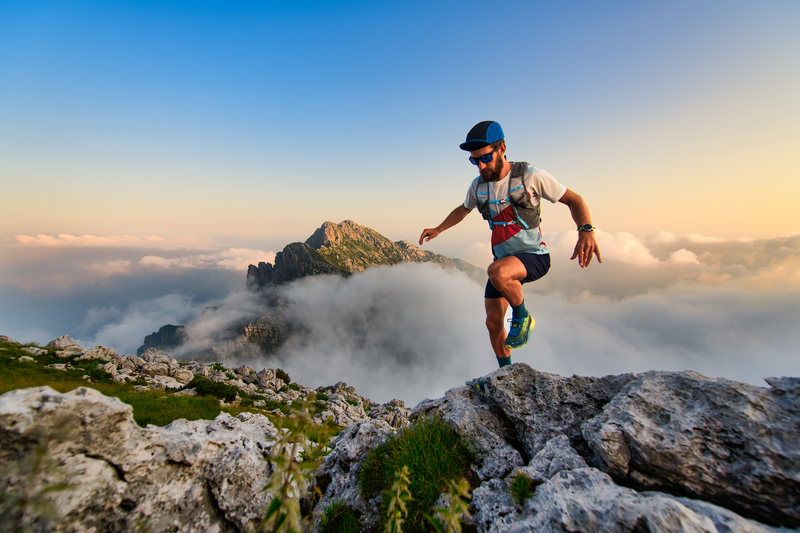
94% of researchers rate our articles as excellent or good
Learn more about the work of our research integrity team to safeguard the quality of each article we publish.
Find out more
ORIGINAL RESEARCH article
Front. Endocrinol. , 28 September 2022
Sec. Cancer Endocrinology
Volume 13 - 2022 | https://doi.org/10.3389/fendo.2022.995972
Glucose-6-phosphate isomerase (GPI) plays an important part in gluconeogenesis and glycolysis through the interconversion of D-glucose-6-phosphate and D-fructose-6-phosphate, and its clinical significance still remains unclear in breast cancer (BRCA). We analyzed the expressions of GPI in BRCA patients to determine prognostic values. Our results showed that the expression levels of GPI were upregulated in BRCA patients, and a high GPI expression is correlated with poor overall survival (OS) in BRCA. At the same time, a high GPI expression is correlated with poor clinicopathological characteristics, such as stage III, over 60 years old, N3, HER2 negative, and estrogen receptor (ER) positive. Further analysis of the influence of GPI on the prognosis of BRCA suggested that 50 genes and 10 proteins were positively correlated with GPI, and these genes and proteins were mainly involved in cell cycle signaling pathways. In addition, in this study, we observed that GPI was closely related to N6-methyladenosine (m6A) RNA methylation modification and immune cell infiltration and ferroptosis-related gene expression in BRCA, and there was a difference in m6A RNA methylation alterations, immune cell infiltration, and ferroptosis-related gene expression between the high GPI expression group and the low GPI expression group. Finally, we found that GPI in BRCA had 2.6% gene alterations, and BRCA patients with gene alteration of GPI had a poor prognosis in disease-free survival (DFS). Altogether, our work strongly suggested that GPI may serve as a new prognostic biomarker for BRCA patients.
1. The expression of GPI affects the survival rate of breast cancer patients
2. GPI is closely related to cell cycle regulatory genes
3. GPI expression is correlated to immune cell infiltration
4. GPI expression is associated with m6A RNA methylation regulation and gene alteration
5. GPI expression is associated with ferroptosis genes in BRCA
Female breast cancer has now been the most commonly occurring cancer in the world in 2020, with approximately 2.3 million new cases, accounting for 11.7% of overall cancer cases. Among women, breast cancer is the leading cause of cancer death, with 685,000 deaths (1). Recently, surgery, radiotherapy, chemotherapy, immunotherapy, and targeted drug therapy have made a great amount of progress in treating breast cancer (2, 3). However, there is still a poor survival rate for some metastatic breast cancer patients, and only one-fifth of these patients survive over 5 years (4). Due to tumor heterogeneity, the current prognostic biomarkers for breast cancer have some drawbacks (5). Therefore, it is imperative to find more effective prognostic biomarkers for optimizing the treatments of breast cancer.
Carcinogenesis, progression, and metastasis are involved in epigenetic and genetic alterations, including gene mutations, variation of metabolic enzymes (3), alteration of the tumor microenvironment (5), and DNA methylation change (6). As one of the glucose-metabolizing enzymes, GPI plays an important part in gluconeogenesis and glycolysis through the interconversion of D-glucose-6-phosphate and D-fructose-6-phosphate (7, 8). Furthermore, GPI can be released to the outside of cells functioning as a cytokine or growth factor (9). In patients, the levels of GPI are significantly higher in both serum and synovial fluid (9). In recent years, it has been reported that there was an aberrant expression of GPI in several cancers (10–12). A high expression of GPI in lung adenocarcinoma and renal cell carcinoma was related to poor prognosis (10, 11). However, the clinical significance of GPI in human breast cancer remains unclear. Bioinformatics analysis has been applied to survey the role of GPI in cancers (10).
In the present study, according to the analysis of GPI gene expressions in published databases, we evaluated the relationship between GPI expression and the prognosis of breast cancer patients and conducted an analysis of the influence of GPI on the prognosis. Our results indicated that GPI can be used as a biomarker to predict the prognosis of breast cancer patients.
The study has been authorized by the Ethics Committee of Hunan Provincial People’s Hospital/The First Affiliated Hospital of Hunan Normal University (document no. 2022-49) and practiced in accordance with the research principles described in the Declaration of Helsinki.
TIMER2 (Tumor Immune Estimation Resource, version 2, http://timer.cistrome.org/) (13) was used to analyze the expression profiling of GPI between tumor types and adjacent normal tissues, and the relationship between GPI expression and immune cell infiltration was evaluated.
The RNA-Seq expression data of GPI in breast cancer (BRCA) come from The Cancer Genome Atlas (TCGA) (https://portal.gdc.cancer.gov/). Therefore, many tumor data and adjacent normal tissue data were retained. For some tumors without normal or with highly limited normal tissues, TCGA normal and Genotype-Tissue Expression (GTEx) data were matched. The samples selected included GPI gene expression data and associated clinical information, such as age, pathological stage, race, and histological type.
A total of 20 samples of paraffin-embedded breast cancer tissues and their matched paracancerous tissues were gained from breast cancer patients who were treated at Hunan Provincial People’s Hospital/The First Affiliated Hospital of Hunan Normal University. Tumor tissue and its adjacent normal tissues were made into 4-mm paraffin sections, and these sections were treated with primary rabbit monoclonal antibodies of GPI (1:500 dilution; Cell Signaling Technology, Danvers, MA, USA) at 4°C overnight. After being washed with phosphate-buffered saline (PBS), each section was treated with horseradish peroxidase (HRP)-labeled goat anti-rabbit as a secondary antibody (1:1500 dilution; Beyotime, Shanghai, China) for 2 h at 37°C. The sections were stained with 3,3′-diaminobenzidine (DAB) and counterstained with hematoxylin (Beyotime, Shanghai, China). A semiquantitative integration method was used to analyze staining intensity using ImageJ software.
An R package was used to estimate the correlation between GPI expression and the survival rate of different clinical features in BRCA patients, and the hazard ratio (HR) and log-rank p-value of the 95% confidence interval were calculated.
To investigate the biological processes that GPI may be involved in, using the Gene Expression Profiling Interactive Analysis (GEPIA) database (http://gepia.cancer-pku.cn/index.html) (14), an analysis of the 25 genes with positive and negative correlations of GPI was carried out. The genes were enriched by Gene Ontology (GO) containing molecular function (MF), biological processes (BP), and cellular constituents (CC). Kyoto Encyclopedia of Genes and Genomes (KEGG) pathway analyses were visualized by the R package “ggplot2”. A corrected p < 0.05 was considered to be statistically significant. In addition, the “clusterProfiler” R package was applied to conduct GO enrichment analysis. The data for BP, CC, MF, and KEGG were visualized in Network.
STRINGS (www.string-db.org) (15) is a network tool for the analysis of protein–protein interaction (PPI). In this study, we performed a PPI analysis of GPI to explore their functions in human breast cancer. The basic settings were as follows: meaning of network edges: “evidence”, active interaction sources: “experiments and textmining”, the minimum required interaction score: “medium confidence (0.400)”, and max number of interactors to show: “no more than 10 interactors” in 1st shell.
By the cBioPortal tool (https://www.cbioportal.org/) (16, 17), the data of gene amplification, missense mutation, truncating mutation, and deep deletion in BRCA of all TCGA tumors were collected. The mutated site information of GPI can be visualized in the schematic diagram of the protein structure or the three-dimensional (3D) structure by the “Mutations” module. The data on the overall survival (OS), progression-free survival (PFS), disease-free survival (DFS), and disease-specific survival (DSS) for BRCA cases with or without GPI genetic alteration were obtained.
We chose clinicopathological prognostic indexes and established a series of the table to evaluate the 1-, 3-, and 5-year OS probability of BRCA patients. In comparison with the observed actual probability via a calibration curve, the accuracy of the predicted probability of OS in the line chart was tested.
Analyses were performed by using tools in Hiplot (https://hiplot.org) (18), a comprehensive and easy-to-use web service for boosting publication-ready biomedical data visualization. Gene expression data and clinical information were visualized by the R package “ggplot2” R package. The Wilcoxon signed-rank test and logistic regression were used to analyze the relationship between the clinical features of BRCA and the expression of GPI. Log-rank test was used to test the p-value. Spearman’s correlation analysis was used to describe the correlation between quantitative variables without normal distribution. The statistical method used was single-sample gene set enrichment analysis (ssGSEA) by R package “GSVA” of tumor-infiltrating immune cells from the gene expression profiles of BRCA samples in TCGA datasets. Ferroptosis-related genes are derived from the Liu et al. (19) systematic analysis of the aberrances and functional implications of ferroptosis in cancer. The m6A-related genes were derived from Xu’s (20) research on the molecular characterization and clinical significance of m6A modulators across 33 cancer types. The analysis methods were visualized by “ggplot2” and “pheatmap”. In all analyses, *, **, and *** indicate p < 0.05, p < 0.01, and p < 0.001, respectively.
We applied the TIMER2 approach to analyze the expression status of GPI across various cancer types of TCGA. As shown in Figure 1A, in the tumor tissues, such as BRCA (breast invasive carcinoma), CHOL (cholangiocarcinoma), COAD (colon adenocarcinoma), ESC (esophageal carcinoma), HNSC (head and neck squamous cell carcinoma), KICH (kidney chromophobe), KIRC (kidney renal clear cell carcinoma), HCC (hepatocellular carcinoma), LUAD (lung adenocarcinoma), LUSC (lung squamous cell carcinoma), PRAD (prostate adenocarcinoma), READ (rectum adenocarcinoma), STAD (stomach adenocarcinoma), UCEC (uterine corpus endometrial carcinoma), and BLCA (bladder urothelial carcinoma) (p < 0.01), the expression level of GPI was significantly higher than in adjacent normal tissues (p < 0.01). Using the normal tissue of the GTEx dataset as a control, we further evaluated the expression difference of GPI between the normal tissues and tumor tissues (Figure 1B); compared with that in the corresponding normal tissues, the GPI expression level was significantly higher in tumor tissues such as ACC (adrenocortical carcinoma), DLBC (diffuse large B-cell lymphoma), GBM (glioblastoma multiforme), PAAD (pancreatic adenocarcinoma), UCS (uterine carcinosarcoma), THYM (thymoma), OV (ovarian serous cystadenocarcinoma), and TGCT (testicular germ cell tumors) (p < 0.05). However, the expression level of GPI was significantly higher in normal tissues than in tumor tissues such as LAML (acute myeloid leukemia) and LGG (brain lower-grade glioma) (p < 0.001).
Figure 1 GPI expression levels, clinical characteristics, and immunohistochemistry in human cancer. (A) The expression status of the GPI gene in different cancers was analyzed through TIMER2. (B) For the type of ACC, DLBC, GBM, LAML, LGG, PAAD, UCS, TGCT, THYM, and OV, in the TCGA project, the corresponding normal tissues of the GTEx database were included as controls. (C, D) The expression of GPI in BRCA (IHC). (E) Expression level of GPI in tumor tissues from patients with different clinical characteristics in TCGA database [Age, ER status, PR status, T stage, and PAM50]. *p < 0.05; **p < 0.01; ***p < 0.001. TIMER2, Tumor Immune Estimation Resource, version 2; TCGA, The Cancer Genome Atlas; GTEx, Genotype-Tissue Expression; IHC, immunohistochemistry; ER, estrogen receptor; PR, progesterone receptor.
We investigated the protein expression of GPI in BRCA and its paired adjacent tissues by immunohistochemistry (IHC). The results showed that the protein levels of GPI are higher in BRCA tissues than in the adjacent tissues (Figures 1C, D).
The association identified between GPI expression and clinical features in patients with BRCA is summarized in Table 1. As shown in Figure 1E, we used the Bonferroni method to correct the multiple hypothesis tests (Dunn’s test) of the significance level. Meanwhile, GPI mRNA expression was significantly lower in BRCA patients (age > 60) than in BRCA patients (age ≤ 60) (p.adj < 0.01). Compared with that in BRCA patients who are estrogen receptor (ER) or progesterone receptor (PR) negative, GPI mRNA expression was significantly lower in BRCA patients who are ER or PR positive (p.adj < 0.001). In addition, GPI mRNA expression was significantly lower in BRCA patients with T1 than in BRCA patients with T2/T4 (p.adj < 0.01) and was significantly lower in T3 than in T4 (p.adj < 0.01).
To identify whether GPI expression affects patient survival, we divided BRCA patients in the TCGA database into high and low GPI expression groups in order to perform survival analysis. The Kaplan–Meier survival analysis showed that the high expression of GPI was related to the poor prognosis of OS (HR = 1.44, p = 0.022) and progression-free interval (PFI) (HR = 1.55, p = 0.012) in BRCA patients (Figures 2A, B). In BRCA, subgroup analysis showed that a high GPI expression was significantly correlated with poor prognosis in the following cases: pathological stage III (HR = 1.84, p = 0.044), patients over 60 years old (HR = 2.32, p < 0.001), N2 (HR = 2.89, p = 0.017), N3 (HR = 4.68, p = 0.001), M0 (HR = 1.43, p = 0.048), race: White (HR = 1.62, p = 0.009), histological type: ILC (HR = 3.00, p = 0.006), histological type: IDC (HR = 1.56, p = 0.02), HER2 status: negative (HR = 1.67, p = 0.046), ER status: positive (HR = 1.62, p = 0.024), and menopause status: post (HR = 2.17, p < 0.001). The opposite are M1 (HR = 4.22, p = 0.005) and menopause status: pre (HR = 0.28, p < 0.003) (Figures 2C–P).
Figure 2 Kaplan–Meier survival curve analysis of the prognostic significance of high and low expression of GPI in BRCA using TCGA database. (A, B) Kaplan–Meier estimates of the overall survival (OS) and progression-free interval (PFI) probability of TCGA patients in all BRCA patients. Pathological staging stage III (C), age greater than 60 years (D), N2 (E), N3 (F), M0 (G), M1 (H), race: White (I), histological type: ILC (J), histological type: IDC (K), HER2 status: negative (L), ER status: positive (M), PR status: positive (N), menopause status: post (O), and menopause status: pre (P).
To understand the biological function of GPI in BRCA, we used the R package to detect the co-expression pattern of GPI in BRCA of the TCGA database. The red dot indicates the top 25 genes that were positively correlated with GPI, and the blue dot represents the bottom 25 genes that were negatively correlated with GPI (Figure 3A). We used the “ClusterProfiler” R package to perform functional annotation and pathway enrichment analysis of GPI from 600 nodes representing genes, and we found that cell cycle signaling pathways, nuclear division, and mitotic nuclear division were enriched among these genes (Figures 3B, C). At the same time, we made a more intuitive GO and KEGG network map to show the connection between pathways (Figure 3D). We performed a PPI network analysis of GPI at different transcription levels by STRING to study the potential interactions between them; the results showed that the PPI network diagram contained GPI proteins and 10 proteins that were closely related to GPI proteins (Figure 3E).
Figure 3 GPI functional and interaction network. (A) Heatmap showing the top 50 genes in BRCA that were positively and negatively related to GPI. Red represents positively related genes, and blue represents negatively related genes. (B, C) Gene Ontology (GO) term and Kyoto Encyclopedia of Genes and Genomes (KEGG) pathway analyses of GPI-related genes in BRCA. (D) Network of GO and KEGG enriched terms. (E) Protein–protein interaction (PPI) network of different expressed GPI. ***p < 0.001.
The above bioinformatics analysis indicated that GPI was significantly enriched in the cell cycle pathway. We further analyzed the correlation between GPI and the cell cycle regulatory genes in TCGA-BRCA, and the results showed that the expressions of the cell cycle regulatory genes, such as CCNA2, CCNB1, CCNB2, CCNE1, CHEK1, BUB1B, ESPL1, PTTG1, PCNA, PKMYT1, CDC45, PLK1, MCM2, MCM4, MCM6, E2F1, CDC6, CDC20, CDC25A, and CDC25C, were positively correlated with GPI (r > 0.3, p < 0.001) (Figures 4A–T).
Figure 4 Correlation analysis between GPI and the cell cycle regulatory genes in BRCA. (A) MCM2, (B) ESPL1, (C) E2F1, (D) CHEK1, (E) MCM6, (F) MCM4, (G) CDC6, (H) CDC45, (I) PCNA, (J) PKMYT1, (K) CCNE1, (L) CDC25C, (M) PTTG1, (N) CCNA2, (O) CCNB2, (P) CDC25A, (Q) PLK1, (R) BUB1B, (S) CCNB1, and (T) CDC20.
To explore the correlation between the expression level of GPI and tumor immune response, we investigated immune infiltration in BRCA with different GPI expression levels. The results showed that the infiltration levels of immune cells, such as CD8+ T cells, Eosinophils, iDC [immature DC], Mast cells, NK CD56bright cells, NK cells, pDC [Plasmacytoid DC], T helper cells, Tcm [T central memory], Tem [T effector memory], and Th17 cells, in BRCA patients with high GPI expression were significantly lower than those in patients with low GPI expression. In contrast, the infiltration levels of immune cells, including aDC [activated DC], Macrophages, NK CD56dim cells, Th1 cells, Th2 cells, and Treg, in BRCA patients with high GPI expression were significantly higher than those in patients with low GPI expression. In addition, the data showed that there was no significant difference in the infiltration levels of B cells, Cytotoxic cells, DC, Neutrophils, T cells, Tfh [T follicular helper], and Tgd [T gamma delta] between patients with high and low GPI expression (Figures 5A–N). Based on the results after adjusting for tumor purity, we observed that the level of GPI expression was significantly correlated with multiple immune markers, including CD20, CD70, CD25, CD278, CD191, CD195, CD360, CD196, FOXP3, CD73, PD-1, CTLA4, LAG3, CD68, NOS2, CD163, CD206, CD86, CD14, CD57, KIR3DL1, CD7, CD16, CD1C, and CD141 (p < 0.05, Table 2).
Figure 5 Correlation analysis of GPI expression and immune infiltration in BRCA. (A, B) Differential distribution of immune cells in patients with high GPI expression and low GPI expression. (C–N) Correlation between the expression level of GPI and immune infiltration in BRCA: (C) Mast cells, (D) pDC, (E) NK CD56bright cells, (F) CD8 T cells, (G) Eosinophils, (H) NK cells, (I) iDC, (J) Th2 cells, (K) TReg, (L) Macrophages, (M) CD56dim cells, and (N) aDC. *p < 0.05; **p < 0.01; ***p < 0.001. ns, no significance.
To investigate whether GPI expression is related to m6A modification, we analyzed the relation between the expression of GPI and 20 m6A-related genes in BRCA of TCGA data (Figure 6A). The results showed that GPI expression was significantly positively correlated with 11 m6A-related genes in BRCA, including WTAP (r = 0.073, p = 0.016), RBM15 (r = 0.185, p < 0.001), RBM15B (r = 0.07, p = 0.019), YTHDF1 (r = 0.192, p < 0.001), YTHDF2 (r = 0.207, p < 0.001), HNRNPC (r = 0.118, p < 0.001), IGF2BP1 (r = 0.227, p < 0.001), IGF2BP2 (r = 0.173, p < 0.001), IGF2BP3 (r = 0.232, p < 0.001), HNRNPA2B1 (r = 0.134, p < 0.001), and ALKBH5 (r = 0.067, p = 0.027) (Figures 6B, C). In addition, were divided 1,083 tumor samples into two groups, based on GPI expression, including 542 samples in the high-expression group and 541 samples in the low-expression group. We analyzed the expression of 20 m6A-related genes between different GPI expression level groups in BRCA. The results showed that, compared with that in the low GPI expression group, the expression of RBN15, YTHDF1, YTHDF2, HNRNPC, IGF2BP1, IGF2BP2, IGF2BP3, and HNRNPA2B1 increased in the high GPI expression group (p < 0.05). In contrast, compared with that in the high GPI expression group, the expression of METTL3, METTL14, RBM15B, ZC3H13, YTHDC1, YTHDC2, and FTO increased in the low GPI expression group of GPI (p < 0.05) (Figures 6D, E). The above results indicated that GPI was closely related to m6A modification in BRCA.
Figure 6 Correlation analysis of GPI expression, m6A, and ferroptosis-related genes in BRCA. (A) Heatmap showing correlation between GPI and m6A-related genes, (B) YTHDF1, (C) YTHDF2, and (D, E) differential m6A-related genes in patients with high GPI expression and low GPI expression. (F) Heatmap showing correlation between GPI and ferroptosis-related genes, (G) CS, (H) ATP5MC3, (I) HSPA5, (J–L) differential ferroptosis-related genes in patients with high GPI expression and low GPI expression. *p < 0.05; **p < 0.01; ***p < 0.001.
A prominent role for ferroptosis in cancer development and treatment is emerging. We then explored whether there was an association between ferroptosis genes and GPI expression in BRCA. We analyzed the TCGA BRCA dataset to study the correlation between the expression of GPI and 25 ferroptosis-related genes in BRCA (Figure 6F). The results showed that GPI expression was significantly positively correlated with 15 ferroptosis-related genes in BRCA, including HSPA5 (0.293, p < 0.001), EMC2 (0.121, p < 0.001), SLC7A11 (0.172, p < 0.001), MT1G (0.174, p < 0.001), FANCD2 (0.267, p < 0.001), CISD1 (0.233, p < 0.001), FDFT1 (0.136, p < 0.001), SLC1A5 (0.276, p < 0.001), TFRC (0.287, p < 0.001), RPL8 (0.126, p < 0.001), LPCAT3 (0.083, p = 0.006), CS (0.292, p < 0.001), CARS1 (0.266, p < 0.001), ATP5MC3 (0.445, p < 0.001), and ATL1 (0.081, p = 0.007). We draw a scatter plot to show the correlation between GPI and ferroptosis-related genes (Figures 6G–I). In addition, we divided 1,083 tumor samples into two groups based on GPI expression, with 542 samples in the high-expression group and 541 samples in the low-expression group. We tried to analyze the differential expression of 25 ferroptosis-related genes between different GPI expression groups to determine whether the ferroptosis is different between high GPI expression level and low GPI expression level in BRCA (Figures 6J–L). The results showed that, compared with that in the low expression group, the expression of HSPA5, EMC2, SLC7A11, MT1G, FANCD2, CISD1, FDFT1, SLC1A5, TFRC, RPL8, CS, CARS1 and ATP5MC3 increased in the high expression group of GPI (p < 0.05). In contrast, compared with that in the high expression group, the expression of CDKN1A, NFE2L2, SAT1, NCOA4, CLS2, and DPP4 increased in the low expression group of GPI (p < 0.05). The above results indicated that GPI was closely related to ferroptosis in BRCA.
To understand the mutation level of GPI in BRCA, we analyzed its genome and copy number. We analyzed the OncoPrint map of the GPI gene of BRCA patients in TCGA dataset using a cBioPortal map, and the results showed that GPI had 2.6% gene missense mutations, truncating mutations, amplification, and deep deletion (Figure 7A). In Figure 7B, we showed additional mutations and their location within GPI. We found genetic alteration and their location in the GPI domain. For instance, a missense mutation, W391C alteration, in the GPI domain, was only detected in one case of BRCA. We acquired the W391C site visualized on the 3D structure of GPI protein (Figure 7B). To see whether there is a relationship between certain genetic alterations of GPI and the clinical survival of patients, we systematically studied and correlated these in BRCA patients. The result showed that BRCA patients with genetic alteration of GPI had a poor prognosis in DFS (p = 0.0403), but not PFS (p = 0.162), OS (p = 0.923), and DSS (p = 0.697), compared with patients without GPI alterations (Figure 7C).
Figure 7 Mutation feature of GPI and survival prediction in BRCA. We analyzed the mutation features of GPI for BRCA using the cBioPortal tool. (A) GPI genome changes and (B) mutation site (W391C) in the 3D structure of GPI are displayed. (C) We also analyzed the potential correlation between mutation status, disease-free survival, progression-free survival, disease-specific survival, and overall BRCA. (D) Nomogram for predicting the 1-, 3-, and 5-year overall survival rates. (E) Calibration for nomogram.
To better predict BRCA patients’ prognosis, a nomogram was constructed based on the Cox regression analysis results using the RMS R package (Figure 7D). Five prognostic factor variables, GPI expression, age, PAM50, T stage, and N stage, were included in the model. Based on multivariate Cox analysis, a point scale was used to assign points to these variables. The straight line was drawn upward to determine the points of the variables, and the sum of the points assigned to each variable was rescaled to a range of 0–100. The points of each variable were accumulated and recorded as the total points. The probability of BRCA patient survival at 1, 3, and 5 years was determined by drawing a line from the total point axis straight down to the outcome axis. The prediction results of the nomogram calibration curve of OS were consistent with all patients’ observation results (Figure 7E).
The previous reports have indicated that GPI is significantly related to tumor metastasis and poor prognosis of some tumors (10–12). In this study, database analysis showed that the expression levels of GPI in many human tumors were frequently altered. Although the role of GPI in the development and prognosis of some cancers has been partially elucidated, there were few bioinformatics analyses of GPI expression and function in breast cancer. It was the first time to study the expression, gene alteration, regulatory pathway, and biological function of GPI in breast cancer and its influence on the prognosis in patients with breast cancer via bioinformatics analysis. In this study, except for LAML and LGG, the expression level of GPI in plenty of tumors was significantly higher than in adjacent normal tissues. IHC from our breast carcinoma specimen also demonstrated this result. In addition, our study indicated that the expression level of GPI was significantly related to the tumor stage, ER or PR state, and age. Similarly, Han's study (10) pointed out that the expression level of GPI in LUAD was significantly higher than that in paracarcinoma tissues. Rose (21) reported that serum GPI was often elevated in patients with breast cancer. In addition, we tried to examine the correlation of expression and prognostic of the GPI gene in BRCA and found that a high GPI expression was correlated with poor OS in BRCA. At the same time, a high GPI expression was correlated with poor clinicopathological characteristics, such as stage III, over 60 years old, N3, HER2 negative, and ER negative. The prediction results of the nomogram calibration curve of OS were consistent with all patients’ observation results. In agreement with our results, some studies also showed that increased expression levels of GPI were associated with poor prognosis in patients with clear cell-renal cell carcinoma (11), lung carcinomas (10, 22), endometrial carcinoma (23), and BRCA (24). These results strongly indicated that GPI may be used as a biomarker for prognosis.
Previous studies showed that GPI was associated with a great deal of genes or proteins (10, 25). This study aimed to clarify the underlying biological function of GPI and the influence of GPI on the prognosis of BRCA. We performed the analysis of the GO network, KEGG network, PPI network, gene alteration, and regulation of cell pathways. We found that 25 genes and 10 proteins were positively correlated with GPI. Further analysis suggested that the functions of these genes and proteins are mainly involved in cell cycle signaling pathways, nuclear division, and mitotic nuclear division of the cell cycle regulatory genes, including CCNA2, CCNB1, CCNB2, CCNE1, CHEK1, BUB1B, ESPL1, PTTG1, PCNA, PKMYT1, CDC45, PLK1, MCM2, MCM4, MCM6, E2F1, CDC6, CDC20, CDC25A, and CDC25C. Similarly, Han's results (10) showed that GPI was positively correlated with cell cycle regulatory genes in LUAD and that knockout GPI can prevent LUAD cells from transitioning from the G2 phase to the M phase. In addition, in this study, we observed that GPI was closely related to m6A RNA methylation modification in BRCA, and there was a difference in m6A RNA methylation alterations between the high GPI expression group and the low GPI expression group. Interestingly, our results showed that GPI in BRCA had 2.6% gene alterations, such as missense mutations, truncating mutations, amplification, and deep deletion. BRCA patients with gene alterations of GPI had a poor prognosis in DFS. Recent studies have verified that ferroptosis is a new form of regulated cell death and is related to the progress of BRCA (26, 27). In the present study, the database analysis revealed the correlation between GPI and ferroptosis-related genes in BRCA and that there was a difference in ferroptosis-related gene expression between the high GPI expression group and the low GPI expression group. The development, progress, metastasis, and clinical survival results of cancer were related to its tumor microenvironment consisting of immune cells, the extracellular matrix, and inflammatory mediators (28). Some reports indicated that GPI-induced arthritis was involved in a great deal of immune cells (29, 30). This study explored the correlation between the expression of GPI and the level of immune infiltration of BRCA. Our results showed that there was a different immune cell infiltration between the high GPI expression group and the low GPI expression group, which was similar to Han's results (10). These findings showed that GPI may play an important role in the tumor immune microenvironment regulation in BRCA. These data suggested that GPI may regulate the progression of BRCA by regulating the cell cycle, m6A RNA methylation modification, gene alteration, and tumor microenvironment.
In summary, our study confirmed that the expression of GPI is significantly upregulated and is closely correlated to the poor prognosis of BRCA patients. GPI may affect the progression of BRCA by regulating the cell cycle, m6A RNA methylation modification, gene alteration, and immune infiltration, which may serve as a new prognostic biomarker for BRCA patients.
The original contributions presented in the study are included in the article/Supplementary Material. Further inquiries can be directed to the corresponding author.
This study was reviewed and approved by Hunan Provincial People’s Hospital, the First Affiliated Hospital of Hunan Normal University. The patients/participants provided their written informed consent to participate in this study.
JNY, YZ, LZ, ST and PY and helped with data collection. JY, JZ and JY wrote the manuscript. JNY, PF, LL and CZ designed and oversaw the study. All authors contributed to the article and approved the submitted version.
Author ST was employed by Huazhi Medical Laboratory Co., Ltd.
The remaining authors declare that the research was conducted in the absence of any commercial or financial relationships that could be construed as a potential conflict of interest.
All claims expressed in this article are solely those of the authors and do not necessarily represent those of their affiliated organizations, or those of the publisher, the editors and the reviewers. Any product that may be evaluated in this article, or claim that may be made by its manufacturer, is not guaranteed or endorsed by the publisher.
The Supplementary Material for this article can be found online at: https://www.frontiersin.org/articles/10.3389/fendo.2022.995972/full#supplementary-material
1. Sung H, Ferlay J, Siegel RL, Laversanne M, Soerjomataram I, Jemal A, et al. Global cancer statistics 2020: GLOBOCAN estimates of incidence and mortality worldwide for 36 cancers in 185 countries. CA Cancer J Clin (2021) 71:209–49. doi: 10.3322/caac.21660
2. Yi J, Chen S, Yi P, Luo J, Fang M, Du Y, et al. Pyrotinib sensitizes 5-Fluorouracil-Resistant HER2(+) breast cancer cells to 5-fluorouracil. Oncol Res (2020) 28:519–31. doi: 10.3727/096504020X15960154585410
3. Yamashita T, Masuda N, Saji S, Araki K, Ito Y, Takano T, et al. Trastuzumab, pertuzumab, and eribulin mesylate versus trastuzumab, pertuzumab, and a taxane as a first-line or second-line treatment for HER2-positive, locally advanced or metastatic breast cancer: Study protocol for a randomized controlled, non-inferiority, phase III trial in Japan (JBCRG-M06/EMERALD). Trials (2020) 21:391. doi: 10.1186/s13063-020-04341-y
4. Cardoso F, Castiglione M. Locally recurrent or metastatic breast cancer: ESMO clinical recommendations for diagnosis, treatment and follow-up. Ann Oncol (2009) 20 Suppl 4:15–8. doi: 10.1093/annonc/mdp115
5. Yi J, Tan S, Zeng Y, Zou L, Zeng J, Zhang C, et al. Comprehensive analysis of prognostic and immune infiltrates for FOXPs transcription factors in human breast cancer. Sci Rep (2022) 12:8896. doi: 10.1038/s41598-022-12954-3
6. Bae YK, Shim YR, Choi JH, Kim MJ, Gabrielson E, Lee SJ, et al. Gene promoter hypermethylation in tumors and plasma of breast cancer patients. Cancer Res Treat (2005) 37:233–40. doi: 10.4143/crt.2005.37.4.233
7. Knight AL, Yan X, Hamamichi S, Ajjuri RR, Mazzulli JR, Zhang MW, et al. The glycolytic enzyme, GPI, is a functionally conserved modifier of dopaminergic neurodegeneration in parkinson’s models. Cell Metab (2014) 20:145–57. doi: 10.1016/j.cmet.2014.04.017
8. Kim JW, Dang CV. Multifaceted roles of glycolytic enzymes. Trends Biochem Sci (2005) 30:142–50. doi: 10.1016/j.tibs.2005.01.005
9. Kassahn D, Kolb C, Solomon S, Bochtler P, Illges H. Few human autoimmune sera detect GPI. Nat Immunol (2002) 3:411–2. doi: 10.1038/ni0502-411b
10. Han J, Deng X, Sun R, Luo M, Liang M, Gu B, et al. GPI is a prognostic biomarker and correlates with immune infiltrates in lung adenocarcinoma. Front Oncol (2021) 11:752642. doi: 10.3389/fonc.2021.752642
11. Lucarelli G, Rutigliano M, Sanguedolce F, Galleggiante V, Giglio A, Cagiano S, et al. Increased expression of the autocrine motility factor is associated with poor prognosis in patients with clear cell-renal cell carcinoma. Med (Baltimore) (2015) 94:e2117. doi: 10.1097/MD.0000000000002117
12. van Veen M, Matas-Rico E, van de Wetering K, Leyton-Puig D, Kedziora KM, De Lorenzi V, et al. Negative regulation of urokinase receptor activity by a GPI-specific phospholipase c in breast cancer cells. Elife (2017) 6:e23649. doi: 10.7554/eLife.23649
13. Li T, Fan J, Wang B, Traugh N, Chen Q, Liu JS, et al. TIMER: A web server for comprehensive analysis of tumor-infiltrating immune cells. Cancer Res (2017) 77:e108–108e110. doi: 10.1158/0008-5472.CAN-17-0307
14. Tang Z, Li C, Kang B, Gao G, Li C, Zhang Z. GEPIA: a web server for cancer and normal gene expression profiling and interactive analyses. Nucleic Acids Res (2017) 45:W98–98W102. doi: 10.1093/nar/gkx247
15. Szklarczyk D, Gable AL, Lyon D, Junge A, Wyder S, Huerta-Cepas J, et al. TRING v11: protein-protein association networks with increased coverage, supporting functional discovery in genome-wide experimental datasets. Nucleic Acids Res (2019) 47:D607–607D613. doi: 10.1093/nar/gky1131
16. Gao J, Aksoy BA, Dogrusoz U, Dresdner G, Gross B, Sumer SO, et al. Integrative analysis of complex cancer genomics and clinical profiles using the cBioPortal. Sci Signal (2013) 6:pl1. doi: 10.1126/scisignal.2004088
17. Cerami E, Gao J, Dogrusoz U, Gross BE, Sumer SO, Aksoy BA, et al. The cBio cancer genomics portal: an open platform for exploring multidimensional cancer genomics data. Cancer Discov (2012) 2:401–4. doi: 10.1158/2159-8290.CD-12-0095
18. Li J, Miao B, Wang S, Dong W, Xu H, Si C, et al. Hiplot: a comprehensive and easy-to-use web service for boosting publication-ready biomedical data visualization. Brief Bioinform (2022) 23:15. doi: 10.1093/bib/bbac261
19. Liu Z, Zhao Q, Zuo ZX, Yuan SQ, Yu K, Zhang Q, et al. Systematic analysis of the aberrances and functional implications of ferroptosis in cancer. iScience (2020) 23:101302. doi: 10.1016/j.isci.2020.101302
20. Li Y, Xiao J, Bai J, Tian Y, Qu Y, Chen X, et al. Molecular characterization and clinical relevance of m(6)A regulators across 33 cancer types. Mol Cancer (2019) 18:137. doi: 10.1186/s12943-019-1066-3
21. ROSE A, WEST M, ZIMMERMAN HJ. Serum enzymes in disease v. isocitric dehydrogenase, malic dehydrogenase, and glycolytic enzymes in patients with carcinoma of the breast. Cancer (1961) 14:726–33. doi: 10.1002/1097-0142(199007/08)14:4<726::aid-cncr2820140409>3.0.co;2-1
22. Dobashi Y, Watanabe H, Sato Y, Hirashima S, Yanagawa T, Matsubara H, et al. Differential expression and pathological significance of autocrine motility factor/glucose-6-phosphate isomerase expression in human lung carcinomas. J Pathol (2006) 210:431–40. doi: 10.1002/path.2069
23. Wu ST, Liu B, Ai ZZ, Hong ZC, You PT, Wu HZ, et al. Esculetin inhibits cancer cell glycolysis by binding tumor PGK2, GPD2, and GPI. Front Pharmacol (2020) 11:379. doi: 10.3389/fphar.2020.00379
24. Gallardo-Pérez JC, Adán-Ladrón de Guevara A, Marín-Hernández A, Moreno-Sánchez R, Rodríguez-Enríquez S. HPI/AMF inhibition halts the development of the aggressive phenotype of breast cancer stem cells. Biochim Biophys Acta Mol Cell Res (2017) 1864:1679–90. doi: 10.1016/j.bbamcr.2017.06.015
25. Funasaka T, Hogan V, Raz A. Phosphoglucose isomerase/autocrine motility factor mediates epithelial and mesenchymal phenotype conversions in breast cancer. Cancer Res (2009) 69:5349–56. doi: 10.1158/0008-5472.CAN-09-0488
26. Zhu J, Dai P, Liu F, Li Y, Qin Y, Yang Q, et al. Upconverting nanocarriers enable triggered microtubule inhibition and concurrent ferroptosis induction for selective treatment of triple-negative breast cancer. Nano Lett (2020) 20:6235–45. doi: 10.1021/acs.nanolett.0c00502
27. Dias Lopes NM, Marinello PC, Sanches LJ, da Silva Brito WA, Lovo-Martins MI, Pinge-Filho P, et al. Patterns of cell death induced by metformin in human MCF-7 breast cancer cells. Pathol Res Pract (2020) 216:153199. doi: 10.1016/j.prp.2020.153199
28. Wang M, Chang M, Li C, Chen Q, Hou Z, Xing B, et al. Tumor-Microenvironment-Activated reactive oxygen species amplifier for enzymatic cascade cancer Starvation/Chemodynamic/Immunotherapy. Adv Mater (2022) 34:e2106010. doi: 10.1002/adma.202106010
29. Frey O, Bruns L, Morawietz L, Dunussi-Joannopoulos K, Kamradt T. B cell depletion reduces the number of autoreactive T helper cells and prevents glucose-6-phosphate isomerase-induced arthritis. PloS One (2011) 6:e24718. doi: 10.1371/journal.pone.0024718
Keywords: Glucose-6-phosphate isomerase (GPI), biomarker, prognosis, breast cancer, immune infiltrates, cell cycle
Citation: Zeng J, Yi J, Tan S, Zeng Y, Zou L, Zhang C, Liu L, Yi P, Fan P and Yu J (2022) GPI: An indicator for immune infiltrates and prognosis of human breast cancer from a comprehensive analysis. Front. Endocrinol. 13:995972. doi: 10.3389/fendo.2022.995972
Received: 16 July 2022; Accepted: 01 September 2022;
Published: 28 September 2022.
Edited by:
Raghuveer Kavarthapu, National Institutes of Health (NIH), United StatesReviewed by:
Vijaya Kumar Pidugu, National Cancer Institute (NIH), United StatesCopyright © 2022 Zeng, Yi, Tan, Zeng, Zou, Zhang, Liu, Yi, Fan and Yu. This is an open-access article distributed under the terms of the Creative Commons Attribution License (CC BY). The use, distribution or reproduction in other forums is permitted, provided the original author(s) and the copyright owner(s) are credited and that the original publication in this journal is cited, in accordance with accepted academic practice. No use, distribution or reproduction is permitted which does not comply with these terms.
*Correspondence: Jie Yu, eXV4aWFvamllMjAxMkBzaW5hLmNvbQ==; Peizhi Fan, RmFucHpoNjRAMTYzLmNvbQ==
†These authors have contributed equally to this work
Disclaimer: All claims expressed in this article are solely those of the authors and do not necessarily represent those of their affiliated organizations, or those of the publisher, the editors and the reviewers. Any product that may be evaluated in this article or claim that may be made by its manufacturer is not guaranteed or endorsed by the publisher.
Research integrity at Frontiers
Learn more about the work of our research integrity team to safeguard the quality of each article we publish.