- 1The Hormel Institute, University of Minnesota, Austin, MN, United States
- 2Department of Biochemistry and Pharmacology, Universidade Federal do Piaui, Piauí, Brazil
Heterogeneity is a complex feature of cells and tissues with many interacting components. Depending on the nature of the research context, interacting features of cellular, drug response, genetic, molecular, spatial, temporal, and vascular heterogeneity may be present. We describe the various forms of heterogeneity with examples of their interactions and how they play a role in affecting cellular phenotype and drug responses in breast cancer. While cellular heterogeneity may be the most widely described and invoked, many forms of heterogeneity are evident within the tumor microenvironment and affect responses to the endocrine and cytotoxic drugs widely used in standard clinical care. Drug response heterogeneity is a critical determinant of clinical response and curative potential and also is multifaceted when encountered. The interactive nature of some forms of heterogeneity is readily apparent. For example, the process of metastasis has the properties of both temporal and spatial heterogeneity within the host, whereas each individual metastatic deposit may exhibit cellular, genetic, molecular, and vascular heterogeneity. This review describes the many forms of heterogeneity, their integrated activities, and offers some insights into how heterogeneity may be understood and studied in the future.
Introduction
Endocrine resistance is a major clinical problem for the treatment of hormone-receptor (HR+) positive breast cancer (BC). HR+ tumors comprise the most prevalent molecular subtype, representing over 70% of all newly diagnosed breast cancers each year. However, using endocrine monotherapies to improve the overall survival (OS) rates for patients with HR+ breast cancer has shown only modest further increases since the introduction of tamoxifen in the early 1970s. As monotherapies, only Fulvestrant has shown an ability to increase OS; aromatase inhibitors confer no OS benefit relative to tamoxifen (1–3). Adding a CDK4,6 inhibitor such as abemaciclib or ribociclib to any of the current endocrine therapies improves OS beyond that of the endocrine monotherapy (4–6). Nonetheless, many patients experience a recurrence of their HR+ breast cancer, often many years after completing an apparently successful treatment regimen (7). Recurrence is almost always fatal, with the predicted number of breast cancer deaths from all molecular subtypes in the United States alone expected to exceed 42,000 in 2023 (8).
Endocrine therapies that target the activation state of estrogen receptor-α (ER; ESR1) fall into two broadly defined agent classes – antiestrogens and aromatase inhibitors. Antiestrogens act primarily by competing with endogenous 17β-estradiol and other estrogens for binding to ER and blocking its activation. Tamoxifen and structurally related triphenylethylene-like compounds are mostly partial agonists and often described as selective estrogen receptor modulators (SERMs). The ER antagonist properties of tamoxifen are evident in its ability to reduce the risk of breast cancer mortality by almost one-third (7), whereas its agonist properties are largely responsible for the increased risk of endometrial cancer associated with long term use (9). Fulvestrant and related compounds also compete with estrogens for ER binding but can target the receptor protein for degradation and are often referred to as selective estrogen receptor downregulators or degraders (SERDs). The mechanistic importance of ER degradation as a driver of antineoplastic activity, relative to competing for estrogen binding to ER, may be minor (10, 11). Both SERDs and SERMS occupy the ligand binding domain of ER and induce a conformation in the protein that alters the ability of ER to act as a transcription regulator. Aromatase inhibitors act by blocking the enzyme activity of the aromatase protein that converts androgens to estrogens, depriving ER of its activating ligands. Hence, the ER protein remains as a largely inactive transcriptional regulator unless modified by selective mutation or phosphorylation.
Two ligand independent actions can alter ER function – mutation or phosphorylation of specific residues. ER can be phosphorylated at multiple residues, with serine-167 (S-167) and serine-118 (S-118) in the AF1 region of the ER protein being the most prevalent (12). Higher expression of either pS-167 and/or pS-118 is mostly associated with favorable clinical outcome in patients treated with tamoxifen (13–16). Increased expression of p-S118 in secondary breast tumors post relapse may be predictive of longer survival (17). The presence of ESR1 mutations following failure on an aromatase inhibitor-based regimen is widely reported, with 18%-55% of recurrent tumors expressing one or more ER mutations (18–21). Two sites, D538 and Y537, account for most of these ER mutations. Meta-analysis of the effects of ESR1 mutations in breast cancer revealed that D538G is the most frequently detected mutation and is associated with poor relapse free survival. Most of the ESR1 mutations detected were associated with aromatase inhibitor treatment (22). Since the prevalent ESR1 mutations encode a constitutively active ER protein, these mutations appear to drive up to ~40% of aromatase inhibitor resistant tumors. Drivers of the remaining 50-60% of these aromatase inhibitor resistant tumors remain to be discovered. In clinical studies, the benefit of antiestrogen therapy does not appear to be substantially affected by the presence of these ER mutations (23, 24). Unlike aromatase inhibitor therapies, recurrence following an antiestrogen therapy is associated with a relatively small increase in the prevalence of ER mutations (22).
Whether by loss of access to natural ligands as occurs with aromatase inhibition, or as a consequence of binding an antiestrogen, altered ER function directly affects cell fate by compromising two major cell functions – survival and proliferation. The most readily apparent of these changes is an often rapid slowing of proliferation, usually a result of cells accumulating in the G1, or exiting to the G0, phase of the cell cycle. Cell death occurs more slowly. Both changes are observed in endocrine sensitive, HR+ experimental models and in many HR+ breast tumors in patients. For example, in endocrine treated breast tumors reduced proliferation is evident from the drop in expression of the Ki67 proliferation marker (25, 26), whereas tumor shrinkage reflects increased cell death. Not surprisingly, these changes occur on different time scales, with changes in Ki67 detected within days and tumor shrinkage often occurring over many weeks or months (26, 27). Unlike the rapid induction of cell death that can occur with chemotherapy and often leads to a complete pathological response (pCR) that predicts a good clinical response, the much slower induction of cell death and tumor shrinkage that accompany endocrine therapies rarely lead to a rapid pCR (28, 29). Nonetheless, both cytotoxic and endocrine interventions confer a broadly comparable increase in OS (28, 30), most likely reflecting the ability of each modality to increase the rate of breast cancer cell death within tumors.
The time scales for cell death and tumor shrinkage are likely further affected by the cell populations targeted by each type of intervention. For example, cytotoxic chemotherapy may kill proliferating infiltrating immune cells and cancer associated fibroblasts (CAFs) in the tumor microenvironment (TME) that are often ER-negative and likely not killed by endocrine therapies. While cellular heterogeneity can be one determinant of responsiveness to any given therapy, heterogeneity is complex and multifaceted.
The complex nature of heterogeneity
Breast tumors and their associated microenvironments are often described as being highly heterogeneous. Generally, this is taken to reflect cellular heterogeneity, although the precise nature of the heterogeneity invoked may not be defined explicitly. Cellular heterogeneity usually refers to the presence of different cell types within the TME and exhibits both spatial and temporal variability within an individual tumor and among different colonized sites in the same patient (primary tumor and distant metastatic sites). While tumor cells may remodel some features of their microenvironment to create a supportive niche at a site distant from the primary tumor, the microenvironment of each metastasis will reflect, to some degree, the normal tissue in which it resides. In breast cancer, common metastatic sites include bone, brain, lung, lymph nodes, skin, and viscera. Location in the body creates one form of spatial heterogeneity (Figure 1A). Each metastasis arises over time, conferring a form of temporal heterogeneity (Figure 1B).
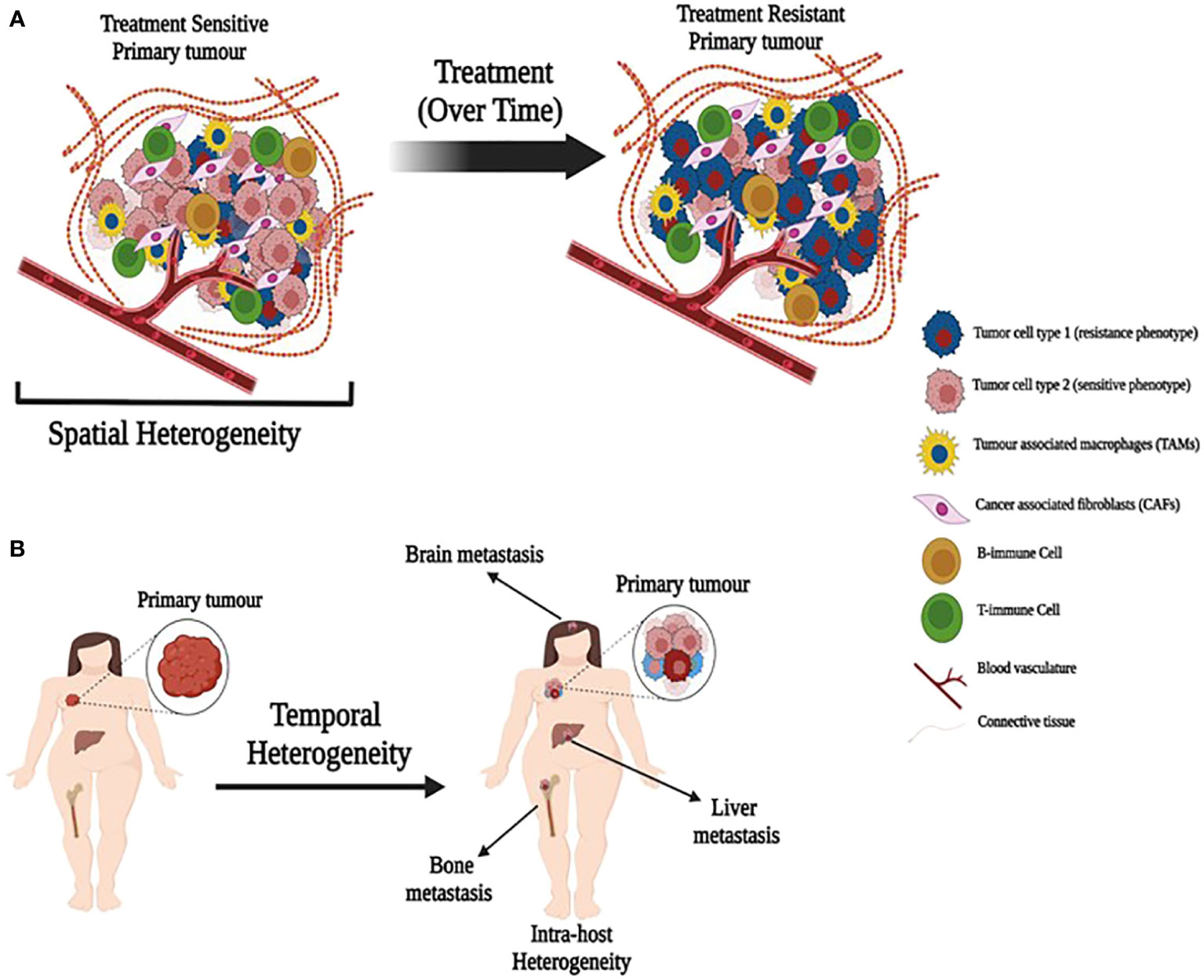
Figure 1 Illustration depicting spatial and temporal heterogeneity. (A) A primary tumor with heterogeneous population of treatment sensitive and resistant cancer cells along with tumor associated cells. Treatment over time may allow expansion of resistant cells. (B) Primary tumors metastasize over time to produce metastatic tumors in different organs with different cell composition (temporal heterogeneity) that may also lead to intra-host heterogeneity.
Cellular heterogeneity reflects the presence of cancer cells, normal and/or stromal cells such as non-transformed adipocyte, epithelial, myoepithelial, fibroblast and myofibroblast cells, and infiltrating immune cells including various T-cells, macrophages, dendritic and natural killer (NK) cells. As definitively normal cells, their genome sequence profiles would be expected to be similar. However, each of these cell types has a different phenotype and function and will also express different transcriptome, proteome, metabolome and epigenome profiles (molecular heterogeneity). These various profiles also exhibit dynamism (creating another form of temporal heterogeneity) as each cell type responds to the changing extrinsic signals it receives and the intrinsic responses and functions it performs in response. For example, macrophages in the TME may exist in different states that can be M1-like (pro-inflammatory, anti-tumorigenic) or M2-like (anti-inflammatory, pro-tumorigenic) (31, 32). Hence, there is significant spatiotemporal molecular heterogeneity in the TME, reflecting the location of each cell type and its immediate neighbors and their respective interactions and cell states.
The tumor cell compartment of the TME also exhibits spatiotemporal heterogeneity in its genome, epigenome, transcriptome, and proteome profiles. The genetic heterogeneity from acquired mutations is inherent in cancer, with different cancer cells exhibiting some features of their genetic ancestry in a manner that allows investigators to follow the genetic evolution of the cancer cells in a tumor (33). These various features of heterogeneity are affected further by the nature of the tumor vasculature, which creates additional forms of heterogeneity because of its variable ability (or inability) to deliver adequate nutrients and remove waste throughout a tumor ecosystem. Vascular heterogeneity can create areas of inadequate oxygen (and nutrient) supply leading to intratumoral regions of hypoxia and anoxia, causing affected cells to stop proliferating or, in more extreme cases, die (regions of necrosis). Vascular heterogeneity also can affect drug delivery, with the inability to deliver cytotoxic concentrations of a drug to all tumor cells in a manner that contributes to drug response heterogeneity. Drug response heterogeneity also is complex and can reflect a mix of activities that are intrinsic and/or extrinsic to the cancer cells.
Since it is not possible to cover in depth all forms and mechanisms of heterogeneity, we provide insights mostly from the perspective of responsiveness to therapeutic interventions and the regulation of breast cancer cell fate. For the purposes of this review, we consider cell fate as the balanced outcomes among cell survival-death and proliferation-growth arrest. Readers interested in more in-depth reviews may find the following citations of interest; these address heterogeneity in the context of metastasis (34–36) and the interaction of immune and cancer cells in the TME (37), often from the common perspective of effects on drug responsiveness (38–41).
Cellular heterogeneity - the tumor microenvironment
One of the clearest examples of cellular heterogeneity is within the TME, which plays a potentially multifaceted role, in collaboration with the extracellular matrix (ECM), in therapy resistance acquisition (42–46). As noted above, the TME comprises a variety of components such as vascular endothelial cells, adipocytes, pericytes, stroma/stem-like cells (MSCs) and extracellular matrix. The TME is subject to dynamic turnover that produces both spatial and temporal intra- and inter-tumor heterogeneity that can directly influence drug responsiveness. In this section, we consider the mechanisms used by TME components to modify endocrine responses in ER+ breast cancer cells.
Breast cancer-associated fibroblasts
Cancer-associated fibroblasts (CAF) are among the most important components of the stromal cell population within the TME, and have been shown to participate in many features of cancer progression including altered drug responsiveness (Figure 2). CAF subpopulations in breast cancer express different markers such as α-SMA, FAP, PDGFRα, PDGFRβ, CD29, NG2, FSP1, vimentin, PDPN (47–50), CD146 (51) and CAV1 (52). Other markers include the more recently implicated GPR77 and CD10 (53), CX chemokine ligand 12 (CXCL12) (54), MHC-II gene and CD74 (55), Fibulin-1 and SGRG-1 (56); CDK1, CD53 and CRABP1 (55). These markers define heterogeneous subsets of CAFs from different origins (myCAFs, iCAFs, apCAFs, vCAFs, mCAFs and developmental CAFs) that can present different and contrasting roles in breast cancer [for review see (57)], including promotion of chemoresistance or sensitivity. Besides heterogeneity in marker expression, origin and function, the localization of subsets of these cells in the TME also contributes to CAF heterogeneity and can affect their role in cancer progression. Applying simultaneous detection of the vCAF marker Nidogen-2, the mCAF marker PDGFRα, and the dCAF marker SCRG1 identified three distinct stromal populations with divergent growth patterns and localization in relation to the nests of breast tumor cells (56).
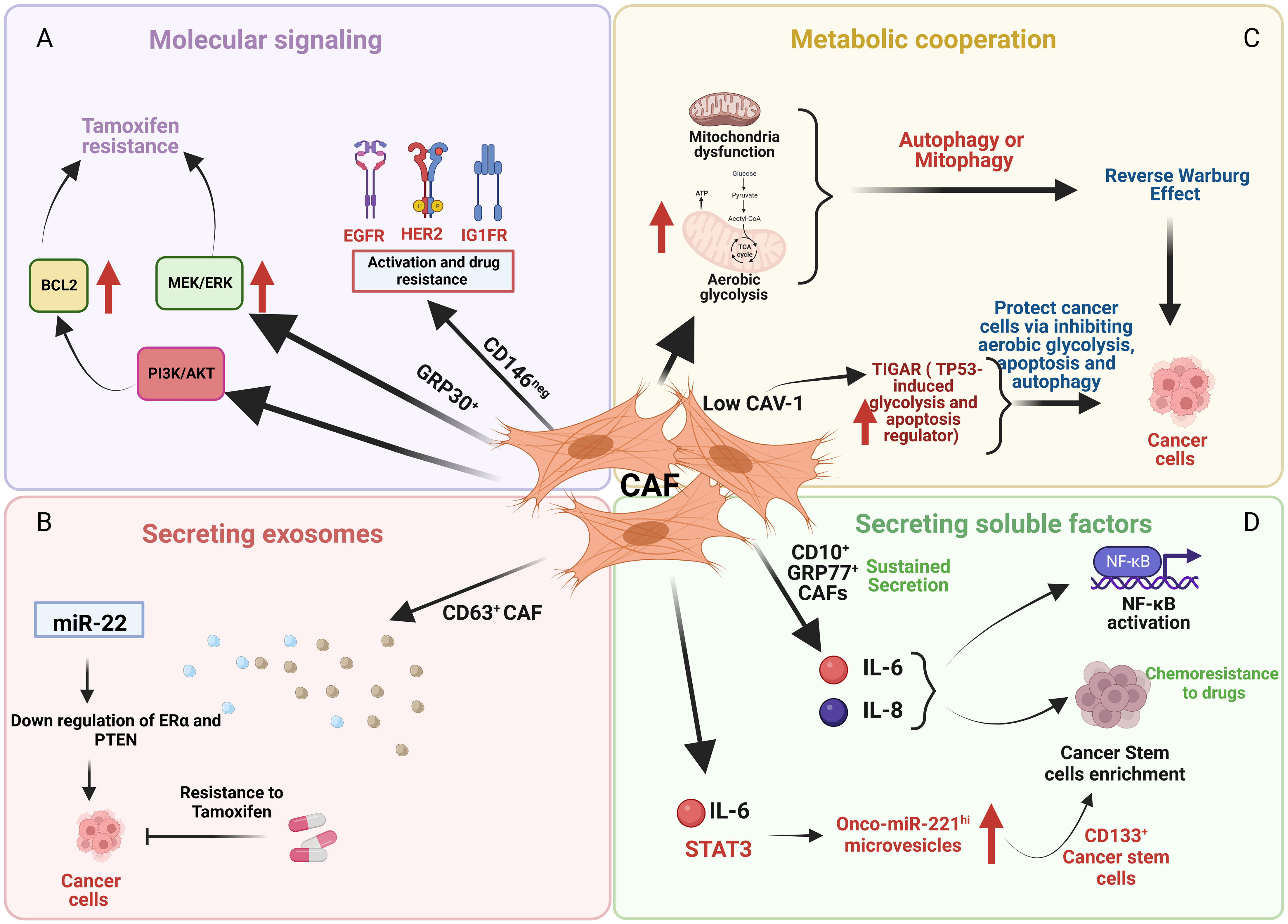
Figure 2 An illustration depicting the effects of cancer associated fibroblasts (CAF) cells on cancer cells through various mechanisms. (A) CD146 negative (CD146neg) CAF activate EGFR, HER2, and IGFR in breast cancer cells that promotes tamoxifen resistance whereas GPR30 positive (GPR30+) CAF cells induce tamoxifen resistance by upregulating MEK/ERK and PI3K/AKT signaling. (B) CD63 positive (CD63+) CAF promote tamoxifen resistance by downregulating ER and PTEN mediated by exosomes containing miR22. (C) Low caveolin-1 (CAV-1) expression in CAF upregulates TIGAR that protects cancer cells by inhibiting aerobic glycolysis, apoptosis and autophagy. (D) CD10 positive (CD10+) and GPR77 positive (GPR77+) CAF induces chemoresistance by sustained secretion of IL-6 and IL-8 and enrichment of stromal cancer stem cells.
Mechanisms of CAF-induced endocrine resistance in breast cancer
CAFs use different/heterogeneous mechanisms to drive ER+ breast cancer cells to acquire endocrine resistance. Mechanisms of the activated TME that can induce therapy resistance include promotion of pro-survival pathways, stemness traits, and/or metabolic reprogramming; CAFs can regulate each of these mechanisms to induce endocrine resistance in ER+ breast cancer cells.
Examples of different CAF subsets regulating several pro-survival mechanisms are evident. For example, CD146 expression defines two subsets of CAFs, CD146-positive (CD146pos) and CD146-negative (CD146neg) in luminal breast cancer (Figure 2A) (51). In these breast tumors, CD146neg fibroblasts exhibit decreased ER expression, whereas ER+/CD146pos fibroblasts remained estrogen responsive and antiestrogen sensitive. Breast cancer cells influenced by CD146neg fibroblasts may escape estrogen-dependent proliferation and exhibit tamoxifen resistance through activation of EGFR, HER2, and IGF1R (51). Weigel et al. (2012) implicated a role for enhanced platelet-derived growth factor receptor (PDGF)/Abl signaling in aromatase inhibitor-resistant breast cancers (58). Both tumor and fibroblast expression of PDGFRα and PDGFRβ was significantly correlated in pre-treatment and relapse samples and high post-treatment tumor and fibroblast PDGFRβ levels were associated with a short time to treatment failure (TTF).
GPR30 (G-protein receptor 30)-expressing CAFs induce tamoxifen resistance involving MEK/ERK signaling in ERα-positive breast cancer cells and tumors (Figure 2A) (59). CD63+ CAFs may promote tamoxifen resistance by secreting exosomes rich in miR-22, which can induce downregulation of ER and PTEN, to confer tamoxifen resistance on breast cancer cells (Figure 2B) (60).
Several other studies reported the induction of pro-survival signaling through PI3K/AKT and MEK/ERK by CAFs to promote endocrine resistance (61–63). For example, activation of the PI3K/AKT pathway that phosphorylates S-167 of ER increased BCL2 expression and altered resistance to tamoxifen following activation of fibroblast growth factor receptor 2 (FGFR2). These activities reversed tamoxifen-driven ER stabilization and promoted ER phosphorylation and proteasomal degradation (61).
Activated signaling through PI3K/AKT/mTOR is often driven by mutations in either AKT and/or PIK3CA (64–66) and is clinically actionable with drugs that target each of these signaling nodes. Several clinical trials with these drugs have been completed and others are ongoing; none has yet produced any notable increase in OS. While some studies show improvements in the clinical benefit ratio, these are often associated with significant dose limiting toxicities (see (67) for review). A major challenge is feed-back activation within the signaling feature when one node is inhibited, making shutting down the signaling more difficult. While novel drug combinations and scheduling could mitigate some of this feedback activation, these are likely also to be accompanied by significant toxicity for patients. Moreover, the potential of cellular or molecular heterogeneity to further limit responsiveness is likely to remain a challenge. While patients can be selected for specific drugs based on the AKT and/or PIK3CA mutational profile of their tumor, it remains difficult to predict which tumors are driven by the detected mutation, perhaps reflecting the difficulty in predicting the true penetrance of the mutation (where the presence of the mutation is responsible for the observed tumor phenotype).
Examples of metabolic cooperation between CAFs and tumor cells that can alter endocrine responsiveness include the loss of stromal caveolin-1 (Cav-1), a predictive marker of poor clinical outcome in breast cancer patients treated with tamoxifen (68). Martinez-Outschoorn et al. showed that mitochondrial dysfunction, oxidative stress and aerobic glycolysis are increased in CAV1-downregulated CAFs (69). The authors proposed that defective mitochondria are removed from these cells by the autophagy/mitophagy induced by oxidative stress. These autophagic processes could provide nutrients (such as lactate) to stimulate mitochondrial biogenesis and oxidative metabolism in adjacent cancer cells. Martinez-Outschoorn et al. showed that low CAV-1 CAFs, after treatment with tamoxifen, increased TIGAR (TP53-induced glycolysis and apoptosis regulator) expression (Figure 2C) (70). TIGAR is a p53-regulated gene that simultaneously inhibits glycolysis, autophagy and apoptosis and decreases ROS, thereby promoting oxidative mitochondrial metabolism in ER+ breast cancer cells. CAFs showed increased glucose uptake and glycolytic activity, impaired mitochondrial function, and increased generation of lactate and ketone bodies; conversely, epithelial cancer cells in coculture display increased mitochondrial activity and protection from apoptosis (70).
CAF subpopulations can promote breast cancer stemness and treatment resistance including resistance to endocrine therapies. CD10+ GPR77+ CAFs induced chemoresistance via sustained secretion of IL-6 and IL-8 (Figure 2D) (53). Sansone et al. determined that the IL6-pStat3 pathway (required for CAF proliferation) promoted the biogenesis of onco-miR-221hi CAF microvesicles (MV) which, in combination with hormone therapy, established stromal cancer stem cell (CSC) niches, specifically those with CD133hi cells (Figure 2D) (71).
Targeting CAF heterogeneity and their different molecular mechanisms could prevent or reverse resistance to endocrine therapies and suggests one approach for the development of more effective therapeutic interventions.
Tumor associated macrophages
Macrophages are abundant in the TME (72). The role(s) of tumor-associated macrophages (TAM) in the pathogenesis of cancer depend on their phenotype and polarization (31), which can be affected by the TME (73, 74). The importance of TAM localization within different tumor compartments has been reviewed by others (31). TAM phenotypes are often characterized as either M1, which promotes proinflammatory and tumoricidal responses, or M2, with anti-inflammatory and pro-tumor responses (32). Markers of M1 TAM include HLA-DR and CD80/86; M2 markers include CD206, CD163, CD204, and stabilin-1. In breast cancer, the M2 markers CD47, COX-2, MMP9, TIE2, YKL-39, YKL-40, PD-L1 were also identified (31). TAMs can play a role in endocrine resistance; for example, macrophages may induce endocrine resistance in ER+ breast cancer cells by inducing sustained release of TNF-α and IL-6 from breast cancer cells, resulting in activation of NFκB, STAT3, and ERK-1 and hyperphosphorylation of ERα (75). Formation of an NFκB/STAT3/phospho-ER complex in cyclin D1 gene correlated with increased proliferation, independent of ER ligand status (75).
Breast cancer stem cells
Cancer stem cells are a population of tumor cells with the capacity to self-renew and to generate more stem cells and also to differentiate into other cell types (76). Breast cancer stem cells contribute to breast cancer heterogeneity, expressing a highly diverse profile of markers in different tumor subtypes ( (77) for Review). Markers reported to be associated with the ER-positive, Luminal A and/or Luminal B, subtype include: CD44+/CD24-/low, MUC1+/CD24+, CD44+/Vimentin+, CD44+/Osteonectin+, CD24+/CK18+, CD24+/GATA+ (50). The marker ALDH1 has the highest expression in HER2+ and basal-like tumors (77).
While playing a role in breast cancer initiation and growth, evidence of the role of breast cancer stem cells in metastasis (78) and therapeutic resistance (79) has also been reported. Simoes et al. showed that both tamoxifen and fulvestrant induce STAT3 phosphorylation and activation of the STAT3 target genes MUC1 and OSMR in ALDH+ cells from endocrine-resistant patient samples (80). The SOX2 pathway, which is associated with stem cell characteristics and involved in embryonic development (81), and the Wnt pathway that is associated with the epithelial to mesenchymal transition (82), were both activated after endocrine treatment of ER+ breast cancer cells. Activation was accompanied by an increase in CD24 but not CD44 expression. Together, these observations suggest an early adaptation to endocrine stress with increased stemness that enables the survival of emerging hormone-resistant cell populations (83).
The Notch pathway can regulate breast cancer stem cell activity (84, 85) and has been implicated in endocrine therapy resistance (86–88). FK506-binding protein like (FKBPL), an anti-tumour protein that belongs to the family of immunophilins, was shown to inhibit endocrine therapy resistant CD44+ cancer stem cells in ER+ disease, via modulation of the components of the Notch pathway, DLL4 and Notch4 (89). Liu et al. showed that everolimus, a mTOR antagonist, in combination with letrozole inhibited MCF-7 ESA(+)CD44(+)CD24(-/low) stem cells via PI3K/mTOR signaling (78).
Molecular heterogeneity
The evolution of clonal tumor cell populations alters intratumor heterogeneity spatially and/or temporally. Earlier studies have indicated intratumoral heterogeneity of breast cancer biomarkers, such as ER, PR, and HER2 (90–92). Studies using large scale genome analysis have mapped the complex mutational landscape and confirmed the presence of genetic clonal subpopulations in breast tumors (93–98). Typically, single biopsies are used to determine the features of a tumor. However, single biopsies are temporally and spatially restricted because the cells collected often represent a small fraction of the tumor composition present at a single time point. Therefore, a biopsy may not capture the entire diversity of tumor cell populations and can fail to reflect fully spatial heterogeneity. For example, two different tumor cell populations can exist spatially as ‘collision tumors’; these may only be observed when the entire tumor is analyzed, such as after surgical resection. Indeed, when the clonal architecture of cancer cells was monitored after four months of neoadjuvant aromatase inhibitor therapy, tumors of independent origin were found that were not captured in the baseline biopsy (99).
One approach to overcome this challenge is to perform multiregion sequencing of biopsies from the same tumor. For example, multiregion sequencing from 8 biopsies of single treatment naïve breast tumor found subclonal diversification and geographically constrained patterns of subclonal growth leading to extensive and statistically significant spatial heterogeneity of point mutations (100). Due to geographically restricted subclonal growth, in 4 cancers (out of 12), the detected driver mutation was present only in 1-3 samples from 8 needle biopsies (100). The challenge of both spatial and molecular heterogeneity in subpopulations requires careful consideration when searching for newly detected mutations after treatment, especially if a single needle biopsy was used to determine heterogeneity at baseline. Recent studies have confirmed that most ductal carcinoma in situ (DCIS) lesions that give rise to recurrent invasive breast cancer share some clonal relationship with the initial DCIS (101). Any treatment that fails to eliminate all driver clones in DCIS will likely lead to recurrence, independent of how effective the treatment may appear when tumor shrinkage is used as the marker of response. Normal mammary cells associated with tumors in breast cancer patients may also harbor pathogenic variants of p53 and PIK3CA (102), suggesting that clonal (and genetic/molecular) heterogeneity may be a fundamental feature of mammary cells.
Genetic heterogeneity
Cancer conforms to evolutionary rules defined by the rates at which clones mutate, adapt, and grow. Acquired gene mutations are inherent for cancer cells, where some gene mutations are defined as driver mutations that have known functions capable of conferring survival and proliferation advantages (103, 104). Tumors contains different subpopulations of cells (subclones) that can be distinguished based on different characteristics affecting their phenotype including genetic alterations such as single-nucleotide variants (SNVs), small insertions or deletions (indels), somatic copy number alterations (CNAs), and structural variants. Chromosomal and genomic alterations have been documented in most cancers including breast cancers (98, 105, 106), some of which clearly affect biological processes and functions (107) that may facilitate acquired resistance to specific therapeutic interventions (108). Analysis of the whole genome sequences of 560 breast cancer patients revealed 93 protein-coding genes that contained probable driver mutations (109). The ten most frequently mutated driver genes accounted for 62% of all driver genes and included TP53, PTEN, ERBB2, FGFR1 locus, GATA3, RB1, and MAP3K1. TP53, RB1, and PTEN were more frequently found in ER negative breast cancers. PIK3CA, CCND1, and FGR1 mutations were more frequently detected in ER+ breast cancers (109). Other studies have also reported alterations in these genes in breast cancer (98, 110). Metastatic breast cancers frequently share the majority of their genomic alterations with the corresponding primary disease, indicating pre-existing clones. However, metastases at distant sites also continue to acquire new mutations that were not previously detected or are subclonal in the primary disease (110, 111). For example, ESR1 activating mutations are rarely present in primary ER+ breast cancer, even in those 15–20% of patients that show intrinsic resistance to hormonal therapies (112). However, these mutations are enriched in ER+ metastatic tumor samples from patients with acquired endocrine resistance (30–40% of ER+ patients), mostly following failure of an aromatase inhibitor-based intervention (113). International Cancer Genome Consortium (ICGC) and Cancer Genome Atlas (TCGA) have thoroughly explored the genetics of primary breast cancer and sequencing of breast cancer samples. These studies revealed nine cancer genes (TP53, ESR1, GATA3, KMT2C, NCOR1, AKT1, NF1, RIC8A and RB1) to be highly mutated in the metastatic disease when compared with early breast cancer (114). Importantly, genomic comparisons of primary and metastatic samples showed that metastatic breast tumors frequently possess higher numbers of mutations (mutational load), including driver mutations and somatic copy number aberrations, than matched primary tumors (115). Some of these mutations are associated with endocrine therapy response (110, 116, 117). For instance, the prevalence of activating ERBB2 mutations and NF1 loss of function increased two-fold in endocrine resistant tumors; regulators of ER mediated transcription were also enriched in endocrine therapy resistant tumors (117).
Transcriptional heterogeneity
Transcriptional heterogeneity plays a key role in the development of different cell states. Different transcriptional programs contribute to dynamic plasticity and allow co-existence of tumor cells with differential drug sensitivities that may support the development of resistance to specific therapies (118). Earlier studies in lung cancer cells showed that in a drug sensitive cell population a small proportion of cells are reversibly drug resistant because of chromatin mediated signaling (119). Using single cell RNA-seq analysis, the transcriptomic heterogeneity of different type of cells in tumors has been cataloged (120). Profiling tumors from 11 breast cancer patients using single cell RNA-seq reported both common and diverse transcript signatures from cancer cells of the same tumor (121), whereas most non-cancer cells were immune cells. This study further revealed that in the ER+/HER2+ breast cancer subtype, the primary tumors showed predominant ER signaling but cells from the metastatic lymph nodes expressed mostly HER2-activated genes. Conversely, neoadjuvant treatment of HER2+ breast cancer changed the gene expression profile of the tumor to a pattern that resembled triple negative breast cancer (121). Thus, both disease progression and treatment can contribute to temporal transcriptional heterogeneity.
Single cell studies have revealed that transcript heterogeneity also exists in commonly used breast cancer cell lines. In MCF7 cells, intracellular transcriptomic heterogeneity was dominated by cell cycle states but a rare subpopulation of MCF7 cells showed a unique transcriptional feature with an apoptotic signature (122). Using single cell transcriptomics, spatially resolved data identified cell modules with different activated pathways in a single tumor. Pockets of basal neoplastic cells in tumors were detected in tumors characterized as ER+ positive breast cancer with bulk sequencing (123). In addition, a lobular carcinoma with low cellularity for neoplastic cells was misassigned as ‘normal-like’ by bulk sequencing (123).
Extensive transcriptional heterogeneity was reported in triple negative breast cancers (TNBC), with certain subpopulation of cells showing a highly malignant gene signature (124). A minor breast cancer cell population with mesenchymal properties could immunosuppress the TME and help the entire tumor to evade immunotherapy (125). Existence of subclonal populations in a primary TNBC tumor may be responsible for metastatic dissemination and seeding of cells in distant organs (126). Using primary and its paired metastatic cells coupled with single cell sequencing of three TNBC patient derived xenografts, metastatic cells were found to be proficient in oxidative phosphorylation, whereas its matched primary tumor cells were dependent on glycolysis (127).
High intercell variability at the transcript level may be responsible for metastasis and resistance to chemotherapy that can be attributed to differences in splicing machinery (128). In ER+ breast cancers, development of resistance to endocrine therapy was found to be gradual and required multistep adaptation (129). Using single cell methods the pre-existence of therapy resistance subclones defined by distinct transcript profile of single cells may be unlikely (129). In a recent report that studied serial biopsies using single cell RNA sequencing from early stage ER+ breast cancer tumors treated with AI therapy plus CDK4/6 inhibitors, results revealed an emergence of common resistant phenotype that showed loss of estrogen signaling and upregulation JNK signaling (130).
While the use of single cell RNA sequencing has begun to offer new insights into cellular and molecular heterogeneity, many studies inadequately consider the limitations of current technologies. Few methods are yet able to sample more than ~50% of the entire transcriptome, leaving many RNA species undetected. Leveraging bulk sequencing data on the same samples may increase the ability to see further into the transcriptome. While this limitation is clearly problematic for studies into mechanism, it is less challenging for biomarker and similar studies where the entire transcriptome does not need to be sampled adequately.
Metabolic heterogeneity
Metabolic reprogramming is a hallmark of cancers that progress (131, 132) and drives resistance to therapies including endocrine-based interventions (133). However, all tumors or tumor cells may not show the same metabolic adaptations and may exhibit heterogeneous metabolic phenotypes and metabolic plasticity (134, 135). For example, different anatomic sites show different metabolic dependencies. Glucose oxidation was dominant in brain and lung tumors, but glycolytic intermediates were elevated in renal cancer cells, where glucose oxidation was suppressed (136–138). Conversely, different subtypes of breast cancer show different dependencies for glutamine. Generally, luminal breast cancer cells can resist glutamine deprivation but basal cells are dependent on external glutamine (139). However, glutamine is required for proliferation in advanced HR+ breast cancer cells (140, 141). Differences in nutrient and oxygen availabilities impacts metabolic dependencies. Vascular heterogeneity causes oxygen gradients in tumors that can render the environment hypoxic for those tumor cells distant from blood vessels (142). Hypoxic conditions activate hypoxia inducible factors (HIFs), while in the presence of sufficient oxygen the proto-oncogene c-Myc may drive a transcriptional program that promotes uptake of nutrients and increases the rate of glycolysis (142). Indeed, c-Myc is involved in glucose and glutamine uptake in endocrine therapy resistant cells and promote cell proliferation (143, 144). HIF1-alpha is also involved in endocrine therapy resistance by stimulating the expression of various glycolytic enzymes in endocrine therapy resistance (145–148).
Drug response heterogeneity
The ability of a systemic therapy to eradicate a tumor and its metastases is largely dependent on the ability to overcome the heterogeneity of responses in individual cells. Drug response heterogeneity is multifactorial and reflects the combined effects of many of the other forms of heterogeneity discussed above. Cytotoxic drugs generally target cellular features or functions associated with replication, e.g., DNA synthesis (drugs that include alkylating agents, antimetabolites, anthracyclines) or the dynamics of microtubule turnover and their function during mitosis (vinca alkaloids destabilize and taxanes stabilize microtubule dynamics).
Cancer cell replication in solid tumors is asynchronous, with many cells arrested in G0/G1 and so insensitive to drugs that target the replication machinery while the cells remain growth arrested. This form of resistance is often referred to as ‘kinetic resistance’. Some cells may remain arrested for a sufficient period that the drug target is released from its inhibition before the cells receive and respond to a mitogenic stimulus. Cells can then enter S-phase with the ability to complete the cell cycle and continue to proliferate.
Endocrine therapies are also affected by kinetic resistance, although the response pattern can differ from that seen with cytotoxic drugs. In ER+ breast tumors, a drop in proliferation as induced by an endocrine therapy and reflected by an often dramatic reduction in Ki67 expression (149, 150), can predict a good clinical response (151). Since endocrine therapies are usually given daily for 5-10 years (152), the growth inhibitory activities that arrested cells into G1 may further drive them into G0, effectively taking them out of the cell cycle for prolonged periods. This prolonged growth arrest may allow cells that do not undergo cell death in response to the stress of an endocrine therapy to rewire their signaling and cellular functions to ensure that they survive but remain growth inhibited (23). Rewiring is likely epigenetically maintained (and so reversible) enabling cells to re-enter the cell cycle when conditions are favorable and appropriate mitogenic signals are received and executed. The dormancy strongly associated with ER+ breast cancer (153–155) is one reflection of these types of events, as is the response reported to some therapeutic regimens that include an inhibitor of enzymes that induce and maintain epigenetic modifications (156, 157).
The molecular and genetic heterogeneity within cancer cells also contributes to drug response heterogeneity. For example, not all cells in an ER+/HER2+ tumor concurrently express both functional ER and HER2, yet drugs that target each can induce significant clinical benefit. Nonetheless, all ER+/HER2+ tumors are not cured with combined anti-ER and anti-HER2 interventions and there can be some cells in these tumors that may express neither ER nor HER2. Genetic heterogeneity, when applied to an actionable mutation in genes such as PIK3CA (Alpelisib) or AKT (Capivasertib), likely contributes at least partly to the inability of drugs targeting these mutations to yet show a major improvement in overall survival (158–162). Since not all cells express the mutated gene, and some may express the mutation but are no longer driven by its signaling, many tumors may exhibit a phenotype where the penetrance of the mutation may appear low. For example, some tumors with a PIK3CA mutation many not exhibit endocrine resistance and/or respond to a PIK3CA inhibitor.
One of these drug response modifying features is the vascular heterogeneity that is often a significant feature of breast and other solid tumors. Systemically administered drugs must reach the cells that express their molecular targets, and do so in sufficient concentrations to kill the cancer cells. Vascular heterogeneity can lead to different perfusion gradients in tumors, where some regions may receive a cytotoxic level of a drug and others do not - also creating a form of spatial heterogeneity. Vascular heterogeneity may induce a multiple drug resistant-like phenotype since inadequate vascularity may prevent many drugs, independent of their chemical structure or mechanism of action, from reaching some areas of a tumor. Regions that are poorly vascularized may also be hypoxic, with induction of hypoxia stress responses already active in surviving cells in a manner that may also confer resistance to some drugs that reach these hypoxic cells (163–165).
Cellular heterogeneity within the TME likely also contributes to drug response heterogeneity. The presence of multiple interacting cell types can alter the drug responsiveness though their ability to communicate and modify cellular and molecular functions. Among the more common mechanisms are paracrine (such as the secretion of growth factors (166–168) or microvesicles (169–172) and juxtacrine (often through gap junctional intercellular communication) (173, 174). Thus, cellular heterogeneity can affect molecular heterogeneity and so also drug response heterogeneity.
Spatial heterogeneity also contributes to drug response heterogeneity. At the macrolevel, different metastatic sites in the same patient may respond differently to a systemic therapy. For example, brain metastases can be difficult to manage because the blood-brain barrier can prevent adequate concentrations of many drugs from reaching brain lesions. It is likely that vascular heterogeneity further compounds this issue, since it can confer some degree of spatial heterogeneity at the microlevel (within a single tumor) as noted above for areas of hypoxia.
The combined features of cellular, molecular, vascular and spatial heterogeneity are often further modified by temporal heterogeneity. For example, many omics data are collected as massively parallel snapshots of the cell population samples (175). Tumor cells and the TME are often in a dynamic state. Tumor cells are often genetically unstable and acquire additional mutations over time. As many solid tumor progress, genetic heterogeneity increases. Since the length of time for the acquisition of new mutations and/or other molecular features differs in cells, we face another temporal feature – biological time. Some breast tumors may grow rapidly, others remain indolent and may grow very slowly. Acquiring new mutations is often a consequence of failure to recognize and repair errors in replication. The probability of acquiring a new uncorrected error is related to the frequency of DNA replication, and so is expected to be greater in rapidly proliferating than growth arrested cells. Hence, differences in biological time, such as the time it takes to acquire new driver mutations, can create spatial heterogeneity (some areas of a tumor or some metastases in a host may grow at different rates and experience differences in biological time). Since biological time is related to the acquisition and loss of biological features/functions, biological time can also create spatial, temporal, genetic, and molecular heterogeneity.
Changes in the TME, including the dynamic influx and activation/inactivation of key immune effector cells, ensure that interactions between the TME and the tumor cell compartment are constantly changing. Over time, some cells acquire the ability to invade locally and eventually leave the primary tumor to seed metastases, such that the genetic, molecular and cellular features of the tumor also change. Each of these, whether alone and/or in combination, may affect the response of a tumor to a drug and may do so differently over time. In this example, time can be temporal or biological, reflecting the evolution of the tumor and its associated TME. Application of a drug can further change these features over time. For example, breast tumors with many growth arrested cells due to an endocrine intervention may be relatively unresponsive due to kinetic resistance. However, once these cells escape inhibition, perhaps with a wave of proliferating cells driving rapid emergence from dormancy (no longer exhibiting kinetic resistance), the same cells may be more responsive to drugs that require cells to be progressing through the cell cycle to be effective.
Pharmacogenetic differences, reflecting the differential expression of isoforms of genes that affect drug metabolism, also can affect drug response heterogeneity. This form of heterogeneity is most evident at the population level, and among individual patients rather than within a tumor and its TME (176–178). Other factors that differ among patients and affect the pharmacokinetics or pharmacodynamics of a drug can also produce drug response heterogeneity but are beyond the scope of this review (179, 180).
Concluding comments and future directions
We have described several of the key forms of heterogeneity and some of their interactions and modifying factors. For example, genetic heterogeneity is one driver of molecular heterogeneity and cellular heterogeneity is one reflection of molecular heterogeneity. A focus on cellular heterogeneity has become common in recent years. However, this seems likely to change as the multifaceted nature of heterogeneity becomes more widely appreciated and studied. Advances in spatial omics, such as single cell DNA and RNA sequencing, are likely to change our understanding of many forms of heterogeneity and their interactions. Nonetheless, current limitations in these new methods are evident, as is often true when a new technology emerges. For example, the depth of omics coverage can be limited for each single cell. In a transcriptome of perhaps 50,000 different transcripts, less than 50% may be detected, unambiguous assignment of sequences to their correct mRNA can be difficult, and noise in the data may be quite high (181, 182). The number of cells that can be studied is limited, often to a few hundred or thousand cell in a tumor cell population of millions (a clinically palpable 1 cm tumor has ~109 cells) (183). All of these cells concurrently reflect the effects of cellular, molecular, genetic, spatial, and temporal heterogeneity in ways that may not be reflected in the population sample as analyzed. Single cell proteomics and metabolomics face similar challenges, where depth, coverage and the ability to unambiguously assign a signal to a single molecular entity may be no better, or perhaps lower, with current technologies (184–186).
Current spatial omics technologies represent only one series of tools with which to explore the complexity of heterogeneity. Given the rapidity of technological advances in general, it is hoped that the current limitations with these tools will soon be overcome. It will be particularly important to constrain the cost and maximize the availability of these and other new technologies not yet brought to market, to allow the full breadth of the research community to have access and so more effectively advance knowledge.
Author contributions
All authors contributed to the writing and editing of this review. All authors contributed to the article and approved the submitted version.
Funding
This work was supported by awardts to R. Clarke from the US Department of Defense Breast Cancer Research Program (CA171885) and from the Jayne Koskinas and Ted Giovanis Foundation and The Hormel Foundation.
Conflict of interest
The authors declare that the research was conducted in the absence of any commercial or financial relationships that could be construed as a potential conflict of interest.
Publisher’s note
All claims expressed in this article are solely those of the authors and do not necessarily represent those of their affiliated organizations, or those of the publisher, the editors and the reviewers. Any product that may be evaluated in this article, or claim that may be made by its manufacturer, is not guaranteed or endorsed by the publisher.
References
1. Goldhirsch A, Wood WC, Gelber RD, Coates AS, Thurlimann B, Senn HJ. Meeting highlights: updated international expert consensus on the primary therapy of early breast cancer. J Clin Oncol (2003) 21(17):3357–65. doi: 10.1200/JCO.2003.04.576
2. Ingle JN. Adjuvant endocrine therapy in postmenopausal breast cancer. Clin Cancer Res (2003) 9(1 Pt 2):480S–5S. doi: 10.1200/JCO.18.01160
3. Mouridsen HT, Robert NJ. Benefit with aromatase inhibitors in the adjuvant setting for postmenopausal women with breast cancer. MedGenMed (2005) 7(3):20.
4. Sledge GW Jr., Toi M, Neven P, Sohn J, Inoue K, Pivot X, et al. The effect of abemaciclib plus fulvestrant on overall survival in hormone receptor-positive, ERBB2-negative breast cancer that progressed on endocrine therapy-MONARCH 2: A randomized clinical trial. JAMA Oncol (2020) 6(1):116–24. doi: 10.1001/jamaoncol.2019.4782
5. Im SA, Lu YS, Bardia A, Harbeck N, Colleoni M, Franke F, et al. Overall survival with ribociclib plus endocrine therapy in breast cancer. N Engl J Med (2019) 381(4):307–16. doi: 10.1056/NEJMoa1903765
6. Byers KF. Ribociclib and abemaciclib: CDK4/6 inhibitors for the treatment of hormone receptor-positive metastatic breast cancer. J Adv Pract Oncol (2021) 12(1):100–7. doi: 10.6004/jadpro.2021.12.1.8
7. Early Breast Cancer Trialists’ Collab Group. Tamoxifen for early breast cancer: an?overview of the randomised trials. Lancet (1998) 351(9114):1451–67. doi: 10.1016/S0140-6736(97)11423-4
8. Siegel RL, Miller KD, Fuchs HE, Jemal A. Cancer statistics, 2022. CA Cancer J Clin (2022) 72(1):7–33. doi: 10.3322/caac.21708
9. Hu R, Hilakivi-Clarke L, Clarke R. Molecular mechanisms of tamoxifen-associated endometrial cancer (Review). Oncol Lett (2015) 9(4):1495–501. doi: 10.3892/ol.2015.2962
10. Patel HK, Bihani T. Selective estrogen receptor modulators (SERMs) and selective estrogen receptor degraders (SERDs) in cancer treatment. Pharmacol Ther (2018) 186:1–24. doi: 10.1016/j.pharmthera.2017.12.012
11. Wardell SE, Yllanes AP, Chao CA, Bae Y, Andreano KJ, Desautels TK, et al. Pharmacokinetic and pharmacodynamic analysis of fulvestrant in preclinical models of breast cancer to assess the importance of its estrogen receptor-alpha degrader activity in antitumor efficacy. Breast Cancer Res Treat (2020) 179(1):67–77. doi: 10.1007/s10549-019-05454-y
12. de Leeuw R, Neefjes J, Michalides R. A role for estrogen receptor phosphorylation in the resistance to tamoxifen. Int J Breast Cancer (2011) 2011:232435. doi: 10.4061/2011/232435
13. Jiang J, Sarwar N, Peston D, Kulinskaya E, Shousha S, Coombes RC, et al. Phosphorylation of estrogen receptor-alpha at Ser167 is indicative of longer disease-free and overall survival in breast cancer patients. Clin Cancer Res (2007) 13(19):5769–76. doi: 10.1158/1078-0432.CCR-07-0822
14. Kok M, Holm-Wigerup C, Hauptmann M, Michalides R, Stal O, Linn S, et al. Estrogen receptor-alpha phosphorylation at serine-118 and tamoxifen response in breast cancer. J Natl Cancer Inst (2009) 101(24):1725–9. doi: 10.1093/jnci/djp412
15. Murphy LC, Niu Y, Snell L, Watson P. Phospho-serine-118 estrogen receptor-alpha expression is associated with better disease outcome in women treated with tamoxifen. Clin Cancer Res (2004) 10(17):5902–6. doi: 10.1158/1078-0432.CCR-04-0191
16. Yamashita H, Nishio M, Toyama T, Sugiura H, Kondo N, Kobayashi S, et al. Low phosphorylation of estrogen receptor alpha (ERalpha) serine 118 and high phosphorylation of ERalpha serine 167 improve survival in ER-positive breast cancer. Endocr Relat Cancer (2008) 15(3):755–63. doi: 10.1677/ERC-08-0078
17. Yamashita H, Nishio M, Kobayashi S, Ando Y, Sugiura H, Zhang Z, et al. Phosphorylation of estrogen receptor alpha serine 167 is predictive of response to endocrine therapy and increases postrelapse survival in metastatic breast cancer. Breast Cancer Res (2005) 7(5):R753–764. doi: 10.1186/bcr1285
18. Jeselsohn R, Yelensky R, Buchwalter G, Frampton G, Meric-Bernstam F, Gonzalez-Angulo AM, et al. Emergence of constitutively active estrogen receptor-alpha mutations in pretreated advanced estrogen receptor-positive breast cancer. Clin Cancer Res (2014) 20(7):1757–67. doi: 10.1158/1078-0432.CCR-13-2332
19. Merenbakh-Lamin K, Ben-Baruch N, Yeheskel A, Dvir A, Soussan-Gutman L, Jeselsohn R, et al. D538G mutation in estrogen receptor-alpha: A novel mechanism for acquired endocrine resistance in breast cancer. Cancer Res (2013) 73(23):6856–64. doi: 10.1158/0008-5472.CAN-13-1197
20. Robinson DR, Wu YM, Vats P, Su F, Lonigro RJ, Cao X, et al. Activating ESR1 mutations in hormone-resistant metastatic breast cancer. Nat Genet (2013) 45(12):1446–51. doi: 10.1038/ng.2823
21. Toy W, Shen Y, Won H, Green B, Sakr RA, Will M, et al. ESR1 ligand-binding domain mutations in hormone-resistant breast cancer. Nat Genet (2013) 45(12):1439–45. doi: 10.1038/ng.2822
22. Najim O, Seghers S, Sergoynne L, Van Gaver H, Papadimitriou K, Wouters K, et al. The association between type of endocrine therapy and development of estrogen receptor-1 mutation(s) in patients with hormone-sensitive advanced breast cancer: A systematic review and meta-analysis of randomized and non-randomized trials. Biochim Biophys Acta Rev Cancer (2019) 1872(2):188315. doi: 10.1016/j.bbcan.2019.188315
23. Clarke R, Tyson JJ, Dixon JM. Endocrine resistance in breast cancer–an overview and update. Mol Cell Endocrinol (2015) 418 Pt 3:220–34. doi: 10.1016/j.mce.2015.09.035
24. Segal CV, Dowsett M. Estrogen receptor mutations in breast cancer–new focus on an old target. Clin Cancer Res (2014) 20(7):1724–6. doi: 10.1158/1078-0432.CCR-14-0067
25. Inwald EC, Klinkhammer-Schalke M, Hofstadter F, Zeman F, Koller M, Gerstenhauer M, et al. Ki-67 is a prognostic parameter in breast cancer patients: results of a large population-based cohort of a cancer registry. Breast Cancer Res Treat (2013) 139(2):539–52. doi: 10.1007/s10549-013-2560-8
26. Urruticoechea A, Smith IE, Dowsett M. Proliferation marker ki-67 in early breast cancer. J Clin Oncol (2005) 23(28):7212–20. doi: 10.1200/JCO.2005.07.501
27. Bottini A, Berruti A, Bersiga A, Brizzi MP, Bruzzi P, Aguggini S, et al. Relationship between tumour shrinkage and reduction in Ki67 expression after primary chemotherapy in human breast cancer. Br J Cancer (2001) 85(8):1106–12. doi: 10.1054/bjoc.2001.2048
28. Spring LM, Gupta A, Reynolds KL, Gadd MA, Ellisen LW, Isakoff SJ, et al. Neoadjuvant endocrine therapy for estrogen receptor-positive breast cancer: A systematic review and meta-analysis. JAMA Oncol (2016) 2(11):1477–86. doi: 10.1001/jamaoncol.2016.1897
29. Tan MC, Al Mushawah F, Gao F, Aft RL, Gillanders WE, Eberlein TJ, et al. Predictors of complete pathological response after neoadjuvant systemic therapy for breast cancer. Am J Surg (2009) 198(4):520–5. doi: 10.1016/j.amjsurg.2009.06.004
30. Early Breast Cancer Trialists’ Collab Group. Systemic treatment of early breast cancer by hormonal, cytotoxic, or immune therapy. 133 randomised trials involving 31,000 recurrences and 24,000 deaths among 75,000 women. Lancet (1992) 339(8784):1–15. doi: 10.1016/0140-6736(92)90139-T
31. Larionova I, Tuguzbaeva G, Ponomaryova A, Stakheyeva M, Cherdyntseva N, Pavlov V, et al. Tumor-associated macrophages in human breast, colorectal, lung, ovarian and prostate cancers. Front Oncol (2020) 10:566511. doi: 10.3389/fonc.2020.566511
32. Xiao M, He J, Yin L, Chen X, Zu X, Shen Y. Tumor-associated macrophages: Critical players in drug resistance of breast cancer. Front Immunol (2021) 12:799428. doi: 10.3389/fimmu.2021.799428
33. Maley CC, Aktipis A, Graham TA, Sottoriva A, Boddy AM, Janiszewska M, et al. Classifying the evolutionary and ecological features of neoplasms. Nat Rev Cancer (2017) 17(10):605–19. doi: 10.1038/nrc.2017.69
34. Ganesh K, Massague J. Targeting metastatic cancer. Nat Med (2021) 27(1):34–44. doi: 10.1038/s41591-020-01195-4
35. Massague J, Ganesh K. Metastasis-initiating cells and ecosystems. Cancer Discovery (2021) 11(4):971–94. doi: 10.1158/2159-8290.CD-21-0010
36. Tan K, Naylor MJ. Tumour microenvironment-immune cell interactions influencing breast cancer heterogeneity and disease progression. Front Oncol (2022) 12:876451. doi: 10.3389/fonc.2022.876451
37. Thol K, Pawlik P, McGranahan N. Therapy sculpts the complex interplay between cancer and the immune system during tumour evolution. Genome Med (2022) 14(1):137. doi: 10.1186/s13073-022-01138-3
38. Mehraj U, Dar AH, Wani NA, Mir MA. Tumor microenvironment promotes breast cancer chemoresistance. Cancer Chemother Pharmacol (2021) 87(2):147–58. doi: 10.1007/s00280-020-04222-w
39. Mehraj U, Ganai RA, Macha MA, Hamid A, Zargar MA, Bhat AA, et al. The tumor microenvironment as driver of stemness and therapeutic resistance in breast cancer: New challenges and therapeutic opportunities. Cell Oncol (Dordr) (2021) 44(6):1209–29. doi: 10.1007/s13402-021-00634-9
40. Wilson BE, Gorrini C, Cescon DW. Breast cancer immune microenvironment: from pre-clinical models to clinical therapies. Breast Cancer Res Treat (2022) 191(2):257–67. doi: 10.1007/s10549-021-06431-0
41. Zeng X, Liu C, Yao J, Wan H, Wan G, Li Y, et al. Breast cancer stem cells, heterogeneity, targeting therapies and therapeutic implications. Pharmacol Res (2021) 163:105320. doi: 10.1016/j.phrs.2020.105320
42. Eke I, Cordes N. Focal adhesion signaling and therapy resistance in cancer. Semin Cancer Biol (2015) 31:65–75. doi: 10.1016/j.semcancer.2014.07.009
43. Kesh K, Gupta VK, Durden B, Garrido V, Mateo-Victoriano B, Lavania SP, et al. Therapy resistance, cancer stem cells and ECM in cancer: The matrix reloaded. Cancers (Basel) (2020) 12(10): 3067. doi: 10.3390/cancers12103067
44. Klemm F, Joyce JA. Microenvironmental regulation of therapeutic response in cancer. Trends Cell Biol (2015) 25(4):198–213. doi: 10.1016/j.tcb.2014.11.006
45. Leask A. A centralized communication network: Recent insights into the role of the cancer associated fibroblast in the development of drug resistance in tumors. Semin Cell Dev Biol (2020) 101:111–4. doi: 10.1016/j.semcdb.2019.10.016
46. M JR, V. S. BMI1 and PTEN are key determinants of breast cancer therapy: A plausible therapeutic target in breast cancer. Gene (2018) 678:302–11. doi: 10.1016/j.gene.2018.08.022
47. Aboussekhra A. Role of cancer-associated fibroblasts in breast cancer development and prognosis. Int J Dev Biol (2011) 55(7-9):841–9. doi: 10.1387/ijdb.113362aa
48. Jung YY, Lee YK, Koo JS. Expression of cancer-associated fibroblast-related proteins in adipose stroma of breast cancer. Tumour Biol (2015) 36(11):8685–95. doi: 10.1007/s13277-015-3594-9
49. Pelon F, Bourachot B, Kieffer Y, Magagna I, Mermet-Meillon F, Bonnet I, et al. Cancer-associated fibroblast heterogeneity in axillary lymph nodes drives metastases in breast cancer through complementary mechanisms. Nat Commun (2020) 11(1):404. doi: 10.1038/s41467-019-14134-w
50. Yang SS, Ma S, Dou H, Liu F, Zhang SY, Jiang C, et al. Breast cancer-derived exosomes regulate cell invasion and metastasis in breast cancer via miR-146a to activate cancer associated fibroblasts in tumor microenvironment. Exp Cell Res (2020) 391(2):111983. doi: 10.1016/j.yexcr.2020.111983
51. Brechbuhl HM, Finlay-Schultz J, Yamamoto TM, Gillen AE, Cittelly DM, Tan AC, et al. Fibroblast subtypes regulate responsiveness of luminal breast cancer to estrogen. Clin Cancer Res (2017) 23(7):1710–21. doi: 10.1158/1078-0432.CCR-15-2851
52. Simpkins SA, Hanby AM, Holliday DL, Speirs V. Clinical and functional significance of loss of caveolin-1 expression in breast cancer-associated fibroblasts. J Pathol (2012) 227(4):490–8. doi: 10.1002/path.4034
53. Su S, Chen J, Yao H, Liu J, Yu S, Lao L, et al. CD10(+)GPR77(+) cancer-associated fibroblasts promote cancer formation and chemoresistance by sustaining cancer stemness. Cell (2018) 172(4):841–856 e816. doi: 10.1016/j.cell.2018.01.009
54. Wu SZ, Roden DL, Wang C, Holliday H, Harvey K, Cazet AS, et al. Stromal cell diversity associated with immune evasion in human triple-negative breast cancer. EMBO J (2020) 39(19):e104063. doi: 10.15252/embj.2019104063
55. Sebastian A, Hum NR, Martin KA, Gilmore SF, Peran I, Byers SW, et al. Single-cell transcriptomic analysis of tumor-derived fibroblasts and normal tissue-resident fibroblasts reveals fibroblast heterogeneity in breast cancer. Cancers (Basel) (2020) 12(5): 1307. doi: 10.3390/cancers12051307
56. Bartoschek M, Oskolkov N, Bocci M, Lovrot J, Larsson C, Sommarin M, et al. Spatially and functionally distinct subclasses of breast cancer-associated fibroblasts revealed by single cell RNA sequencing. Nat Commun (2018) 9(1):5150. doi: 10.1038/s41467-018-07582-3
57. Han C, Liu T, Yin R. Biomarkers for cancer-associated fibroblasts. biomark Res (2020) 8(1):64. doi: 10.1186/s40364-020-00245-w
58. Weigel MT, Banerjee S, Arnedos M, Salter J, A'Hern R, Dowsett M, et al. Enhanced expression of the PDGFR/Abl signaling pathway in aromatase inhibitor-resistant breast cancer. Ann Oncol (2013) 24(1):126–33. doi: 10.1093/annonc/mds240
59. Liu L, Liu S, Luo H, Chen C, Zhang X, He L, et al. GPR30-mediated HMGB1 upregulation in CAFs induces autophagy and tamoxifen resistance in ERalpha-positive breast cancer cells. Aging (Albany NY) (2021) 13(12):16178–97. doi: 10.18632/aging.203145
60. Gao Y, Li X, Zeng C, Liu C, Hao Q, Li W, et al. CD63(+) cancer-associated fibroblasts confer tamoxifen resistance to breast cancer cells through exosomal miR-22. Adv Sci (Weinh) (2020) 7(21):2002518. doi: 10.1002/advs.202002518
61. Turczyk L, Kitowska K, Mieszkowska M, Mieczkowski K, Czaplinska D, Piasecka D, et al. FGFR2-driven signaling counteracts tamoxifen effect on ERalpha-positive breast cancer cells. Neoplasia (2017) 19(10):791–804. doi: 10.1016/j.neo.2017.07.006
62. Leyh B, Dittmer A, Lange T, Martens JW, Dittmer J. Stromal cells promote anti-estrogen resistance of breast cancer cells through an insulin-like growth factor binding protein 5 (IGFBP5)/B-cell leukemia/lymphoma 3 (Bcl-3) axis. Oncotarget (2015) 6(36):39307–28. doi: 10.18632/oncotarget.5624
63. Pontiggia O, Sampayo R, Raffo D, Motter A, Xu R, Bissell MJ, et al. The tumor microenvironment modulates tamoxifen resistance in breast cancer: a role for soluble stromal factors and fibronectin through beta1 integrin. Breast Cancer Res Treat (2012) 133(2):459–71. doi: 10.1007/s10549-011-1766-x
64. Engelman JA. Targeting PI3K signalling in cancer: opportunities, challenges and limitations. Nat Rev Cancer (2009) 9(8):550–62. doi: 10.1038/nrc2664
65. Kang S, Bader AG, Vogt PK. Phosphatidylinositol 3-kinase mutations identified in human cancer are oncogenic. Proc Natl Acad Sci U S A (2005) 102(3):802–7. doi: 10.1073/pnas.0408864102
66. Samuels Y, Wang Z, Bardelli A, Silliman N, Ptak J, Szabo S, et al. High frequency of mutations of the PIK3CA gene in human cancers. Science (2004) 304(5670):554. doi: 10.1126/science.1096502
67. Li H, Prever L, Hirsch E, Gulluni F. Targeting PI3K/AKT/mTOR signaling pathway in breast cancer. Cancers (Basel) (2021) 13(14): 3517. doi: 10.3390/cancers13143517
68. Sotgia F, Del Galdo F, Casimiro MC, Bonuccelli G, Mercier I, Whitaker-Menezes D, et al. Caveolin-1-/- null mammary stromal fibroblasts share characteristics with human breast cancer-associated fibroblasts. Am J Pathol (2009) 174(3):746–61. doi: 10.2353/ajpath.2009.080658
69. Martinez-Outschoorn UE, Balliet RM, Rivadeneira DB, Chiavarina B, Pavlides S, Wang C, et al. Oxidative stress in cancer associated fibroblasts drives tumor-stroma co-evolution: A new paradigm for understanding tumor metabolism, the field effect and genomic instability in cancer cells. Cell Cycle (2010) 9(16):3256–76. doi: 10.4161/cc.9.16.12553
70. Martinez-Outschoorn UE, Trimmer C, Lin Z, Whitaker-Menezes D, Chiavarina B, Zhou J, et al. Autophagy in cancer associated fibroblasts promotes tumor cell survival: Role of hypoxia, HIF1 induction and NFkappaB activation in the tumor stromal microenvironment. Cell Cycle (2010) 9(17):3515–33. doi: 10.4161/cc.9.17.12928
71. Sansone P, Berishaj M, Rajasekhar VK, Ceccarelli C, Chang Q, Strillacci A, et al. Evolution of cancer stem-like cells in endocrine-resistant metastatic breast cancers is mediated by stromal microvesicles. Cancer Res (2017) 77(8):1927–41. doi: 10.1158/0008-5472.CAN-16-2129
72. Noy R, Pollard JW. Tumor-associated macrophages: from mechanisms to therapy. Immunity (2014) 41(1):49–61. doi: 10.1016/j.immuni.2014.06.010
73. Stout RD, Jiang C, Matta B, Tietzel I, Watkins SK, Suttles J. Macrophages sequentially change their functional phenotype in response to changes in microenvironmental influences. J Immunol (2005) 175(1):342–9. doi: 10.4049/jimmunol.175.1.342
74. Yang C, He L, He P, Liu Y, Wang W, He Y, et al. Increased drug resistance in breast cancer by tumor-associated macrophages through IL-10/STAT3/bcl-2 signaling pathway. Med Oncol (2015) 32(2):352. doi: 10.1007/s12032-014-0352-6
75. Castellaro AM, Rodriguez-Baili MC, Di Tada CE, Gil GA. Tumor-associated macrophages induce endocrine therapy resistance in ER+ breast cancer cells. Cancers (Basel) (2019) 11(2): 189. doi: 10.3390/cancers11020189
76. Koren S, Bentires-Alj M. Breast tumor heterogeneity: Source of fitness, hurdle for therapy. Mol Cell (2015) 60(4):537–46. doi: 10.1016/j.molcel.2015.10.031
77. Butti R, Gunasekaran VP, Kumar TVS, Banerjee P, Kundu GC. Breast cancer stem cells: Biology and therapeutic implications. Int J Biochem Cell Biol (2019) 107:38–52. doi: 10.1016/j.biocel.2018.12.001
78. Liu Y, Zhang X, Liu J, Hou G, Zhang S, Zhang J. Everolimus in combination with letrozole inhibit human breast cancer MCF-7/Aro stem cells via PI3K/mTOR pathway: an experimental study. Tumour Biol (2014) 35(2):1275–86. doi: 10.1007/s13277-013-1170-8
79. Brooks MD, Burness ML, Wicha MS. Therapeutic implications of cellular heterogeneity and plasticity in breast cancer. Cell Stem Cell (2015) 17(3):260–71. doi: 10.1016/j.stem.2015.08.014
80. Simoes BM, Santiago-Gomez A, Chiodo C, Moreira T, Conole D, Lovell S, et al. Targeting STAT3 signaling using stabilised sulforaphane (SFX-01) inhibits endocrine resistant stem-like cells in ER-positive breast cancer. Oncogene (2020) 39(25):4896–908. doi: 10.1038/s41388-020-1335-z
81. Wang Z, Oron E, Nelson B, Razis S, Ivanova N. Distinct lineage specification roles for NANOG, OCT4, and SOX2 in human embryonic stem cells. Cell Stem Cell (2012) 10(4):440–54. doi: 10.1016/j.stem.2012.02.016
82. Jang GB, Kim JY, Cho SD, Park KS, Jung JY, Lee HY, et al. Blockade of wnt/beta-catenin signaling suppresses breast cancer metastasis by inhibiting CSC-like phenotype. Sci Rep (2015) 5:12465. doi: 10.1038/srep12465
83. Leung EY, Askarian-Amiri ME, Sarkar D, Ferraro-Peyret C, Joseph WR, Finlay GJ, et al. Endocrine therapy of estrogen receptor-positive breast cancer cells: Early differential effects on stem cell markers. Front Oncol (2017) 7:184. doi: 10.3389/fonc.2017.00184
84. Acar A, Simoes BM, Clarke RB, Brennan K. A role for notch signalling in breast cancer and endocrine resistance. Stem Cells Int (2016) 2016:2498764. doi: 10.1155/2016/2498764
85. Harrison H, Simoes BM, Rogerson L, Howell SJ, Landberg G, Clarke RB. Oestrogen increases the activity of oestrogen receptor negative breast cancer stem cells through paracrine EGFR and notch signalling. Breast Cancer Res (2013) 15(2):R21. doi: 10.1186/bcr3396
86. Creighton CJ, Li X, Landis M, Dixon JM, Neumeister VM, Sjolund A, et al. Residual breast cancers after conventional therapy display mesenchymal as well as tumor-initiating features. Proc Natl Acad Sci U S A (2009) 106(33):13820–5. doi: 10.1073/pnas.0905718106
87. Lombardo Y, Faronato M, Filipovic A, Vircillo V, Magnani L, Coombes RC. Nicastrin and Notch4 drive endocrine therapy resistance and epithelial to mesenchymal transition in MCF7 breast cancer cells. Breast Cancer Res (2014) 16(3):R62. doi: 10.1186/bcr3675
88. Simoes BM, O'Brien CS, Eyre R, Silva A, Yu L, Sarmiento-Castro A, et al. Anti-estrogen resistance in human breast tumors is driven by JAG1-NOTCH4-Dependent cancer stem cell activity. Cell Rep (2015) 12(12):1968–77. doi: 10.1016/j.celrep.2015.08.050
89. McClements L, Annett S, Yakkundi A, O'Rourke M, Valentine A, Moustafa N, et al. FKBPL and its peptide derivatives inhibit endocrine therapy resistant cancer stem cells and breast cancer metastasis by downregulating DLL4 and Notch4. BMC Cancer (2019) 19(1):351. doi: 10.1186/s12885-019-5500-0
90. Davis BW, Zava DT, Locher GW, Goldhirsch A, Hartmann WH. Receptor heterogeneity of human breast cancer as measured by multiple intratumoral assays of estrogen and progesterone receptor. Eur J Cancer Clin Oncol (1984) 20(3):375–82. doi: 10.1016/0277-5379(84)90084-1
91. Nassar A, Radhakrishnan A, Cabrero IA, Cotsonis GA, Cohen C. Intratumoral heterogeneity of immunohistochemical marker expression in breast carcinoma: a tissue microarray-based study. Appl Immunohistochem Mol Morphol (2010) 18(5):433–41. doi: 10.1097/PAI.0b013e3181dddb20
92. Pertschuk LP, Axiotis CA, Feldman JG, Kim YD, Karavattayhayyil SJ, Braithwaite L. Marked intratumoral heterogeneity of the proto-oncogene her-2/neu determined by three different detection systems. Breast J (1999) 5(6):369–74. doi: 10.1046/j.1524-4741.1999.97088.x
93. Banerji S, Cibulskis K, Rangel-Escareno C, Brown KK, Carter SL, Frederick AM, et al. Sequence analysis of mutations and translocations across breast cancer subtypes. Nature (2012) 486(7403):405–9. doi: 10.1038/nature11154
94. Ciriello G, Gatza ML, Beck AH, Wilkerson MD, Rhie SK, Pastore A, et al. Comprehensive molecular portraits of invasive lobular breast cancer. Cell (2015) 163(2):506–19. doi: 10.1016/j.cell.2015.09.033
95. Navin N, Krasnitz A, Rodgers L, Cook K, Meth J, Kendall J, et al. Inferring tumor progression from genomic heterogeneity. Genome Res (2010) 20(1):68–80. doi: 10.1101/gr.099622.109
96. Nik-Zainal S, Alexandrov LB, Wedge DC, Van Loo P, Greenman CD, Raine K, et al. Mutational processes molding the genomes of 21 breast cancers. Cell (2012) 149(5):979–93. doi: 10.1016/j.cell.2012.04.024
97. Nik-Zainal S, Van Loo P, Wedge DC, Alexandrov LB, Greenman CD, Lau KW, et al. The life history of 21 breast cancers. Cell (2012) 149(5):994–1007. doi: 10.1016/j.cell.2012.04.023
98. Stephens PJ, Tarpey PS, Davies H, Van Loo P, Greenman C, Wedge DC, et al. The landscape of cancer genes and mutational processes in breast cancer. Nature (2012) 486(7403):400–4. doi: 10.1038/nature11017
99. Miller CA, Gindin Y, Lu C, Griffith OL, Griffith M, Shen D, et al. Aromatase inhibition remodels the clonal architecture of estrogen-receptor-positive breast cancers. Nat Commun (2016) 7:12498. doi: 10.1038/ncomms12498
100. Yates LR, Gerstung M, Knappskog S, Desmedt C, Gundem G, Van Loo P, et al. Subclonal diversification of primary breast cancer revealed by multiregion sequencing. Nat Med (2015) 21(7):751–9. doi: 10.1038/nm.3886
101. Lips EH, Kumar T, Megalios A, Visser LL, Sheinman M, Fortunato A, et al. Genomic analysis defines clonal relationships of ductal carcinoma in situ and recurrent invasive breast cancer. Nat Genet (2022) 54(6):850–60. doi: 10.1038/s41588-022-01082-3
102. Kostecka A, Nowikiewicz T, Olszewski P, Koczkowska M, Horbacz M, Heinzl M, et al. High prevalence of somatic PIK3CA and TP53 pathogenic variants in the normal mammary gland tissue of sporadic breast cancer patients revealed by duplex sequencing. NPJ Breast Cancer (2022) 8(1):76. doi: 10.1038/s41523-022-00443-9
103. Greenman C, Stephens P, Smith R, Dalgliesh GL, Hunter C, Bignell G, et al. Patterns of somatic mutation in human cancer genomes. Nature (2007) 446(7132):153–8. doi: 10.1038/nature05610
104. Sjoblom T, Jones S, Wood LD, Parsons DW, Lin J, Barber TD, et al. The consensus coding sequences of human breast and colorectal cancers. Science (2006) 314(5797):268–74. doi: 10.1126/science.1133427
105. Curtis C, Shah SP, Chin SF, Turashvili G, Rueda OM, Dunning MJ, et al. The genomic and transcriptomic architecture of 2,000 breast tumours reveals novel subgroups. Nature (2012) 486(7403):346–52. doi: 10.1038/nature10983
106. Fiegl M, Tueni C, Schenk T, Jakesz R, Gnant M, Reiner A, et al. Interphase cytogenetics reveals a high incidence of aneuploidy and intra-tumour heterogeneity in breast cancer. Br J Cancer (1995) 72(1):51–5. doi: 10.1038/bjc.1995.276
107. Beca F, Polyak K. Intratumor heterogeneity in breast cancer. Adv Exp Med Biol (2016) 882:169–89. doi: 10.1007/978-3-319-22909-6_7
108. Marusyk A, Janiszewska M, Polyak K. Intratumor heterogeneity: The Rosetta stone of therapy resistance. Cancer Cell (2020) 37(4):471–84. doi: 10.1016/j.ccell.2020.03.007
109. Nik-Zainal S, Davies H, Staaf J, Ramakrishna M, Glodzik D, Zou X, et al. Landscape of somatic mutations in 560 breast cancer whole-genome sequences. Nature (2016) 534(7605):47–54. doi: 10.1038/nature17676
110. Fumagalli C, Ranghiero A, Gandini S, Corso F, Taormina S, De Camilli E, et al. Inter-tumor genomic heterogeneity of breast cancers: comprehensive genomic profile of primary early breast cancers and relapses. Breast Cancer Res (2020) 22(1):107. doi: 10.1186/s13058-020-01345-z
111. Yates LR, Knappskog S, Wedge D, Farmery JHR, Gonzalez S, Martincorena I, et al. Genomic evolution of breast cancer metastasis and relapse. Cancer Cell (2017) 32(2):169–184 e167. doi: 10.1016/j.ccell.2017.07.005
112. Jeselsohn R, Buchwalter G, De Angelis C, Brown M, Schiff R. ESR1 mutations-a mechanism for acquired endocrine resistance in breast cancer. Nat Rev Clin Oncol (2015) 12(10):573–83. doi: 10.1038/nrclinonc.2015.117
113. Belachew EB, Sewasew DT. Molecular mechanisms of endocrine resistance in estrogen-positive breast cancer. Front Endocrinol (Lausanne) (2021) 12:599586. doi: 10.3389/fendo.2021.599586
114. Bertucci F, Ng CKY, Patsouris A, Droin N, Piscuoglio S, Carbuccia N, et al. Genomic characterization of metastatic breast cancers. Nature (2019) 569(7757):560–4. doi: 10.1038/s41586-019-1056-z
115. Brown D, Smeets D, Szekely B, Larsimont D, Szasz AM, Adnet PY, et al. Phylogenetic analysis of metastatic progression in breast cancer using somatic mutations and copy number aberrations. Nat Commun (2017) 8:14944. doi: 10.1038/ncomms14944
116. Guerini-Rocco E, Gray KP, Fumagalli C, Reforgiato MR, Leone I, Rafaniello Raviele P, et al. Genomic aberrations and late recurrence in postmenopausal women with hormone receptor-positive early breast cancer: Results from the SOLE trial. Clin Cancer Res (2021) 27(2):504–12. doi: 10.1158/1078-0432.CCR-20-0126
117. Razavi P, Chang MT, Xu G, Bandlamudi C, Ross DS, Vasan N, et al. The genomic landscape of endocrine-resistant advanced breast cancers. Cancer Cell (2018) 34(3):427–438 e426. doi: 10.1016/j.ccell.2018.08.008
118. Gambardella G, Viscido G, Tumaini B, Isacchi A, Bosotti R, di Bernardo D. A single-cell analysis of breast cancer cell lines to study tumour heterogeneity and drug response. Nat Commun (2022) 13(1):1714. doi: 10.1038/s41467-022-29358-6
119. Sharma SV, Lee DY, Li B, Quinlan MP, Takahashi F, Maheswaran S, et al. A chromatin-mediated reversible drug-tolerant state in cancer cell subpopulations. Cell (2010) 141(1):69–80. doi: 10.1016/j.cell.2010.02.027
120. Kolodziejczyk AA, Kim JK, Svensson V, Marioni JC, Teichmann SA. The technology and biology of single-cell RNA sequencing. Mol Cell (2015) 58(4):610–20. doi: 10.1016/j.molcel.2015.04.005
121. Chung W, Eum HH, Lee HO, Lee KM, Lee HB, Kim KT, et al. Single-cell RNA-seq enables comprehensive tumour and immune cell profiling in primary breast cancer. Nat Commun (2017) 8:15081. doi: 10.1038/ncomms15081
122. Chen F, Ding K, Priedigkeit N, Elangovan A, Levine KM, Carleton N, et al. Single-cell transcriptomic heterogeneity in invasive ductal and lobular breast cancer cells. Cancer Res (2021) 81(2):268–81. doi: 10.1158/0008-5472.CAN-20-0696
123. Wu SZ, Al-Eryani G, Roden DL, Junankar S, Harvey K, Andersson A, et al. A single-cell and spatially resolved atlas of human breast cancers. Nat Genet (2021) 53(9):1334–47. doi: 10.1038/s41588-021-00911-1
124. Karaayvaz M, Cristea S, Gillespie SM, Patel AP, Mylvaganam R, Luo CC, et al. Unravelling subclonal heterogeneity and aggressive disease states in TNBC through single-cell RNA-seq. Nat Commun (2018) 9(1):3588. doi: 10.1038/s41467-018-06052-0
125. Dongre A, Rashidian M, Eaton EN, Reinhardt F, Thiru P, Zagorulya M, et al. Direct and indirect regulators of epithelial-mesenchymal transition-mediated immunosuppression in breast carcinomas. Cancer Discovery (2021) 11(5):1286–305. doi: 10.1158/2159-8290.CD-20-0603
126. Echeverria GV, Powell E, Seth S, Ge Z, Carugo A, Bristow C, et al. High-resolution clonal mapping of multi-organ metastasis in triple negative breast cancer. Nat Commun (2018) 9(1):5079. doi: 10.1038/s41467-018-07406-4
127. Davis RT, Blake K, Ma D, Gabra MBI, Hernandez GA, Phung AT, et al. Transcriptional diversity and bioenergetic shift in human breast cancer metastasis revealed by single-cell RNA sequencing. Nat Cell Biol (2020) 22(3):310–20. doi: 10.1038/s41556-020-0477-0
128. Nguyen A, Yoshida M, Goodarzi H, Tavazoie SF. Highly variable cancer subpopulations that exhibit enhanced transcriptome variability and metastatic fitness. Nat Commun (2016) 7:11246. doi: 10.1038/ncomms11246
129. Hong SP, Chan TE, Lombardo Y, Corleone G, Rotmensz N, Bravaccini S, et al. Single-cell transcriptomics reveals multi-step adaptations to endocrine therapy. Nat Commun (2019) 10(1):3840. doi: 10.1038/s41467-019-11721-9
130. Griffiths JI, Chen J, Cosgrove PA, O'Dea A, Sharma P, Ma C, et al. Serial single-cell genomics reveals convergent subclonal evolution of resistance as early-stage breast cancer patients progress on endocrine plus CDK4/6 therapy. Nat Cancer (2021) 2(6):658–71. doi: 10.1038/s43018-021-00215-7
131. Boroughs LK, DeBerardinis RJ. Metabolic pathways promoting cancer cell survival and growth. Nat Cell Biol (2015) 17(4):351–9. doi: 10.1038/ncb3124
132. McGuirk S, Audet-Delage Y, St-Pierre J. Metabolic fitness and plasticity in cancer progression. Trends Cancer (2020) 6(1):49–61. doi: 10.1016/j.trecan.2019.11.009
133. Blundon MA, Dasgupta S. Metabolic dysregulation controls endocrine therapy-resistant cancer recurrence and metastasis. Endocrinology (2019) 160(8):1811–20. doi: 10.1210/en.2019-00097
134. Chen PH, Cai L, Huffman K, Yang C, Kim J, Faubert B, et al. Metabolic diversity in human non-small cell lung cancer cells. Mol Cell (2019) 76(5):838–851 e835. doi: 10.1016/j.molcel.2019.08.028
135. Kim J, DeBerardinis RJ. Mechanisms and implications of metabolic heterogeneity in cancer. Cell Metab (2019) 30(3):434–46. doi: 10.1016/j.cmet.2019.08.013
136. Courtney KD, Bezwada D, Mashimo T, Pichumani K, Vemireddy V, Funk AM, et al. Isotope tracing of human clear cell renal cell carcinomas demonstrates suppressed glucose oxidation In vivo. cell metab. 28(5) (2018) 793-800:e792. doi: 10.1016/j.cmet.2018.07.020
137. Hensley CT, Faubert B, Yuan Q, Lev-Cohain N, Jin E, Kim J, et al. Metabolic heterogeneity in human lung tumors. Cell (2016) 164(4):681–94. doi: 10.1016/j.cell.2015.12.034
138. Maher EA, Marin-Valencia I, Bachoo RM, Mashimo T, Raisanen J, Hatanpaa KJ, et al. Metabolism of [U-13 c]glucose in human brain tumors in vivo. NMR BioMed (2012) 25(11):1234–44. doi: 10.1002/nbm.2794
139. Kung HN, Marks JR, Chi JT. Glutamine synthetase is a genetic determinant of cell type-specific glutamine independence in breast epithelia. PloS Genet (2011) 7(8):e1002229. doi: 10.1371/journal.pgen.1002229
140. Demas DM, Demo S, Fallah Y, Clarke R, Nephew KP, Althouse S, et al. Glutamine metabolism drives growth in advanced hormone receptor positive breast cancer. Front Oncol (2019) 9:686. doi: 10.3389/fonc.2019.00686
141. Hamadneh L, Abuarqoub R, Alhusban A, Bahader M. Upregulation of PI3K/AKT/PTEN pathway is correlated with glucose and glutamine metabolic dysfunction during tamoxifen resistance development in MCF-7 cells. Sci Rep (2020) 10(1):21933. doi: 10.1038/s41598-020-78833-x
142. Gordan JD, Thompson CB, Simon MC. HIF and c-myc: sibling rivals for control of cancer cell metabolism and proliferation. Cancer Cell (2007) 12(2):108–13. doi: 10.1016/j.ccr.2007.07.006
143. Sengupta S, Biarnes MC, Jordan VC. Cyclin dependent kinase-9 mediated transcriptional de-regulation of cMYC as a critical determinant of endocrine-therapy resistance in breast cancers. Breast Cancer Res Treat (2014) 143(1):113–24. doi: 10.1007/s10549-013-2789-2
144. Shajahan-Haq AN, Cook KL, Schwartz-Roberts JL, Eltayeb AE, Demas DM, Warri AM, et al. MYC regulates the unfolded protein response and glucose and glutamine uptake in endocrine resistant breast cancer. Mol Cancer (2014) 13:239. doi: 10.1186/1476-4598-13-239
145. Jogi A, Ehinger A, Hartman L, Alkner S. Expression of HIF-1alpha is related to a poor prognosis and tamoxifen resistance in contralateral breast cancer. PloS One (2019) 14(12):e0226150. doi: 10.1371/journal.pone.0226150
146. Jones BC, Pohlmann PR, Clarke R, Sengupta S. Treatment against glucose-dependent cancers through metabolic PFKFB3 targeting of glycolytic flux. Cancer Metastasis Rev (2022) 41(2):447–58. doi: 10.1007/s10555-022-10027-5
147. Kimbro KS, Simons JW. Hypoxia-inducible factor-1 in human breast and prostate cancer. Endocr Relat Cancer (2006) 13(3):739–49. doi: 10.1677/erc.1.00728
148. Woo YM, Shin Y, Lee EJ, Lee S, Jeong SH, Kong HK, et al. Inhibition of aerobic glycolysis represses Akt/mTOR/HIF-1alpha axis and restores tamoxifen sensitivity in antiestrogen-resistant breast cancer cells. PloS One (2015) 10(7):e0132285. doi: 10.1371/journal.pone.0132285
149. Dowsett M, Smith IE, Ebbs SR, Dixon JM, Skene A, Griffith C, et al. Short-term changes in ki-67 during neoadjuvant treatment of primary breast cancer with anastrozole or tamoxifen alone or combined correlate with recurrence-free survival. Clin Cancer Res (2005) 11(2 Pt 2):951s–8s. doi: 10.1158/1078-0432.951s.11.2
150. Zhang A, Wang X, Fan C, Mao X. The role of Ki67 in evaluating neoadjuvant endocrine therapy of hormone receptor-positive breast cancer. Front Endocrinol (Lausanne) (2021) 12:687244. doi: 10.3389/fendo.2021.687244
151. Smith I, Robertson J, Kilburn L, Wilcox M, Evans A, Holcombe C, et al. Long-term outcome and prognostic value of Ki67 after perioperative endocrine therapy in postmenopausal women with hormone-sensitive early breast cancer (POETIC): an open-label, multicentre, parallel-group, randomised, phase 3 trial. Lancet Oncol (2020) 21(11):1443–54. doi: 10.1016/S1470-2045(20)30458-7
152. Rugo HS, Rumble RB, Macrae E, Barton DL, Connolly HK, Dickler MN, et al. Endocrine therapy for hormone receptor-positive metastatic breast cancer: American society of clinical oncology guideline. J Clin Oncol (2016) 34(25):3069–103. doi: 10.1200/JCO.2016.67.1487
153. Dowling RJO, Sparano JA, Goodwin PJ, Bidard FC, Cescon DW, Chandarlapaty S, et al. Toronto Workshop on late recurrence in estrogen receptor-positive breast cancer: Part 2: Approaches to predict and identify late recurrence, research directions. JNCI Cancer Spectr (2019) 3(4):pkz049. doi: 10.1093/jncics/pkz049
154. Werner S, Heidrich I, Pantel K. Clinical management and biology of tumor dormancy in breast cancer. Semin Cancer Biol (2022) 78:49–62. doi: 10.1016/j.semcancer.2021.02.001
155. Zhang XH, Giuliano M, Trivedi MV, Schiff R, Osborne CK. Metastasis dormancy in estrogen receptor-positive breast cancer. Clin Cancer Res (2013) 19(23):6389–97. doi: 10.1158/1078-0432.CCR-13-0838
156. Connolly RM, Zhao F, Miller KD, Lee MJ, Piekarz RL, Smith KL, et al. E2112: Randomized phase III trial of endocrine therapy plus entinostat or placebo in hormone receptor-positive advanced breast cancer. a trial of the ECOG-ACRIN cancer research group. J Clin Oncol (2021) 39(28):3171–81. doi: 10.1200/JCO.21.00944
157. Yardley DA, Ismail-Khan RR, Melichar B, Lichinitser M, Munster PN, Klein PM, et al. Randomized phase II, double-blind, placebo-controlled study of exemestane with or without entinostat in postmenopausal women with locally recurrent or metastatic estrogen receptor-positive breast cancer progressing on treatment with a nonsteroidal aromatase inhibitor. J Clin Oncol (2013) 31(17):2128–35. doi: 10.1200/JCO.2012.43.7251
158. Andre F, Ciruelos E, Rubovszky G, Campone M, Loibl S, Rugo HS, et al. Alpelisib for PIK3CA-mutated, hormone receptor-positive advanced breast cancer. N Engl J Med (2019) 380(20):1929–40. doi: 10.1056/NEJMoa1813904
159. Andre F, Ciruelos EM, Juric D, Loibl S, Campone M, Mayer IA, et al. Alpelisib plus fulvestrant for PIK3CA-mutated, hormone receptor-positive, human epidermal growth factor receptor-2-negative advanced breast cancer: final overall survival results from SOLAR-1. Ann Oncol (2021) 32(2):208–17. doi: 10.1016/j.annonc.2020.11.011
160. Turner S, Chia S, Kanakamedala H, Hsu WC, Park J, Chandiwana D, et al. Effectiveness of alpelisib + fulvestrant compared with real-world standard treatment among patients with HR+, HER2-, PIK3CA-mutated breast cancer. Oncologist (2021) 26(7):e1133–42. doi: 10.1002/onco.13804
161. Howell SJ, Casbard A, Carucci M, Ingarfield K, Butler R, Morgan S, et al. Fulvestrant plus capivasertib versus placebo after relapse or progression on an aromatase inhibitor in metastatic, oestrogen receptor-positive, HER2-negative breast cancer (FAKTION): overall survival, updated progression-free survival, and expanded biomarker analysis from a randomised, phase 2 trial. Lancet Oncol (2022) 23(7):851–64. doi: 10.1016/S1470-2045(22)00284-4
162. Smyth LM, Tamura K, Oliveira M, Ciruelos EM, Mayer IA, Sablin MP, et al. Capivasertib, an AKT kinase inhibitor, as monotherapy or in combination with fulvestrant in patients with AKT1 (E17K)-mutant, ER-positive metastatic breast cancer. Clin Cancer Res (2020) 26(15):3947–57. doi: 10.1158/1078-0432.CCR-19-3953
163. Akman M, Belisario DC, Salaroglio IC, Kopecka J, Donadelli M, De Smaele E, et al. Hypoxia, endoplasmic reticulum stress and chemoresistance: dangerous liaisons. J Exp Clin Cancer Res (2021) 40(1):28. doi: 10.1186/s13046-020-01824-3
164. McAleese CE, Choudhury C, Butcher NJ, Minchin RF. Hypoxia-mediated drug resistance in breast cancers. Cancer Lett (2021) 502:189–99. doi: 10.1016/j.canlet.2020.11.045
165. Yong L, Tang S, Yu H, Zhang H, Zhang Y, Wan Y, et al. The role of hypoxia-inducible factor-1 alpha in multidrug-resistant breast cancer. Front Oncol (2022) 12:964934. doi: 10.3389/fonc.2022.964934
166. Najafi M, Goradel NH, Farhood B, Salehi E, Solhjoo S, Toolee H, et al. Tumor microenvironment: Interactions and therapy. J Cell Physiol (2019) 234(5):5700–21. doi: 10.1002/jcp.27425
167. Swayden M, Chhouri H, Anouar Y, Grumolato L. Tolerant/Persister cancer cells and the path to resistance to targeted therapy. Cells (2020) 9(12): 2601. doi: 10.3390/cells9122601
168. Uribe ML, Marrocco I, Yarden Y. EGFR in cancer: Signaling mechanisms, drugs, and acquired resistance. Cancers (Basel) (2021) 13(11): 2748. doi: 10.3390/cancers13112748
169. Jena BC, Mandal M. The emerging roles of exosomes in anti-cancer drug resistance and tumor progression: An insight towards tumor-microenvironment interaction. Biochim Biophys Acta Rev Cancer (2021) 1875(1):188488. doi: 10.1016/j.bbcan.2020.188488
170. Lowry MC, Gallagher WM, O'Driscoll L. The role of exosomes in breast cancer. Clin Chem (2015) 61(12):1457–65. doi: 10.1373/clinchem.2015.240028
171. Namee NM, O'Driscoll L. Extracellular vesicles and anti-cancer drug resistance. Biochim Biophys Acta Rev Cancer (2018) 1870(2):123–36. doi: 10.1016/j.bbcan.2018.07.003
172. Yu S, Wei Y, Xu Y, Zhang Y, Li J, Zhang J. Extracellular vesicles in breast cancer drug resistance and their clinical application. Tumour Biol (2016) 37(3):2849–61. doi: 10.1007/s13277-015-4683-5
173. Kandouz M, Batist G. Gap junctions and connexins as therapeutic targets in cancer. Expert Opin Ther Targets (2010) 14(7):681–92. doi: 10.1517/14728222.2010.487866
174. Sinyuk M, Mulkearns-Hubert EE, Reizes O, Lathia J. Cancer connectors: Connexins, gap junctions, and communication. Front Oncol (2018) 8:646. doi: 10.3389/fonc.2018.00646
175. Clarke R, Ressom HW, Wang A, Xuan J, Liu MC, Gehan EA, et al. The properties of high-dimensional data spaces: implications for exploring gene and protein expression data. Nat Rev Cancer (2008) 8(1):37–49. doi: 10.1038/nrc2294
176. Hertz DL, Henry NL, Rae JM. Germline genetic predictors of aromatase inhibitor concentrations, estrogen suppression and drug efficacy and toxicity in breast cancer patients. Pharmacogenomics (2017) 18(5):481–99. doi: 10.2217/pgs-2016-0205
177. Longo R, D'Andrea M, Sarmiento R, Gasparini G. Pharmacogenetics in breast cancer: focus on hormone therapy, taxanes, trastuzumab and bevacizumab. Expert Opin Investig Drugs (2010) 19 Suppl 1:S41–50. doi: 10.1517/13543781003732701
178. Sini V, Botticelli A, Lunardi G, Gori S, Marchetti P. Pharmacogenetics and aromatase inhibitor induced side effects in breast cancer patients. Pharmacogenomics (2017) 18(8):821–30. doi: 10.2217/pgs-2017-0006
179. Aymanns C, Keller F, Maus S, Hartmann B, Czock D. Review on pharmacokinetics and pharmacodynamics and the aging kidney. Clin J Am Soc Nephrol (2010) 5(2):314–27. doi: 10.2215/CJN.03960609
180. Czock D, Keller F, Rasche FM, Haussler U. Pharmacokinetics and pharmacodynamics of systemically administered glucocorticoids. Clin Pharmacokinet (2005) 44(1):61–98. doi: 10.2165/00003088-200544010-00003
181. Macosko EZ, Basu A, Satija R, Nemesh J, Shekhar K, Goldman M, et al. Highly parallel genome-wide expression profiling of individual cells using nanoliter droplets. Cell (2015) 161(5):1202–14. doi: 10.1016/j.cell.2015.05.002
182. Ren X, Kang B, Zhang Z. Understanding tumor ecosystems by single-cell sequencing: promises and limitations. Genome Biol (2018) 19(1):211. doi: 10.1186/s13059-018-1593-z
183. DeVita VT Jr., Young RC, Canellos GP. Combination versus single agent chemotherapy: a review of the basis for selection of drug treatment of cancer. Cancer (1975) 35(1):98–110. doi: 10.1002/1097-0142(197501)35:1<98::AID-CNCR2820350115>3.0.CO;2-B
184. Ali A, Davidson S, Fraenkel E, Gilmore I, Hankemeier T, Kirwan JA, et al. Single cell metabolism: current and future trends. Metabolomics (2022) 18(10):77. doi: 10.1007/s11306-022-01934-3
185. Sivanich MK, Gu TJ, Tabang DN, Li L. Recent advances in isobaric labeling and applications in quantitative proteomics. Proteomics (2022) 19-20:e2100256. doi: 10.1002/pmic.202100256
Keywords: heterogeneity, endocrine therapy, drug resistance, breast cancer, systems biology
Citation: Andrade de Oliveira K, Sengupta S, Yadav AK and Clarke R (2023) The complex nature of heterogeneity and its roles in breast cancer biology and therapeutic responsiveness. Front. Endocrinol. 14:1083048. doi: 10.3389/fendo.2023.1083048
Received: 28 October 2022; Accepted: 02 February 2023;
Published: 23 February 2023.
Edited by:
Luisa Helguero, University of Aveiro, PortugalReviewed by:
Bela Ozsvari, University of Salford, United KingdomClodia Osipo, Loyola University Chicago, United States
Copyright © 2023 Andrade de Oliveira, Sengupta, Yadav and Clarke. This is an open-access article distributed under the terms of the Creative Commons Attribution License (CC BY). The use, distribution or reproduction in other forums is permitted, provided the original author(s) and the copyright owner(s) are credited and that the original publication in this journal is cited, in accordance with accepted academic practice. No use, distribution or reproduction is permitted which does not comply with these terms.
*Correspondence: Robert Clarke, clarker@umn.edu