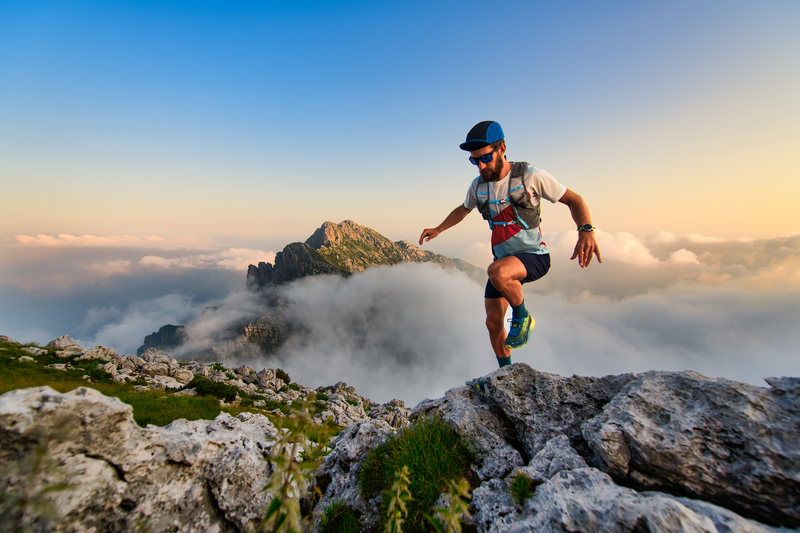
94% of researchers rate our articles as excellent or good
Learn more about the work of our research integrity team to safeguard the quality of each article we publish.
Find out more
ORIGINAL RESEARCH article
Front. Endocrinol. , 06 February 2023
Sec. Bone Research
Volume 14 - 2023 | https://doi.org/10.3389/fendo.2023.1089796
This article is part of the Research Topic Underlying Mechanisms and Treatment for Intervertebral Disc Disease View all 14 articles
Lower back pain (LBP) is a leading cause of disability in the elderly and intervertebral disc degeneration (IDD) is the major contributor to LBP. Ferroptosis is a newly discovered programmed cell death, characterized by iron-dependent lethal lipid peroxidation. Growing evidence has shown that ferroptosis plays important roles in various human diseases. However, the underlying mechanism of ferroptosis in IDD remains elusive. This study is aimed to uncover the key roles of ferroptosis in the pathogenesis and progression of IDD comprehensively. To investigate the ferroptosis related differentially expressed genes (FRDEGs) in IDD, we analyzed the microarray data from the Gene Expression Omnibus (GEO) database. Then we performed functional enrichment analysis and protein-protein interaction (PPI) network analysis, and screened out the hub FRDEGs. To further evaluate the predictive value of these hub FRDEGs, we performed ROC analysis based on the GSE124272 dataset. A total of 80 FRDEGs were identified, including 20 downregulated and 60 upregulated FRDEGs. The FRDEGs were primarily involved in the biological processes of response to chemical, and response to stress. KEGG pathway enrichment analysis showed that the FRDEGs were mainly involved in ferroptosis, TNF signaling pathway, HIF-1 signaling pathway, NOD-like receptor signaling pathway, and IL-17 signaling pathway. Ten hub OSRDEGs were obtained according to the PPI analysis, including HMOX1, KEAP1, MAPK1, HSPA5, TXNRD1, IL6, PPARA, JUN, HIF1A, DUSP1. The ROC analysis and RT-qPCR validation results suggested that most of the hub FRDEGs might be potential signature genes for IDD. This study reveals that ferroptosis might provide promising strategy for the diagnosis and treatment of IDD.
Lower back pain (LBP), a common musculoskeletal problem, is one of the leading causes of disability in the elderly worldwide (1, 2). It is acknowledged by a number of reports that the major contributor to LBP is intervertebral disc degeneration (IDD) (3, 4). Physiologically, the intervertebral disc (IVD) is an avascular structure of the human body in adults. The IVD is composed of three distinct regions, including the internal nucleus pulposus (NP), the peripheral annulus fibrosus (AF), and the inferior and superior cartilaginous endplates (CEP), with characterized cell types in each region respectively. Pathologically, the pathogenesis and progression of IDD is promoted by various and complicated factors, including aging, oxidative stress, inflammation, and mechanical stress, etc. The IDD process is characterized of degeneration of the NP, rapture of the AF, and calcification of the CEP (5, 6). However, the specific molecular mechanisms of disc degeneration remain elusive currently.
Ferroptosis is a newly discovered mode of regulated cell death, which differs from apoptosis, necrosis, and autophagy in morphological, biochemical, and genetic aspects (7). The cells that undergo ferroptosis have distinctive morphological characteristics, including cell membrane disruption and vesiculation, mitochondrial shrinkage with lessened cristae, mitochondrial membrane condensation and outer membrane rupture (8). In mechanism, ferroptosis is typified by intracellular iron-dependent lipid peroxidation and reactive oxygen species (ROS) accumulation to lethal levels (8, 9). Ferroptosis is implicated in various biological activities, including iron homeostasis, lipid peroxidation metabolism, glutathione (GSH) metabolism (10). Ferroptosis is negatively regulated by glutathione peroxidase 4 (GPX4), which is responsible for scavenging intracellular lipid peroxide through GSH (7, 11). It has been reported that the suppression of GPX4 or inhibition of GSH can effectively induce ferroptosis (11). Ferroptosis is also regulated by some other critical pathways, such as p53 signaling, Nrf2 signaling, Hippo signaling as well as mitochondrial signaling pathway (12).
Growing evidence has suggested that ferroptosis is interrelated with multiple pathophysiological contexts, including cancer, degenerative diseases, diabetes, cardiovascular diseases, etc (13, 14). Moreover, it has been demonstrated that ferroptosis might be also associated with some skeletal diseases, including osteoarthritis, osteoporosis, as well as rheumatoid arthritis (15). Osteoarthritis (OA) is a common degenerative joint disorder worldwide, and ferroptosis has been shown to play regulating roles in the pathogenesis and progression of OA. Recently, Miao et al. found that ferroptosis existed in OA, during which the key regulator GPX4 played critical roles in the chondrocyte cell death and extracellular matrix (ECM) degradation (16). Furthermore, by using single cell RNA sequencing analysis, Lv et al. (17) identified an important ferroptosis-associated target named TRPV1 in OA. And TRPV1 activation could protect chondrocytes from ferroptosis and mitigate the development of OA via modulating GPX4. A recent study revealed that some specific ferroptosis-related genes could be promising biomarkers for osteoporosis diagnosis and interventions, including the ER, VDR, IL-6, COL1A1, COL1A2, and PTH (18). Besides, growing evidence has also found the involvement of ferroptosis in the pathogenesis of rheumatoid arthritis, and targeting ferroptosis could be a promising therapeutic strategy for inflammatory arthritis.
Growing evidence has demonstrated that iron overload, closely associated with ferroptosis, is a common phenomenon in the aging process (19, 20). Unexpectedly, recent studies have revealed that this special type of cell death might also be related to IDD, a very common degenerative musculoskeletal disease that progresses with age. In 2021, Zhang et al. (21) established the IDD animal models and found that the levels of iron and Heme Oxygenase 1 (HO-1) were obviously elevated, and the levels of ferritin light chain markedly decreased in IDD compared to control. More recently, a study reported that iron overload could be an independent risk factor for IDD and it promoted endplate degeneration and calcification through oxidative stress and ferroptosis (22). However, the underlying mechanism of ferroptosis in IDD remains elusive and still needs further investigations. In the current research work, we aimed to explore the key roles of ferroptosis in the pathogenesis and progression of IDD comprehensively by using mature and recognized bioinformatic analysis methods, and hope to provide novel diagnostic and therapeutic targets for IDD.
The data used in this study is available in the Gene Expression Omnibus (GEO) repository (https://www.ncbi.nlm.nih.gov/geo/), with an accession number of GSE56081 for the differentially expressed genes (DGEs) identification dataset. The GEO dataset GSE124272 was used as a validation analysis dataset. The GSE56081 dataset contained five degenerated disc NP tissues and five control NP tissues (23). The GSE124272 dataset contained whole blood samples obtained from eight patients with IDD and eight healthy controls (24). The ferroptosis related genes, including ferroptosis markers, ferroptosis drivers, ferroptosis suppressors and unclassified genes, were acquired from the FerrDb online database (http://www.zhounan.org/ferrdb/current/). This study was approved by the Ethics Committee of Peking University Third Hospital.
The DGEs in IDD were acquired by using the R package “limma”, and the criterions of identifying the DEGs were set as the fold change > 2 and the adjusted p value < 0.05. The FRDEGs were acquired by the intersection of DGEs based on GSE56081 and ferroptosis related genes based on FerrDb database by using the Venn diagram. Volcano plot of the DEGs, and hierarchical cluster heatmap of the FRDEGs were obtained by the R package “ggplot2”.
The Gene Ontology (GO) analysis and Kyoto Encyclopedia of Genes and Genomes (KEGG) analysis were conducted for the FRDEGs and hub FRDEGs. The GO is an international standardized gene function classification system which is composed of three categories: biological process (BP), cell component (CC), as well as molecular function (MF). The GO analysis was conducted by the R package “clusterProfiler” (version 3.14.3) based on the GO annotations in R package “org.hs.eg.db” (version 3.1.0). The KEGG analysis was applied to determine related signaling pathways for FRDEGs. The KEGG analysis was conducted by the R package “clusterProfiler” (version 3.14.3) based on the latest KEGG pathway genes annotations, which were obtained from the KEGG rest API (https://www.kegg.jp/kegg/rest/keggapi.html). The corresponding item with a p value < 0.05 was considered statistically significant. The enriched items were plotted by using the R package “ggplot2”.
The PPI network analysis of the FRDEGs was conducted based on the STRING database v11.5 (https://cn.string-db.org/), a commonly used tool to evaluate the protein-protein interactions. The protein interaction pairs with score > 0.40 were further imported to the Cytoscape software v3.2 (https://cytoscape.org/) to establish PPI network. In the PPI network, the nodes represented the FRDEGs enriched in the STRING database, and the edges (connections between nodes) represented the interactions between different FRDEGs. The PPI score was obtained by using the degree analysis method in the CytoHubba plug-in, and the top ten significantly connected nodes were selected as the hub FRDEGs for further analysis.
To evaluate the relationships among these hub FRDEGs, the correlations among the hub FRDEGs were conducted by using Pearson correlation analysis. In the Pearson’s correlation analysis, the r value refers the correlation coefficient and was used to evaluate the effect size. And then the correlation matrix heatmap and the scatter plots were mapped by using the R package “ggplot2”.
Receiver operating characteristics (ROC) analysis was applied to obtain the area under the curve (AUC) values by using the R software package “pROC” (version 1.17.0.1). In brief, the gene expression of the corresponding hub FRDEGs was obtained according to the GEO dataset GSE124272. Then the roc function of “pROC” was applied for ROC analysis, and the ci function of “pROC” was used to evaluate AUC and confidence interval. In the ROC curve, the sensitivity values were plotted on the Y-axis, and the false positive rates (1-specificity) values were plotted on the X-axis. The ROC curve with an AUC value >= 0.70 was considered to indicate an adequate predictive value.
The rat NP cells were treated with different concentrations (0, 25, 50, 75 μM) of tert-butyl hydroperoxide (TBHP, Sigma-Aldrich, St. Louis, MO, USA) for 24 h to establish an in vitro IDD cell model, as reported previously (25, 26). The cell viability of the rat NP cells was evaluated by the cell counting kit- (CCK-) 8 assay (Dojindo, Japan). In brief, the cells were seeded in 24-well plates and incubated for 24 h, and then treated with TBHP with different concentrations. Subsequently, 20 μL of CCK-8 solution was added to each well with 200 μL culture medium. The cells were then incubated at 37°C for 2 h, and then the absorbance signal at 450 nm was detected using the SpectraMax iD3 spectrophotometer (Molecular Devices).
Total RNA from the rat NP cells in each group was isolated by the SteadyPure Universal RNA Extraction Kit (AG21017, Accurate Biology, China) following the manufacturer’s instructions. RNA purity and concentration were determined by the DHS NanoPro 2020 spectrophotometer. Then the RNA was reverse-transcribed to cDNA by the Evo M-MLV Mix Kit with gDNA Clean for qPCR (AG11728, Accurate Biology, China). The qPCR assay was performed by using a SYBR Green Premix Pro Taq HS qPCR Kit (ROX Plus) (AG11718, Accurate Biology, China) with the QuantStudio 3 Real-Time PCR System (Applied Biosystems, USA). The qPCR conditions were set as follows: 95°C for 30 s, 40 cycles of 95°C for 5 s, and 60°C for 30 s; followed by a melt curve stage of 95°C for 15 s, 60°C for 1 min and 95°C for 15 s. Relative expression levels of genes was calculated by using the 2−ΔΔCT method and normalized to GAPDH. The primers used in the present study are listed as follows (5’-3’). HMOX1 FORWARD: GGGTCAGGTGTCCAGGGAAGG; HMOX1 REVERSE: TGGGTTCTGCTTGTTTCGCTCTATC; KEAP1 FORWARD: TGCTCAACCGCTTGCTGTATGC; KEAP1 REVERSE: TCATCCGCCACTCATTCCTCTCC; MAPK1 FORWARD: TGAAGACACAGCACCTCAGCAATG; MAPK1 REVERSE: GGTGTTCAGCAGGAGGTTGGAAG; HSPA5 FORWARD: CGGAGGAGGAGGACAAGAAGGAG; HSPA5 REVERSE: ATACGACGGTGTGATGCGGTTG; TXNRD1 FORWARD: CACGGATGAGGAGCAGACCAATG; TXNRD1 REVERSE: CATACAGCCTCTGAGCCAGCAATC; IL6 FORWARD: ACTTCCAGCCAGTTGCCTTCTTG; IL6 REVERSE: TGGTCTGTTGTGGGTGGTATCCTC; PPARA FORWARD: ACGATGCTGTCCTCCTTGATGAAC; PPARA REVERSE: ATGATGTCGCAGAATGGCTTCCTC; JUN FORWARD: GGAAACGACCTTCTACGACGATGC; JUN REVERSE: GGAGGTGCGGCTTCAGATTGC; HIF1A FORWARD: CCGCCACCACCACTGATGAATC; HIF1A REVERSE: GTGAGTACCACTGTATGCTGATGCC; DUSP1 FORWARD: GCCACCATCTGCCTTGCTTACC; DUSP1 REVERSE: GATAATACTCCGCCTCTGCTTCACG; GAPDH FORWARD: GACATGCCGCCTGGAGAAAC; GAPDH REVERSE: AGCCCAGGATGCCCTTTAGT.
The data in IDD cell model were analyzed by GraphPad Prism software and were presented as means ± standard deviation of three independent experiments. The difference between groups was analyzed by unpaired Student’s t test. The correlation analysis among the hub FRDEGs was performed by Pearson analysis. A p value of less than 0.05 was considered to be statistically significant.
First, we screened out the differentially expressed genes (DGEs) in IDD compared to control based on GSE56081 dataset. The volcano plot showed there are 2269 DGEs in total, including 847 downregulated and 1422 upregulated genes (Figure 1A). Subsequently, we intersected the DGEs in IDD with the ferroptosis-related genes based on FerrDb database. A total of 80 ferroptosis related differentially expressed genes (FRDEGs) were determined, as presented in the Venn diagram (Figure 1B). Among these 80 FRDEGs, 20 were downregulated and 60 were upregulated, as shown in Table 1. The hierarchical cluster heatmap demonstrated that the expression of ferroptosis-related genes in IDD group was obviously different from that in control group (Figure 2).
Figure 1 Identification of the differentially expressed genes between IDD and control groups. (A) The volcano plot of the differentially expressed genes in GSE56081 microarray data from the GEO database, in which the X-axis refers to the -log10(p value) and the Y-axis refers to the log2(fold change). The red dots represent the upregulated genes, and the blue dots represent the downregulated genes; the gray dots represent the genes without significant differential expression. (B) Venn diagram of the differentially expressed genes analyzed according to GSE56081 and the ferroptosis-related genes based on FerrDb database.
Figure 2 The hierarchical cluster heatmap of the 80 ferroptosis related differentially expressed genes (FRDEGs). The color scale indicates the relative gene expression of each sample. The red represents upregulated genes in IDD group compared to control, and the blue represents downregulated genes in IDD group. Among these FRDEGs, 20 FRDEGs are downregulated and 60 FRDEGs are upregulated.
The Gene Ontology (GO) is an international standardized gene function classification system which is commonly used to classify the predicted genes function. There are three main GO categories: biological process (BP), cellular component (CC), and molecular function (MF). As for the GO enrichment analysis for biological process, these FRDEGs are mainly involved in response to chemical, response to stress, and cellular response to chemical stimulus (Figure 3A). As shown in Figure 3B, the most significantly enriched GO items for cellular component are endomembrane system, vesicle, and extracellular region part. As for the molecular function, these FRDEGs are mainly involved in enzyme binding, transition metal ion binding, and oxidoreductase activity (Figure 3C). And then, we conducted the Kyoto Encyclopedia of Genes and Genomes (KEGG) analysis for the 80 FRDEGs. As indicated in Figure 3D, results show that the FRDEGs are primarily involved in the following significant pathways: Ferroptosis, TNF signaling pathway, HIF-1 signaling pathway, NOD-like receptor signaling pathway, and IL-17 signaling pathway, etc.
Figure 3 Functional enrichment analysis of the FRDEGs. (A) The top 10 significantly enriched GO terms in the category of biological process (BP) for the FRDEGs. (B) The top 10 significantly enriched GO terms in the category of cellular component (CC). (C) The top 10 significantly enriched GO terms in the category of molecular function (MF). (D) Kyoto Encyclopedia of Genes and Genomes (KEGG) pathway enrichment analysis for the FRDEGs.
The PPI network analysis was performed based on the STRING database and visualized by Cytoscape software (Figure 4A). Then we determined the top ten hub FRDEGs based on the PPI score, including HMOX1, KEAP1, MAPK1, HSPA5, TXNRD1, IL6, PPARA, JUN, HIF1A, and DUSP1, as shown in Figure 4B. Detailed information of these hub FRDEGs was presented in Table 2. Among these hub FRDEGs, KEAP1 and IL6 were downregulated in IDD, and the rest six hub genes were obviously upregulated. Most of these hub genes had either promoting or inhibiting effects on ferroptosis, and the potential roles of TXNRD1 on ferroptosis was not fully understand. Next, we performed Pearson correlation analysis to evaluate the relationships among these hub FRDEGs (Figure 4C). The most negatively related pair was KEAP1-MAPK1, and the most positively related pairs were HSPA5-JUN, HSPA5-TXNRD1, HSPA5-DUSP1, and DUSP1-JUN. The correlation analysis of HSPA5-JUN pair was presented in Figure 4D, with a r value of 0.99 and p value < 0.01. The correlation analysis of KEAP1-MAPK1 pair was shown in Figure 4E, with a r value of -0.93 and p value < 0.01.
Figure 4 PPI analysis of the FRDEGs. (A) PPI analysis of these FRDEGs. (B) The top 10 hub FRDEGs. (C) Correlation analysis among the top 10 hub FRDEGs by Pearson analysis. (D) Correlation analysis between HSPA5 and JUN by Pearson analysis. (E) Correlation analysis between KEAP1 and MAPK1 by Pearson analysis.
To further investigate the potential molecular functions of the top ten hub FRDEGs, we performed functional and pathway enrichment analysis. As for the GO enrichment analysis in the category of biological process, these hub FRDEGs are mainly involved in response to oxidative stress, response to toxic substance, response to inorganic substance, and cellular response to oxidative stress (Figure 5A). In the category of cellular component, the most significantly enriched GO items include nuclear part, caveola, neuron projection cytoplasm, and plasma membrane raft (Figure 5B). In the molecular function, the FRDEGs are mainly associated with transcription factor binding, protein domain specific binding, enzyme binding, and ubiquitin protein ligase binding (Figure 5C). Subsequently, we performed KEGG pathway enrichment analysis for the ten hub genes. As shown in Figure 5D, it is suggested that the most meaningful and significantly enriched pathways include Th17 cell differentiation and HIF-1 signaling pathway.
Figure 5 Function analysis of the hub FRDEGs. (A) The top 10 significantly enriched GO terms in the category of biological process for these hub FRDEGs. (B) The top 10 significantly enriched GO terms in the category of cellular component. (C) The top 10 significantly enriched GO terms in the category of molecular function. (D) KEGG pathway analysis for these hub FRDEGs.
To evaluate the predictive value of these hub FRDEGs, we performed ROC analysis based on the GSE124272 dataset. The ROC curve reveals that the AUC values of six hub genes are greater than or equal to 0.70, including KEAP1, MAPK1, HSPA5, TXNRD1, JUN, and HIF1A (Figures 6A–J). The above data suggested that these six hub FRDEGs have potential predictive values for IDD.
Figure 6 Validation of these hub FRDEGs based on the GSE124272 dataset. (A–J) ROC analysis of the ten hub FRDEGs, including HMOX1, KEAP1, MAPK1, HSPA5, TXNRD1, IL6, PPARA, JUN, HIF1A, and DUSP1. The X-axis represents the (1-Specificity), and the Y-axis represents the Sensitivity.
To further validate the expression of theses hub FRDEGs in IDD, we established the IDD cell model by treating rat disc NP cells with TBHP. As shown in Figure 7A, the TBHP treatment for 24 h obviously inhibited the cell viability of rat NP cells in a dose-dependent manner, and the concentration of 75 μM was selected for subsequent experiments. Then we performed validation experiments for theses hub FRDEGs in the IDD cell model by RT-qPCR assay. As shown in Figures 7B–K, TBHP had significant effects on the mRNA expression levels of the hub FRDEGs. Compared to control group, the expression levels of HMOX1, KEAP1 and HSPA5 were downregulated in TBHP group, and the expression levels of IL6 and DUSP1 were upregulated in TBHP group.
Figure 7 Validation of these hub FRDEGs in IDD cell model. (A) The cell viability of the rat NP cells treated with different concentrations of TBHP for 24 h was determined by the CCK-8 analysis. (B–K) The mRNA expression levels of these ten hub FRDEGs in the rat NP cells with or without TBHP treatment were determined by RT-qPCR assay. ns, not significant. ∗p < 0.05, ∗∗∗p < 0.001, n = 3.
IDD is the one of the leading causes of LBP, which is a very common musculoskeletal disease and has brought a heavy healthcare burden and great socioeconomic cost globally (1–3). Ferroptosis is a newly discovered type of programmed cell death which is distinct from other types of cell death. More and more studies have demonstrated that ferroptosis is closely associated with multiple human diseases, especially degenerative skeletal diseases, including osteoarthritis, osteoporosis, and inflammatory arthritis (15). Interestingly, ferroptosis might also be interrelated with the pathogenesis and progression of IDD. In the present research, we performed comprehensive bioinformatic analysis to uncover the significant roles of ferroptosis in IDD. Firstly, we screened out the DGEs in IDD according to the public datasets, including 847 downregulated genes and 1422 upregulated genes. Then we found that the expression of ferroptosis-related DGEs in IDD group was different from that in control group. We have identified 80 FRDEGs, including 20 downregulated FRDEGs and 60 upregulated FRDEGs.
In this research work, we conducted functional enrichment analysis to investigate the enriched GO items and significant pathways based on these FRDEGs. These FRDEGs are mainly related to response to chemical, response to stress, and cellular response to chemical stimulus, etc. It is worth noting that the FRDEGs are closely associated with several important pathways in IDD, including TNF signaling pathway, HIF-1 signaling pathway, and IL-17 signaling pathway. TNF-α, a member of the tumor necrosis factor (TNF) superfamily, was reported to be dysregulated in degenerated IVDs (27). Moreover, TNF signaling is deeply involved in various pathological process during IDD, including extracellular matrix (ECM) degradation, apoptosis, autophagy inflammatory responses (27). Hypoxia-inducible factors (HIFs) are transcription factors that that plays essential roles in the cellular response to low oxygen (28). The hypoxia inducible factor 1 subunit alpha (HIF1A), one of the key FRDEGs identified in this study, was reported to be decreased with the disc degeneration and participated in the IDD process through interacting with autophagy (29). However, another research work by Wang et al. (30) demonstrated that HIF1A expression was dysregulated in cartilaginous endplate and annulus fibrosus tissues of IDD patients and mouse models. They also found that aberrant activation of HIF1A in EP and AF tissues was a pathological factor for DDD, and inhibition of its aberrant activation prevented the IDD development in animal models. Interleukin-17 (IL-17), namely IL-17A, is a key cytokine of IL-17 family and is primarily secreted by T helper 17 (Th17) cells. IL-17 can trigger various signal pathways to exert regulating effects on mammalian cells (31, 32). Importantly, the expression of IL-17 was positively correlated the degree of IDD, and it could promote the IDD progression by regulating ECM metabolism, inflammatory responses, neo-angiogenesis, and NP cell autophagy and proliferation (33). It is suggested that the FRDEGs might play roles in regulating these critical signaling pathways during IDD initiation and progression, which needs further investigations in the future.
Moreover, we conducted the PPI network analysis to further identify the key and hub genes among these FRDEGs. We determined ten most important hub FRDEGs, as follows: HMOX1, KEAP1, MAPK1, HSPA5, TXNRD1, IL6, PPARA, JUN, HIF1A, and DUSP1. Then we performed functional and pathway enrichment analysis to further explore the potential molecular functions of the ten hub genes. Importantly, these hub FRDEGs are primarily involved in response to oxidative stress and cellular response to oxidative stress. It is suggested that these hub FRDEGs are closely related to oxidative stress. Oxidative stress has played key roles in the pathogenesis and development of IDD, as reported in previous studies from ours and others (34–37). Interestingly, oxidative stress is closely associated with ferroptosis. In 2009, Reardon et al. (38) reported that iron injections could upregulate skeletal muscle iron content and promote oxidative stress in mice. Recently, researchers have found that iron overload was an independent risk factor for IDD and it accelerated IDD development via oxidative stress and ferroptosis in endplate chondrocytes (22). Besides, oxidative stress could induce ferroptosis in AF cells and NP cells in an autophagy‐dependent way during IDD process (39). Therefore, the interaction between oxidative stress and ferroptosis has significantly contributed to the disc degeneration. In the pathway enrichment analysis, the most meaningful and significantly enriched pathways include Th17 cell differentiation and HIF-1 signaling pathway, and related contents have been discussed above.
We further performed ROC analysis to evaluate the predictive value of the hub FRDEGs, and also established IDD cell model to validate these genes expression in the current study. Results of ROC analysis showed that six out of the ten hub FRDEGs might be potential signature genes for IDD, including KEAP1, MAPK1, HSPA5, TXNRD1, JUN, and HIF1A. KEAP1, the Kelch like ECH associated protein 1, has been found to exert promoting functions on ferroptosis (40). KEAP1 is a redox sensor for ROS and electrophiles and negatively modulates the activation of nuclear factor E2-related factor 2 (NRF2) signaling (41). In fact, the KEAP1-NRF2 complex is a redox-sensitive transcriptional regulatory system to protect cells against oxidative stress injury (42). In our previous review article, we have comprehensively discussed the roles of KEAP1-NRF2 system in IDD progression (4). This crucial antioxidant defense system could regulate the NP cell apoptosis, senescence, extracellular matrix (ECM) metabolism, inflammatory responses, and EP calcification in IDD. MAPK1, mitogen-activated protein kinase 1, is a core signal transductor of the MAPK/ERK signaling pathway, and it could facilitate ferroptosis through regulating ROS production (43). Evidence has shown that the MAPK1 expression was increased in the degenerated disc tissues and it could accelerate the development of disc degeneration (44). HSPA5, heat shock protein family A (Hsp70) member 5, was found to resist ferroptosis in cancer cells (45). Mechanistically, HSPA5 can bind to GPX4 protein and suppress the degradation of GPX4, which is a vital suppressor of intracellular lipid peroxidation and ferroptosis as described above. However, whether and how HSPA5 participates in IDD process is unclear so far. Though the effects of TXNRD1 (thioredoxin reductase 1) on ferroptosis is not fully understood, accumulating evidence has shown that TXNRD1 might also take part in the lipid peroxidation and ferroptosis (46, 47). The roles of TXNRD1 in IDD is not so clear either. JUN, namely Jun proto-oncogene, was demonstrated to inhibit erastin-induced ferroptosis in Schwann cells (48). A previous work has revealed that the c-Jun could alleviate IDD by modulating TGF-β (49). Previous studies have reported that HIF1A could exert promoting or inhibiting effects in regulating ferroptosis (50, 51). Moreover, as mentioned above, HIF1A has exerted critical regulatory functions in the disc degeneration process, and it could be also an important target to prevent the IDD development (29, 30, 52). Therefore, these key FRDEGs might be closely involved in the initiation and progression of IDD.
Accumulating studies have attempted to explore the underlying mechanisms of IDD by bioinformatic analysis, including inflammatory response, immune infiltration, mitochondrial dysfunction, etc (53, 54). The present study focused on the association between ferroptosis with IDD, and provided novel insights into the pathogenesis of IDD. However, certain limitations should be noted when interpreting our findings. First, we did not perform microarrays or RNA sequencing in this study. The gene expression data was obtained from the GEO database, and especially, the validation dataset was from blood samples of IDD patients and healthy controls. Second, the IDD cell model used in our study could not reflect the intact IDD microenvironment in vivo, which was far more complexed than in vitro models. And this may be part of the cause for some hub FRDEGs with different expression patterns in the microarray analysis and in the IDD cell model results. Lastly, the cellular and molecular biology experiments did not unveil specific mechanisms of ferroptosis in IDD, which needed more in-depth research to explore in the future.
In summary, this study illuminated that the expression pattern of ferroptosis related genes in IDD was distinct from controls. We have determined 80 FRDEGs dysregulated in IDD. Furthermore, ten most important OSRDEGs were identified and validated, including HMOX1, KEAP1, MAPK1, HSPA5, TXNRD1, IL6, PPARA, JUN, HIF1A, DUSP1. These hub FRDEGs had close relationships with oxidative stress and some other crucial signaling pathway, which were deeply involved in IDD initiation and development. These identified hub FRDEGs might be potential signature genes for IDD. This work reveals that ferroptosis might provide a novel and promising strategy for the diagnosis and treatment of the disc degeneration.
The original contributions presented in the study are included in the article/supplementary material. Further inquiries can be directed to the corresponding author.
WL designed and supervised the study. QX and YZ performed the experiments, analyzed the data, and wrote the manuscript. All authors contributed to the article and approved the submitted version.
This study was supported by the National Natural Science Foundation of China (Grant No. 82172480).
The authors declare that the research was conducted in the absence of any commercial or financial relationships that could be construed as a potential conflict of interest.
All claims expressed in this article are solely those of the authors and do not necessarily represent those of their affiliated organizations, or those of the publisher, the editors and the reviewers. Any product that may be evaluated in this article, or claim that may be made by its manufacturer, is not guaranteed or endorsed by the publisher.
1. Maher C, Underwood M, Buchbinder R. Non-specific low back pain. Lancet (2017) 389(10070):736–47. doi: 10.1016/s0140-6736(16)30970-9
2. Zhou Z, Hui ES, Kranz GS, Chang JR, de Luca K, Pinto SM, et al. Potential mechanisms underlying the accelerated cognitive decline in people with chronic low back pain: A scoping review. Ageing Res Rev (2022), 82:101767. doi: 10.1016/j.arr.2022.101767
3. Binch ALA, Fitzgerald JC, Growney EA, Barry F. Cell-based strategies for ivd repair: Clinical progress and translational obstacles. Nat Rev Rheumatol (2021) 17(3):158–75. doi: 10.1038/s41584-020-00568-w
4. Xiang Q, Zhao Y, Lin J, Jiang S, Li W. The Nrf2 antioxidant defense system in intervertebral disc degeneration: Molecular insights. Exp Mol Med (2022) 54(8):1067–75. doi: 10.1038/s12276-022-00829-6
5. Sakai D, Grad S. Advancing the cellular and molecular therapy for intervertebral disc disease. Adv Drug Delivery Rev (2015) 84:159–71. doi: 10.1016/j.addr.2014.06.009
6. Sampara P, Banala RR, Vemuri SK, Av GR, Gpv S. Understanding the molecular biology of intervertebral disc degeneration and potential gene therapy strategies for regeneration: A review. Gene Ther (2018) 25(2):67–82. doi: 10.1038/s41434-018-0004-0
7. Dixon SJ, Lemberg KM, Lamprecht MR, Skouta R, Zaitsev EM, Gleason CE, et al. Ferroptosis: An iron-dependent form of nonapoptotic cell death. Cell (2012) 149(5):1060–72. doi: 10.1016/j.cell.2012.03.042
8. Stockwell BR, Friedmann Angeli JP, Bayir H, Bush AI, Conrad M, Dixon SJ, et al. Ferroptosis: A regulated cell death nexus linking metabolism, redox biology, and disease. Cell (2017) 171(2):273–85. doi: 10.1016/j.cell.2017.09.021
9. Dixon SJ, Stockwell BR. The role of iron and reactive oxygen species in cell death. Nat Chem Biol (2014) 10(1):9–17. doi: 10.1038/nchembio.1416
10. Wu S, Zhu C, Tang D, Dou QP, Shen J, Chen X. The role of ferroptosis in lung cancer. biomark Res (2021) 9(1):82. doi: 10.1186/s40364-021-00338-0
11. Yang WS, SriRamaratnam R, Welsch ME, Shimada K, Skouta R, Viswanathan VS, et al. Regulation of ferroptotic cancer cell death by Gpx4. Cell (2014) 156(1-2):317–31. doi: 10.1016/j.cell.2013.12.010
12. Ge C, Zhang S, Mu H, Zheng S, Tan Z, Huang X, et al. Emerging mechanisms and disease implications of ferroptosis: Potential applications of natural products. Front Cell Dev Biol (2021) 9:774957. doi: 10.3389/fcell.2021.774957
13. Toyokuni S, Ito F, Yamashita K, Okazaki Y, Akatsuka S. Iron and thiol redox signaling in cancer: An exquisite balance to escape ferroptosis. Free Radic Biol Med (2017) 108:610–26. doi: 10.1016/j.freeradbiomed.2017.04.024
14. Conrad M, Angeli JP, Vandenabeele P, Stockwell BR. Regulated necrosis: Disease relevance and therapeutic opportunities. Nat Rev Drug Discovery (2016) 15(5):348–66. doi: 10.1038/nrd.2015.6
15. Liu X, Wang T, Wang W, Liang X, Mu Y, Xu Y, et al. Emerging potential therapeutic targets of ferroptosis in skeletal diseases. Oxid Med Cell Longev (2022) 2022:3112388. doi: 10.1155/2022/3112388
16. Miao Y, Chen Y, Xue F, Liu K, Zhu B, Gao J, et al. Contribution of ferroptosis and Gpx4’s dual functions to osteoarthritis progression. EBioMedicine (2022) 76:103847. doi: 10.1016/j.ebiom.2022.103847
17. Lv Z, Han J, Li J, Guo H, Fei Y, Sun Z, et al. Single cell rna-seq analysis identifies ferroptotic chondrocyte cluster and reveals Trpv1 as an anti-ferroptotic target in osteoarthritis. EBioMedicine (2022) 84:104258. doi: 10.1016/j.ebiom.2022.104258
18. Hu Y, Han J, Ding S, Liu S, Wang H. Identification of ferroptosis-associated biomarkers for the potential diagnosis and treatment of postmenopausal osteoporosis. Front Endocrinol (Lausanne) (2022) 13:986384. doi: 10.3389/fendo.2022.986384
19. DeRuisseau KC, Park YM, DeRuisseau LR, Cowley PM, Fazen CH, Doyle RP. Aging-related changes in the iron status of skeletal muscle. Exp Gerontol (2013) 48(11):1294–302. doi: 10.1016/j.exger.2013.08.011
20. Kim BJ, Ahn SH, Bae SJ, Kim EH, Lee SH, Kim HK, et al. Iron overload accelerates bone loss in healthy postmenopausal women and middle-aged men: A 3-year retrospective longitudinal study. J Bone Miner Res (2012) 27(11):2279–90. doi: 10.1002/jbmr.1692
21. Zhang Y, Han S, Kong M, Tu Q, Zhang L, Ma X. Single-cell rna-seq analysis identifies unique chondrocyte subsets and reveals involvement of ferroptosis in human intervertebral disc degeneration. Osteoarthr Cartilage (2021) 29(9):1324–34. doi: 10.1016/j.joca.2021.06.010
22. Wang W, Jing X, Du T, Ren J, Liu X, Chen F, et al. Iron overload promotes intervertebral disc degeneration Via inducing oxidative stress and ferroptosis in endplate chondrocytes. Free Radical Biol Med (2022) 190:234–46. doi: 10.1016/j.freeradbiomed.2022.08.018
23. Wan ZY, Song F, Sun Z, Chen YF, Zhang WL, Samartzis D, et al. Aberrantly expressed long noncoding rnas in human intervertebral disc degeneration: A microarray related study. Arthritis Res Ther (2014) 16(5):465. doi: 10.1186/s13075-014-0465-5
24. Wang Y, Dai G, Li L, Liu L, Jiang L, Li S, et al. Transcriptome signatures reveal candidate key genes in the whole blood of patients with lumbar disc prolapse. Exp Ther Med (2019) 18(6):4591–602. doi: 10.3892/etm.2019.8137
25. Li Y, Pan D, Wang X, Huo Z, Wu X, Li J, et al. Silencing Atf3 might delay tbhp-induced intervertebral disc degeneration by repressing npc ferroptosis, apoptosis, and ecm degradation. Oxid Med Cell Longev (2022) 2022:4235126. doi: 10.1155/2022/4235126
26. Kang L, Xiang Q, Zhan S, Song Y, Wang K, Zhao K, et al. Restoration of autophagic flux rescues oxidative damage and mitochondrial dysfunction to protect against intervertebral disc degeneration. Oxid Med Cell Longev (2019) 2019:7810320. doi: 10.1155/2019/7810320
27. Wang C, Yu X, Yan Y, Yang W, Zhang S, Xiang Y, et al. Tumor necrosis factor-A: A key contributor to intervertebral disc degeneration. Acta Biochim Biophys Sin (2017) 49(1):1–13. doi: 10.1093/abbs/gmw112
28. McGettrick AF, O’Neill LAJ. The role of hif in immunity and inflammation. Cell Metab (2020) 32(4):524–36. doi: 10.1016/j.cmet.2020.08.002
29. He R, Wang Z, Cui M, Liu S, Wu W, Chen M, et al. Hif1a alleviates compression-induced apoptosis of nucleus pulposus derived stem cells Via upregulating autophagy. Autophagy (2021) 17(11):3338–60. doi: 10.1080/15548627.2021.1872227
30. Wang Z, Chen H, Tan Q, Huang J, Zhou S, Luo F, et al. Inhibition of aberrant Hif1α activation delays intervertebral disc degeneration in adult mice. Bone Res (2022) 10(1):2. doi: 10.1038/s41413-021-00165-x
31. Konieczny P, Xing Y, Sidhu I, Subudhi I, Mansfield KP, Hsieh B, et al. Interleukin-17 governs hypoxic adaptation of injured epithelium. Science (2022) 377(6602):eabg9302. doi: 10.1126/science.abg9302
32. Mimpen JY, Baldwin MJ, Cribbs AP, Philpott M, Carr AJ, Dakin SG, et al. Interleukin-17a causes osteoarthritis-like transcriptional changes in human osteoarthritis-derived chondrocytes and synovial fibroblasts in vitro. Front Immunol (2021) 12:676173. doi: 10.3389/fimmu.2021.676173
33. Tan JH, Li ZP, Liu LL, Liu H, Xue JB. Il-17 in intervertebral disc degeneration: Mechanistic insights and therapeutic implications. Cell Biol Int (2022) 46(4):535–47. doi: 10.1002/cbin.11767
34. Zhao Y, Xiang Q, Lin J, Jiang S, Li W. Oxidative stress in intervertebral disc degeneration: New insights from bioinformatic strategies. Oxid Med Cell Longev (2022) 2022:2239770. doi: 10.1155/2022/2239770
35. Song Y, Li S, Geng W, Luo R, Liu W, Tu J, et al. Sirtuin 3-dependent mitochondrial redox homeostasis protects against ages-induced intervertebral disc degeneration. Redox Biol (2018) 19:339–53. doi: 10.1016/j.redox.2018.09.006
36. Li Y, Chen L, Gao Y, Zou X, Wei F. Oxidative stress and intervertebral disc degeneration: Pathophysiology, signaling pathway, and therapy. Oxid Med Cell Longev (2022) 2022:1984742. doi: 10.1155/2022/1984742
37. Kang L, Liu S, Li J, Tian Y, Xue Y, Liu X. The mitochondria-targeted anti-oxidant mitoq protects against intervertebral disc degeneration by ameliorating mitochondrial dysfunction and redox imbalance. Cell Prolif (2020) 53(3):e12779. doi: 10.1111/cpr.12779
38. Reardon TF, Allen DG. Iron injections in mice increase skeletal muscle iron content, induce oxidative stress and reduce exercise performance. Exp Physiol (2009) 94(6):720–30. doi: 10.1113/expphysiol.2008.046045
39. Yang RZ, Xu WN, Zheng HL, Zheng XF, Li B, Jiang LS, et al. Involvement of oxidative stress-induced annulus fibrosus cell and nucleus pulposus cell ferroptosis in intervertebral disc degeneration pathogenesis. J Cell Physiol (2021) 236(4):2725–39. doi: 10.1002/jcp.30039
40. Fan Z, Wirth AK, Chen D, Wruck CJ, Rauh M, Buchfelder M, et al. Nrf2-Keap1 pathway promotes cell proliferation and diminishes ferroptosis. Oncogenesis (2017) 6(8):e371. doi: 10.1038/oncsis.2017.65
41. Itoh K, Wakabayashi N, Katoh Y, Ishii T, Igarashi K, Engel JD, et al. Keap1 represses nuclear activation of antioxidant responsive elements by Nrf2 through binding to the amino-terminal Neh2 domain. Genes Dev (1999) 13(1):76–86. doi: 10.1101/gad.13.1.76
42. Yamamoto M, Kensler TW, Motohashi H. The Keap1-Nrf2 system: A thiol-based sensor-effector apparatus for maintaining redox homeostasis. Physiol Rev (2018) 98(3):1169–203. doi: 10.1152/physrev.00023.2017
43. Su L, Jiang X, Yang C, Zhang J, Chen B, Li Y, et al. Pannexin 1 mediates ferroptosis that contributes to renal Ischemia/Reperfusion injury. J Biol Chem (2019) 294(50):19395–404. doi: 10.1074/jbc.RA119.010949
44. Zhou M, He SJ, Liu W, Yang MJ, Hou ZY, Meng Q, et al. Ezh2 upregulates the expression of Mapk1 to promote intervertebral disc degeneration Via suppression of mir-129-5p. J Gene Med (2022) 24(3):e3395. doi: 10.1002/jgm.3395
45. Zhu S, Zhang Q, Sun X, Zeh HJ 3rd, Lotze MT, Kang R, et al. Hspa5 regulates ferroptotic cell death in cancer cells. Cancer Res (2017) 77(8):2064–77. doi: 10.1158/0008-5472.Can-16-1979
46. Tang D, Chen X, Kang R, Kroemer G. Ferroptosis: Molecular mechanisms and health implications. Cell Res (2021) 31(2):107–25. doi: 10.1038/s41422-020-00441-1
47. Guo W, Wu Z, Chen J, Guo S, You W, Wang S, et al. Nanoparticle delivery of mir-21-3p sensitizes melanoma to anti-Pd-1 immunotherapy by promoting ferroptosis. J Immunother Cancer (2022) 10(6). doi: 10.1136/jitc-2021-004381
48. Gao D, Huang Y, Sun X, Yang J, Chen J, He J. Overexpression of c-jun inhibits erastin-induced ferroptosis in schwann cells and promotes repair of facial nerve function. J Cell Mol Med (2022) 26(8):2191–204. doi: 10.1111/jcmm.17241
49. Lei M, Wang K, Li S, Zhao K, Hua W, Wu X, et al. The c-jun signaling pathway has a protective effect on nucleus pulposus cells in patients with intervertebral disc degeneration. Exp Ther Med (2020) 20(5):123. doi: 10.3892/etm.2020.9251
50. Yang YC, Zhang MY, Liu JY, Jiang YY, Ji XL, Qu YQ. Identification of ferroptosis-related hub genes and their association with immune infiltration in chronic obstructive pulmonary disease by bioinformatics analysis. Int J Chron Obstruct Pulmon Dis (2022) 17:1219–36. doi: 10.2147/copd.S348569
51. Yang M, Chen P, Liu J, Zhu S, Kroemer G, Klionsky DJ, et al. Clockophagy is a novel selective autophagy process favoring ferroptosis. Sci Adv (2019) 5(7):eaaw2238. doi: 10.1126/sciadv.aaw2238
52. Li Y, Liu S, Pan D, Xu B, Xing X, Zhou H, et al. The potential role and trend of Hif−1α in intervertebral disc degeneration: Friend or foe? (Review). Mol Med Rep (2021) 23(4). doi: 10.3892/mmr.2021.11878
53. Lan T, Hu Z, Guo W, Yan B, Zhang Y. Development of a novel inflammatory-associated gene signature and immune infiltration patterns in intervertebral disc degeneration. Oxid Med Cell Longev (2022) 2022:2481071. doi: 10.1155/2022/2481071
Keywords: lower back pain, intervertebral disc degeneration, nucleus pulposus, ferroptosis, oxidative stress
Citation: Xiang Q, Zhao Y and Li W (2023) Identification and validation of ferroptosis-related gene signature in intervertebral disc degeneration. Front. Endocrinol. 14:1089796. doi: 10.3389/fendo.2023.1089796
Received: 04 November 2022; Accepted: 04 January 2023;
Published: 06 February 2023.
Edited by:
Shibao Lu, Xuanwu Hospital, Capital Medical University, ChinaReviewed by:
Chensheng Qiu, Medical College of Wisconsin, United StatesCopyright © 2023 Xiang, Zhao and Li. This is an open-access article distributed under the terms of the Creative Commons Attribution License (CC BY). The use, distribution or reproduction in other forums is permitted, provided the original author(s) and the copyright owner(s) are credited and that the original publication in this journal is cited, in accordance with accepted academic practice. No use, distribution or reproduction is permitted which does not comply with these terms.
*Correspondence: Weishi Li, cHVoM2xpd2Vpc2hpQGJqbXUuZWR1LmNu
†These authors have contributed equally to this work
Disclaimer: All claims expressed in this article are solely those of the authors and do not necessarily represent those of their affiliated organizations, or those of the publisher, the editors and the reviewers. Any product that may be evaluated in this article or claim that may be made by its manufacturer is not guaranteed or endorsed by the publisher.
Research integrity at Frontiers
Learn more about the work of our research integrity team to safeguard the quality of each article we publish.