- 1Department of Emergency, Hunan Provincial People’s Hospital, The First Affiliated Hospital of Hunan Normal University, Changsha, Hunan, China
- 2Clinical Research Center for Emergency and Critical Care in Hunan Province, Hunan Provincial People’s Hospital, The First Affiliated Hospital of Hunan Normal University, Changsha, China
- 3Department of Cardiology, Hunan Provincial People’s Hospital, The First Affiliated Hospital of Hunan Normal University, Changsha, China
- 4Clinical Research Center for Heart Failure of Hunan Province, Hunan Provincial People’s Hospital, The First Affiliated Hospital of Hunan Normal University, Changsha, China
- 5Emergency Department, The First Affiliated Hospital of Hunan Normal University, Changsha, China
- 6Institute of Emergency Medicine, Hunan Provincial People’s Hospital, The First Affiliated Hospital of Hunan Normal University, Changsha, China
Objective: Sepsis is a life-threatening condition secondary to infection that evolves into a dysregulated host response and is associated with acute organ dysfunction. Sepsis-induced cardiac dysfunction is one of the most complex organ failures to characterize. This study performed comprehensive metabolomic profiling that distinguished between septic patients with and without cardiac dysfunction.
Method: Plasma samples collected from 80 septic patients were analysed by untargeted liquid chromatography-mass spectrometry (LC-MS) metabolomics. Principal component analysis (PCA), partial least squares discrimination analysis (PLS-DA), and orthogonal partial least square discriminant analysis (OPLS-DA) were applied to analyse the metabolic model between septic patients with and without cardiac dysfunction. The screening criteria for potential candidate metabolites were as follows: variable importance in the projection (VIP) >1, P < 0.05, and fold change (FC) > 1.5 or < 0.7. Pathway enrichment analysis further revealed associated metabolic pathways. In addition, we constructed a subgroup metabolic analysis between the survivors and non-survivors according to 28-day mortality in the cardiac dysfunction group.
Results: Two metabolite markers, kynurenic acid and gluconolactone, could distinguish the cardiac dysfunction group from the normal cardiac function group. Two metabolites, kynurenic acid and galactitol, could distinguish survivors and non-survivors in the subgroup analysis. Kynurenic acid is a common differential metabolite that could be used as a candidate for both diagnosis and prognosis for septic patients with cardiac dysfunction. The main associated pathways were amino acid metabolism, glucose metabolism and bile acid metabolism.
Conclusion: Metabolomic technology could be a promising approach for identifying diagnostic and prognostic biomarkers of sepsis-induced cardiac dysfunction.
Introduction
In critically ill patients, sepsis remains a common condition that is associated with high mortality and substantial morbidity (1). Cardiac dysfunction caused by sepsis, referred to as sepsis-induced cardiomyopathy, has long been a subject of interest because mortality can be greatly increased if sepsis is combined with this complication (2). Thus, it is critical to assess the probability of septic cardiomyopathy and predict clinical outcome in the early stage of patient admission. However, the prognostic and therapeutic importance of physiological changes observed in sepsis-induced cardiac dysfunction remains poorly understood. In addition, although early application of ultrasonography is helpful for the diagnosis of septic cardiomyopathy, it lacks the basis for prognostic prediction. There is also no robust evidence to identify the association of troponin and brain natriuretic peptide (BNP) with septic cardiomyopathy (3). Troponins, including troponin T and troponin I, are widely used markers for myocardial injury. The proportion of patients with elevated troponin T is up to 80% in sepsis (4), but no evidence has been found to identify the relationship between left ventricular (LV) dysfunction and troponin T (5). Another study reported that troponin I level was also not an independent predictor of mortality rate in sepsis (6). BNP, secreted by cardiomyocytes of the ventricle, is dependent on LV filling pressures and myocardial wall stretch (7). Julien et al. reported that BNP plasma levels are possibly useful for detecting myocardial dysfunction, but persistence of high BNP levels is associated with age and acute kidney injury (8). These confounders should also be considered.
Metabolomics technology is an emerging omics science developed after genomics and proteomics. As an important part of systems biology, this technology qualitatively and quantitatively analyses a broad spectrum of small molecule metabolites, especially energy metabolites, in organisms (9). The metabolome influences cellular physiology by regulating other levels of “omics”, including the genome, epigenome, transcriptome and proteome (10). Recent advances in metabolomic technology have led to its increasing application in biomedicine. In particular, the application of metabolomics provides a strategic advantage for elucidating the mechanism of disease, discovering biomarkers, and innovating new therapeutics (11).
Emerging studies highlight the potential application of metabolomics technology in sepsis (12). Feng and colleagues reported that sepsis-induced acute kidney injury is accompanied by an increased oxygen consumption, systemic aerobic and anaerobic metabolism, and abnormal fatty acid metabolism (13). A preliminary study demonstrated that 2-ethyl-2-hydroxybutyric acid regulates the expression of programmed cell death protein-1 on the surface of CD4+ T cells through the action of interleukin-2 or lactate, thereby affecting the prognosis of septic patients (14). However, to our knowledge, no study has been reported to clarify metabolomics changes in sepsis-induced cardiac dysfunction.
In this study, nontargeted metabolomic profiling was utilized to investigate metabolomic alterations in patients with sepsis-induced cardiac dysfunction and to provide evidence for early metabolic biomarkers for diagnosis and prognosis. Moreover, related metabolic pathways were also explored.
Materials and methods
Study design and participants
Between November 2020 and March 2022, adults who had been admitted to the emergency department of Hunan Provincial People’s Hospital were selected for the study. Patients with sepsis were included in the study within the first 24 hours after admission. The inclusion criteria of patients were based on Sepsis 3.0 in the Third International Consensus Conference. Sepsis was defined as life-threatening organ dysfunction induced by a dysregulated host response to infection and a Sequential Organ Failure Assessment (SOFA) score ≥ 2 (15). Sepsis-induced cardiac dysfunction was defined as impaired but reversible cardiac dysfunction under echocardiography, including LV systolic dysfunction, LV diastolic dysfunction, and right ventricle (RV) systolic dysfunction (16). Patients who met the exclusion criteria were as follows: (1) age < 18 years old; (2) pregnancy; and (3) history of heart disease, such as acute coronary ischaemia, LV insufficiency, dilated cardiomyopathy, hypertrophic cardiomyopathy, valvular heart disease, or recurrent arrhythmia (5). The study complied with the guidelines of the Declaration of Helsinki and the Conference for Coordination of Clinical Practice and was approved by the Ethics Committee of Hunan Provincial People’s Hospital. Informed consent was obtained from all participants.
Blood sampling
Fasting venous blood was obtained from patients diagnosed with sepsis within 24 hours of admission. Blood samples were collected in ethylenediaminetetraacetic acid (EDTA) anticoagulant tubes and centrifuged for 10 min at 4°C and 3,000 rpm to obtain plasma. The fasting plasma samples were stored in a -80°C freezer and kept frozen until metabolomic analysis.
Metabolite extraction and data processing
One hundred microlitres of the plasma sample was transferred to a 1.5 mL Eppendorf tube, and 20 μL of L-2-chlorophenylalanine (0.3 mg/mL) was dissolved in methanol as an internal standard. The tube was vortexed for 10 seconds. Subsequently, 300 μL of protein precipitant (methanol and acetonitrile, 2:1, vol/vol) was added, and the mixture was vortexed for 1 min. Then, the whole sample was sonicated for 10 min in an ice-water bath and stored at -20°C for 30 min. The extract was centrifuged at 4°C (13,000 rpm) for 10 min. The supernatant of each sample was collected. Quality control samples (QCs) were prepared by mixing equal volumes of extracts from all samples.
An ACQUITY UPLC I-Class system (Waters Corporation, Milford, USA) and a VION IMS QTOF mass spectrometer (Waters Corporation, Milford, USA) were used to analyse the metabolic profiles in both ESI positive and ESI negative ion modes. The target compounds were separated by an ACQUITY UPLC BEH C18 column (1.7 μm, 2.1 × 100 mm) at 45°C with 2 μL sample injection. Water and acetonitrile, both containing 0.1% formic acid, were used as mobile phases A and B, respectively. The gradient was set as follows: 0–2 min, 5% B; 4 min, 30% B; 8 min, 50% B; 10 min, 80% B; 14 min, 100% B; 15 min, 100% B; 15.1 min, 5% B and 16 min, 5% B. The flow rate was 0.35 mL/min, and the column temperature was 45 °C.
Primary and secondary mass spectrometry data were collected by a VION IMS QTOF mass spectrometer. The parameters of mass spectrometry were set as follows: a low-energy scan (CE 4 eV) and a high-energy scan (CE ramp 20–45 eV) to fragment the ions. Argon was used as the collision-induced dissociation gas; scan time: 0.2 s; interscan delay: 0.02 s; capillary voltage, 1 kV (negative mode) or 2 kV (positive mode); capillary voltage: 2.5 kV; cone voltage: 40 V; source temperature: 115°C; desolvation gas temperature: 450°C; and desolvation gas flow, 900 L/h.
The original data were analysed by Progenesis QI V2.3 (Nonlinear Dynamics, Newcastle, UK) software for baseline filtering, peak recognition, integration, retention time correction, peak alignment and normalization. Compound identification was based on the precise mass-to-charge ratio (m/z), MS2 fragments, and isotopic distribution using the Human Metabolome Database (HMDB) or Metlin, and self-built databases to do qualitative analysis. The metabolites in the self-built database are all established by standards, which contain retention time, first-level accurate mass information and second-level mass spectrum fragment information. The module pathway analysis was based on the KEGG database.
Statistical analysis
Clinical data were analysed using SPSS Statistics 25 software (IBM®, Armonk, NY, USA). Data are represented as the mean ± standard deviation or median and interquartile range. Continuous variables were compared using Student’s t test or the Mann−Whitney U test. Student’s t test is used when two samples are small and meet the conditions of normal distribution and homogeneity of variance. The Mann−Whitney U test was used when the samples did not meet the conditions of normal distribution and homogeneity of variance. Categorical variables between the two groups were compared by Fisher’s exact probability method. P < 0.05 was considered statistically significant.
The metabolic profiles were imported into R for principal component analysis (PCA) to observe the overall distribution among the samples and the stability of the entire analysis process. Partial least squares discriminant analysis (PLS-DA) and orthogonal partial least squares discriminant analysis (OPLS-DA) were used to distinguish differential metabolites between groups. To prevent overfitting, 7-fold cross-validation and 200 response permutation tests were utilized to evaluate the quality of the model. Variable importance of projection (VIP) values obtained from the OPLS-DA model were used to rank the overall contribution of each variable to group discrimination. A two-tailed Student’s t test was further used to verify whether the differences in metabolites between groups were significant. Differential metabolites were selected with VIP >1.0, P < 0.05, and fold change (FC) >1.5 or <0.7. Binary logistic regression analysis was constructed to screen independent risk factors. Receiver operating characteristic (ROC) curves were constructed to evaluate the diagnostic ability of differential metabolites between the tested groups.
Results
Study design and clinical synopsis
Initially, a total of 132 patients were enrolled. Based on the exclusion criteria, 37 patients with normal cardiac function and 43 patients with cardiac dysfunction were eventually included in our study. The flow chart is shown in Figure 1.
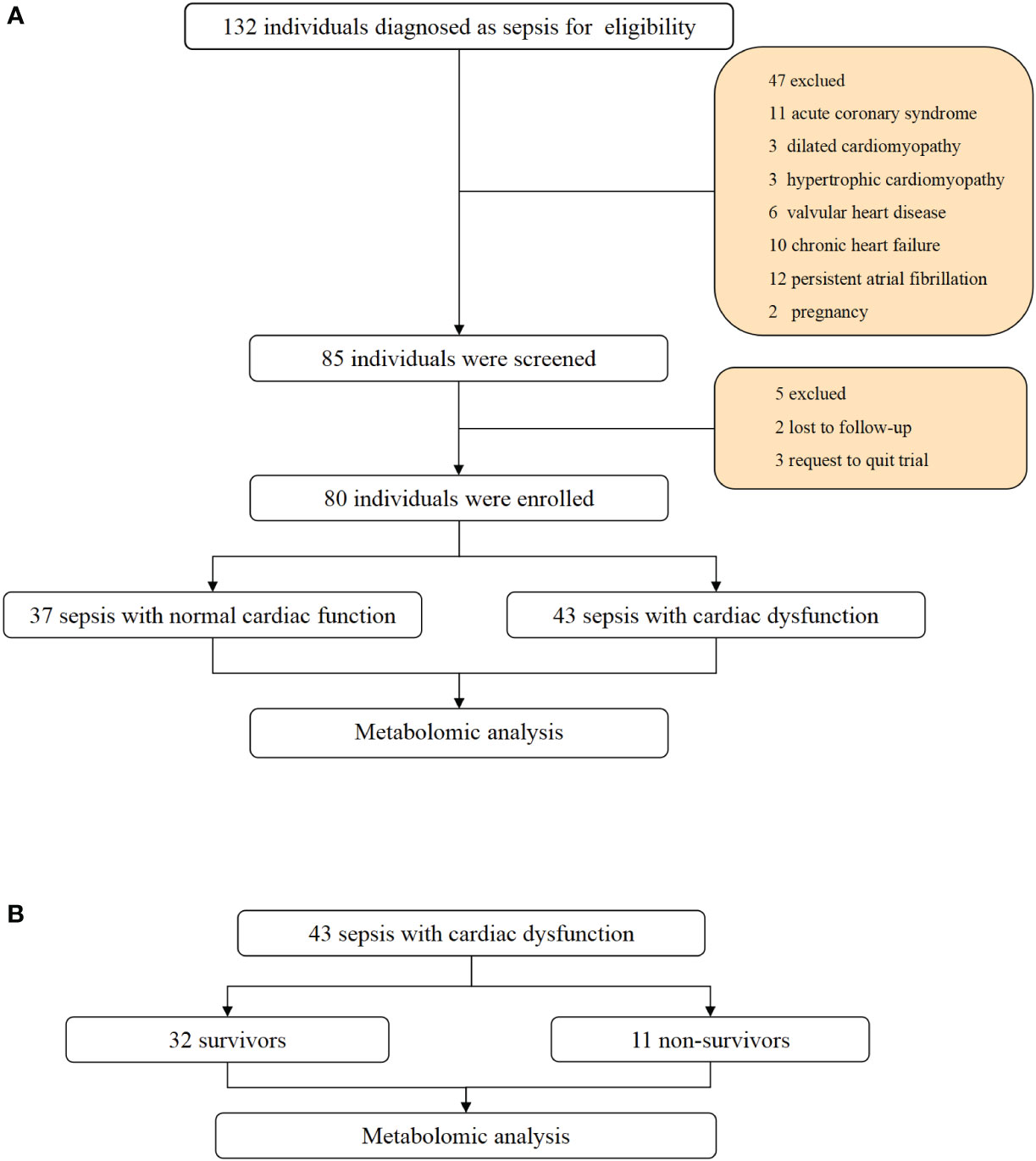
Figure 1 Flowchart and study design. (A) Flowchart of individuals enrollment and study design in total patients with sepsis. (B) flowchart of subgroup analysis between survivors and non-survivors in sepsis with cardiac dysfunction.
The clinical characteristics are shown in Table 1. Cardiac dysfunction is common in sepsis, affecting 54% (n=43) of patients. No significant differences in sex, age, comorbidities, or site of infection were noted between the two groups. Lactate levels and troponin I levels were higher in the cardiac dysfunction group (P=0.037 and P=0.023, respectively). N-terminal pro-brain natriuretic peptide (NT-proBNP) was elevated in the cardiac dysfunction group, but the difference was not statistically significant (P=0.051). The 28-day mortality was increased in the cardiac dysfunction group, although the difference was not statistically significant (P=0.178). ROC curves were constructed to determine the predictive value of variables in diagnosing sepsis-induced cardiac dysfunction. The AUC for lactate level (AUC 0.636, SE 95% confidence interval 0.513 to 0.759, P = 0.037) was equivalent to troponin I (AUC 0.647, SE 95% confidence interval 0.519 to 0.775, P = 0.024) and NT-proBNP (AUC 0.627, SE, 95% confidence interval 0.505 to 0.750, P = 0.051). The three AUCs were less than 0.75, indicating that the three variables were not robust enough for diagnosing sepsis-induced cardiac dysfunction.
Metabolite analysis and model validation in the normal cardiac function group and cardiac dysfunction group
The PCA model in the positive and negative ion modes showed that the instrument was stable throughout the experiment. The red squares, green triangles, and green circles in the figure represent the cardiac dysfunction group, normal cardiac function group, and QC group, respectively (Figures 2A, B).
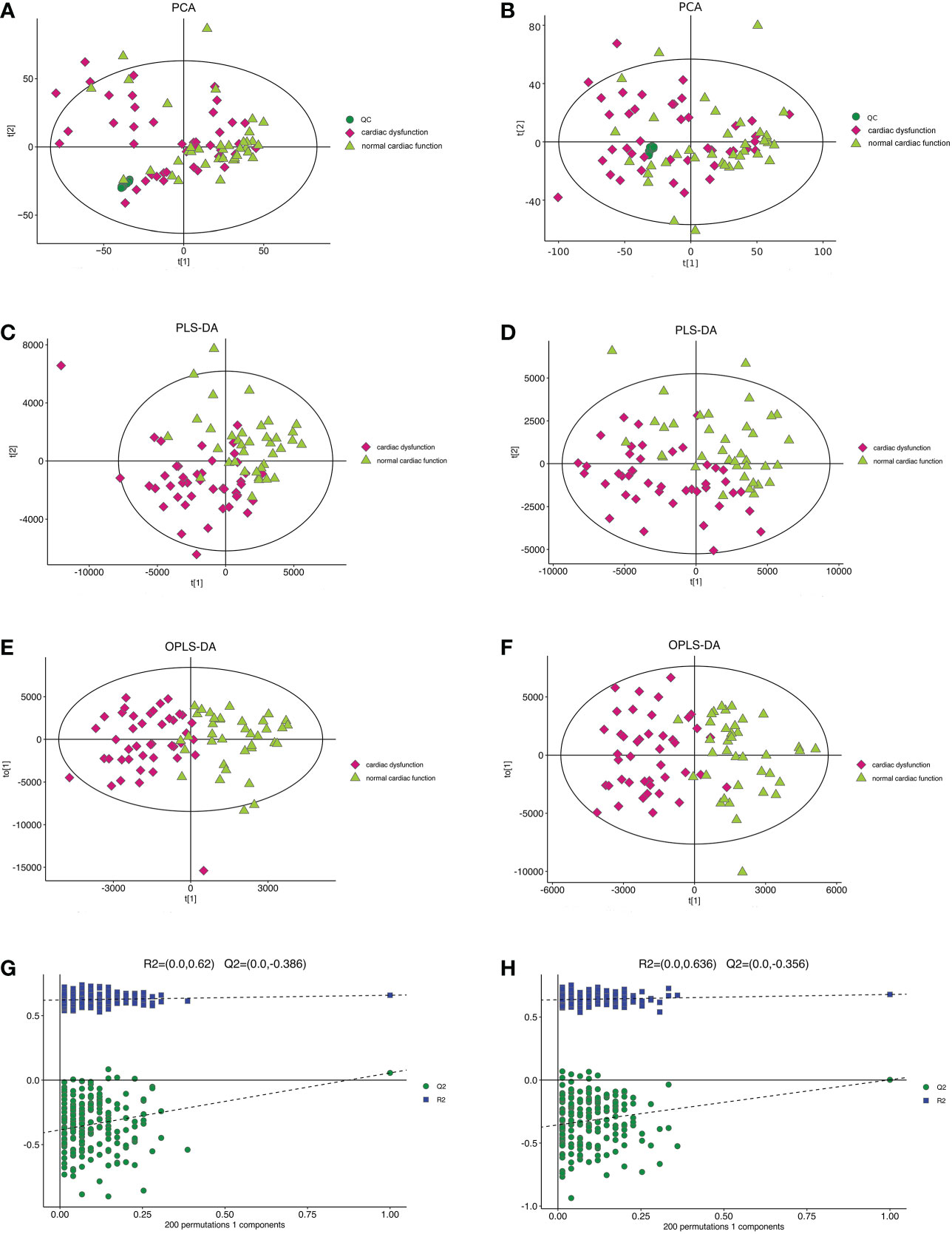
Figure 2 The multidimensional results in positive and negative ionization mode in total patients. (A) PCA score plot in positive ionization mode [R2X (cum) = 0.519, Q2 (cum) = 0.442]. (B) PCA score plot in negative ionization mode [R2X (cum) = 0.502, Q2 (cum) = 0.439]. (C) PLS-DA score plot in positive ionization mode [R2X (cum) = 0.219, R2Y (cum) = 0.756, Q2 (cum) = 0.563]. (D) PLS-DA score plot in negative ionization mode [R2X(cum) = 0.249, R2Y(cum) = 0.778 Q2(cum) = 0.595]. (E) OPLS-DA score plot in positive ionization mode [R2X (cum) = 0.219, R2Y (cum) = 0.756, Q2 (cum) = 0.459]. (F) OPLS-DA score plot in negative ionization mode [R2X(cum) = 0.249, R2Y(cum) = 0.778 Q2(cum) = 0.398]. (G) Permutation test in positive ionization mode (R2 = 0.620, Q2= -0.386). (H) Permutation test in negative ionization mode (R2 = 0.636, Q2= -0.356). PCA, principal component analysis; PLS-DA, partial least squares discrimination analysis; OPLS-DA, orthogonal partial least squares-discriminant analysis.
Then, the PLS-DA method was applied to analyse the metabolite profile: in the positive ion mode, there were significant differences between the normal cardiac function group and the cardiac dysfunction group (CV-ANOVA,F=17.796, P<0.001]; in the negative ion mode, the two groups also exhibited significant differences (CV-ANOVA, F=19.066, P<0.001) (Figures 2C, D). These findings indicate that the PLS-DA model could be used to distinguish septic patients with cardiac dysfunction from those with normal cardiac function.
To achieve the greatest separation of differential metabolites between the two groups, OPLS-DA was performed based on values of VIP > 1. OPLS-DA demonstrated notable separation in metabolic profiles between the cardiac dysfunction group and the normal cardiac function group (Figures 2E, F). The permutation chart verified the validity of the model. R2 and Q2 were generated by permutation test. When R2 > 0, Q2 < 0, it indicates that the model is reliable (17). The results of the permutation chart showed that the models were reliable (Figures 2G, H).
Screening of differential metabolites between the normal cardiac function group and cardiac dysfunction group
Univariate statistical analysis was applied to screen differential metabolites. A volcano plot was utilized to visualize the P value, VIP and fold change value. The red dots represent the upregulated metabolites, while the blue dots represent the downregulated metabolites (Figures 3A, C). The heatmap intuitively displays differential metabolites in different samples. In the heatmap of positive and negative ion modes, the Z scores of potential biomarkers were labelled in terms of the types of metabolites (Figures 3B, D). The most differential metabolites, which can be candidate for biomarkers, are presented in Table 2. The screening criteria for potential biomarkers were as follows: VIP >1, P < 0.05, and FC > 1.5 or < 0.7 (17). Seventeen metabolites differed significantly between the normal cardiac function group and the cardiac dysfunction group.
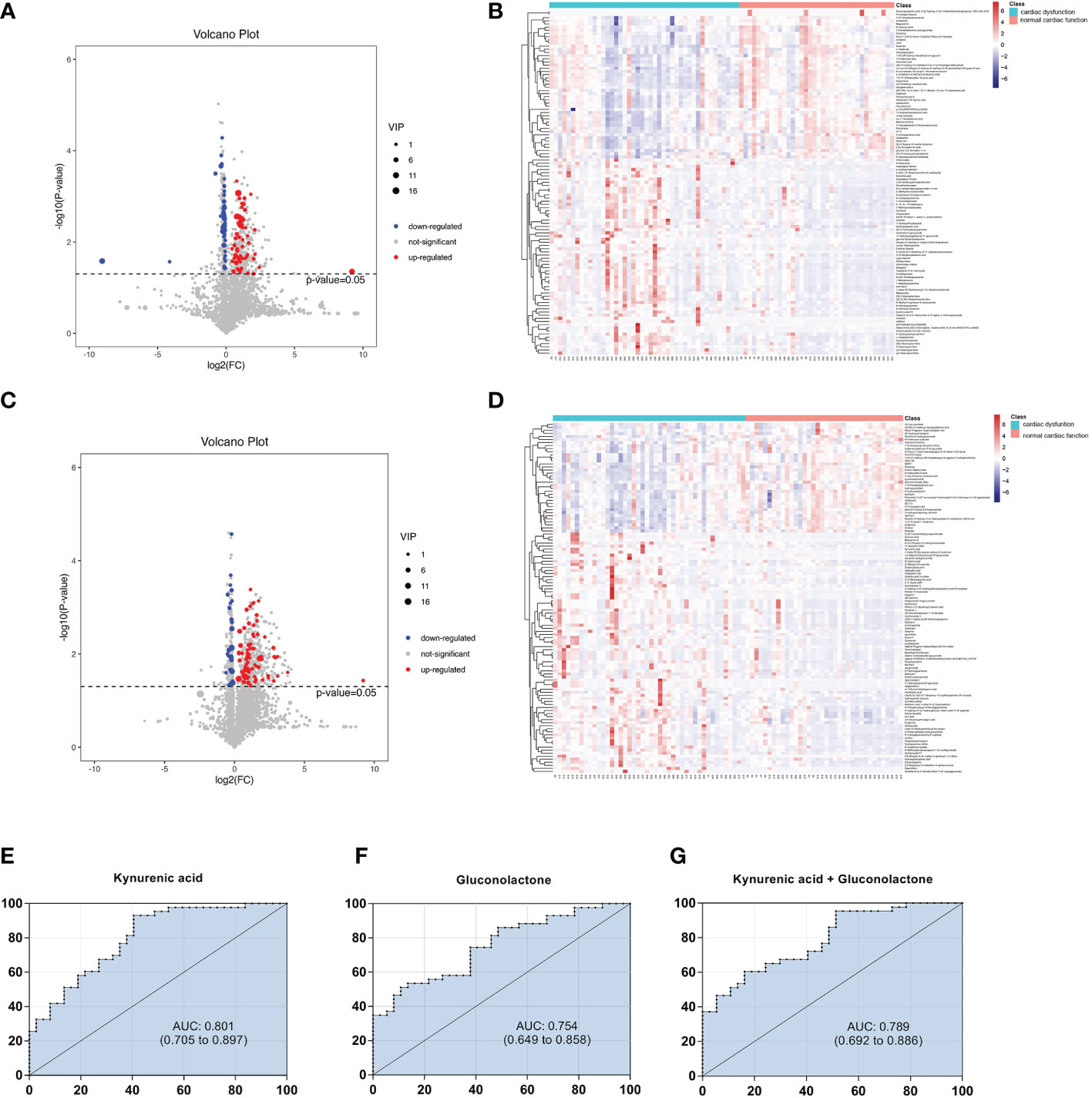
Figure 3 Volcano diagrams and heatmaps in total patients. (A) Volcano map in positive ionization mode. (B) Heatmap in positive ionization mode. (C) Volcano map in negative ionization mode. (D) Heatmap in negative ionization mode. In the volcano diagrams, the red dots represent up-regulated metabolites, and the gray dots represent no significant difference, and the blue dots represent down-regulated metabolites. (E–G) ROC curve analysis of the ability of differential metabolites to predict sepsis with cardiac dysfunction.
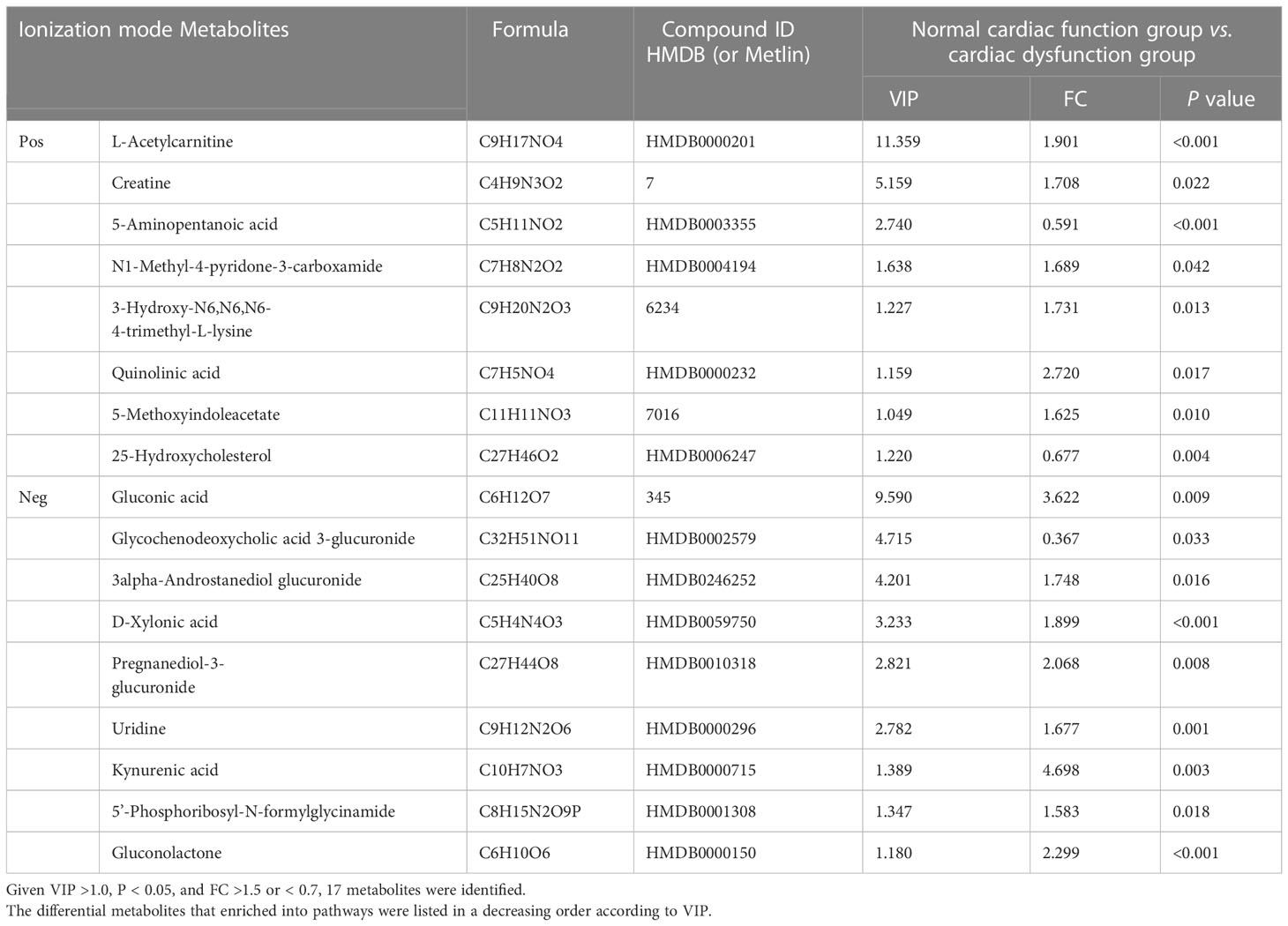
Table 2 In two ionization modes, differential metabolites between normal cardiac function group and cardiac dysfunction group.
Subsequently, we constructed binary logistic regression analysis to screen risk factors and ROC curve analysis to evaluate the predictive ability in the differential metabolites. Kynurenic acid, gluconolactone, 3-hydroxy-N6,N6,N6-trimethyl-L-lysine and 25-hydroxycholesterol were indentified as independent risk factors for sepsis-induced cardiac dysfunction, and the AUC values of kynurenic acid and gluconolactone were > 0.75 (Supplymentary Tables 1, 2). The AUCs for kynurenic acid and gluconolactone were 0.801, 0.754, respectively. We also constructed ROC analysis combining kynurenic acid and gluconolactone. However, the AUC for combining kynurenic acid and gluconolactone was 0.789, which was not superior to kynurenic acid (Figures 3E–G).
Differential metabolic pathways between the normal cardiac function group and cardiac dysfunction group
KEGG and HMDB were applied to analyse cardiac dysfunction-associated metabolites, and the results were submitted to MetaboAnalyst. In the top 20 metabolic pathway enrichment maps, significance is indicated by logarithm of the reciprocal of P value based on 10 (Figure 4A). In the top 20 bubble chart, the colour and size of each circle are determined by P values. (Figure 4B). The following three important metabolic pathways were identified: tryptophan metabolism, the pentose phosphate pathway, and lysine degradation. The results of pathway enrichment analysis for these differential metabolites are summarized in Supplementary Table 3.
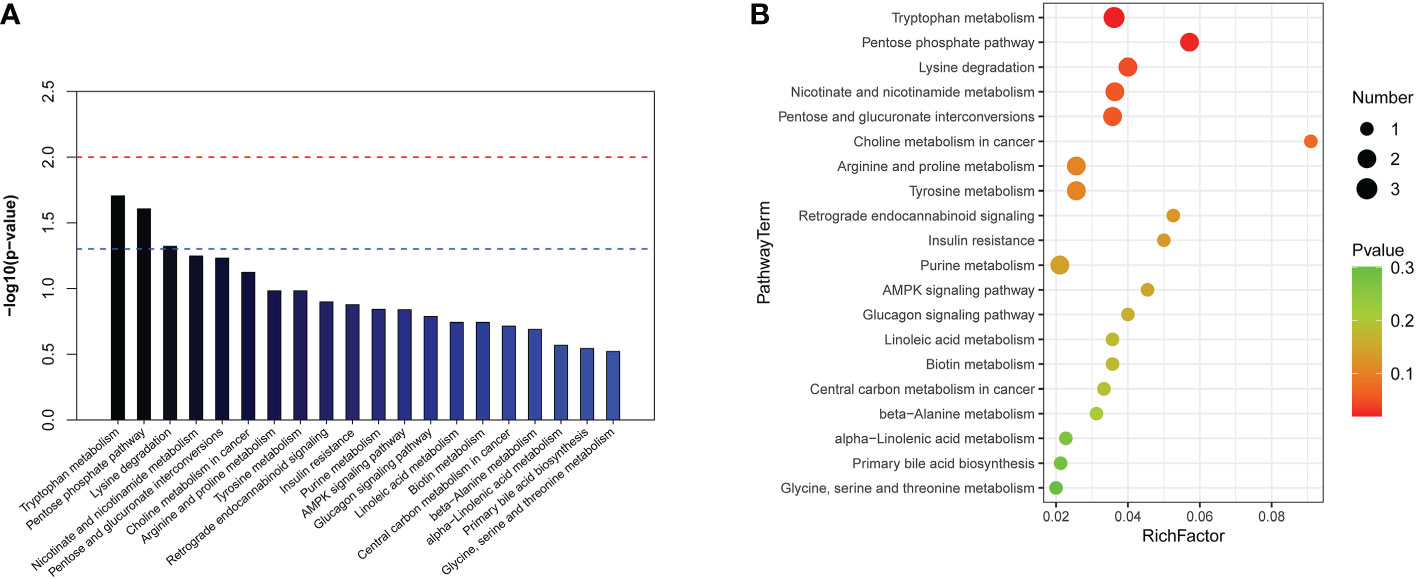
Figure 4 The significantly enriched pathways involved in total patients. (A) Top-20 metabolic pathway enrichment map.The red line indicates a P value of 0.01, and the blue line indicates a P value of 0.05. When the top of the bar is higher than the blue line, the pathway is considered to be significant. (B) Top-20 bubble chart. The colour changed from green to red indicates that significance increases in turn; the larger the point is, the greater the number of metabolites enriched in the pathway.
Metabolite analysis and model validation in the survivors and non-survivors of cardiac dysfunction group
It has been reported that mortality can be increased if sepsis is combined with cardiac dysfunction (2). In our study, 28-day mortality was increased in the cardiac dysfunction group, although the difference was not statistically significant due to the small sample size. It is crucial for physicians to predict clinical outcome exactly in the early stage of patient admission; thus, clarifying the metabolic changes in non-survivors is of great importance. A comparison between the survivors and non-survivors in the cardiac dysfunction group was performed. The PCA, PLS-DA and OPLS-DA models distinguished the metabolic profiles of the survivors from those of the non-survivors. The blue squares, red triangles and green circles in the figure represent the non-survivors, survivors and QC samples, respectively. PLS-DA analysis of the metabolite profile of the plasma sample: results in the positive ion mode suggested that there were significant differences between survivors and non-survivors (CV-ANOVA, F=23.301, P<0.001); in the negative ion mode, the two groups also exhibited significant differences (CV-ANOVA, F=26.217, P<0.001). In the OPLS-DA model, permutation tests showed that the model is reliable. (Figures 5A–H).
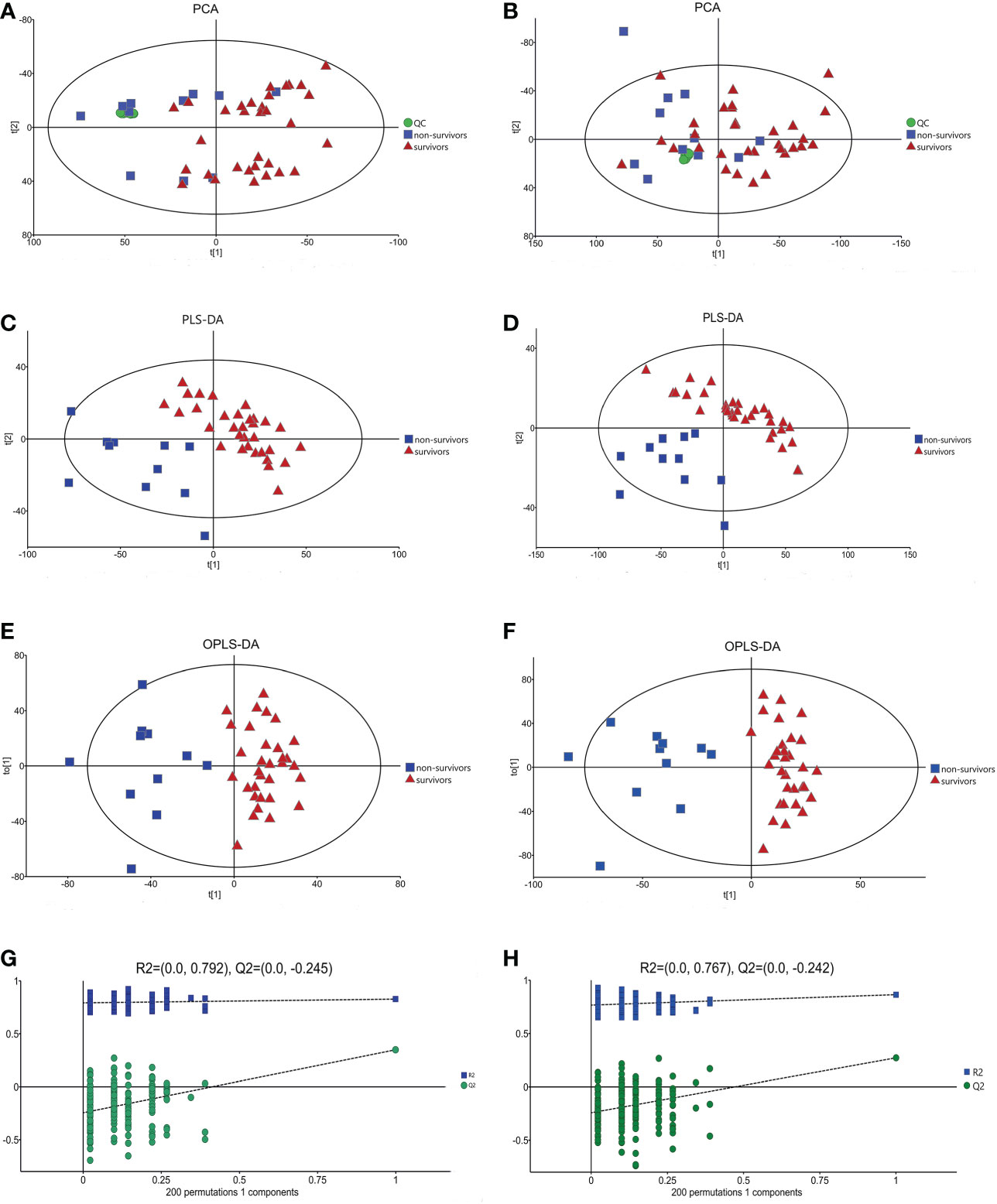
Figure 5 The multidimensional results in positive and negative ionization between survivors and non-surviviors of cardiac dysfunction group. (A) PCA score plot in positive ionization mode [R2X (cum) = 0.497, Q2 (cum) = 0.401]. (B) PCA score plot in negative ionization mode [R2X (cum) = 0.507, Q2 (cum) = 0.369]. (C) PLS-DA score plot in positive ionization mode [R2X (cum) = 0.409, R2Y (cum) = 0.787 Q2 (cum) = 0.582]. (D) PLS-DA score plot in negative ionization mode [R2X(cum) = 0.447, R2Y(cum) = 0.795, Q2(cum) = 0.576]. (E) OPLS-DA score plot in positive ionization mode [R2X (cum) = 0.409, R2Y (cum) = 0.787 Q2 (cum) = 0.483]. (F) OPLS-DA score plot in negative ionization mode [R2X(cum) = 0.447, R2Y(cum) = 0.795, Q2(cum) = 0.462]. (G) Permutation test in positive ionization mode(R2 = 0.792, Q2= -0.245). (H) Permutation test in negative ionization mode(R2 = 0.767, Q2= -0.242).
Screening of differential metabolites between the survivors and non-survivors of cardiac dysfunction group
Volcano maps and heatmaps were generated (Figures 6A–D). The most differential metabolites are presented in Table 3 following these criteria: VIP >1, P < 0.05, and FC > 1.5 or < 0.7. Twenty-five metabolites differed significantly between the survivors and non-survivors.
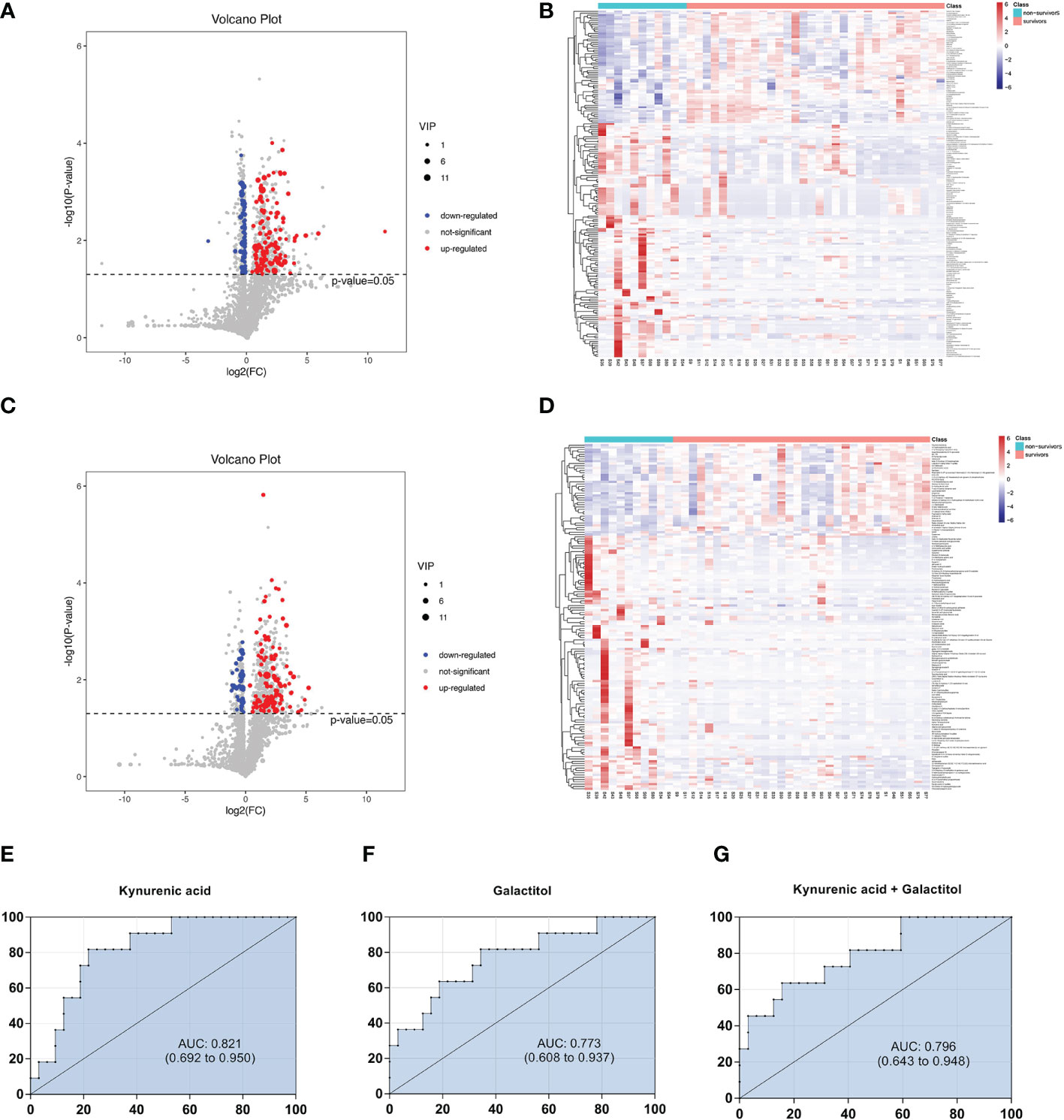
Figure 6 Volcano diagrams and heatmaps between survivors and non-surviviors of cardiac dysfunction group. (A) Volcano map in positive ionization mode. (B) Heatmap in positive ionization mode. (C) Volcano map in negative ionization mode. (D) Heatmap in negative ionization mode. (E–G) ROC curve analysis of the ability of differential metabolites to predict 28-mortality in sepsis with cardiac dysfunction.
Then, we constructed binary logistic regression analysis to screen risk factors and ROC curve analysis to evaluate the predictive ability. Only kynurenic acid and galactitol were indentified as independent risk factors for 28-day mortality in sepsis-induced cardiac dysfunction and the AUC values were > 0.75 (Supplementary Tables 4, 5).The AUCs for kynurenic acid and galactitol were 0.821, 0.773, respectively. We also constructed ROC analysis combining kynurenic acid and galactitol. Whereas, the AUC for combining kynurenic acid and alactitol was 0.796, which was not superior to kynurenic acid (Figures 6E–G).
Differential metabolic pathways between survivors and non-survivors in the cardiac dysfunction group
In the top 20 metabolic pathway enrichment maps and bubble chart (Figures 7A, B), the following three important metabolic pathways were identified as differential metabolic pathways between the survivors and non-survivors: galactose metabolism, primary bile acid biosynthesis, and phenylalanine metabolism. The results of pathway enrichment analysis for these differential metabolites are summarized in Supplementary Table 6.
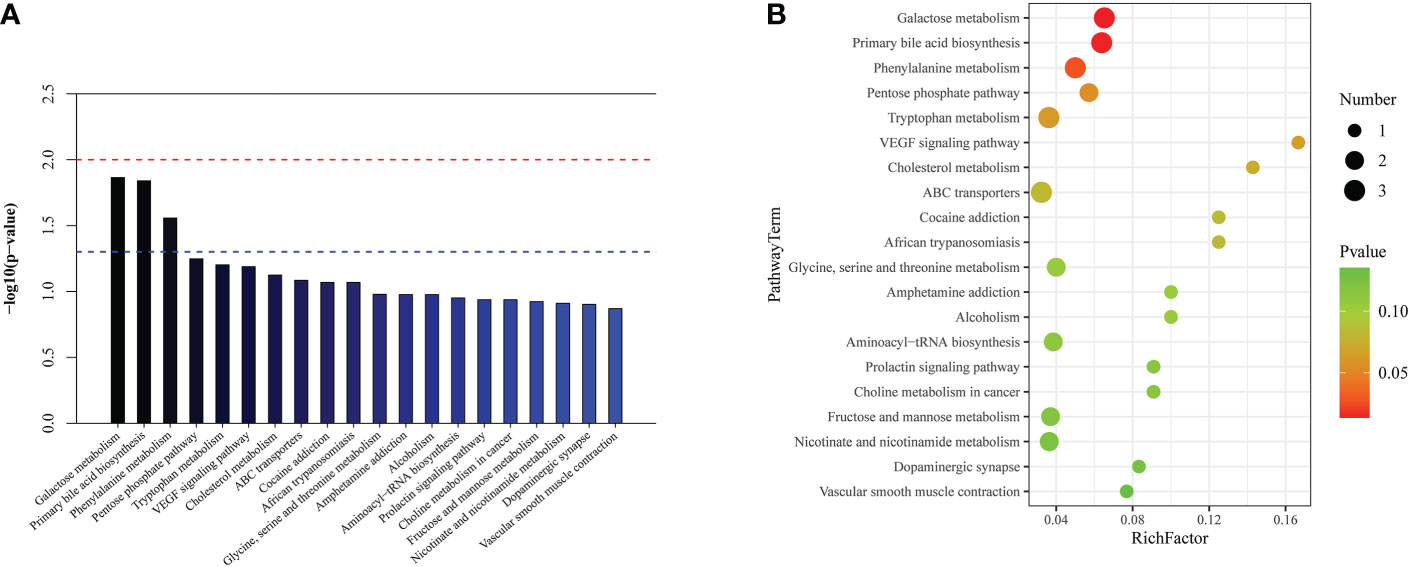
Figure 7 The significantly enriched pathways involved in cardiac dysfunction group. (A) Top-20 metabolic pathway enrichment map. (B) Top-20 bubble chart.
Discussion
Metabolomics is a rapidly developing technology that has been applied in many fields, such as biomarker investigation. To the best of our knowledge, this is the first study to perform metabolic analysis for septic patients with cardiac dysfunction. We first identified that key metabolites were changed during the development of sepsis-induced cardiac dysfunction. Two metabolite markers, kynurenic acid, and gluconolactone could distinguish the cardiac dysfunction group from the normal cardiac function group. Accordingly, we also discovered three important metabolic pathways related to sepsis-induced cardiac dysfunction, including tryptophan metabolism, pentose phosphate pathway, and lysine degradation. Next, we performed a comparison between the survivors and non-survivors in the cardiac dysfunction group. Two metabolites, including kynurenic acid and galactitol, could distinguish survivors and non-survivors in patients with sepsis-induced cardiac dysfunction. The crucial metabolic pathways are galactose metabolism, primary bile acid biosynthesis, and phenylalanine metabolism.
The most important finding was the identification of kynurenic acid as a potential metabolite biomarker for diagnosing sepsis-induced cardiac dysfunction and predicting poor outcomes. Kynurenic acid is a key degradation metabolite of tryptophan through the kynurenine pathway. Compared with kynurenine, kynurenic acid is not metabolized and stable in solution (18). Hence, kynurenic acid is reported to be a potential candidate laboratory biomarker of metabolic diseases as atherosclerosis and diabetes (19). Tryptophan is an essential amino acid that can only be provided from nutrition intake. Its metabolites play key roles in a variety of physiological processes, from cell growth and maintenance to coordinating the organism’s response to the environment (20). Tryptophan catabolism by the kynurenine pathway is a pivotal metabolic pathway closely associated with the innate immune system (21). In the early stage of sepsis, indoleamine 2,3-dioxygenase 1 (IDO1), a key enzyme that converts tryptophan to kynurenine, is activated and enhances proinflammatory effects that may result in severe tissue damage and septic shock (22). Afterwards, the accumulation of kynurenine and its metabolites, such as kynurenic acid, can induce immunotolerance or even immunoparalysis through the expansion of regulatory T cells (Tregs) and T helper 2 (Th2) cells and with the mitigation of inflammatory Th1 and Th17-mediated responses (23). Immunosuppression may contribute to the inability to overcome secondary infection and late mortality. These findings may explain why kynurenic acid levels were remarkably elevated in the cardiac dysfunction group and the non-survivors in this group. To our knowledge, this is the first study to find increased kynurenic acid in sepsis-induced cardiac injury. However, the underlying mechanism needs further investigation.
Our data also revealed that another important metabolite, gluconolactone, in the pentose phosphate pathway could be used to distinguish the cardiac dysfunction group and normal cardiac function group. Gluconolactone is considered to be a free radical scavenger. It relieves ischaemia/perfusion-induced cardiac injury although activation of extracellular signal-regulated kinase signalling (24). However, the levels of gluconolactone were not greatly increased in the non-survivors of the cardiac dysfunction group. According to relevant literature and our results, we speculate that the alteration of gluconolactone might be a protective adaptive response to overcome the cardiotoxic effects of sepsis.
Another important finding is that the level of galactitol was markedly increased in the non-survivors of the cardiac dysfunction group. ROC analysis demonstrated that it is an independent risk factor to predict 28-day mortality in cardiac dysfunction patients. Galactitol, a downstream metabolite of galactose metabolism, is generated by the hydrogenation of galactose via aldose reductase and cannot be further metabolized (25). A study also reported that galactitol emerged as the most obvious differential product in sepsis-related liver injury, suggesting that galactose metabolites are related to liver injury in the endotoxaemic state (26). However, the underlying mechanisms of galactitol and sepsis-induced organ dysfunction need further discussion. Under physiological conditions, aldose reductase has relatively low affinity for galactose. In the case of galactose accumulation, the production of galactitol increases, and multiple-aspect damage is induced. Galactitol leads to the exhaustion of nicotinamide adenine dinucleotide phosphate and the reduction of glutathione reductase activity and then acts as a metabolic toxin to the body and induces the accumulation of free radicals (25). Galactitol is incapable of diffusing across cellular membranes because of poor liposolubility, resulting in an increase in intracellular osmotic pressure (27). The free radicals together with the osmotic effect of galactitol eventually result in rupture of the cellular membrane and impairment of mitochondrial DNA and proteins (28). Further studies are needed to verify these potential mechanisms in sepsis.
Particular changes in the metabolic pathway occurred in this study. In the cardiac dysfunction group of septic patients, we found that the altered pathways were mainly involved in tryptophan metabolism, lysine degradation, and pentose phosphate pathway. In the non-survivors of the cardiac dysfunction group, we found that the changed pathways were mainly involved in galactose metabolism, primary bile acid biosynthesis and phenylalanine metabolism. Tryptophan metabolism, lysine degradation and phenylalanine metabolism belong to amino acid metabolism pathways. Both pentose phosphate pathway and galactose metabolism belong to the glucose metabolism pathways. Primary bile acid biosynthesis is associated with bile acid metabolism. Among these, tryptophan metabolism deserves special attention. Kynurenic acid, a candidate biomarker for diagnosing sepsis-induced cardiac dysfunction and predicting outcome, is an important metabolite of tryptophan metabolism. An increasing number of studies have revealed that tryptophan and its metabolites play key roles in inflammation-associated processes (29). In addition to influencing T-cell immunity and leading to immune tolerance, emerging studies have highlighted the pivotal role of tryptophan metabolism in modulating B-cell functions and humorall immunity (30). Aryl hydrocarbon receptor, a receptor that responds to tryptophan metabolites, affects the proliferation and switching of the immunoglobulin isotype in B cells (31). It has been reported that B cells and neutrophils regulate each other in bone marrow, and B cells modulate neutrophils’ tissue-damaging properties by influencing neutrophils in sepsis (32). Given the above, tryptophan metabolism has a major influence on clinical outcomes in critically ill septic patients.
Some limitations of our study should be considered. This study is a small, single-centre trial. Blood samples were analysed at only one time point. Further research with more participants will be performed to comprehensively evaluate the timing and dynamic changes in differential metabolites and metabolic pathways, providing a more precise view of changes during convalescence or deterioration. Our study also has some advantages. Currently, this is the first study to evaluate differential metabolites in septic patients with cardiac dysfunction. Patients were prospectively observed until 28 days after emergency admission. Mitochondrial function has a close relationship with cardiovascular disease. Future studies should include correlation analysis between the differential metabolites and mitochondrial bioenergetics and homeostasis to explore the underlying mechanism.
Conclusion
We used metabolomics technique to demonstrate that the metabolites of patients with sepsis-induced cardiac dysfunction change substantially and are mainly associated with the metabolic pathways of amino acid metabolism, glucose metabolism and bile acid metabolism. We also clearly distinguished septic patients with and without cardiac dysfunction using metabolites. As a consequence, kynurenic acid and gluconolactone are candidate biomarkers for diagnosing sepsis-induced cardiac dysfunction; kynurenic acid and galactitol are candidate biomarkers for predicting 28-day mortality. Kynurenic acid is a common differential metabolite in the two analyses and could be used as a diagnostic and prognostic biomarker. The differential metabolites and pathways may also be useful as targets for the development of new therapies for septic patients with cardiac dysfunction.
Data availability statement
The original contributions presented in the study are included in the article/Supplementary Material. Further inquiries can be directed to the corresponding authors.
Ethics statement
The studies involving human participants were reviewed and approved by the Ethics Committee of Hunan Provincial People’s Hospital. The patients/participants provided their written informed consent to participate in this study.
Author contributions
Conceptualization, YC, WX and XH. Methodology, YC and ZL. Formal analysis, YC and ZL. Investigation and data curation, YJ and WX. Writing—original draft preparation, YC, YP and WM. Writing—review and editing, CF and JH. Funding acquisition, YC, XH. WX and XH are the master investigators of the manuscript, take responsibility for guiding the whole work, from start to publication article. All authors contributed to the article and approved the submitted version.
Funding
This work was supported by Clinical Medical Technology Innovation Guidance Program of Hunan Province [2020SK50909], Traditional Chinese Medicine Research Program of Hunan Province [C2023020], Natural Science Foundation of Hunan Province [2020JJ8082], Program of Clinical Research Center for Emergency and Critical Care in Hunan Province [2021SK4011], Major Program of Hunan Province Science and Technology Innovation [2020SK1010]
Acknowledgments
All the authors would like to express appreciation to all the patients for their cooperation. Furthermore, our thanks would go to the foundation from Clinical Medical Technology Innovation Guidance Program of Hunan Province [2020SK50909], Traditional Chinese Medicine Research Program of Hunan Province [C2023020], Natural Science Foundation of Hunan Province [2020JJ8082], Program of Clinical Research Center for Emergency and Critical Care in Hunan Province [2021SK4011], Major Program of Hunan Province Science and Technology Innovation [2020SK1010]
Conflict of interest
The authors declare that the research was conducted in the absence of any commercial or financial relationships that could be construed as a potential conflict of interest.
Publisher’s note
All claims expressed in this article are solely those of the authors and do not necessarily represent those of their affiliated organizations, or those of the publisher, the editors and the reviewers. Any product that may be evaluated in this article, or claim that may be made by its manufacturer, is not guaranteed or endorsed by the publisher.
Supplementary material
The Supplementary Material for this article can be found online at: https://www.frontiersin.org/articles/10.3389/fendo.2023.1060470/full#supplementary-material
References
1. Cecconi M, Evans L, Levy M, Rhodes A. Sepsis and septic shock. LANCET (2018) 392(10141):75–87. doi: 10.1016/S0140-6736(18)30696-2
2. Beesley SJ, Weber G, Sarge T, Nikravan S, Grissom CK, Lanspa MJ, et al. Septic cardiomyopathy. Crit Care Med (2018) 46(4):625–34. doi: 10.1097/CCM.0000000000002851
3. Ehrman RR, Sullivan AN, Favot MJ, Sherwin RL, Reynolds CA, Abidov A, et al. Pathophysiology, echocardiographic evaluation, biomarker findings, and prognostic implications of septic cardiomyopathy: A review of the literature. Crit Care (2018) 22(1):112. doi: 10.1186/s13054-018-2043-8
4. Vasile VC, Chai H-S, Abdeldayem D, Afessa B, Jaffe AS. Elevated cardiac troponin T levels in critically ill patients with sepsis. Am J OF Med (2013) 126(12):1114–21. doi: 10.1016/j.amjmed.2013.06.029
5. Pulido JN, Afessa B, Masaki M, Yuasa T, Gillespie S, Herasevich V, et al. Clinical spectrum, frequency, and significance of myocardial dysfunction in severe sepsis and septic shock. MAYO CLINIC Proc (2012) 87(7):620–8. doi: 10.1016/j.mayocp.2012.01.018
6. Innocenti F, Palmieri V, Stefanone VT, D'Argenzio F, Cigana M, Montuori M, et al. Comparison of troponin I levels versus myocardial dysfunction on prognosis in sepsis. Internal Emergency Med (2022) 17(1):223–31. doi: 10.1007/s11739-021-02701-3
7. Hollenberg SM, Singer M. Pathophysiology of sepsis-induced cardiomyopathy. Nat Rev Cardiol (2021) 18(6):424–34. doi: 10.1038/s41569-020-00492-2
8. Charpentier J, Luyt C-E, Fulla Y, Vinsonneau C, Cariou A, Grabar S, et al. Brain natriuretic peptide: A marker of myocardial dysfunction and prognosis during severe sepsis. Crit Care Med (2004) 32(3):660–5. doi: 10.1097/01.ccm.0000114827.93410.d8
9. Guijas C, Montenegro-Burke JR, Warth B, Spilker ME, Siuzdak G. Metabolomics activity screening for identifying metabolites that modulate phenotype. Nat Biotechnol (2018) 36(4):316–20. doi: 10.1038/nbt.4101
10. Rinschen MM, Ivanisevic J, Giera M, Siuzdak G. Identification of bioactive metabolites using activity metabolomics. Nat Rev Mol Cell Biol (2019) 20(6):353–67. doi: 10.1038/s41580-019-0108-4
11. van der Hooft JJJ, Mohimani H, Bauermeister A, Dorrestein PC, Duncan KR, Medema MH. Linking genomics and metabolomics to chart specialized metabolic diversity. Chem Soc Rev (2020) 49(11):3297–314. doi: 10.1039/d0cs00162g
12. Trongtrakul K, Thonusin C, Pothirat C, Chattipakorn SC, Chattipakorn N. Past experiences for future applications of metabolomics in critically ill patients with sepsis and septic shocks. Metabolites (2021) 12(1):1–32. doi: 10.3390/metabo12010001
13. Ping F, Li Y, Cao Y, Shang J, Zhang Z, Yuan Z, et al. Metabolomics analysis of the development of sepsis and potential biomarkers of sepsis-induced acute kidney injury. Oxid Med Cell Longev (2021) 2021:6628847. doi: 10.1155/2021/6628847
14. Bu Y, Wang H, Ma X, Han C, Jia X, Zhang J, et al. Untargeted metabolomic profiling of the correlation between prognosis differences and PD-1 expression in sepsis: A preliminary study. Front Immunol (2021) 12:594270. doi: 10.3389/fimmu.2021.594270
15. Singer M, Deutschman CS, Seymour CW, Shankar-Hari M, Annane D, Bauer M, et al. The third international consensus definitions for sepsis and septic shock (Sepsis-3). JAMA (2016) 315(8):801–10. doi: 10.1001/jama.2016.0287
16. Heureux M, Sternberg M, Brath L, Turlington J, Kashiouris MG. Sepsis-induced cardiomyopathy: A comprehensive review. Curr Cardiol Rep (2020) 22(5):35. doi: 10.1007/s11886-020-01277-2.
17. Du Y, Fan P, Zou L, Jiang Y, Gu X, Yu J, et al. Serum metabolomics study of papillary thyroid carcinoma based on HPLC-Q-TOF-MS/MS. Front Cell Dev Biol (2021) 9:593510. doi: 10.3389/fcell.2021.593510
18. Cervenka I, Agudelo LZ, Ruas JL. Kynurenines: Tryptophan's metabolites in exercise, inflammation, and mental health. SCIENCE (2017) 357(6349):eaaf9794. doi: 10.1126/science.aaf9794
19. Zhen D, Liu J, Zhang XD, Song Z. Kynurenic acid acts as a signaling molecule regulating energy expenditure and is closely associated with metabolic diseases. Front Endocrinol (2022) 13:847611. doi: 10.3389/fendo.2022.847611
20. Dehhaghi M, Kazemi SPH, Guillemin G. Microorganisms, tryptophan metabolism, and kynurenine pathway: A complex interconnected loop influencing human health status. Int J tryptophan Res IJTR (2019) 12:178646919852996. doi: 10.1177/1178646919852996
21. Krupa A, Kowalska I. The kynurenine pathway-new linkage between innate and adaptive immunity in autoimmune endocrinopathies. Int J Mol Sci (2021) 22(18):9879. doi: 10.3390/ijms22189879
22. Badawy AA-B. Kynurenine pathway of tryptophan metabolism: Regulatory and functional aspects. Int J tryptophan Res IJTR (2017) 10:1178646917691938. doi: 10.1177/1178646917691938
23. Krupa A, Krupa MM, Pawlak K. Kynurenine pathway-an underestimated factor modulating innate immunity in sepsis-induced acute kidney injury? Cells (2022) 11(16):2604. doi: 10.3390/cells11162604
24. Qin X, Liu B, Gao F, Hu Y, Chen Z, Xu J, et al. Gluconolactone alleviates myocardial Ischemia/Reperfusion injury and arrhythmias via activating PKCϵ/Extracellular signal-regulated kinase signaling. Front Physiol (2022) 13:856699. doi: 10.3389/fphys.2022.856699
25. Conte F, van Buuringen N, Voermans NC, Lefeber DJ. Galactose in human metabolism, glycosylation and congenital metabolic diseases: Time for a closer look. Biochim ET Biophys ACTA-GENERAL Subj (2021) 1865(8):129898. doi: 10.1016/j.bbagen.2021.129898
26. Li T, Bai J, Du Y, Tan P, Zheng T, Chen Y, et al. Thiamine pretreatment improves endotoxemia-related liver injury and cholestatic complications by regulating galactose metabolism and inhibiting macrophage activation. Int Immunopharmacol (2022) 108:108892. doi: 10.1016/j.intimp.2022.108892
27. Coelho Ana I, Berry GT, Rubio-Gozalbo ME. Galactose metabolism and health. Curr Opin IN Clin Nutr AND Metab Care (2015) 18(4):422–7. doi: 10.1097/MCO.0000000000000189
28. Bo-Htay C, Palee S, Apaijai N, Chattipakorn SC, Chattipakorn N. Effects of d-galactose-induced ageing on the heart and its potential interventions. J OF Cell AND Mol Med (2018) 22(3):1392–410. doi: 10.1111/jcmm.13472
29. Kanova M, Kohout P. Tryptophan: A unique role in the critically ill. Int J Mol Sci (2021) 22(21):11714. doi: 10.3390/ijms222111714
30. Dagenais-Lussier X, Loucif H, Beji C, Telittchenko R, Routy JP, van Grevenynghe J. Latest developments in tryptophan metabolism: Understanding its role in b cell immunity. Cytokine Growth FACTOR Rev (2021) 59:111–7. doi: 10.1016/j.cytogfr.2021.02.003
31. Vaidyanathan B, Chaudhry A, Yewdell WT, Angeletti D, Yen WF, Wheatley AK, et al. The aryl hydrocarbon receptor controls cell-fate decisions in b cells. J OF Exp Med (2017) 214(1):197–208. doi: 10.1084/jem.20160789
Keywords: sepsis, cardiac dysfunction, metabolomics, diagnosis, prognosis
Citation: Cao Y, Liu Z, Ma W, Fang C, Pei Y, Jing Y, Huang J, Han X and Xiao W (2023) Untargeted metabolomic profiling of sepsis-induced cardiac dysfunction. Front. Endocrinol. 14:1060470. doi: 10.3389/fendo.2023.1060470
Received: 03 October 2022; Accepted: 23 January 2023;
Published: 16 February 2023.
Edited by:
Yao Lu, Third Xiangya Hospital, Central South University, ChinaReviewed by:
Yaping Shao, Dalian Medical University, ChinaWeidong Dai, Tea Research Institute (CAAS), China
Copyright © 2023 Cao, Liu, Ma, Fang, Pei, Jing, Huang, Han and Xiao. This is an open-access article distributed under the terms of the Creative Commons Attribution License (CC BY). The use, distribution or reproduction in other forums is permitted, provided the original author(s) and the copyright owner(s) are credited and that the original publication in this journal is cited, in accordance with accepted academic practice. No use, distribution or reproduction is permitted which does not comply with these terms.
*Correspondence: Xiaotong Han, eGlhb3RvbmdfaGFuQHNpbmEuY29t; Weiwei Xiao, eGlhb3dlaXdlaUBodW5udS5lZHUuY24=
†These authors have contributed equally to this work and share first authorship