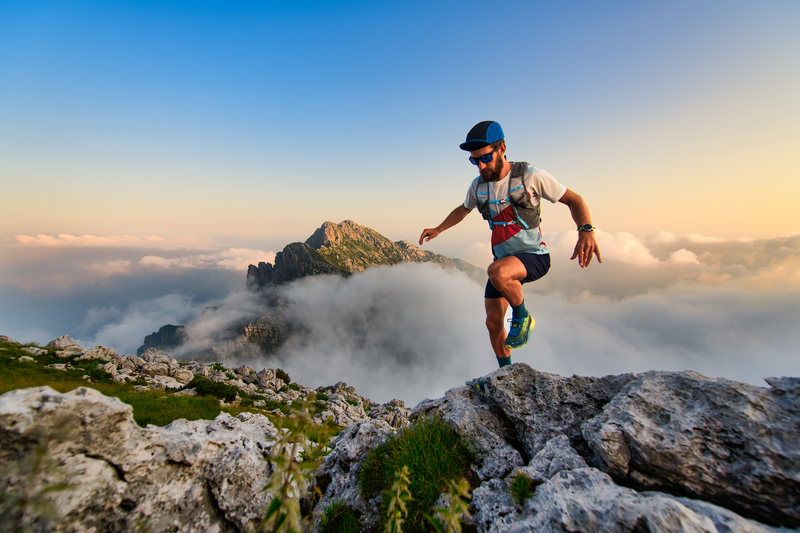
94% of researchers rate our articles as excellent or good
Learn more about the work of our research integrity team to safeguard the quality of each article we publish.
Find out more
ORIGINAL RESEARCH article
Front. Endocrinol. , 31 January 2023
Sec. Reproduction
Volume 14 - 2023 | https://doi.org/10.3389/fendo.2023.1027805
This article is part of the Research Topic Women in Reproductive Endocrinology 2022 View all 9 articles
Background: A number of live birth predictive model during assisted reproductive technology treatment have been available in recent years, but few targeted evaluating the chances of live birth in poor ovarian response(POR) patients. The aim of this study was to develop a nomogram based on POSEIDON criteria to predict live birth in patients with expected POR.
Methods: This retrospective cohort study using clinical data from 657 patients in POSEIDON Groups 3 and 4 (antral follicle count [AFC] ≤5 and AMH <1.2 ng/ml) in the Center for Reproductive Medicine, First Affiliated Hospital of Xinjiang Medical University, and Construction a nomogram model t
Results: Among 657 expected POR patients, 111 (16.89%) had live births, and 546 (83.11%) did not have live births. These were divided into a training set(n=438) and a validation set (n=219). Multivariate logistic regression analysis showed that the age (OR = 0.91, 95% CI: 0.86–0.97), BMI (OR = 1.98, 95% CI: 1.09–3.67), AMH (OR = 3.48, 95% CI: 1.45–8.51), normal fertilized oocytes (OR = 1.40, 95% CI: 1.21–1.63), and the basal FSH (OR = 0.89, 95% CI: 0.80–0.98) of the female were independent factors predicting live birth in patients with expected POR. Then, an individualized nomogram prediction model was built from these five factors. The area under the ROC curve of the live birth prediction model was 0.820 in the training set and 0.879 in the validation set.
Conclusion: We have developed a nomogram combining clinical and laboratory factors to predict the probability of live birth in patients with an expected POR during IVF/ICSI, which can helpful for clinician in decision-making. However, the data comes from the same center, needs a prospective multicenter study for further in-depth evaluation and validation of this prediction model.
The rapid development of assisted reproductive technology (ART) has enabled many infertile patients to have a child by in vitro fertilization-embryo transfer (IVF-ET) and its expanded technologies (1). Since 1978, the world’s first test-tube baby was born, more than 6,000,000 families worldwide have achieved pregnancy through ART, bringing hope for infertile couples to conceive a child (2). In the treatment process, the controlled ovarian hyperstimulation (COH) technique can promote the simultaneous development and maturation of multiple follicles through medication, thus improving the pregnancy rate, which is crucial for IVF-ET success. However, under appropriate ovarian stimulation, the number of retrieved oocytes is still lower than expected in some patients, and this phenomenon is called poor ovarian response (POR) (3, 4). To date, the POR incidence ranges from 9% and 24% in the IVF cycle, leading to a decline of ovarian response in ART treatment, a reduction of retrieved oocytes, an increase of clinical cycle cancelation and miscarriage rate, and the risk of embryonic aneuploidy (5).
Improving the IVF-ET outcome in patients with POR has always been a focus of reproductive medicine research. The Bologna criteria developed by the European Society of Human Reproduction and Embryology (ESHRE) in 2011 are the common diagnostic criteria for POR. However, in the POR definition made by Bologna criteria, the populations with different clinical characteristics and prognosis are placed in the same category, and no further classification is made between the true positive of POR caused by low ovarian reserve and the false positive of POR caused by the deficiency of gonadotrophin (Gn) or its receptor (5).
In 2016, Alviggi et al. proposed the POSEIDON criteria as a new classification. The POSEIDON criteria divide infertile patients into four groups according to age, anti-mullerian hormone (AMH), and antral follicle count (AFC): Group 1: patients aged below 35 years with a good ovarian reserve (AFC ≥ 5, AMH ≥ 1.2 ng/mL) but unexpected POR. Group 1 is further divided into group 1a: the number of retrieved oocytes is < 4; and group 1b: the number of retrieved oocytes is 4–9 (6). Group 2: patients aged 35 years or older with good ovarian reserve (AFC ≥ 5, AMH ≥ 1.2 ng/mL) and unexpected POR. Group 2 is also further divided into group 2a: the number of retrieved oocytes is < 4; and group 2b: the number of retrieved oocytes is 4–9. Group 3: patients aged below 35 years with a diminished ovarian reserve (AFC < 5, AMH < 1.2 ng/mL). Group 4: patients aged 35 years or older with diminished ovarian reserve (AFC < 5, AMH < 1.2 ng/mL). In order to reflect the reproductive potential of these patients in a better way, the POSEIDON criteria classify patients into the “expected POR group (groups 3 and 4)” and the “unexpected POR group (groups 1 and 2)” based on POR heterogeneity (7–9). The POSEIDON criteria aim to guide individualized management and for more detailed stratification of of POR patients to reduce the population heterogeneity of the Bologna criteria
Although ART technology is slowly developing, its success rate remains approximately 40%. Its low pregnancy rate and low live birth rate enlarged both the financial and psychological burdens on infertile families and brought tremendous trouble to the clinicians (10, 11). A number of studies have predicted the pregnancy outcome after IVF/ICSI, and most of the known predictors have already become part of the conventional diagnostic procedure for infertile patients. However, few studies have predicted the pregnancy outcome for the POR patients (12, 13). Therefore, in this study, we aimed to explore the independent predictive factors associated with pregnancy outcome in POR and developed a nomogram model for live birth after IVF/ICSI procedure, in order to implement a personalized reproductive strategy for patients with expected POR.
This study retrospectively analyzed 3,142 patients undergoing IVF/ICSI treatment in the Reproductive Center of the First Affiliated Hospital of Xinjiang Medical University between January 2017 and December 2020 were collected. After excluding the patients with hydrosalpinx, ovarian surgery history, ovarian tumor, endometrial lesion, polycystic ovary syndrome, and the couples with chromosomal abnormalities, a total of 657 patients that conformed to POSEIDON criteria for group 3 and 4 (AFC ≤ 5, AMH < 1.2 ng/mL) were finally included. They were contained only for one cycle with transfer of one or two fresh embryos (the first cycle after fulfilling the criteria).
Our center made ovulation induction protocols according to the patients’ condition and ovarian reserve. The protocols mainly included the early-follicular-phase long-acting GnRH-agonist long protocol, Mid-luteal phase short-acting GnRH-a long protocol, antagonist protocol and natural cycle. We determine the Initial dose of gonadotropin (Merck Schlano, Italy) according to the patient`s age, BMI, AFC and previous ovarian response to stimulation. Vaginal ultrasound is used to monitor the growth of follicles every 1-2 days, and the dosage of gonadotropins is adjusted according to the patient’s peripheral blood luteinizing hormone (LH), estradiol (E2) and progesterone levels to obtain as many mature oocytes as possible. If at least 1–2 dominant follicles with a diameter of ≥ 20 mm were observed, or three follicles with a diameter of ≥ 18 mm had been monitored previously, the blood E2 level would be measured to determine whether to stop the Gn injection, and determine the recombinant human chorionic gonadotropin (hCG) injection dosage. When the leading follicles reached a mean diameter of 14 mm, 0.25mg hCG (Livzon Pharmaceuticals, China) was given to trigger ovulation. Transvaginal ultrasound-guided oocyte retrieval was performed at 34–36 hours after HCG injection. Embryos were transferred on day 3. Progesterone was injected vaginally or intramuscularly daily during the luteal phase until 2 weeks after ET.
The baseline data and clinical characteristics of patients were collected to analyze the POR predictors. The categorical variables included the education background, infertility type, and pregnancy history. The continuous variables included age, body mass index (BMI), age of menarche, average menstrual cycle, and duration of infertility; AFC on the third day of the menstrual cycle, endometrial thickness, LH, E2, progesterone, FSH, and AMH levels; dosing days, initial dose and total dose of Gn, endometrial thickness and hormone levels on the day of HCG injection. BMI greater than 24 kg/m2 can be diagnosed as overweight. AFC was detected using transvaginal ultrasonography on the third day of the menstrual cycle. Endometrial thickness was observed on the day of HCG injection, and endometrial thickness >7 mm is endometrial receptivity markers as prognostic factors for conceiving.
Normal fertilization was confirmed when the presence of two pronuclei (2PN) and the extrusion of the second polar body(2PB) were observed 16–18 h after IVF/ICSI (14, 15). Cleavage embryos were classified as high-quality embryos (grade I and II embryos) if they had seven to nine cells on day 3 and as same-sized blastomeres if with less than 20% blastomeric fragments. Embryos graded III or IV including those that had less than seven cells on day 3, and no less than 20% fragmentation were called poor quality.
The primary outcome of the nomogram was live birth, defined as a pregnancy that continues with at least one live-born fetus after 28 weeks of gestation and survived for more than one month.
All data were performed using R software (version 4.0.2). Patients were divided into a training set and a validation set by the sampling techniques of random numbers.The bilateral p-values < 0.05 were considered statistically significant. The mean± standard deviation (SD) of continuous variables were compared using the t-test, analysis of variance, or Mann–Whitney U test, and the numbers (percentages) of categorical variables were compared using the χ2 test or Fisher’s exact test.The univariate and multivariate logistic regression analyses were performed on the influencing factors. The statistically significant variables were included in the multivariate logistic regression analysis, finally selected into the model via the forward stepwise approach, and visualized as the nomogram scoring system. The nomogram scoring system was evaluated as follows: (1) the discrimination power of the nomogram scoring system was evaluated by the area under the ROC curve; (2) the prediction accuracy was evaluated by the calibration curve; (3) the clinical practicality of the nomogram scoring system was evaluated.
This study was approved by the Ethics Committee of the First Affiliated Hospital of Xinjiang Medical University. In this study, all enrolled patients provided their formal consent. The data of this study were analyzed anonymously and the personal identifiers were completely removed. This study was conducted in compliance with the “Declaration of Helsinki”.
Between January 2017 and December 2020, 657 patients meeting the POSEIDON criteria for the “expected POR group” were included in this study. These patients were allocated into either training set (n = 438) or validation set (n = 219) for model establishment and validation. The baseline characteristics were shown in Table 1, and no significant difference was noted between the two groups (P > 0.05). In the training set, live birth was achieved in 80 patients (15.98%).
The univariate and multivariate logistic regression analyses of live birth in the training set is shown in Table 2. According to the univariate analysis, the live birth in expected POR patients was associated with their age, BMI, AMH, normal fertilized oocytes, basal FSH, and total Gn (P < 0.05). Statistically significant variables were screened out by the univariate analysis and entered into the unconditional binary multivariate logistic regression. Multivariate logistic regression analysis showed that female age (OR = 0.91, 95% CI: 0.86–0.97), BMI (OR = 1.98, 95% CI: 1.09–3.67), AMH (OR = 3.48, 95% CI: 1.45–8.51), normal fertilized oocytes (OR = 1.40, 95% CI: 1.21–1.63), and the basal FSH (OR = 0.89, 95% CI: 0.80–0.98) were significantly associated with live birth in expected POR patients. Collinearity diagnostics to the above independent factors were performed. The variance inflation factors (VIFs) were 1.142, 1.007, 1.193, 1.298, and 1.009, respectively, suggesting no multicollinearity among the five independent factors.
We performed five independent factors in the prediction model. Next, the training set was used to build the individualized nomogram prediction model. According to the nomogram, the corresponding score of each predictor was obtained. The probability of live birth in patients with expected POR can be derived from the sum of these scores, as shown in Figure 1.
The predictive effect of the nomogram scoring system was evaluated using the ROC curve and its area under the curve (AUC). The area under the ROC curve of the live birth prediction model for patients with POR was 0.820 in the training set (Figure 2) and 0.879 in the validation set (Figure 3). This value indicated that the nomogram model has a high-quality predictive value.
Figure 2 ROC curves for validating the discrimination power of the nomogram. (A) Development group. (B) Validation group.
Figure 3 Calibration plots of the nomogram for the probability of the development group and validation group.
Some clinical prediction models have been developed to estimate the chances of IVF success in different populations over the last three decades (16, 17). In a clinical pregnancy failure prediction model based on 281 POR patients, is still conducted according to the Bologna criteria, cannot be distinguished the biological heterogeneity of patients with POR (18, 19). Another study found independent predictors of live birth in POR patients based on the POSEIDON criteria, but failed to develop a complete live birth predictive model (20). Conforti et al. found that, with the increasing age of the first delivery in recent years, the proportion of patients in group 4 under POSEIDON criteria is as high as 55%, and the proportion of patients in group 3 is about 10% (21). Diminished ovarian reserve becomes a common feature of these two cohorts. However, the factors contributing to this “expected” POR remain uncertain and it has been difficult to develop clinical management strategies based on the characteristics and prognoses of POR patients. Therefore, our study has developed a complete nomogram with external validations for expected POR, and the performance is quite good (AUC of 0.820). We hope that this nomogram will provide useful counseling tools regarding pregnancy prospects for POR patients and guide clinicians in their assessment of IVF-ET treatment outcomes.
Consistent with multiple studies, we uncovered that age is a critical factor influencing the ART outcome in POR patients (22). A classic report on the impact of a woman’s age on fertility indicated that, as the age increasing, the female infertility rate also escalates: 6% in women aged 20–24 years, 9% in women aged 25–29 years, 15% in women aged 30–34 years, 30% in women aged 35–39 years, and 64% in women aged 40–44 years (23). The ovarian reserve of female gradually declines with the increasing age, and the quantity and quality of oocytes decrease significantly, which severely negatively impacts the successful pregnancy of patients undergoing IVF/ICSI treatment (24). The large-cohort retrospective study conducted by Hogan et al. found that oocyte donor age is a key factor affecting the oocyte recipient’s cumulative live birth rate, but the oocyte recipient age is irrelative with the cumulative live birth rate. Additionally, the pregnancy rate is significantly higher in recipients of oocytes from oocyte donors aged below 35 years than in recipients of oocytes from oocyte donors aged above 35 years (25). In this study, the nomogram obtained from the univariate and multivariate logistic regression analyses revealed that the clinical pregnancy rate of expected POR patients decreased with age, which is consistent with the observations from previous studies.
Obesity will affect female fertility. A study has shown that the elevated insulin levels in obese patients will stimulate the production of ovarian androgens, which can be converted into more estrogens, formulating a negative feedback on the hypothalamic-pituitary-ovarian axis, thus suppress FSH formation, which may exhibit as the ovulatory dysfunction caused by the growth of non-dominant follicles (26). There are different opinions among the researchers towards the impact of BMI on ovulation induction in IVF. Sarais et al. categorized 1602 fresh embryo transfer recipients according to BMI, and concluded that there is no significant difference among the groups regarding to the total dose of Gn for ovarian stimulation, the duration of Gn effect, the number of oocytes retrieved, and the fertilization (27). While our study indicated that a high total dose of Gn is required for obese individuals, but no difference is observed among various BMI groups in the initial dose of Gn for ovarian stimulation and duration, which is consistent with the meta-analysis by Sermondade et al. (28). Obesity may affect the ovarian response to Gn or drug metabolism, and lower the in vivo effective concentration of drugs. Therefore, reducing the body weight prior to an IVF cycle may improve the outcome of IVF cycle in over-weighted women.
AMH can inhibit the proliferation and growth of follicles by limiting the functions of growth factors and Gn. It reflects primordial follicles’ condition directly and predicts the patient’s ovarian reserve effectively. Also, its stability is not affected by the menstrual cycle (29). Morin et al. demonstrated that more than 0.09 embryos would be obtained for every additional 0.1 ng/mL AMH; patients with AMH value higher than 0.52 ng/mL would obtain at least 0.62 more embryos than those with AMH value less than 0.25 ng/mL (30). Our previous research demonstrated that AMH had a linear and specific quantitative correlation with the number of embryos per stimulation cycle in POR patients. Similarly, AMH could evaluate the number of embryos effectively in patients with expected POR (31). Again, our conclusions that AMH is strongly associated with the pregnancy outcome in patients with expected POR, which is in line with the findings of other studies in the field (32).
Estrogen levels in females would be reduced as the decline of ovarian reserve, resulting in an increase of FSH secretion. The elevated basal FSH (bFSH) level is associated with the declined ovarian reserve (33). Therefore, bFSH has been widely used in reproductive medicine to evaluate the ovarian reserve and its response. Some studies suggested that the serum level of bFSH will increase significantly only when the ovarian reserve is severely declined, and it fluctuates greatly during and within the menstrual cycles, therefore bFSH has no optimal sensitivity or specificity on ovarian response prediction (34). In this study, multiple indicators were applied to evaluate the pregnancy outcome in POR patients, which could effectively improve the prediction accuracy.
The number of retrieved oocytes is quite essential for the outcome of ART treatment. Retrieved oocytes can only be fertilized at metaphase II (MII). Limited retrieved oocytes result in less excellent embryos or even no embryo available for transfer, which eventually ends up with a high IVF cycle cancelation rate and a major reduction in the clinical pregnancy rate. A study of 1706 IVF cycles in a conventional long-term protocol showed that, for the young patients with less than 5 oocytes retrieved, the clinical pregnancy rate is only 14% and the IVF cycle cancelation rate is up to 40% (35). Figueira Rde et al. concluded that, there is no significant difference in fertilization rate, excellent embryo rate, and implantation rate between patients aged below 35 years with four or less MII oocytes and patients with a normal ovarian response; they also observed that the small number of retrieved oocytes largely affected embryo quantity rather than embryo quality in young patients (36). Therefore, our study showed that the number of retrieved oocytes is an effective indicator for pregnancy outcome prediction in patients with expected POR. As more oocytes are retrieved, there will be a higher chance to obtain more fertilized oocytes and excellent embryos.
Validation of the nomogram is crucial to avoid the model overfitting and determine its generalizability. In this study, the calibration curve demonstrated the best concordance between the prediction and actual observation, thus guaranteed the reproducibility and reliability of the developed nomogram. This user-friendly scoring system enables both the clinicians and the patients to make individual prognosis of the pregnancy outcome. Nevertheless, our study still has shortcomings: (1) This is a retrospective study, and selection bias cannot be fully avoided. However, strict inclusion criteria were developed based on the POSEIDON criteria, and sufficient clinical samples were collected so that the study population could truly reflect the situation of the disease occurrence. (2) The data for this study were obtained from the same center. Although patient samples from different periods were used to validate the model, we still need evidence from other centers for validation. Therefore, we would perform a prospective multicenter study for further in-depth evaluation and validation of this prediction model.
The original contributions presented in the study are included in the article/supplementary material. Further inquiries can be directed to the corresponding author.
The studies involving human participants were reviewed and approved by This study was approved by the Ethics Committee of the First Affiliated Hospital of Xinjiang Medical University. Written informed consent for participation was not required for this study in accordance with the national legislation and the institutional requirements.
GX and YNZ: Conceived the study and manuscript writing. YJZ and PW: Data acquisition. ZW: Editing of this manuscript. CL: Clinical consultation. MZ: Data preprocessing. XL: Resources, study design, supervision, and finally manuscript approval. All authors contributed to the article and approved the submitted version.
This work was supported by the National Natural Science Foundation of China (81960289), State Key Laboratory of Pathogenesis, Prevention and Treatment of High Incidence Diseases in Central Asia (SKL-HIDCA-2020-JZ4).
The authors thank the women who participated in this study and all the physicians and nurses at the Center for Reproductive Medicine, First Affiliated Hospital of Xinjiang Medical University.
The authors declare that the research was conducted in the absence of any commercial or financial relationships that could be construed as a potential conflict of interest.
All claims expressed in this article are solely those of the authors and do not necessarily represent those of their affiliated organizations, or those of the publisher, the editors and the reviewers. Any product that may be evaluated in this article, or claim that may be made by its manufacturer, is not guaranteed or endorsed by the publisher.
1. Polyzos NP, Drakopoulos P, Parra J, Pellicer A, Santos-Ribeiro S, Tournaye H, et al. Cumulative live birth rates according to the number of oocytes retrieved after the first ovarian stimulation for in vitro fertilization/intracytoplasmic sperm injection: a multicenter multinational analysis including approximately 15,000 women. Fertil Steril (2018) 110(4):661–670e661. doi: 10.1016/j.fertnstert.2018.04.039
2. Zhang Y, Zhang C, Shu J, Guo J, Chang HM, Leung PCK, et al. Adjuvant treatment strategies in ovarian stimulation for poor responders undergoing IVF: a systematic review and network meta-analysis. Hum Reprod Update (2020) 26(2):247–63. doi: 10.1093/humupd/dmz046
3. Drakopoulos P, Bardhi E, Boudry L, Vaiarelli A, Makrigiannakis A, Esteves SC, et al. Update on the management of poor ovarian response in IVF: the shift from Bologna criteria to the poseidon concept. Ther Adv Reprod Health (2020) 14:2633494120941480. doi: 10.1177/2633494120941480
4. Abu-Musa A, Haahr T, Humaidan P. Novel physiology and definition of poor ovarian response; clinical recommendations. Int J Mol Sci (2020) 21(6):2110. doi: 10.3390/ijms21062110
5. Polyzos NP, Devroey P. A systematic review of randomized trials for the treatment of poor ovarian responders: is there any light at the end of the tunnel? Fertil Steril (2011) 96(5):1058–1061.e1057. doi: 10.1016/j.fertnstert.2011.09.048
6. Ferraretti AP, La Marca A, Fauser BC, Tarlatzis B, Nargund G, Gianaroli L. ESHRE consensus on the definition of 'poor response' to ovarian stimulation for in vitro fertilization: the Bologna criteria. Hum Reprod (2011) 26(7):1616–24. doi: 10.1093/humrep/der092
7. Drakopoulos P, Blockeel C, Stoop D, Camus M, de Vos M, Tournaye H, et al. Conventional ovarian stimulation and single embryo transfer for IVF/ICSI. how many oocytes do we need to maximize cumulative live birth rates after utilization of all fresh and frozen embryos? Hum Reprod (2016) 31(2):370–6. doi: 10.1093/humrep/dev316
8. Grynberg M, Labrosse J. Understanding follicular output rate (FORT) and its implications for POSEIDON criteria. Front Endocrinol (Lausanne) 10 (2019) 246:246. doi: 10.3389/fendo.2019.00246
9. Poseidon G, Alviggi C, Andersen CY, Buehler K, Conforti A, De Placido G, et al. A new more detailed stratification of low responders to ovarian stimulation: from a poor ovarian response to a low prognosis concept. Fertil Steril (2016) 105(6):1452–3. doi: 10.1016/j.fertnstert.2016.02.005
10. Leijdekkers JA, Eijkemans MJC, van Tilborg TC, Oudshoorn SC, McLernon DJ, Bhattacharya S, et al. Predicting the cumulative chance of live birth over multiple complete cycles of in vitro fertilization: an external validation study. Hum Reprod (2018) 33(9):1684–95. doi: 10.1093/humrep/dey263
11. Zhang M, Tian H-Q, Bu T, Li X, Wan X-H, Wang D-L, et al. Development and validation of a nomogram for predicting the probability of live birth in infertile women. Reprod Dev Med (2019) 02):77–83. doi: 10.4103/2096-2924.262388
12. Hunault CC, te Velde ER, Weima SM, Macklon NS, Eijkemans MJ, Klinkert ER, et al. A case study of the applicability of a prediction model for the selection of patients undergoing in vitro fertilization for single embryo transfer in another center. Fertil Steril (2007) 87(6):1314–21. doi: 10.1016/j.fertnstert.2006.11.052
13. Nelson SM, Lawlor DA. Predicting live birth, preterm delivery, and low birth weight in infants born from in vitro fertilisation: a prospective study of 144,018 treatment cycles. PLoS Med (2011) 8(1):e1000386. doi: 10.1371/journal.pmed.1000386
14. Dong L, Lian F, Wu H, Xiang S, Li Y, Wei C, et al. Reproductive outcomes of dual trigger with combination GnRH agonist and hCG versus trigger with hCG alone in women undergoing IVF/ICSI cycles: a retrospective cohort study with propensity score matching. [J] .BMC Pregnancy Childbirth (2022) 22(1):583. doi: 10.1186/s12884-022-04899-2
15. Sifer C, Sasportes T, Barraud V, Poncelet C, Rudant J, Porcher R, et al. World health organization grade 'a' motility and zona-binding test accurately predict IVF outcome for mild male factor and unexplained infertilities. Hum Reprod (2005) 20(10):2769–75. doi: 10.1093/humrep/dei118
16. ElMokhallalati Y, van Eekelen R, Bhattacharya S, McLernon DJ. Treatment-independent live birth after in-vitro fertilisation: A retrospective cohort study of 2,133 women. [J] .Hum Reprod (2019) 34(8):1470–8. doi: 10.1093/humrep/dez099
17. Gao H, Liu DE, Li Y, Wu X, Tan H. Early prediction of live birth for assisted reproductive technology patients: a convenient and practical prediction model. Sci Rep (2021) 11(1):331. doi:10.1038/s41598-020-79308-9
18. Li F, Lu R, Zeng C, Li X, Xue Q. Development and validation of a clinical pregnancy failure prediction model for poor ovarian responders during IVF/ICSI. Front Endocrinol (Lausanne) (2021) 12:717288. doi:10.3389/fendo.2021.717288
19. Boza A, Oguz SY, Misirlioglu S, Yakin K, Urman B. Utilization of the Bologna criteria: a promise unfulfilled? a review of published and unpublished/ongoing trials. Fertil Steril (2018) 109(1):104–109.e102. doi: 10.1016/j.fertnstert.2017.09.024
20. Li F, Ye T, Kong H, Li J, Hu L, Jin H, et al. In Vitro Predictive factors for live birth in fresh In vitro Fertilization/Intracytoplasmic sperm injection treatment in poor ovarian reserve patients classified by the POSEIDON criteria. Front Endocrinol (Lausanne) (2021) 12:630832. doi: 10.3389/fendo.2021.630832
21. Conforti A, Esteves SC, Picarelli S, Iorio G, Rania E, Zullo F, et al. Novel approaches for diagnosis and management of low prognosis patients in assisted reproductive technology: the POSEIDON concept. Panminerva Med (2019) 61(1):24–9. doi: 10.23736/S0031-0808.18.03511-5
22. Wang YA, Healy D, Black D, Sullivan EA. Age-specific success rate for women undertaking their first assisted reproduction technology treatment using their own oocytes in Australia 2002-2005. Hum Reprod (2008) 23(7):1633–8. doi: 10.1093/humrep/den135
23. Menken J, Trussell J, Larsen U. Age and infertility. Science (1986) 233(4771):1389–94. doi: 10.1126/science.3755843
24. Candiani M, Ottolina J, Posadzka E, Ferrari S, Castellano LM, Tandoi I, et al. Assessment of ovarian reserve after cystectomy versus 'one-step' laser vaporization in the treatment of ovarian endometrioma: a small randomized clinical trial. Hum Reprod (2018) 33(12):2205–11. doi: 10.1093/humrep/dey305
25. Hogan RG, Wang AY, Li ZY, Hammarberg K, Johnson L, Mol BW, et al. Oocyte donor age has a significant impact on oocyte recipients' cumulative live-birth rate: A population-based cohort study. Fertility Sterility (2019) 112(4):724–30. doi: 10.1016/j.fertnstert.2019.05.012
26. Broughton DE, Moley KH. Obesity and female infertility: potential mediators of obesity's impact. Fertility Sterility (2017) 107(4):840–7. doi: 10.1016/j.fertnstert.2017.01.017
27. Sarais V, Pagliardini L, Rebonato G, Papaleo E, Candiani M, Viganio P. A comprehensive analysis of body mass index effect on in vitro fertilization outcomes. Nutrients (2016) 8(3):109. doi: 10.3390/nu8030109
28. Sermondade N, Huberlant S, Bourhis-Lefebvre V, Arbo E, Gallot V, Colombani M, et al. Female obesity is negatively associated with live birth rate following IVF: A systematic review and meta-analysis. Hum Reprod Update (2019) 25(4):439–51. doi: 10.1093/humupd/dmz011
29. Broer SL, Broekmans FJM, Laven JSE, Fauser BCJM. Anti-mullerian hormone: ovarian reserve testing and its potential clinical implications. Hum Reprod Update (2014) 20(5):688–701. doi: 10.1093/humupd/dmu020
30. Morin SJ, Patounakis G, Juneau CR, Neal SA, Scott RT, Seli E. Diminished ovarian reserve and poor response to stimulation in patients <38 years old: a quantitative but not qualitative reduction in performance. Hum Reprod (2018) 33(8):1489–98. doi: 10.1093/humrep/dey238
31. Tian HQ, Mao XM, Su N, La XL. The correlation between AMH and number of embryos in POSEIDON groups: a retrospective cohort study. Reprod Biomed Online (2021) 42(4):842–8. doi: 10.1016/j.rbmo.2020.12.010
32. Meireles AJC, Bilibio JP, Lorenzzoni PL, Conto E, Nascimento FCD, Cunha-Filho JSD. Association of FSHR, LH, LHR, BMP15, GDF9, AMH, and AMHR polymorphisms with poor ovarian response in patients undergoing in vitro fertilization. JBRA Assist Reprod (2021) 25(3):439–46. doi: 10.5935/1518-0557.20210004
33. Hu LL, Sun B, Ma YJ, Li L, Wang F, Shi H, et al. The relationship between serum delta FSH level and ovarian response in IVF/ICSI cycles. Front Endocrinol (2020) 11:536100. doi: 10.3389/fendo.2020.536100
34. Bancsi LF, Broekmans FJ, Mol BW, Habbema JD, te Velde ER. Performance of basal follicle-stimulating hormone in the prediction of poor ovarian response and failure to become pregnant after in vitro fertilization: a meta-analysis. Fertil Steril (2003) 79(5):1091–100. doi: 10.1016/s0015-0282(03)00078-5
35. Saldeen P, Kallen K, Sundstrom P. The probability of successful IVF outcome after poor ovarian response. Acta Obstet Gynecol Scand (2007) 86(4):457–61. doi: 10.1080/00016340701194948
Keywords: poor ovarian response, POSEIDON criteria, IVF/ICSI transfer, nomogram model, live birth
Citation: Gong X, Zhang Y, Zhu Y, Wang P, Wang Z, Liu C, Zhang M and La X (2023) Development and validation of a live birth prediction model for expected poor ovarian response patients during IVF/ICSI. Front. Endocrinol. 14:1027805. doi: 10.3389/fendo.2023.1027805
Received: 25 August 2022; Accepted: 09 January 2023;
Published: 31 January 2023.
Edited by:
Gian Mario Tiboni, University G. D’Annunzio, ItalyReviewed by:
Rui Yang, Peking University Third Hospital, ChinaCopyright © 2023 Gong, Zhang, Zhu, Wang, Wang, Liu, Zhang and La. This is an open-access article distributed under the terms of the Creative Commons Attribution License (CC BY). The use, distribution or reproduction in other forums is permitted, provided the original author(s) and the copyright owner(s) are credited and that the original publication in this journal is cited, in accordance with accepted academic practice. No use, distribution or reproduction is permitted which does not comply with these terms.
*Correspondence: Xiaolin La, OTA5MjMyOTA1QHFxLmNvbQ==
†These authors have contributed equally to this work
Disclaimer: All claims expressed in this article are solely those of the authors and do not necessarily represent those of their affiliated organizations, or those of the publisher, the editors and the reviewers. Any product that may be evaluated in this article or claim that may be made by its manufacturer is not guaranteed or endorsed by the publisher.
Research integrity at Frontiers
Learn more about the work of our research integrity team to safeguard the quality of each article we publish.