Due to the low sensitivity of the ABI for early-stage PAD identification, finding new biomarkers that can identify PAD in diabetic individuals at an early stage is urgent.
Recent research has suggested that inflammation and lipid metabolism play a significant role in PAD pathogenesis (5, 6). Besides its capacity to transport cholesterol in the opposite direction, high-density lipoprotein cholesterol (HDL-C) has various protective properties, such as those related to infection, inflammation, antioxidants, and thrombosis (7). Obtaining the numbers of neutrophils, monocytes, lymphocytes, and platelets is inexpensive and easy via complete blood count, which are altered when an organism is inflamed. Furthermore, new hematological parameters related to HDL-C and complete blood cells, such as NHR (8), MHR (9), LHR (10), PHR (11), SII (12), SIRI (13, 14), and AISI (15) have been proposed as novel inflammatory biomarkers.
Relevant studies with single or two combined indicators in populations with diabetes or PAD are currently available (16–24). However, until now, no data has been found about how NHR, MHR, LHR, PHR, SII, SIRI, and AISI are linked to T2DM-PAD patients. Herein, the connection was explored between these inflammatory biomarkers and T2DM-PAD patients.
Materials and methods
Study population
Gender-matched individuals with T2DM were consecutively recruited from the Department of Endocrinology and Metabolism of the Liyuan Hospital affiliated to Tongji Medical College, Huazhong University of Science and Technology (Wuhan, China), from 1 June 2020 to 31 September 2022. The inclusion criteria are outlined in Table 1. Based on the T2DM international criteria (ADA) (25), T2DM was defined as a fasting plasma glucose level ≥ 7.0 mmol/L and/or 2-h plasma glucose ≥11.1 mmol/L during oral glucose tolerance tests (OGTT) and/or glycosylated hemoglobin (HbA1c) level ≥ 6.5%. Each patient included in the study was evaluated for a history of PAD symptoms or a verified diagnosis of PAD using the criteria established by the Ad Hoc Committee on Reporting Standards of the Society for Vascular Surgery and the International Society of Cardiovascular Surgery (26, 27). The ABI was measured in patients with PAD-like symptoms, and the physician evaluated the patient’s lower extremities using arterial Doppler-enhanced ultrasonography if they had symptoms in both legs. Patients who had an ABI > 0.90 but showed no symptoms of PAD were not further tested for the disease.
The severity of PAD was assessed using the Fontaine classification, comprising four stages: I - asymptomatic; II - intermittent claudication; III - rest pain; and IV - ischemic ulcers or gangrene (28). Following the recommendations of the Inter-Society Consensus for the Management of Peripheral Arterial Disease (TASC II), patients with ischemic rest pain, ulcers, or gangrene, attributable to objectively proven PAD, were considered affected by critical limb ischemia (CLI) (29). Based on the T2DM – PAD patients’ clinical symptoms, the patients were divided into two groups, the first being those affected by stable PAD (Fontaine’s II) and the second being those affected by CLI (Fontaine’s III and IV).
Demographic and clinical assessment
Data from the electronic medical records of the relevant departments was analyzed. Factors such as age, gender, diagnoses, and lab results were recorded. On the second hospital morning, blood samples were collected from all patients’ periphery. Laboratory personnel unaware of the patient’s diagnoses analyzed the blood samples.
The NHR, MHR, LHR, PHR, SII, SIRI, AISI, and triglyceride glucose index (TyG index) were calculated using the following formulas: NHR = neutrophil/HDL ratio; MHR = monocyte/HDL; LHR = lymphocyte/HDL ratio; PHR = platelet/HDL ratio; SII = platelet × neutrophil-to-lymphocyte ratio, SIRI = monocyte × neutrophil-to-lymphocyte ratio; AISI = neutrophil × platelet× monocyte-to-lymphocyte ratio; TyG index = Ln [Triglyceride (TG, mg/dl) × Fasting plasma glucose (mg/dl)/2].
Statistical analysis
Statistical analyses were done using SPSS version 27.0 software (SPSS, Inc., Chicago, IL, United States). Graphs were created using Prism 9.0 (GraphPad Software). Continuous variables are described as means ± standard deviations (SDs) or medians (interquartile ranges), depending on the data distribution. Categorical variables are expressed as numbers and percentages of patients. Student’s t-test and the Mann-Whitney U test were used to compare the two groups. One-way ANOVA (normally distributed variables) or the Kruskal-Wallis test (non-normally distributed variables) was used to analyze the differences between the three groups. For categorical variables, chi-square tests were performed. Spearman’s correlation analysis was used to look at the connections between the different variables. Indicators with covariance were excluded from the correlation analysis. For example, NHR was covariant with neutrophils and HDL-C, and the Spearman correlation analysis was not conducted between the NHR and both variables. Binary logistic regression analysis was performed to explore the associations between NHR, MHR, PHR, LHR, SII, SIRI, and AISI and PAD. Due to the small variance of MHR as a continuous variable, the count unit was converted to x108/mmol during logistic regression, then included in the regression model. The optimum value for identifying PAD risk in this sample was calculated using ROC curve analysis. The optimal cut-off value was determined by maximizing the Yoden index. A bilateral P< 0.05 was defined as statistically significant.
Results
Comparison of baseline clinical characteristics and laboratory indicators between the PAD group and WPAD group
The demographic and clinical features of T2DM-PAD group and T2DM-WPAD group are summarized in Table 2. Among the 434 diabetic patients enrolled, 218 had PAD, and 216 did not (WPAD). Compared to WPAD patients, PAD patients had a higher prevalence of coronary artery disease (CAD) and hypertension, and showed significantly increased levels of systolic blood pressure (SBP), HDL, low-density lipoprotein (LDL), C-reactive protein (CRP), neutrophils, lymphocytes, monocytes, NHR, MHR, PHR, SII, SIRI, and AISI(P<0.05). The two groups did not differ for gender, history of smoking, drinking, and dyslipidemia, diastolic blood pressure (DBP), fasting glucose, TyG index, HbA1c, TG, total cholesterol (TC), platelets, and LHR (P>0.05). The prevalence of Fontaine stage II, and CLI were 62.8, 12.9, and 11.5% in PAD patients, respectively.
Clinical and laboratory features of T2DM - PAD patients: subgroup analysis using the fontaine classification
The two groups did not differ regarding gender, age, duration of diabetes, history of CAD, hypertension, and dyslipidemia, SBP, DBP and laboratory parameters such as lymphocytes, monocytes, platelets, and LHR (P>0.05) (Table 3). As disease severity increased, fasting glucose, HbA1c, TG, TC, HDL-C, LDL-C, and TyG index presented a decreasing trend (P<0.05), but CRP, neutrophils, monocytes, NHR, MHR, PHR, SII, SIRI, and AISI showed an increasing trend (P<0.05). Fontaine stage II patients had higher percentage of smokers (44.5%) and alcoholics (40.1%) (P<0.05).
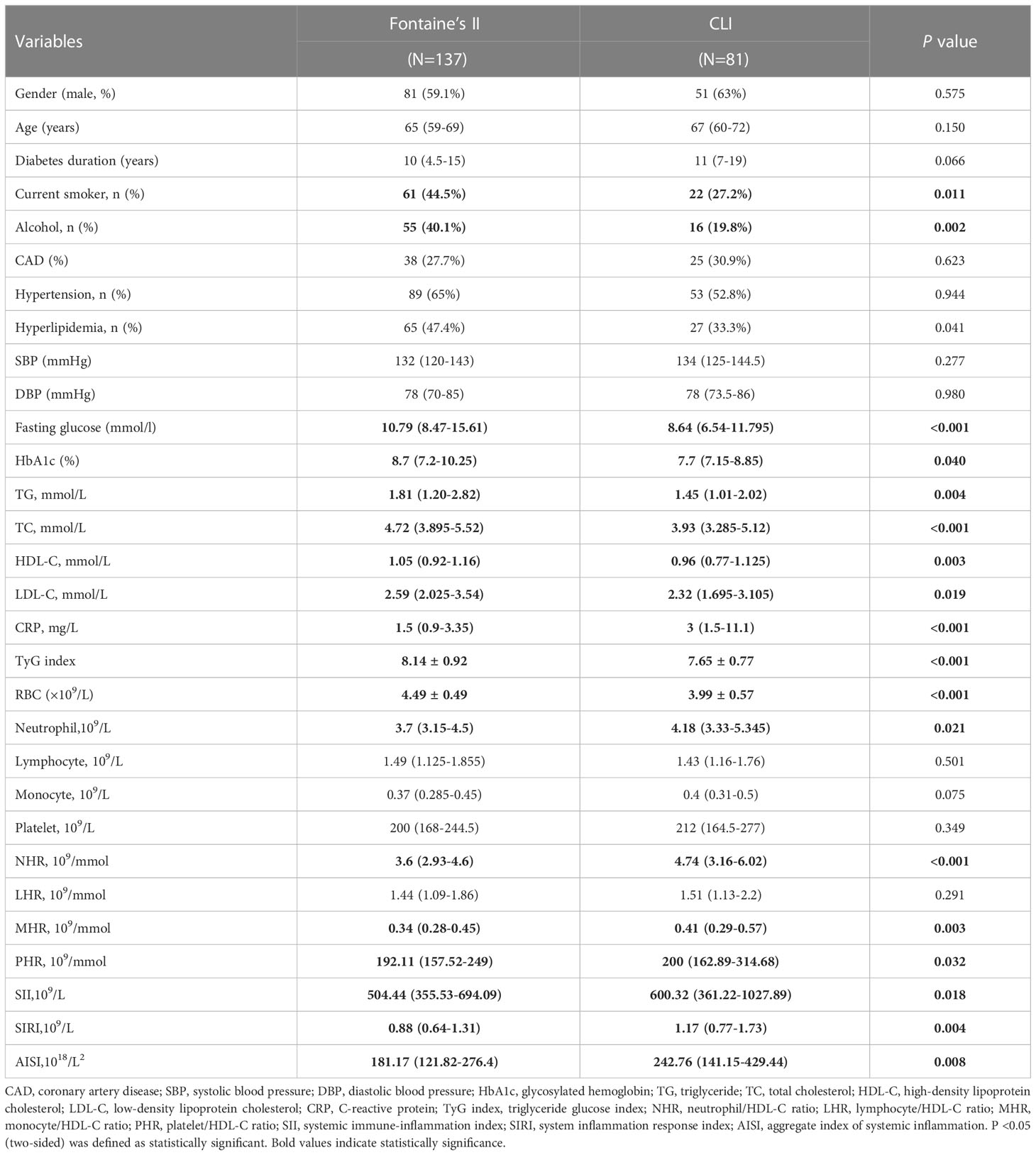
Table 3 Subgroup analysis of the clinical characteristics based on the Fontaine classification in patients with PAD.
The box - plot in Figure 1 indicated that CLI patients had higher levels of NHR, MHR, PHR, SII, SIRI, and AISI, and that all these indices showed an increasing relationship with disease extent.
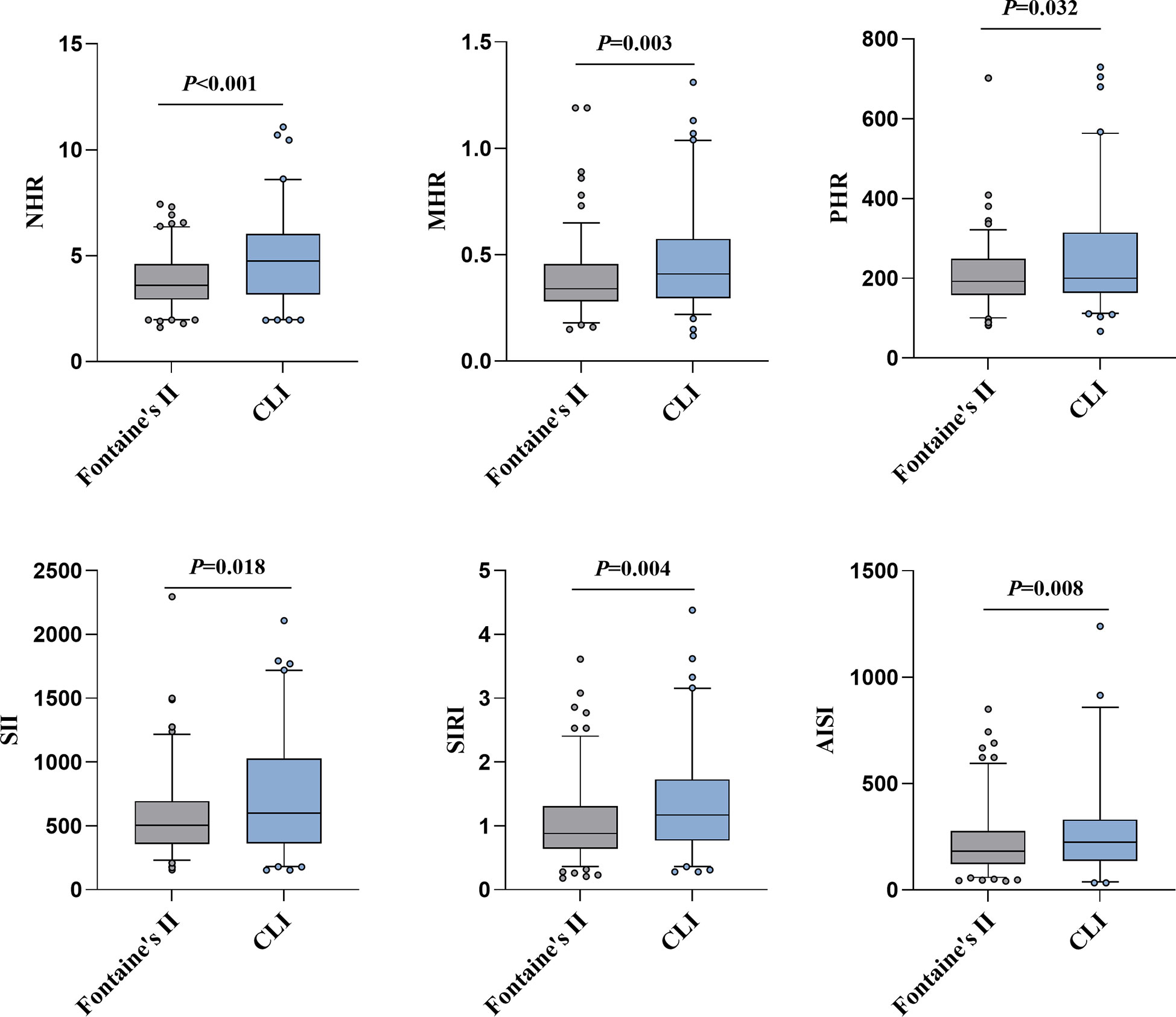
Figure 1 The NHR, MHR, PHR, SII, SIRI and AISI levels according to PAD severity. On the box plots, central lines represent the median, the length of the box represents the interquartile range and the lines extend to minimum and maximum values. Bold values indicate statistically significance.
Correlation of NHR, MHR, PHR, SII, SIRI, and AISI with other indicators of T2DM-PAD patients
Correlations between NHR, MHR, PHR, SII, SIRI, AISI and other indicators in PAD patients were assessed using Spearman correlation analysis. The NHR, MHR, PHR, SII, SIRI, and AISI were significantly correlated with Fontaine grading and CRP (P<0.05) (Table 4). However, these parameters had no significantly correlation with age, history of hypertension or dyslipidemia, SBP, DBP, fasting glucose, and TyG index. The PHR (r=-0.139) revealed a statistically weak connection with disease duration, whereas the NHR, MHR, SII, SIRI, and AISI were unrelated to disease duration. Additionally, a significant positive correlation was found between NHR and lymphocytes (r=0.151), monocytes (r=0.498), platelets (r=0.347), and TC (r=-0.207) (all P<0.05). The MHR was significantly linked with gender (r=-0.192), history of alcohol consumption (r=0.138), TC (r=-0.305), LDL-C (r=-0.192), neutrophils (r=0.455), lymphocytes (r=0.280), and platelets (r=0.204) (all P<0.05). The PHR was significantly associated with history of smoking (r=-0.136), alcohol consumption (r=-0.179) and CAD (r=-0.271), HbA1c (r=0.143), TG (r=0.169), TC (r=-0.133), neutrophils (r=0.270), and monocytes (r=0.182) (all P<0.05). The SII was significantly associated with monocytes (r=0.205) and the SIRI was significantly correlated with gender (r=-0.238) smoking history (r=0.167), TG (r=-0.141), and platelets (r=0.246) (all P<0.05).
Univariate and multivariate logistic regression analysis of the influencing factors for T2DM-PAD occurrence
The univariate logistic regression analysis showed that age, duration of diabetes, SBP, LDL-C, CRP, NHR, MHR, PHR, SII, SIRI, and AISI were independently associated with PAD occurrence in T2DM patients (Table 5). After excluding the effects of confounding factors for binary logistic regression, age, duration of diabetes, NHR, MHR, PHR, SII, SIRI, and AISI were still statistically significant and considered independent risk factors for PAD occurrence in T2DM patients.
Diagnostic performance of different inflammatory indexes for T2DM-PAD
The ROC curve analysis was used to evaluate the ability of NHR, MHR, PHR, SII, SIRI, and AISI to identify T2DM-PAD patients. The results of the ROC curve analysis showed that each of these indicators exhibited a high discriminating value for T2DM-PAD. Both the SIRI (AUC 0.711, 95% CI: 0.663-0.760, P = 0.000, cut-off 0.95) and the NHR (AUC 0.703, 95% CI:0.655-0.751, P = 0.000, cut-off 3.44) had an AUC greater than 0.7 in the ROC analysis of T2DM-PAD. Additionally, the combination model of SIRI and NHR had an AUC of 0.733 (95% CI:0.686-0.779, P = 0.000, cut-off 0.57). Besides, the other indexes with AUCs greater than 0.6 were the MHR (AUC 0.685, 95% CI:0.636–0.735, P = 0.000, cut-off 0.33), AISI (AUC 0.670, 95% CI:0.619–0.721, P = 0.000, cut-off 218.94), SII (AUC 0.648, 95% CI:0.596–0.700, P = 0.000, cut-off 486.96) and PHR (AUC 0.606, 95% CI:0.553–0.658, P = 0.000, cut-off 166.47). The data are presented in Figure 2.
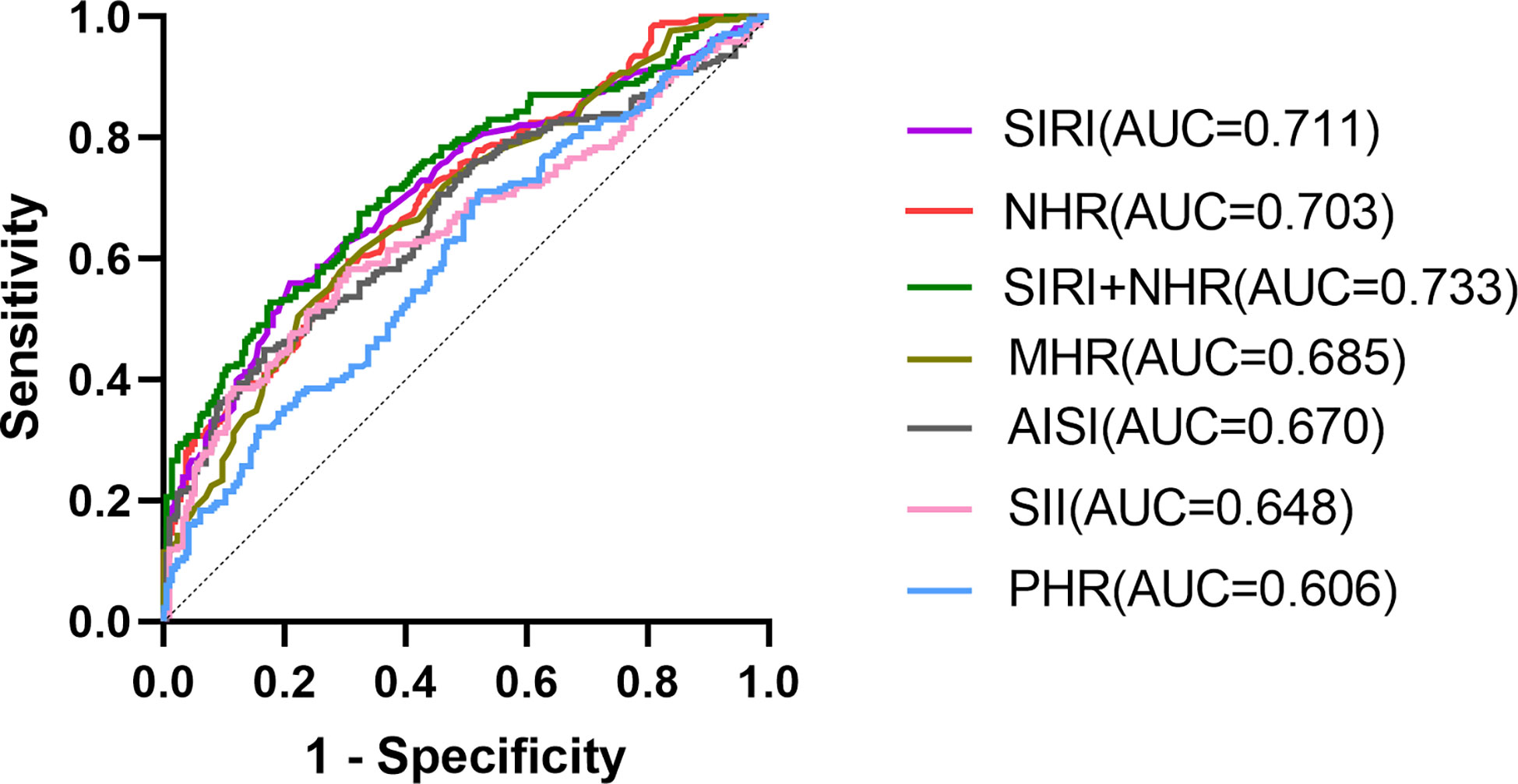
Figure 2 ROC curve analysis of the ability of these biomarkers to predict T2DM – PAD. SIRI (AUC 0.711, 95% CI:0.663–0.760, P = 0.000, cut-off 0.95, sensitivity 56%, specificity 79.2%); NHR (AUC 0.703, 95% CI:0.655–0.751, P = 0.000, cut-off 3.44, sensitivity 59.6%, specificity 69.4%); SIRI+NHR combined model(AUC 0.733, 95% CI:0.686-0.779, P = 0.000, cut-off 0.57, sensitivity 52.8%, specificity 82.4%); MHR(AUC 0.685, 95% CI:0.636–0.735, P = 0.000, cut-off 0.33, sensitivity 61.0%, specificity 70.4%);AISI(AUC 0.670, 95% CI:0.619–0.721, P = 0.000, cut-off 218.94, sensitivity45%, specificity83.3%);SII(AUC 0.648, 95% CI:0.596–0.700, P = 0.000, cut-off 486.96, sensitivity58.3%, specificity 69.4%);PHR (AUC 0.606, 95% CI:0.553–0.658, P = 0.000, cut-off 166.47, sensitivity71.1%, specificity 47.7%).
Discussion
Recently, it has been demonstrated that NHR, MHR, LHR, PHR, SII, SIRI, and AISI are novel inflammatory biomarkers and have significant clinical value due to easy access. Nevertheless, no evidence exists on the relationship between these inflammatory parameters and T2DM - PAD. To our knowledge, there is no published research on the association between SIRI, AISI, and diabetic patients. In this retrospective cross-sectional study, the association was first explored between seven novel serological indicators and T2DM-PAD patients. This study illustrated that NHR, MHR, PHR, SII, SIRI, and AISI were strongly associated with increased PAD prevalence in T2DM patients, and that all these indices were associated with disease severity. Additionally, the ROC curve analysis showed that NHR, SIRI, and their combination might predict the T2DM-PAD occurrence more effectively than other indexes.
The daily life of patients is often severely affected by PAD, imposing a substantial medical expense on individuals and society. Therefore, effective early screening and identification of T2DM - PAD patients is crucial (30). Evidence suggests that diabetes is one of the strongest risk factors for PAD development (31). Hence, only diabetic patients were chosen to be investigated in this study. Several potential biomarkers have been identified for PAD in diabetic patients, including HMGB 1, OPG, FGF 23, Omentin-1, Cyr61, and Sortilin (32–36). However, these biomarkers require complex and costly measurements and are not widely used in the clinic. The new inflammatory indicators presented in this work can be easily obtained using standard laboratory indices and may have substantial clinical use.
It is generally agreed that atherosclerosis is a chronic inflammatory disease of the arterial wall that stems from an insufficient inflammatory response and an imbalanced lipid metabolism. The role of inflammation and lipid metabolism in T2DM and PAD pathogenesis is of great interest (37, 38). Monocytes initiate and promote atherosclerosis progression by releasing pro-inflammatory cytokines, reactive oxygen species, and protein hydrolases (39). Neutrophils, the most abundant leukocyte subtype, exacerbate vessel wall inflammation via apoptosis of small muscle cells (40, 41). In contrast, lymphocytes can impair atherosclerosis progression (36). Platelets have a dual role in atherosclerosis: their adherence to the vascular wall promotes plaque formation (42), whereas their activation promotes inflammation and thrombosis (43). Elevated LDL and reduced HDL levels are key factors in atherosclerosis development and progression (44). The current results showed that PAD patients had significantly higher neutrophils, monocytes, and CRP and significantly fewer lymphocytes and HDL than WPAD patients, as well as a significant reduction in LDL, which could be hypothesized might be related to statin use. However, statin use was not an exclusion criterion, and the supplementary tables showed that the two groups of patients using statins had inflammatory indicators that were not significantly different (See Supplementary Tables 1, 2).
The MHR, NHR, PHR, and LHR have been investigated as novel inflammatory markers derived from peripheral blood cells and HDL-C in many systemic chronic inflammatory diseases. The SII, SIRI, and AISI are novel chronic low-grade inflammatory markers based on peripheral blood cells and platelets. The current results showed that NHR, MHR, PHR, SII, SIRI, and AISI were significantly higher in T2DM-PAD patients in WPAD patients and that they were significantly correlated with disease severity based on the Fontaine classification. Based on the ultrasound results of the lower limbs, we evaluated the degree of PAD disease and then categorized the patients with T2DM - PAD into three subgroups: mild, moderate, and severe. Individuals with severe PAD had higher concentrations of NHR, MHR, PHR, SII, SIRI, and AISI than those with mild PAD. Significant incremental increases were not reflected in the three sub-periods (See Supplementary Figure 1). These results were consistent with L. Santoro et al. that MHR was not associated with ultrasound grading in patients with only PAD (24), suggesting that these inflammatory indicators might be more appropriate for clinical application in combination with the Fontaine classification to assess disease severity. Spearman analysis showed that all indicators were positively associated with the Fontaine classification and CRP. Meanwhile, NHR, MHR, SII, SIRI, and AISI were not correlated with the patients’ age and disease duration, and PHR was weakly negatively correlated with the duration of diabetes. Although high glucose and insulin resistance enhance vascular inflammation (45), the data showed no significant correlation between these inflammatory markers and fasting glucose in T2DM-PAD patients or the total population, which might be due to sample size limits. The TyG index, a surrogate for insulin resistance, is significantly related to the gold standard hyperinsulinemic-orthoglycemic clamp (46) and can be a reliable assessment of insulin resistance in patients. Unfortunately, no correlation between TyG index and these indices was observed in the T2DM-PAD population. But in the total population, NHR (r=0.115), MHR (r=0.095), PHR (r=0.156) were found to be significantly correlated with TyG index (all P<0.05) (See Supplementary Table 3). Further large cohort studies are needed to analyze the relationship of these indicators with glycemic and insulin resistance. The univariate logistic regression showed that age, duration of diabetes, history of hypertension, SBP, CRP, NHR, MHR, PHR, SII, SIRI, and AISI were statistically significant. The multifactorial regression, excluding the effects of confounding factors, showed that NHR, MHR, PHR, SII, SIRI, and AISI were independently associated with T2DM-PAD. This study also demonstrated that age and duration of diabetes might be independent risk factors, consistent with previous studies (30). Combined with the Spearman correlation results, it was hypothesized that disease prediction by NHR, MHR, SII, SIRI, and AISI was not affected by age and duration of diabetes, increasing their clinical adjunctive value, but this hypothesis might require prospective cohorts to verify reliability. The ROC curve analysis showed that NHR, MHR, PHR, SII, SIRI, and AISI could predict T2DM-PAD well. The AUCs of MHR, AISI, SII, and PHR were over 0.6, and NHR and SIRI were over 0.7. The highest AUC (0.733) was detected when the NHR and SIRI were combined, indicating that it was better to use their combination for disease prediction in the clinic. When the combined model of NHR and SIRI value was greater than 0.57, it might suggest that the patient had a higher risk of developing PAD. This finding would serve as an easily available diagnostic aid for clinicians.
However, this current study also has some limitations. First, this was a retrospective cross-sectional study conducted in a single center, unable to determine the causal relationship between disease and indicators. Although these indices showed a good correlation with PAD, further studies are necessary to consider them as independent risk factors for the disease. Second, this research did not exclude patients on statins because neither group had significant differences in emerging inflammatory indicators on statins. Third, all patients’ body mass index (BMI) data were not collected completely and BMI was not included in the analysis, so the possible effect of BMI as a confounding factor may had been overlooked. Fourth, the novel inflammatory indicators were not dynamically monitored. Therefore, whether their changes are related to PAD progression remains unknown. Further prospective studies are required to analyze whether the above indicators reduce atherosclerosis progression. SIRI effectively predicts MACE in patients undergoing percutaneous coronary intervention after acute coronary syndrome (14), and this predictive performance exceeds the neutrophil-lymphocyte ratio (NLR) and monocyte-lymphocyte ratio (MLR) (47). Prospective studies are needed to see if these indices have a similar effect in predicting MACE and MALE for PAD.
Data availability statement
The raw data supporting the conclusions of this article will be made available by the authors, without undue reservation.
Ethics Statement
The study protocol was approved by the ethics committee of the Liyuan Hospital, Tongji Medical College, Huazhong University ofScience and Technology. (Approval IRBID: [2022] IEC CRYJ 0018). All data used in this study were anonymized and the requirement forinformed consent was waived.
Author contributions
SJ and YiS conceived the study plan and contributed to the revision of the final manuscript. YiS collected, analyzed the data and finished the manuscript writing. YZ, YaS and LZ participated in data collection and literature search. WC, LW, MS, BX, RW, ZF, YY and FY contributed to the manuscript writing and data interpretation. All authors contributed to the article and approved the submitted version.
Funding
This work was supported by the National Key & R&D Program of China to SJ (No. 2020YFC2008900) and the grants from the National Natural Science Foundation of China to SJ (No. 82070862).
Conflict of interest
The authors declare that the research was conducted in the absence of any commercial or financial relationships that could be construed as a potential conflict of interest.
The reviewer Y-MW declared a shared affiliation with the authors to the handling editor at the time of review.
Publisher’s note
All claims expressed in this article are solely those of the authors and do not necessarily represent those of their affiliated organizations, or those of the publisher, the editors and the reviewers. Any product that may be evaluated in this article, or claim that may be made by its manufacturer, is not guaranteed or endorsed by the publisher.
Supplementary material
The Supplementary Material for this article can be found online at: https://www.frontiersin.org/articles/10.3389/fendo.2023.1100453/full#supplementary-material
References
1. Jude EB, Oyibo SO, Chalmers N, Boulton AJM. Peripheral arterial disease in diabetic and nondiabetic patients. Diabetes Care (2001) 24(8):1433–7. doi: 10.2337/diacare.24.8.1433
2. Leibson CL, Ransom JE, Olson W, Zimmerman BR, O’Fallon WM, Palumbo PJ. Peripheral arterial disease, diabetes, and mortality. Diabetes Care (2004) 27(12):2843–9. doi: 10.2337/diacare.27.12.2843
3. Nativel M, Potier L, Alexandre L, Baillet-Blanco L, Ducasse E, Velho G, et al. Lower extremity arterial disease in patients with diabetes: A contemporary narrative review. Cardiovasc Diabetol (2018) 17(1):138. doi: 10.1186/s12933-018-0781-1
4. American Diabetes Association. Improving care and promoting health in populations: Standards of medical care in diabetes-2020. Diabetes Care (2020) 43(Suppl 1):S7–13. doi: 10.2337/dc20-S001
5. Low Wang CC, Hess CN, Hiatt WR, Goldfine AB. Clinical update: Cardiovascular disease in diabetes mellitus: Atherosclerotic cardiovascular disease and heart failure in type 2 diabetes mellitus - mechanisms, management, and clinical considerations. Circulation (2016) 133(24):2459–502. doi: 10.1161/CIRCULATIONAHA.116.022194
6. Martín-Timón I, Sevillano-Collantes C, Segura-Galindo A, Del Cañizo-Gómez FJ. Type 2 diabetes and cardiovascular disease: Have all risk factors the same strength? World J Diabetes (2014) 5(4):444–70. doi: 10.4239/wjd.v5.i4.444
7. Tanaka S, Couret D, Tran-Dinh A, Duranteau J, Montravers P, Schwendeman A, et al. High-density lipoproteins during sepsis: From bench to bedside. Crit Care (2020) 24(1):134. doi: 10.1186/s13054-020-02860-3
8. Huang JB, Chen YS, Ji HY, Xie WM, Jiang J, Ran LS, et al. Neutrophil to high-density lipoprotein ratio has a superior prognostic value in elderly patients with acute myocardial infarction: A comparison study. Lipids Health Dis (2020) 19(1):59. doi: 10.1186/s12944-020-01238-2
9. Liu Z, Fan Q, Wu S, Wan Y, Lei Y. Compared with the monocyte to high-density lipoprotein ratio (MHR) and the neutrophil to lymphocyte ratio (NLR), the neutrophil to high-density lipoprotein ratio (NHR) is more valuable for assessing the inflammatory process in parkinson’s disease. Lipids Health Dis (2021) 20:35. doi: 10.1186/s12944-021-01462-4
10. Yu S, Guo X, Li G, Yang H, Zheng L, Sun Y. Lymphocyte to high-density lipoprotein ratio but not platelet to lymphocyte ratio effectively predicts metabolic syndrome among subjects from rural China. Front Cardiovasc Med (2021) 8:583320. doi: 10.3389/fcvm.2021.583320
11. Jialal I, Jialal G, Adams-Huet B. The platelet to high density lipoprotein -cholesterol ratio is a valid biomarker of nascent metabolic syndrome. Diabetes Metab Res Rev (2021) 37(6). doi: 10.1002/dmrr.3403
12. Geraghty JR, Lung TJ, Hirsch Y, Katz EA, Cheng T, Saini NS, et al. Systemic immune-inflammation index predicts delayed cerebral vasospasm after aneurysmal subarachnoid hemorrhage. Neurosurgery (2021) 89(6):1071–9. doi: 10.1093/neuros/nyab354
13. Wang P, Guo X, Zhou Y, Li Z, Yu S, Sun Y, et al. Monocyte-to-high-density lipoprotein ratio and systemic inflammation response index are associated with the risk of metabolic disorders and cardiovascular diseases in general rural population. Front Endocrinol (Lausanne) (2022) 13:944991. doi: 10.3389/fendo.2022.944991
14. Han K, Shi D, Yang L, Wang Z, Li Y, Gao F, et al. Prognostic value of systemic inflammatory response index in patients with acute coronary syndrome undergoing percutaneous coronary intervention. Ann Med (2022) 54(1):1667–77. doi: 10.1080/07853890.2022.2083671
15. Hamad DA, Aly MM, Abdelhameid MA, Ahmed SA, Shaltout AS, Abdel-Moniem AE, et al. Combined blood indexes of systemic inflammation as a mirror to admission to intensive care unit in COVID-19 patients: A multicentric study. J Epidemiol Glob Health (2022) 12(1):64–73. doi: 10.1007/s44197-021-00021-5
16. Wang Y, Zhang J, Li H, Kong W, Zheng J, Li Y, et al. Prognostic value of leucocyte to high-density lipoprotein-cholesterol ratios in COVID-19 patients and the diabetes subgroup. Front Endocrinol (Lausanne) (2021) 12:727419. doi: 10.3389/fendo.2021.727419
17. Yalinbas Yeter D, Eroglu S, Sariakcali B, Bozali E, Vural Ozec A, Erdogan H. The usefulness of monocyte-to-High density lipoprotein and neutrophil-to-Lymphocyte ratio in diabetic macular edema prediction and early anti-VEGF treatment response. Ocul Immunol Inflamm (2022) 30(4):901–6. doi: 10.1080/09273948.2020.1849739
18. Jia J, Liu R, Wei W, Yu F, Yu X, Shen Y, et al. Monocyte to high-density lipoprotein cholesterol ratio at the nexus of type 2 diabetes mellitus patients with metabolic-associated fatty liver disease. Front Physiol (2021) 12:762242. doi: 10.3389/fphys.2021.762242
19. Yu Q, Weng W, Luo H, Yan J, Zhao X. The novel predictive biomarkers for type 2 diabetes mellitus in active pulmonary tuberculosis patients. Infect Drug Resist (2022) 15:4529–39. doi: 10.2147/IDR.S377465
20. Chen JW, Li C, Liu ZH, Shen Y, Ding FH, Shu XY, et al. The role of monocyte to high-density lipoprotein cholesterol ratio in prediction of carotid intima-media thickness in patients with type 2 diabetes. Front Endocrinol (Lausanne) (2019) 10:191. doi: 10.3389/fendo.2019.00191
21. Wang W, Chen ZY, Guo XL, Tu M. Monocyte to high-density lipoprotein and apolipoprotein A1 ratios: Novel indicators for metabolic syndrome in Chinese newly diagnosed type 2 diabetes. Front Endocrinol (Lausanne) (2022) 13:935776. doi: 10.3389/fendo.2022.935776
22. Ceyhun G, Engin MÇ. The Monocyte/High density lipoprotein cholesterol ratio (MHR) as an indicator of the need for amputation in patients with peripheral artery disease developing critical limb ischemia. Angiology (2021) 72(3):268–73. doi: 10.1177/0003319720965808
23. Selvaggio S, Abate A, Brugaletta G, Musso C, Di Guardo M, Di Guardo C, et al. Platelet−to−lymphocyte ratio, neutrophil−to−lymphocyte ratio and monocyte−to−HDL cholesterol ratio as markers of peripheral artery disease in elderly patients. Int J Mol Med (2020) 46(3):1210–6. doi: 10.3892/ijmm.2020.4644
24. Santoro L, Ferraro PM, Nesci A, D’Alessandro A, Macerola N, Forni F, et al. Neutrophil-to-lymphocyte ratio but not monocyte-to-HDL cholesterol ratio nor platelet-to-lymphocyte ratio correlates with early stages of lower extremity arterial disease: An ultrasonographic study. Eur Rev Med Pharmacol Sci (2021) 25(9):3453–9. doi: 10.26355/eurrev_202105_25826
25. American Diabetes Association. Glycemic targets: Standards of medical care in diabetes–2021. Diabetes Care (2021) 44(Supplement_1):S73–84. doi: 10.2337/dc21-S006
26. Aboyans V, Ricco JB, Bartelink MLEL, Björck M, Brodmann M, Cohnert T, et al. 2017 ESC Guidelines on the diagnosis and treatment of peripheral arterial diseases, in collaboration with the European society for vascular surgery (ESVS): Document covering atherosclerotic disease of extracranial carotid and vertebral, mesenteric, renal, upper and lower extremity arteriesEndorsed by: The European stroke organization (ESO)The task force for the diagnosis and treatment of peripheral arterial diseases of the European society of cardiology (ESC) and of the European society for vascular surgery (ESVS). Eur Heart J (2018) 39(9):763–816. doi: 10.1093/eurheartj/ehx095
27. Gerhard-Herman MD, Gornik HL, Barrett C, Barshes NR, Corriere MA, Drachman DE, et al. 2016 AHA/ACC guideline on the management of patients with lower extremity peripheral artery disease: A report of the American college of Cardiology/American heart association task force on clinical practice guidelines. Circulation (2017) 135(12):e726–79. doi: 10.1161/CIR.0000000000000471
28. Hardman RL, Jazaeri O, Yi J, Smith M, Gupta R. Overview of classification systems in peripheral artery disease. Semin Intervent Radiol (2014) 31(4):378–88. doi: 10.1055/s-0034-1393976
29. Norgren L, Hiatt WR, Dormandy JA, Nehler MR, Harris KA, Fowkes FGR, et al. Inter-society consensus for the management of peripheral arterial disease (TASC II). J Vasc Surg (2007) 45 Suppl S:S5–67. doi: 10.1016/j.jvs.2006.12.037
30. American Diabetes Association. Peripheral arterial disease in people with diabetes. Diabetes Care (2003) 26(12):3333–41. doi: 10.2337/diacare.26.12.3333
31. Poredoš P, Šabovič M, Božič Mijovski M, Nikolajević J, Antignani PL, Paraskevas KI, et al. Inflammatory and prothrombotic biomarkers, DNA polymorphisms, MicroRNAs and personalized medicine for patients with peripheral arterial disease. Int J Mol Sci (2022) 23(19):12054. doi: 10.3390/ijms231912054
32. Giovannini S, Tinelli G, Biscetti F, Straface G, Angelini F, Pitocco D, et al. Serum high mobility group box-1 and osteoprotegerin levels are associated with peripheral arterial disease and critical limb ischemia in type 2 diabetic subjects. Cardiovasc Diabetol (2017) 16(1):99. doi: 10.1186/s12933-017-0581-z
33. He X, Hu X, Ma X, Su H, Ying L, Peng J, et al. Elevated serum fibroblast growth factor 23 levels as an indicator of lower extremity atherosclerotic disease in Chinese patients with type 2 diabetes mellitus. Cardiovasc Diabetol (2017) 16(1):77. doi: 10.1186/s12933-017-0559-x
34. Biscetti F, Nardella E, Bonadia N, Angelini F, Pitocco D, Santoliquido A, et al. Association between plasma omentin-1 levels in type 2 diabetic patients and peripheral artery disease. Cardiovasc Diabetol (2019) 18(1):74. doi: 10.1186/s12933-019-0880-7
35. Feng B, Xu G, Sun K, Duan K, Shi B, Zhang N. Association of serum Cyr61 levels with peripheral arterial disease in subjects with type 2 diabetes. Cardiovasc Diabetol (2020) 19(1):194. doi: 10.1186/s12933-020-01171-9
36. Biscetti F, Bonadia N, Santini F, Angelini F, Nardella E, Pitocco D, et al. Sortilin levels are associated with peripheral arterial disease in type 2 diabetic subjects. Cardiovasc Diabetol (2019) 18(1):5. doi: 10.1186/s12933-019-0805-5
37. Rosenson RS, Brewer HB, Ansell BJ, Barter P, Chapman MJ, Heinecke JW, et al. Dysfunctional HDL and atherosclerotic cardiovascular disease. Nat Rev Cardiol (2016) 13(1):48–60. doi: 10.1038/nrcardio.2015.124
38. Poznyak A, Grechko AV, Poggio P, Myasoedova VA, Alfieri V, Orekhov AN. The diabetes mellitus-atherosclerosis connection: The role of lipid and glucose metabolism and chronic inflammation. Int J Mol Sci (2020) 21(5):E1835. doi: 10.3390/ijms21051835
39. Gratchev A, Sobenin I, Orekhov A, Kzhyshkowska J. Monocytes as a diagnostic marker of cardiovascular diseases. Immunobiology (2012) 217(5):476–82. doi: 10.1016/j.imbio.2012.01.008
40. Shah AD, Denaxas S, Nicholas O, Hingorani AD, Hemingway H. Neutrophil counts and initial presentation of 12 cardiovascular diseases: A CALIBER cohort study. J Am Coll Cardiol (2017) 69(9):1160–9. doi: 10.1016/j.jacc.2016.12.022
41. Fernández-Ruiz I. Neutrophil-driven SMC death destabilizes atherosclerotic plaques. Nat Rev Cardiol (2019) 16(8):455. doi: 10.1038/s41569-019-0214-1
42. Massberg S, Brand K, Grüner S, Page S, Müller E, Müller I, et al. A critical role of platelet adhesion in the initiation of atherosclerotic lesion formation. J Exp Med (2002) 196(7):887–96. doi: 10.1084/jem.20012044
43. Gawaz M, Langer H, May AE. Platelets in inflammation and atherogenesis. J Clin Invest (2005) 115(12):3378–84. doi: 10.1172/JCI27196
44. Badimon L, Vilahur G. LDL-cholesterol versus HDL-cholesterol in the atherosclerotic plaque: Inflammatory resolution versus thrombotic chaos. Ann N Y Acad Sci (2012) 1254:18–32. doi: 10.1111/j.1749-6632.2012.06480.x
45. Dandona P, Aljada A, Bandyopadhyay A. Inflammation: The link between insulin resistance, obesity and diabetes. Trends Immunol (2004) 25(1):4–7. doi: 10.1016/j.it.2003.10.013
46. Zhao Q, Cheng YJ, Xu YK, Zhao ZW, Liu C, Sun TN, et al. Comparison of various insulin resistance surrogates on prognostic prediction and stratification following percutaneous coronary intervention in patients with and without type 2 diabetes mellitus. Cardiovasc Diabetol (2021) 20(1):190. doi: 10.1186/s12933-021-01383-7
Keywords: type 2 diabetes, peripheral artery disease, inflammation, lipid metabolism, biomarker
Citation: Song Y, Zhao Y, Shu Y, Zhang L, Cheng W, Wang L, Shu M, Xue B, Wang R, Feng Z, Yin Y, Yu F and Jin S (2023) Combination model of neutrophil to high-density lipoprotein ratio and system inflammation response index is more valuable for predicting peripheral arterial disease in type 2 diabetic patients: A cross-sectional study. Front. Endocrinol. 14:1100453. doi: 10.3389/fendo.2023.1100453
Received: 16 November 2022; Accepted: 01 February 2023;
Published: 16 February 2023.
Edited by:
Maurizio Delvecchio, Giovanni XXIII Children’s Hospital, ItalyReviewed by:
Federico Biscetti, Agostino Gemelli University Polyclinic (IRCCS), ItalyYu-Mei Wang, Huazhong University of Science and Technology, China
Yang Zhang, University of Houston, United States
Copyright © 2023 Song, Zhao, Shu, Zhang, Cheng, Wang, Shu, Xue, Wang, Feng, Yin, Yu and Jin. This is an open-access article distributed under the terms of the Creative Commons Attribution License (CC BY). The use, distribution or reproduction in other forums is permitted, provided the original author(s) and the copyright owner(s) are credited and that the original publication in this journal is cited, in accordance with accepted academic practice. No use, distribution or reproduction is permitted which does not comply with these terms.
*Correspondence: Si Jin, Jinsi@hust.edu.cn