- 1Department of Clinical Epidemiology and Biostatistics, Faculty of Medicine Ramathibodi Hospital, Mahidol University, Bangkok, Thailand
- 2Department of Pharmacy Administration, College of Pharmacy, Rangsit University, Pathum Thani, Thailand
- 3Division of Cardiovascular Medicine, Department of Medicine, Faculty of Medicine, HRH Princess Maha Chakri Sirindhorn Medical Center, Srinakharinwirot University, Nakhon Nayok, Thailand
- 4Centre for Public Health, School of Medicine, Dentistry and Biomedical Sciences, Queen’s University Belfast, Belfast, United Kingdom
- 5Centre for Clinical Epidemiology and Biostatistics, School of Medicine and Public Health, Faculty of Health and Medicine, University of Newcastle, and Hunter Medical Research Institute, New Lambton, NSW, Australia
Introduction: The cardiovascular benefits of multiple antihyperglycemic drugs as add-on therapies to metformin in the real-practice are unclear. This study aimed to directly compare major adverse cardiovascular events (CVE) associated with these multiple drugs.
Methods: An emulation of a target trial was conducted using a retrospective-cohort data of type 2 diabetes mellitus (T2DM) prescribed with second-line drugs on top of metformin, including sodium-glucose cotransporter 2 inhibitors (SGLT2i), dipeptidyl peptidase-4 inhibitors (DPP4i), thiazolidinediones (TZD) and sulfonylureas (SUs). We applied inverse probability weighting and regression adjustment using intention-to-treat (ITT), per-protocol analysis (PPA) and modified ITT. Average treatment effects (ATE) were estimated using SUs as the reference.
Results and Discussion: Among 25,498 patients with T2DM, 17,586 (69.0%), 3,261 (12.8%), 4,399 (17.3%), and 252 (1.0%) received SUs, TZD, DPP4i, and SGLT2i. Median follow-up time was 3.56 (1.36-7.00) years. CVE was identified in 963 patients. The ITT and modified ITT approaches showed similar results; the ATE (i.e., the difference of CVE risks) for SGLT2i, TZD, and DPP4i compared to SUs were -0.020(-0.040, -0.0002), -0.010(-0.017, -0.003), and -0.004(-0.010, 0.002), respectively, indicating 2% and 1% significant absolute risk reduction in CVE in SGLT2i and TZD compared to SUs. These corresponding effects were also significant in the PPA with ATEs of -0.045(-0.060, -0.031), -0.015(-0.026, -0.004), and -0.012(-0.020, -0.004). In addition, SGLT2i had 3.3% significant absolute risk reduction in CVE relative to DPP4i. Our study demonstrated benefits of SGLT2i and TZD in reducing CVE in T2DM patients compared to SUs when added to metformin.
Introduction
Cardiovascular disease (CVD) is a major cause of disability and mortality among people with diabetes (1), with a two to three-fold (2) higher risk in these patients. Studies in middle-aged individuals with type 2 diabetes mellitus (T2DM) living in high- and middle-income countries showed that 27 out of 1,000 individuals died prematurely from CVD each year (3) with coronary artery disease and stroke identified as major contributors (2).
Recent meta-analyses (4–6) of a newer antihyperglycemic drug class [i.e., sodium-glucose cotransporter 2 inhibitors (SGLT2i): empagliflozin (7), canagliflozin (8), and dapagliflozin (9)] have demonstrated benefits in lowering fatal and non-fatal CVD, all-cause mortality, hospitalization with heart failure (HF), and declining kidney function in patients with T2DM (4), irrespective of glycemic control and baseline metformin and/or statin use (10). However, the advantages of SGLT2i in lowering major adverse cardiac events (MACE) were apparent only in those with established atherosclerotic CVD (ASCVD), particularly for patients with HF with both reduced ejection fraction (HFrEF) (11) and preserved ejection fraction (HFpEF) (12, 13), but less evident in patients with T2DM at greater risk of ASCVD (4, 8, 9). The 2021 European Society of Cardiology (ESC) guidelines on CVD prevention (14) advise clinicians to consider the use of SGLT2i in patients with T2DM without ASCVD, HF/HFrEF, or chronic kidney disease (CKD), based on their estimated future CVD risk. Previous studies have confirmed the benefits of SGLT2i as second-line oral antihyperglycemic agents that significantly reduce MACE and hospitalization with HF/HFrEF when compared to the use of other second-line therapies, including dipeptidyl peptidase-4 inhibitors (DPP4i) (5, 6, 15, 16) and sulfonylureas (SUs) (17).
To our knowledge, no direct comparisons of cardiovascular (CV) outcomes have been undertaken for second-line antihyperglycemic drug classes, including SGLT2i, DPP4i, SUs and thiazolidinediones (TZD). Several cohorts (18, 19) based on real-world studies data have compared SGLT2i with DPP4i, but those were mainly undertaken in western countries. Therefore, we conducted a cohort study using real-world practice data from Thai patients with T2DM, to directly compare CV outcomes following second-line oral antihyperglycemic agents as add-on therapies to metformin.
Material and methods
A retrospective cohort study of T2DM was conducted at Ramathibodi Hospital, Bangkok, Thailand, from January 2010 to December 2019. Patients were included if they met the following criteria: adult patients aged 18 years or older diagnosed with T2DM from electronic databases by the International Statistical Classification of Diseases Ninth and Tenth Revisions (ICD-9 and ICD-10), and had prescription records of any of the following second-line antihyperglycemic drugs: SUs, TZD, DPP4i, or SGLT2i, added to metformin, see Figure 1. Participants with history of CVD events or reported CVD events within one month following the first prescription of a second-line treatment were excluded. The study was approved by the Institutional Review Board of the Faculty of Medicine, Ramathibodi Hospital, Mahidol University (COA. MURA2021/522).
Data were retrieved from electronic databases as follows: Baseline characteristics on receipt of second-line treatment included date, age, sex, body mass index, glycemic parameters (i.e., fasting blood glucose (FPG) and glycated hemoglobin (HbA1c)), renal function (i.e., estimated glomerular filtration rate (eGFR) based on the 2009 CKD-EPI creatinine equation), lipid profile (i.e., low-density lipoprotein cholesterol (LDL-C), high-density lipoprotein cholesterol (HDL-C), and triglycerides), underlying diseases (i.e., hypertension (HT), dyslipidemia (DLP), and CKD), and use of statin medication. Electronic medical records, laboratory data, diagnosis, and medication databases at baseline and follow up were retrieved. These data were then linked and merged to form a dynamic cohort dataset.
Treatments and outcomes
Treatments of interest included SGLT2i, DPP4i, TZD, and SUs, which were identified from medication databases. All prescription data during follow up were retrieved including numbers of medication, daily dose/frequency, time to follow up, and time to ascertain medication use during the study period. The primary outcome of interest was time to CVD occurrence, which was identified by the ICD-10 (I20) codes including acute coronary syndrome, ischemic cardiomyopathy, chronic ischemic heart disease, HF/HFrEF, and cerebrovascular disease. The index date was the initial date for receipt of the second-line drug and the end-date was date at CVD occurrence or date of final visit if lost to follow up, or study end date if still CVD-free by December 31st 2019. We emulated a target trial (20) using three analytical approaches: First, an intention-to-treat (ITT) approach considered the initial second-line drug regardless of whether treatment changed over time. Second, a per-protocol analysis (PPA) considered only patients who received a second-line treatment for the entire duration of the study period and did not switch treatments. Third, a modified ITT analysis censored patients at the time they switched treatments before they developed CVD.
Statistical analysis
Frequency (percentage) and mean (SD) or median (range) were used to describe categorical and continuous variables, respectively. The data were compared between second-line drugs using Chi-squared or analysis of variance tests.
We applied treatment effect models with inverse probability weighting and regression adjustment (IPWRA) to estimate associations between each second-line treatment and CVD as follows:
First, the treatment model (TM) was constructed using a multinomial logit model by fitting treatment assignments to variables that were associated with treatment allocations (i.e., age, sex, BMI, FPG, HbA1c, HT, DLP, CKD, LDL-C, HDL-C, triglycerides, and statin use). Only significant variables were retained in the final TM, and a propensity score was estimated accordingly.
Second, the outcome model (OM) was constructed using a logit equation with an inverse probability weighting of treatment allocation (i.e., a propensity score) estimated from the TM. Significant variables associated with CVD were retained in the OM.
Third, the risk of CVD development in each second-line treatment group was estimated as a potential outcome mean (POM). An average treatment effect (ATE) along with 95% confidence intervals (CI) representing the difference between POMs, analogous to a risk difference, was estimated using SUs as the reference.
Finally, the TM model assumptions were assessed as follows: first, standardized mean differences [i.e., the difference of the mean covariates between two treatment groups divided by the standard deviation (21)] and the variance ratio [i.e., the ratio of two covariate variances (22)] were estimated. Then, these were weighted by inverse propensity score estimated from the TM. A TM was valid if the conditional independent assumption was achieved, i.e., weighted standardized mean differences for all covariates were less than 0.2 and the weighted variance ratios were close to 1, indicating that covariates were well balanced across treatment groups after weighting by inverse propensity score. Second, treatment overlap, or positive probability of receiving treatments, was assessed by plotting density of the probabilities for each second-line drug.
All analyses were performed using STATA version 17.0. (Stata Corp., College Station, TX, USA). A P-value < 0.05 was considered statistically significant.
Results
A total of 71,663 patients with T2DM were identified, see Figure 1. Of these, 40,529 were excluded due to having only a single visit, receiving metformin monotherapy only or metformin with another treatment not considered of interest, or receiving triple combination therapy. In addition, a further 5,636 patients were excluded due to a recorded CVD event before receipt of a second-line treatment, or within one month of its prescription. Finally, 25,498 patients were included in the analysis which consisted of 17,586 (69.0%), 3,261 (12.8%), 4,399 (17.3%), and 252 (1.0%) who were in receipt of SUs, TZD, DPP4i, and SGLT2i, respectively.
Baseline characteristics
Patient baseline characteristics are described in Table 1. The mean age and percentage female for each treatment group ranged from 57.1-64.9 years and 56.3%-59.6%, respectively. Before weighting by the inverse propensity score, many participant baseline characteristics included within the ITT approach were not balanced across treatment groups. For example, patients receiving SUs had poorer glycemic control and were more likely to be dyslipidemic. The majority of patients in receipt of DPP4i were older, hypertensive, with lower baseline eGFR, while those in receipt of SGLT2i were more likely to be obese.
The baseline characteristics of patients included in the PPA approach were similar to those included in the ITT analysis. However, there were significant differences between patients included and excluded in the PPA analysis, see Supplementary Table 1. Generally, those included tended to be older, had better FPG control, but poorer eGFR and were more likely to be hypertensive.
Treatment effect model assumptions
Standardized mean differences and the variance ratios before and after weighting by the inverse propensity score are shown as raw and weighted values in Table 2. The absolute raw standardized mean differences ranged from 0.0020 to 0.3048, 0.0219 to 0.6727, and 0.0220 to 0.2742 for DPP4i vs. SUs, SGLT2i vs. SUs, and TZD vs. SUs, respectively. After weighting by inverse propensity score, the absolute weighted standardized mean differences of these corresponding comparisons were closer to zero, i.e., ranging from 0.0024 to 0.0275, 0.0172 to 0.1099, and 0.0036 to 0.0432, and the weighted variance ratios were close to one, indicating that covariates were well balanced. The balance plots and the density plots of the probabilities to receive each second-line drug also overlapped, see Supplementary Figures 1, 2. These suggested that the TM model assumptions hold, and the confounding variables across treatment groups were balanced successfully, providing a more correctly specified TM.
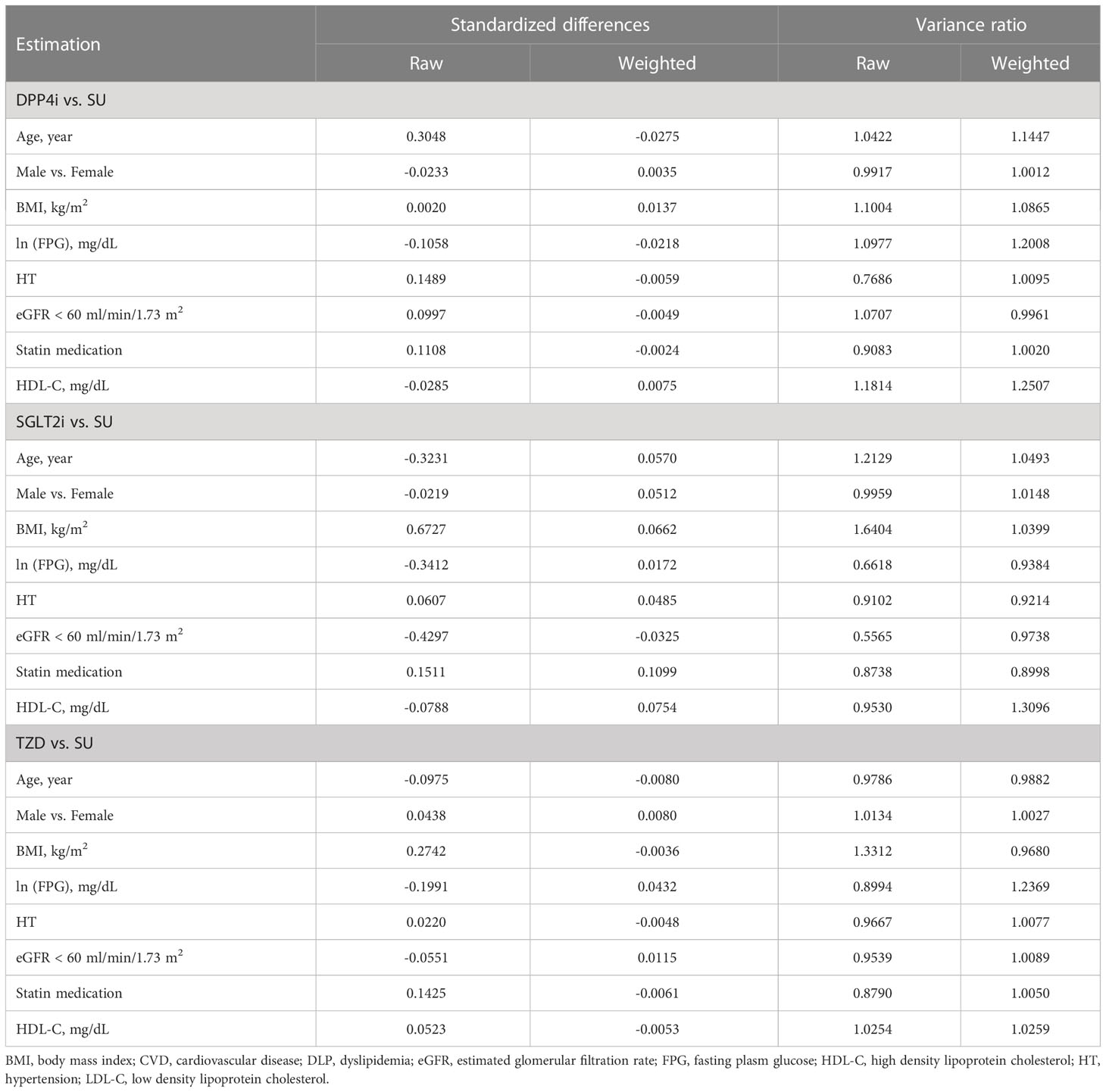
Table 2 Estimations of Standardized mean difference estimates for between treatment group factors before and after weighting by propensity score.
CVD events, TM, and OM
The median follow-up time was 3.56 (IQR: 1.36-7.00) years. Of the 25,498 patients, 963 had a recorded CVD event representing an incidence (95% CI) of 3.8% (3.5%, 4.0%). Most of CVD events were ischemic heart disease (64.4%) followed by cerebrovascular disease (17.6%), see Supplementary Table 2. The CVD incidence (95% CI) for patients taking SGLT2i, TZD, SUs, and DPP4i was 2.4% (0.9%, 5.1%), 2.9% (2.3%, 3.5%), 3.9% (3.6%, 4.2%), and 4.0% (3.5%, 4.6%), respectively. Factors significantly associated with treatment assignment and retained in the final TM to estimate the propensity score included age, sex, BMI, FPG, HT, eGFR, HDL-C, and statin use, see Supplementary Table 3. Similarly, factors significantly associated with CVD development in the OM were age, sex, BMI, HT, eGFR, HDL-C, and statin use (data not shown). Both TM and OM were considered in the IPWRA doubly robust approach, and the results are shown in Tables 3, 4. For the ITT approach, the POMs (95% CI) in the SGLT2i, TZD, DPP4i, and SUs groups were 0.020 (0.001, 0.040), 0.030 (0.024, 0.037), 0.036 (0.031, 0.041), and 0.040 (0.037, 0.043), respectively, indicating the risk of CVD development of 2%, 3%, 3.6%, and 4% in the corresponding treatment groups (Table 3). The ATEs representing the risk difference in the POMs between SGLT2i, TZD, DPP4i vs. SUs were -0.020 (-0.040, -0.0002), -0.010 (-0.017, -0.003), and -0.004 (-0.010, 0.002), respectively, see values above the diagonal line in Table 4, indicating 2% and 1% absolute CVD risk reductions associated with SGLT2i and TZD, when compared to the more commonly prescribed SU group. The comparison of the ATE between SGLT2i and TZD was not significantly different [ATE of -0.010 (-0.031, 0.010)]. In addition, relative risk (RR) along with 95% CIs were estimated by dividing POM of each treatment by POM of a reference treatment, see values underneath the diagonal line in Table 4. The RRs compared to SUs were 0.50 (0.01, 0.99), 0.76 (0.59, 0.93), and 0.89 (0.74, 1.04) for those receiving SGLT2i, TZD, and DPP4i, respectively, indicating that a relative risk reduction for CVD was 50% and 24% in patients who received SGLT2i and TZD, respectively, when compared to SUs. The RR of SGLT2i relative to DPP4i was 0.56 (0.01, 1.12), but this was not significant.
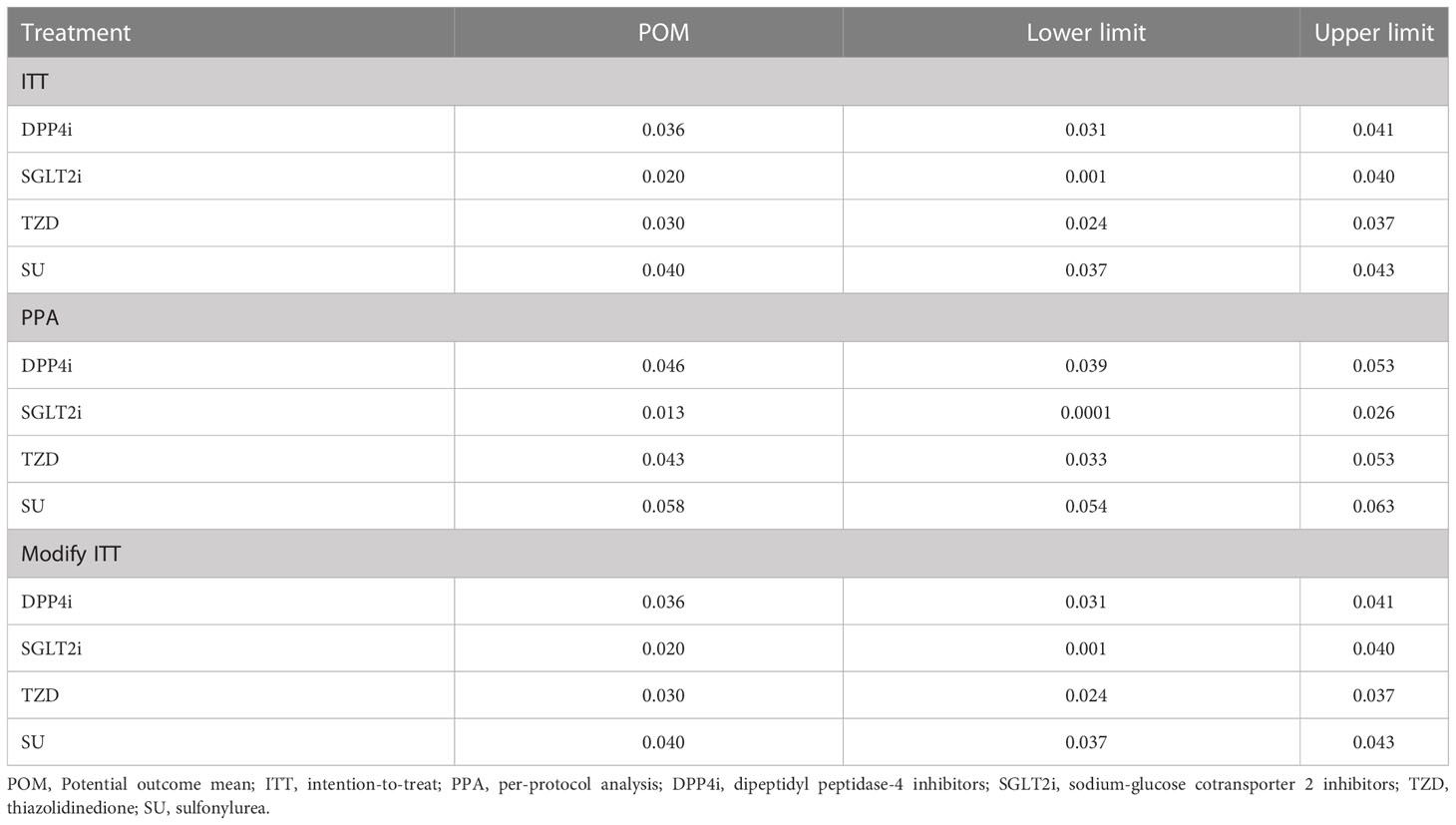
Table 3 Estimation of Potential outcome mean estimates between second-line drugs: Treatment effect model with inverse probability weighting and regression adjustment.
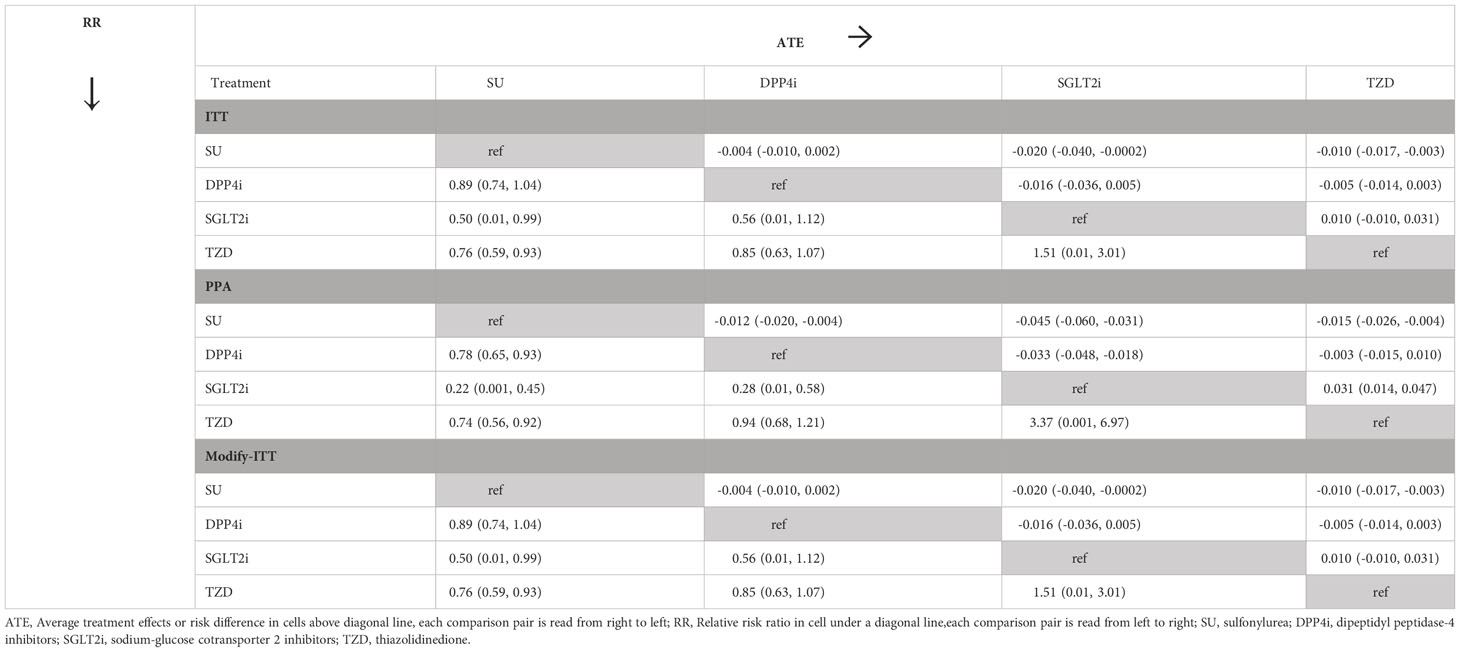
Table 4 Relative treatment effect estimates between second-line drugs: Treatment effect model with inverse probability weighting and regression adjustment.
For the PPA approach, the POMs in the SGLT2i, TZD, DPP4i, and SUs groups were 0.013 (0.0001, 0.026)), 0.043 (0.033, 0.053), 0.046 (0.039, 0.053), and 0.058 (0.054, 0.063), respectively. SGLT2i, TZD, and DPP4i were significantly associated with a 4.5%, 1.5%, and 1.2% reduced CVD risk (representing ATEs of -0.045 (-0.060, -0.031), -0.015 (-0.026, -0.004), and -0.012 (-0.020, -0.004), respectively) when compared to SUs. In addition, SGLT2i were significantly associated with a 3.3% lower CVD risk when compared to DPP4i (ATE: -0.033 (-0.048, -0.018)). The results from the modified ITT were similar to those from the ITT because none of patients switched their second-line treatments before CVD development.
Relative risks (RR) with 95% CI converted from the ATEs are also shown in Table 4. Those receiving SGLT2i, TZD, and DPP4i had 0.50 (0.01, 0.99), 0.76 (0.59, 0.93), and 0.89 (0.74, 1.04) times lower CVD risk than SUs but only SGLT2i and TZD were significant. In addition, the SGLT2i group had 0.56 (0.01, 1.12) times lower CVD risk than DPP4, but this was not significant.
Discussion
To our knowledge, this is the first emulated target trial based on real-world practice data that directly compared CV outcomes associated with second-line antihyperglycemic medications to the low-cost SU drug class, when added to metformin therapy in a Southeast Asian cohort. During the 42-month follow-up period, CVD risk associated with both SGLT2i and TZD treatment options were significantly reduced relative to the SUs drug class across all three approaches. These added benefits were also significant with the DPP4i group when evaluated using the PPA approach.
The findings of this study support other real-world trials (15, 23) highlighting the potential benefit of lower CVD risk associated with SGLT2i when prescribed following metformin therapy. However, no studies have emulated a target trial by directly investigating the effects of second-line drugs and comparing the outcomes to the more commonly prescribed SUs using analytical approaches that imitate an RCT. Notably, TZDs (mainly pioglitazone) have shown desirable effects in reducing CVD risk, when added to metformin (24). Moreover, the lack of a significant reduction in CVD risk associated with DPP4i in the ITT approach may have resulted from changing medication in the follow-up period and similarly, for SGLT2i compared to DPP4i in the PPA approach (Table 4), a comparison which has not been previously described.
The effects of SGLT2i are less powerful on the prevention of ASCVD when compared with the prevention of HF and renal outcomes. The possible mechanisms of action are its ameliorating effects on atherogenesis and established CV risk factors, i.e., weight reduction associated with glucosuria (25), blood pressure lowering by natriuresis and a diuretic effect (26), reduction of albuminuria, inflammation and oxidative markers (27), improving vascular compliance, and endothelial function by attenuating endothelial cell activation, inducing direct vasorelaxation, and reducing endothelial cell dysfunction (28, 29). In addition, the CV benefits, particularly HF and CV-related mortality, may arise as a consequence of renal effects and reduced intraglomerular pressure that leads to renal protection, improved renal function and/or reduced renal stress; this may indirectly improve cardiac function through various pathways, including reduced reactive oxygen species generation, afferent sympathetic nervous system activation, and inflammation (30). Moreover, SGLT2i can improve mitochondrial respiratory function and cardiac energy metabolism in the failing heart (31) when mitochondrial glucose oxidation and energy production is diminished. Extrapolating to the population level, we previously found that SGLT2i could directly lower CKD risk about 14% (32), and in this study, they could lower CVD risk of 2% relative to SUs with a number needed to treat of 140 and 20 per 1000 treated patients, i.e., for each 100 patients treated, we would prevent 14 CKD events and 2 CVD events over ~3.5 years of follow. Our current data also suggested that CKD patients had about 1.71 higher odds of CVD occurrence compared to those free from CKD. Based on mediation analysis (33), an indirect effect of SGLT2i on CVD risk through lowering CKD can be estimated by the product method [i.e., exponential [(-0.14) x ln(1.71)], and indicates a 0.92 odds of reduced CVD risk from lowering CKD, see Supplementary Figure 3.
The beneficial effects of CVD risk reduction associated with pioglitazone was previously investigated in patients with insulin resistance, pre-diabetes and T2DM (34). The underlying mechanism may be due to delayed atheroma progression by reducing the ratio of triglyceride/high-density lipoprotein-cholesterol (35) and increasing cholesterol efflux capacity (36) which has been reported to be inversely associated with the incidence of CV events in a population-based cohort (37). Similarly, apart from the glucose-lowering effect, DPP4i may also exert a positive influence on CVD and CV risk factors given its reported modest beneficial effects on postprandial lipemia, body weight, blood pressure, inflammatory markers, oxidative stress, and endothelial function in patients with T2DM (38).
DPP4i influence enzyme expression through the cell surface receptors that primarily effect the heart, and are mainly mediated by the products of stromal cell-derived factor-1, a stem cell chemokine that promotes inflammation, regeneration and repair (39). It can cause significant deleterious effects to cardiomyocytes in states of diabetes and other cardiac stress (40). However, DPP4i offer positive inotropic effects by enhancing the actions of glucagon like peptide-1 to stimulate cyclic AMP in cardiomyocytes. Previous pooled analyses demonstrated that DPP4i do not increase the risk of HF in patients with T2DM and a previous history of HF, but should be used with caution especially in patients with established ASCVD and no history of HF (41).
In our setting, SUs were commonly used as second-line drugs, a treatment modality similar to other countries in resource limited settings where more recent second-line medications are more expensive, unavailable or not reimbursable in the Universal Health Coverage in Thailand. Considering the CV effects associated with SUs, recent evidence suggests no difference in MACE outcomes in T2DM patients at high CV risk, relative to modern agents such as gliclazide MR and glimepiride (42–44). International and national guidelines still recommend using SUs (with the exception of glibenclamide), an alpha-glucosidase inhibitor or a DPP4i for dual therapy after metformin failure (45). Recently, the GRADE study (46) was conducted to assess treatment efficacy of insulin glargine, glimepiride (SUs), liraglutide (glucagon-like peptide-1 receptor agonists [GLP-1 RA]), and sitagliptin (DPP4i) on microvascular and CVD outcomes when added to metformin. This study found that adding liraglutide could significantly lower the risk of any CVD about 29% relative to other three combined treatments [hazard ratio = 0.71 (95% CI: 0.56, 0.90)]. However, SGLT2i was not considered in this study, and our study did not assess GLP1-RA due to insufficient data. Both treatments may be beneficial for lowering CV events in T2DM patients, in which the choice of second-line oral antihyperglycemic agents should be individualized based on the patient’s clinical needs, preference, and economic constraints, particularly in countries with limited resources.
Our study had several strengths. This study was the first analysis of second-line antihyperglycemic medications for CVD risk reduction in a large-scale population with T2DM based on real-world practice. We also applied three analytical approaches (ITT, PPA and modified ITT) to emulate a target trial on our real-world data. We evaluated the role of newer second-line agents compared to the more commonly used approach of SUs which are still the most commonly prescribed medication in resource limited countries. Furthermore, pioglitazone, which was the only TZD still available and commonly prescribed, was found to provide similar protective benefits as SGLT2i, which would support their continued use in real-world practice.
This study had some limitations. Data used in this study was based on a single center which may limit its generalizability. Only a small number of patients received SGLT2i in Ramathibodi Hospital since its release in 2015. As such, the estimated relative treatment effect is imprecise. Likewise, similarly limited data for GLP-1 RA were available, and none of the patient developed CVD, therefore we were unable to estimate its relative treatment effect. Further multi-center studies are needed to explore the potential benefits of CVD risk reduction associated with both SGLT2i and GLP-1 RA prescribed as an add-on to metformin in those with T2DM. In addition, different drug dosages of individual drugs were not evaluated and should be further explored. Finally, neither CV nor all-cause mortality was considered because none of patients died in the SGLT2i group. These and other important outcomes including HFrEF and peripheral artery disease should also be further considered in longer follow up period.
Conclusion
Our study demonstrated the potential benefits associated with SGLT2i and TZD (pioglitazone) in reducing the risk of CVD in T2DM patients compared to the more commonly prescribed SUs, in real-world practice data. Further multi-center studies should be performed to evaluate GLP-1 RA following metformin treatment to assess its effects compared to other second-line antihyperglycemic agents.
Data availability statement
The raw data supporting the conclusions of this article will be made available by the authors, without undue reservation.
Ethics statement
The studies involving human participants were reviewed and approved by Institutional Review Board of the Faculty of Medicine, Ramathibodi Hospital, Mahidol University (COA. MURA2021/522). The patients/participants provided their written informed consent to participate in this study.
Author contributions
SS contributed substantially to conception and study design, acquisition, analysis, and interpretation of the data, and drafted the article. TL contributed substantially to analysis and interpretation of the data, and drafted the article. TA contributed substantially to data interpretation, drafted and revised the article. PL contributed substantially to data acquisition, analysis, and revised the article. GJM, and JA contributed substantially to data interpretation and revised the article. AT contributed substantially to conception, study design, analysis and interpretation of the data, and revised the article. All authors contributed to the article and approved the submitted version.
Funding
This study was supported by the National Research Council of Thailand (NRCT) N42A640323. The funding agency did not have any role in the design or conduct of this study.
Conflict of interest
The authors declare that the research was conducted in the absence of any commercial or financial relationships that could be construed as a potential conflict of interest.
Publisher’s note
All claims expressed in this article are solely those of the authors and do not necessarily represent those of their affiliated organizations, or those of the publisher, the editors and the reviewers. Any product that may be evaluated in this article, or claim that may be made by its manufacturer, is not guaranteed or endorsed by the publisher.
Supplementary material
The Supplementary Material for this article can be found online at: https://www.frontiersin.org/articles/10.3389/fendo.2023.1094221/full#supplementary-material
References
1. International Diabetes Federation. Diabetes and cardiovascular disease report. Brussels: International Diabetes Federation (2016) p. 1–140.
2. Einarson TR, Acs A, Ludwig C, Panton UH. Prevalence of cardiovascular disease in type 2 diabetes: a systematic literature review of scientific evidence from across the world in 2007-2017. Cardiovasc Diabetol (2018) 17(1):83. doi: 10.1186/s12933-018-0728-6
3. Chen HF, Li CY. Effect-modifications by age and sex on the risks of coronary artery disease and revascularization procedures in relation to diabetes. Diabetes Res Clin Pract (2007) 75(1):88–95. doi: 10.1016/j.diabres.2006.05.020
4. Zelniker TA, Wiviott SD, Raz I, Im K, Goodrich EL, Bonaca MP, et al. SGLT2 inhibitors for primary and secondary prevention of cardiovascular and renal outcomes in type 2 diabetes: a systematic review and meta-analysis of cardiovascular outcome trials. Lancet (2019) 393(10166):31–9. doi: 10.1016/S0140-6736(18)32590-X
5. Mascolo A, Scavone C, Scisciola L, Chiodini P, Capuano A, Paolisso G. SGLT-2 inhibitors reduce the risk of cerebrovascular/cardiovascular outcomes and mortality: A systematic review and meta-analysis of retrospective cohort studies. Pharmacol Res (2021) 172:105836. doi: 10.1016/j.phrs.2021.105836
6. Giugliano D, Longo M, Signoriello S, Maiorino MI, Solerte B, Chiodini P, et al. The effect of DPP-4 inhibitors, GLP-1 receptor agonists and SGLT-2 inhibitors on cardiorenal outcomes: a network meta-analysis of 23 CVOTs. Cardiovasc Diabetology. (2022) 21(1):42. doi: 10.1186/s12933-022-01474-z
7. Zinman B, Wanner C, Lachin JM, Fitchett D, Bluhmki E, Hantel S, et al. Empagliflozin, cardiovascular outcomes, and mortality in type 2 diabetes. New Engl J Med (2015) 373(22):2117–28. doi: 10.1056/NEJMoa1504720
8. Neal B, Perkovic V, Mahaffey KW, De Zeeuw D, Fulcher G, Erondu N, et al. Canagliflozin and cardiovascular and renal events in type 2 diabetes. New Engl J Med (2017) 377(7):644–57. doi: 10.1056/NEJMoa1611925
9. Wiviott SD, Raz I, Bonaca MP, Mosenzon O, Kato ET, Cahn A, et al. Dapagliflozin and cardiovascular outcomes in type 2 diabetes. New Engl J Med (2018) 380(4):347–57. doi: 10.1056/NEJMoa1812389
10. Li C-x, Liang S, Gao L, Liu H. Cardiovascular outcomes associated with SGLT-2 inhibitors versus other glucose-lowering drugs in patients with type 2 diabetes: A real-world systematic review and meta-analysis. PloS One (2021) 16(2):e0244689. doi: 10.1371/journal.pone.0244689
11. McDonagh TA, Metra M, Adamo M, Gardner RS, Baumbach A, Böhm M, et al. 2021 ESC Guidelines for the diagnosis and treatment of acute and chronic heart failure. Eur Heart J (2021) 42(36):3599–726. doi: 10.1093/eurheartj/ehab368
12. Anker SD, Butler J, Filippatos G, Ferreira JP, Bocchi E, Böhm M, et al. Empagliflozin in heart failure with a preserved ejection fraction. New Engl J Med (2021) 385(16):1451–61. doi: 10.1056/NEJMoa2107038
13. Solomon SD, McMurray JJV, Claggett B, de Boer RA, DeMets D, Hernandez AF, et al. Dapagliflozin in heart failure with mildly reduced or preserved ejection fraction. New Engl J Med (2022) 387(12):1089–98. doi: 10.1056/NEJMoa2206286
14. Visseren FLJ, Mach F, Smulders YM, Carballo D, Koskinas KC, Bäck M, et al. 2021 ESC Guidelines on cardiovascular disease prevention in clinical practice. Eur Heart J (2021) 42(34):3227–337. doi: 10.1093/eurheartj/ehab484
15. Udell JA, Yuan Z, Rush T, Sicignano NM, Galitz M, Rosenthal N. Cardiovascular outcomes and risks after initiation of a sodium glucose cotransporter 2 inhibitor. Circulation (2018) 137(14):1450–9. doi: 10.1161/CIRCULATIONAHA.117.031227
16. Goring S, Hawkins N, Wygant G, Roudaut M, Townsend R, Wood I, et al. Dapagliflozin compared with other oral anti-diabetes treatments when added to metformin monotherapy: a systematic review and network meta-analysis. Diabetes Obes Metab (2014) 16(5):433–42. doi: 10.1111/dom.12239
17. Ridderstråle M, Andersen KR, Zeller C, Kim G, Woerle HJ, Broedl UC. Comparison of empagliflozin and glimepiride as add-on to metformin in patients with type 2 diabetes: a 104-week randomised, active-controlled, double-blind, phase 3 trial. Lancet Diabetes Endocrinol (2014) 2(9):691–700. doi: 10.1016/S2213-8587(14)70120-2
18. Kosiborod M, Cavender MA, Fu AZ, Wilding JP, Khunti K, Holl RW, et al. Lower risk of heart failure and death in patients initiated on sodium-glucose cotransporter-2 inhibitors versus other glucose-lowering drugs: The CVD-REAL study (Comparative effectiveness of cardiovascular outcomes in new users of sodium-glucose cotransporter-2 inhibitors). Circulation (2017) 136(3):249–59. doi: 10.1161/CIRCULATIONAHA.117.029190
19. Birkeland KI, Bodegard J, Banerjee A, Kim DJ, Norhammar A, Eriksson JW, et al. Lower cardiorenal risk with sodium-glucose cotransporter-2 inhibitors versus dipeptidyl peptidase-4 inhibitors in patients with type 2 diabetes without cardiovascular and renal diseases: A large multinational observational study. Diabetes Obes Metab (2021) 23(1):75–85. doi: 10.1111/dom.14189
20. Hernán MA, Robins JM. Using big data to emulate a target trial when a randomized trial is not available. Am J Epidemiol (2016) 183(8):758–64. doi: 10.1093/aje/kwv254
21. Stuart EA, Lee BK, Leacy FP. Prognostic score-based balance measures can be a useful diagnostic for propensity score methods in comparative effectiveness research. J Clin Epidemiol. (2013) 66(8 Suppl):S84–S90.e1. doi: 10.1016/j.jclinepi.2013.01.013
22. Austin PC. Balance diagnostics for comparing the distribution of baseline covariates between treatment groups in propensity-score matched samples. Stat Med (2009) 28(25):3083–107. doi: 10.1002/sim.3697
23. Kosiborod M, Lam CSP, Kohsaka S, Kim DJ, Karasik A, Shaw J, et al. Cardiovascular events associated with SGLT-2 inhibitors versus other glucose-lowering drugs: The CVD-REAL 2 study. J Am Coll Cardiol (2018) 71(23):2628–39. doi: 10.1016/j.jacc.2018.03.009
24. Norhammar A, Bodegård J, Nyström T, Thuresson M, Nathanson D, Eriksson JW. Dapagliflozin and cardiovascular mortality and disease outcomes in a population with type 2 diabetes similar to that of the DECLARE-TIMI 58 trial: A nationwide observational study. Diabetes Obes Metab (2019) 21(5):1136–45. doi: 10.1111/dom.13627
25. Cai X, Ji L, Chen Y, Yang W, Zhou L, Han X, et al. Comparisons of weight changes between sodium-glucose cotransporter 2 inhibitors treatment and glucagon-like peptide-1 analogs treatment in type 2 diabetes patients: A meta-analysis. J Diabetes Investig (2017) 8(4):510–7. doi: 10.1111/jdi.12625
26. Mazidi M, Rezaie P, Gao HK, Kengne AP. Effect of sodium-glucose cotransport-2 inhibitors on blood pressure in people with type 2 diabetes mellitus: A systematic review and meta-analysis of 43 randomized control trials with 22 528 patients. J Am Heart Assoc (2017) 6(6):1–12. doi: 10.1161/JAHA.116.004007
27. Imprialos K, Faselis C, Boutari C, Stavropoulos K, Athyros V, Karagiannis A, et al. SGLT-2 inhibitors and cardiovascular risk in diabetes mellitus: A comprehensive and critical review of the literature. Curr Pharm Des (2017) 23(10):1510–21. doi: 10.2174/1381612823666170124123927
28. Gaspari T, Spizzo I, Liu H, Hu Y, Simpson RW, Widdop RE, et al. Dapagliflozin attenuates human vascular endothelial cell activation and induces vasorelaxation: A potential mechanism for inhibition of atherogenesis. Diabetes Vasc Dis Res (2017) 15(1):64–73. doi: 10.1177/1479164117733626
29. Juni RP, Kuster DWD, Goebel M, Helmes M, Musters RJP, van der Velden J, et al. Cardiac microvascular endothelial enhancement of cardiomyocyte function is impaired by inflammation and restored by empagliflozin. JACC: Basic to Trans Science. (2019) 4(5):575–91. doi: 10.1016/j.jacbts.2019.04.003
30. Lopaschuk GD, Verma S. Mechanisms of cardiovascular benefits of sodium glucose Co-transporter 2 (SGLT2) inhibitors: A state-of-the-Art review. JACC Basic Transl Sci (2020) 5(6):632–44. doi: 10.1016/j.jacbts.2020.02.004
31. Shao Q, Meng L, Lee S, Tse G, Gong M, Zhang Z, et al. Empagliflozin, a sodium glucose co-transporter-2 inhibitor, alleviates atrial remodeling and improves mitochondrial function in high-fat diet/streptozotocin-induced diabetic rats. Cardiovasc Diabetol (2019) 18(1):165. doi: 10.1186/s12933-019-0964-4
32. Siriyotha S, Lukkunaprasit T, Looareesuwan P, Nimitphong H, McKay GJ, Attia J, et al. Effects of second−line antihyperglycemic drugs on the risk of chronic kidney disease: applying a target trial approach to a hospital−based cohort of Thai patients with type 2 diabetes. Cardiovasc Diabetol (2022) 21(1):248. doi: 10.1186/s12933-022-01641-2
33. Baron RM, Kenny DA. The moderator-mediator variable distinction in social psychological research: conceptual, strategic, and statistical considerations. J Pers Soc Psychol (1986) 51(6):1173–82. doi: 10.1037/0022-3514.51.6.1173
34. Liao HW, Saver JL, Wu YL, Chen TH, Lee M, Ovbiagele B. Pioglitazone and cardiovascular outcomes in patients with insulin resistance, pre-diabetes and type 2 diabetes: a systematic review and meta-analysis. BMJ Open (2017) 7(1):e013927. doi: 10.1136/bmjopen-2016-013927
35. Nicholls SJ, Tuzcu EM, Wolski K, Bayturan O, Lavoie A, Uno K, et al. Lowering the triglyceride/high-density lipoprotein cholesterol ratio is associated with the beneficial impact of pioglitazone on progression of coronary atherosclerosis in diabetic patients: insights from the PERISCOPE (Pioglitazone effect on regression of intravascular sonographic coronary obstruction prospective evaluation) study. J Am Coll Cardiol (2011) 57(2):153–9. doi: 10.1016/j.jacc.2010.06.055
36. Khera AV, Cuchel M, de la Llera-Moya M, Rodrigues A, Burke MF, Jafri K, et al. Cholesterol efflux capacity, high-density lipoprotein function, and atherosclerosis. N Engl J Med (2011) 364(2):127–35. doi: 10.1056/NEJMoa1001689
37. Rohatgi A, Khera A, Berry JD, Givens EG, Ayers CR, Wedin KE, et al. HDL cholesterol efflux capacity and incident cardiovascular events. N Engl J Med (2014) 371(25):2383–93. doi: 10.1056/NEJMoa1409065
38. Nauck MA, Meier JJ, Cavender MA, Abd El Aziz M, Drucker DJ. Cardiovascular actions and clinical outcomes with glucagon-like peptide-1 receptor agonists and dipeptidyl peptidase-4 inhibitors. Circulation (2017) 136(9):849–70. doi: 10.1161/CIRCULATIONAHA.117.028136
39. Smelcerovic A, Kocic G, Gajic M, Tomovic K, Djordjevic V, Stankovic-Djordjevic D, et al. DPP-4 inhibitors in the Prevention/Treatment of pulmonary fibrosis, heart and kidney injury caused by COVID-19-A therapeutic approach of choice in type 2 diabetic patients? Front Pharmacol (2020) 11:1185. doi: 10.3389/fphar.2020.01185
40. Chu PY, Mariani J, Finch S, McMullen JR, Sadoshima J, Marshall T, et al. Bone marrow-derived cells contribute to fibrosis in the chronically failing heart. Am J Pathol (2010) 176(4):1735–42. doi: 10.2353/ajpath.2010.090574
41. Pan X, Xu S, Li J, Tong N. The effects of DPP-4 inhibitors, GLP-1RAs, and SGLT-2/1 inhibitors on heart failure outcomes in diabetic patients with and without heart failure history: Insights from CVOTs and drug mechanism. Front Endocrinol (2020) 11. doi: 10.3389/fendo.2020.599355
42. Zoungas S. ADVANCE in context: the benefits, risks and feasibility of providing intensive glycaemic control based on gliclazide modified release. Diabetes Obes Metab (2020) 22(Suppl 2):5–11. doi: 10.1111/dom.13968
43. Vaccaro O, Masulli M, Nicolucci A, Bonora E, Del Prato S, Maggioni AP, et al. Effects on the incidence of cardiovascular events of the addition of pioglitazone versus sulfonylureas in patients with type 2 diabetes inadequately controlled with metformin (TOSCA. IT): randomised multicentre trial. Lancet Diabetes Endocrinol (2017) 5:887–97. doi: 10.1016/S2213-8587(17)30317-0
44. Rosenstock J, Perkovic V, Johansen OE, Cooper ME, Kahn SE, Marx N, et al. Effect of linagliptin vs placebo on major cardiovascular events in adults with type 2 diabetes and high cardiovascular and renal risk: the CARMELINA randomized clinical trial. JAMA (2018) 321:69–79. doi: 10.1001/jama.2018.18269
45. International Diabetes Federation. Recommendations for managing type 2 diabetes in primary care (2017). Belgium: International Diabetes Federation (Accessed 27, 2022).
Keywords: diabetes, cardiovascular events, antihyperglycemic drugs, second-line, metformin
Citation: Siriyotha S, Lukkunaprasit T, Angkananard T, Looareesuwan P, McKay GJ, Attia J and Thakkinstian A (2023) Clinical effectiveness of second-line antihyperglycemic drugs on major adverse cardiovascular events: An emulation of a target trial. Front. Endocrinol. 14:1094221. doi: 10.3389/fendo.2023.1094221
Received: 09 November 2022; Accepted: 13 January 2023;
Published: 30 January 2023.
Edited by:
Gaetano Santulli, Albert Einstein College of Medicine, United StatesReviewed by:
Aimo Kannt, Fraunhofer Institute for Translational Medicine and Pharmacology ITMP, GermanyCristina-Mihaela Lacatusu, Grigore T. Popa University of Medicine and Pharmacy, Romania
Copyright © 2023 Siriyotha, Lukkunaprasit, Angkananard, Looareesuwan, McKay, Attia and Thakkinstian. This is an open-access article distributed under the terms of the Creative Commons Attribution License (CC BY). The use, distribution or reproduction in other forums is permitted, provided the original author(s) and the copyright owner(s) are credited and that the original publication in this journal is cited, in accordance with accepted academic practice. No use, distribution or reproduction is permitted which does not comply with these terms.
*Correspondence: Teeranan Angkananard, dGhlZXJhbmFuYTg5QGdtYWlsLmNvbQ==, dGVlcmFuYW5AZy5zd3UuYWMudGg=; Panu Looareesuwan, cGFudS5sb29AbWFoaWRvbC5lZHU=
†These authors have contributed equally to this work and share first authorship