- 1School of Nursing, Department of Physiological Nursing, University of California, San Francisco, San Francisco, CA, United States
- 2College of Biological Sciences, University of California at Davis, Davis, CA, United States
- 3College of Dentistry, New York University, New York, NY, United States
- 4Department of Epidemiology and Biostatistics, School of Medicine, University of California at San Francisco, San Francisco, CA, United States
- 5School of Nursing, Department of Family Health Care Nursing, University of California, San Francisco, San Francisco, CA, United States
- 6Earle A. Chiles Research Institute, Providence St Joseph Health, Portland, OR, United States
- 7Obstetrics and Gynecology, Reproductive Sciences, School of Medicine, University of California at San Francisco, San Francisco, CA, United States
- 8Department of Epidemiology, College of Public Health, University of Iowa, Iowa City, IA, United States
Purpose: Gestational diabetes (GDM) is associated with increased risk for preterm birth and related complications for both the pregnant person and newborn. Changes in gene expression have the potential to characterize complex interactions between genetic and behavioral/environmental risk factors for GDM. Our goal was to summarize the state of the science about changes in gene expression and GDM.
Design: The systematic review was conducted using the Preferred Reporting Items for Systematic Reviews and Meta-Analyses guidelines.
Methods: PubMed articles about humans, in English, from any date were included if they described mRNA transcriptome or microRNA findings from blood samples in adults with GDM compared with adults without GDM.
Results: Sixteen articles were found representing 1355 adults (n=674 with GDM, n=681 controls) from 12 countries. Three studies reported transcriptome results and thirteen reported microRNA findings. Identified pathways described various aspects of diabetes pathogenesis, including glucose and insulin signaling, regulation, and transport; natural killer cell mediated cytotoxicity; and fatty acid biosynthesis and metabolism. Studies described 135 unique miRNAs that were associated with GDM, of which eight (miR-16-5p, miR-17-5p, miR-20a-5p, miR-29a-3p, miR-195-5p, miR-222-3p, miR-210-3p, and miR-342-3p) were described in 2 or more studies. Findings suggest that miRNA levels vary based on the time in pregnancy when GDM develops, the time point at which they were measured, sex assigned at birth of the offspring, and both the pre-pregnancy and gestational body mass index of the pregnant person.
Conclusions: The mRNA, miRNA, gene targets, and pathways identified in this review contribute to our understanding of GDM pathogenesis; however, further research is warranted to validate previous findings. In particular, longitudinal repeated-measures designs are needed that control for participant characteristics (e.g., weight), use standardized data collection methods and analysis tools, and are sufficiently powered to detect differences between subgroups. Findings may be used to improve early diagnosis, prevention, medication choice and/or clinical treatment of patients with GDM.
1 Introduction
Gestational diabetes (GDM) is associated with an increased risk for preterm birth and related complications for both the pregnant person and newborn (1). GDM is defined as chronic hyperglycemia that begins in the second or third trimester of pregnancy (1). The prevalence of GDM is increasing, and ranges from 1.7-11.6% internationally and from 2.5-7.6% in North America (2). GDM is often associated with pancreatic β-cell dysfunction that may lead to onset of diabetes after pregnancy (1, 3). Additionally, the offspring of pregnant people affected by GDM are more likely to develop attention deficit hyperactivity disorder, autism spectrum disorder, diabetes, and obesity (4–6). The pathogenesis of GDM is unclear, but evidence supports links between obesity, adipokines (the signaling molecules secreted by adipose tissue), or disruptions in oxidative stress mechanisms and GDM incidence (7, 8).
Changes in gene expression have the potential to characterize complex interactions between genetic and behavioral/environmental risk factors for GDM (8–10). Messenger ribonucleic acid (mRNA) are single stranded RNAs that translate genetic information into biologically active molecules. Given that mRNAs characterize, affect, and/or are associated with the expression of genes within an individual’s environment and context, they may be useful biomarkers of risk for GDM and potentially provide insights about the underlying mechanisms of risk (11). A previous review of the literature summarized genes that were differently expressed in GDM compared to other types of diabetes (8, 12). Differently expressed genes were influenced by GDM disease duration, obesity, number of gestations, glucose serum levels and the use of medications (12). However, prior studies have reported discrepant findings and it remains unclear how mRNA expression differs between blood samples from pregnant individuals with GDM relative to healthy individuals without GDM.
MicroRNAs, or miRNAs, are small, non-coding RNAs that interfere with mRNA translation to alter the expression levels of gene products (13, 14). Current biomarkers for GDM do not provide early detection of who is at greatest risk, do not characterize differences in the specific risk profile, and do not always predict resulting complications (15, 16). Circulating miRNAs are promising diagnostic biomarkers that represent environmental or behavioral influences on gene expression, potentially providing a more comprehensive measure of risk (15–19). A prior literature review summarized miRNA expression in the blood of pregnant people with GDM compared with healthy controls and found initial evidence that select miRNAs may be promising biomarkers for early detection of GDM risk, but concluded that there was insufficient overall evidence, particularly from samples of diverse individuals, to draw definitive conclusions (10).The purpose of this systematic review was to summarize the research about differences in circulating transcriptome (mRNA) and miRNA levels of adults with GDM compared with healthy controls. This paper updates previous literature reviews by incorporating both mRNA and miRNA studies in the synthesized analysis for a more robust understanding of the pathogenesis of GDM and associated complications.
2 Materials and methods
The systematic review was conducted using the Preferred Reporting Items for Systematic Reviews and Meta-Analyses (PRISMA) guidelines (19). The research question was formulated using the acronym PICOS, which stands for population, intervention/exposure, comparators, outcomes, and study designs. The study population is pregnant adults with GDM or adults with a history of GDM. The intervention/exposure in this case is an exposure to GDM during pregnancy. The comparator is adults without GDM. The study designs of interest were observational, cohort, quasi experimental, or experimental designs. The literature was searched by two independent reviewers (KL, JC) in PubMed for primary research articles about humans available in English from any date. The search terms, defined by a medical librarian experienced with systematic reviews, were as follows: “diabetes, gestational” [MeSH Terms] AND (“transcriptome” [MeSH Terms] OR “gene expression” [MeSH Terms] OR “micrornas” [MeSH Terms] OR “epigenomics”[MeSH Terms] or “Gene Expression Profiling”[Mesh]) AND ((humans[Filter]) AND (English[Filter])) AND ((humans[Filter]) AND (English[Filter])).
Included articles described the full transcriptome, the miRNAome, or a panel of at least three or more miRNAs in blood samples from adults with GDM compared with adults without GDM. Studies were excluded if they did not include a healthy control group for comparison, or if they were exclusively mechanistic studies conducted in vitro using a pregnant person’s blood samples.
Data were extracted about study and sample characteristics, study methods, instrumentation and data acquisition, validation, key findings, and limitations. This systematic review extracted the data based on the terminology reported by the authors of the studies included, including for race/ethnicity, sex/gender, and trimester in which the blood was drawn. A weighted mean and standard deviation were calculated using Excel software for the participant’s age, body mass index (BMI), and gestational age at the time of the blood draw, grouped by GDM or healthy controls. A formal risk of bias analysis was conducted using the Cochrane criteria for observational studies (20, 21).
3 Results
The PRISMA flow diagram provides an overview of the search strategy (Figure 1). After eliminating duplicates and applying inclusion and exclusion criteria, 16 articles were found representing 1375 adults (n=684 with GDM, n=691 controls) from 12 countries. Three studies reported transcriptome results and 13 reported miRNA-ome findings. An overview of the included studies and their key findings are presented in Tables 1–4.
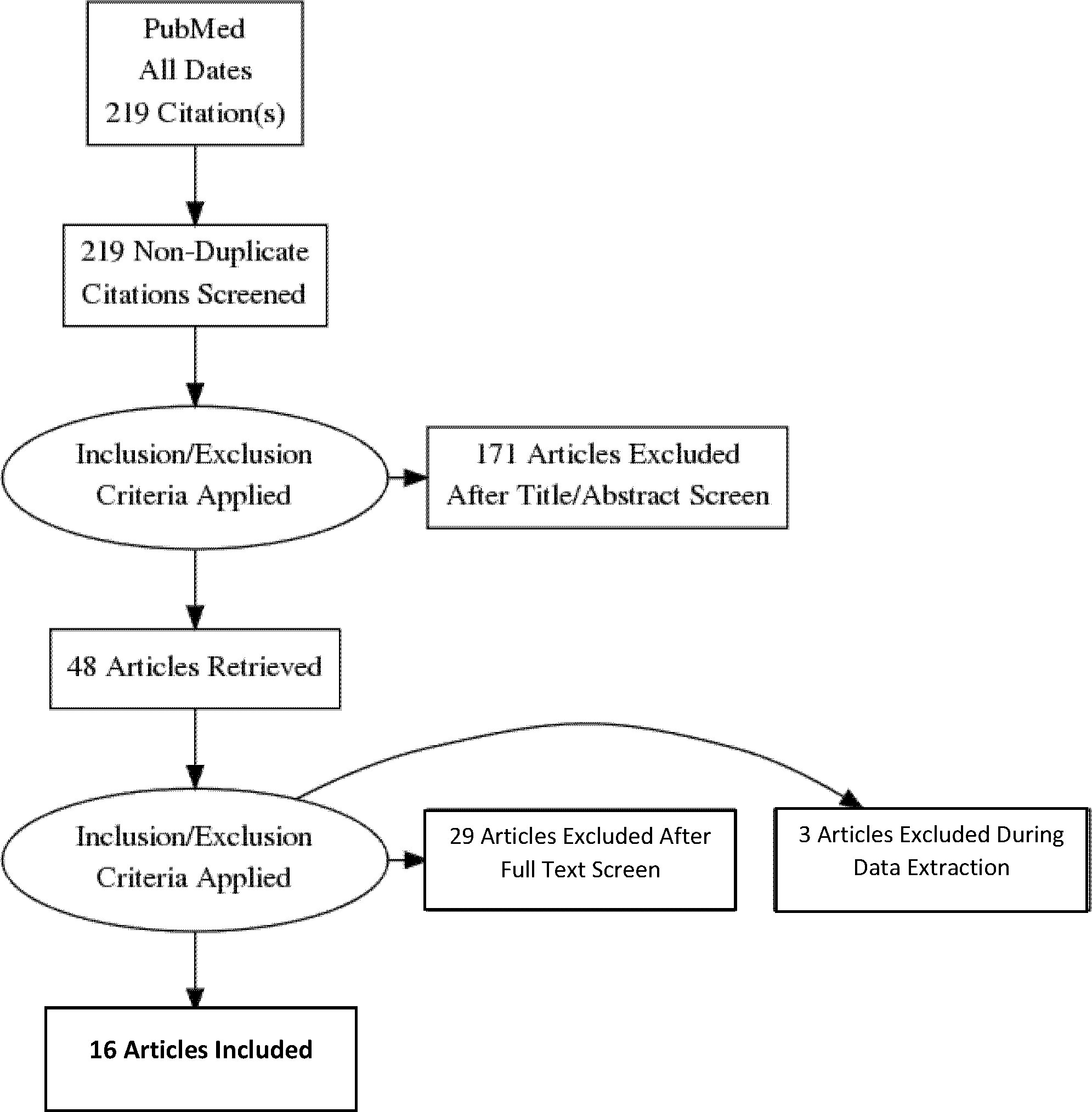
Figure 1 Preferred reporting Items for systematic reviews and meta-analyses flow diagram of the search strategy.
3.1 Sample characteristics
Data were extracted from the included studies about participants’ age, race, ethnicity, nationality, BMI, and gestational age at the time of blood sampling (Tables 1, 2). Overall, participants with GDM were slightly older than participants in the healthy control groups (t(1256) = 4.36 years, p<0.0001). The weighted mean age of the GDM group was 31.9 ± 3.0 years. The weighted mean age of the control group was 31.1 ± 3.5 years.
In the transcriptome studies, the participants’ nationality was reported in two studies, ethnicity was reported in one study, and race was reported in one study. The sample of participants from the transcriptome studies was 75% Greek nationality (22), 14% South African nationality and Black race (23), and 11% Chinese ethnicity (24). The articles did not define how race, ethnicity, or nationality were measured (i.e., self-identified, reference criteria, or other method). Seven of the 13 miRNA studies, representing 48.4% of miRNA participants, did not report race, ethnicity, or nationality of their participants (25–31). Of the miRNA studies that did, two reported nationalities, described as 12% South African; 10% Canadian; and 1% Other. Four studies reported race (12% Black, 55% White, 28% Other). One study reported ethnicity as European (15%) or Non-European (3%). Wander et al. (2017) included Hispanic ethnicity as a category in their race demographic, but they did not report the findings (32).
The time point in which BMI was measured varied by study. Five studies measured pre-pregnancy BMI (23, 29, 30, 32, 33). Ten studies measured BMI at the time of the blood sampling (23–25, 27–29, 31, 33–35). Five studies also measured gestational weight gain (22, 23, 30, 32, 33). The BMI ranges of included participants also varied by study. Overall, the BMI of the GDM group was significantly higher than the control group (t(1256) = 11.26 kg/m2, p<0.0001). The weighted mean BMI of the GDM group was 26.2 ± 3.2 kg/m2 and the control group was 24.2 ± 3.1 kg/m2. The participants in two of the three transcriptome studies had BMIs in the overweight and obese ranges. In ten of the miRNA studies, the BMI of the GDM group was significantly higher than control groups at the time of the blood draw. Two of the miRNA studies differentiated results unique to the lean participants in their samples (30, 32).
Figure 2 provides an overview of the gestational age at the time of the blood sample collection for mRNA and miRNA analysis. Although the gestational age at time of blood draw varied by study, there was no difference in the weighted mean gestational age of participants with GDM compared to controls for the overall sample.
3.2 Study characteristics
Data were extracted about the study design, GDM definition and diagnostic criteria used, exclusion criteria, tissue type, blood sample collection time points, and trimester represented for all included studies (Tables 1, 2). All studies were observational and cross-sectional, except for two studies which measured the miRNAs at three time points each (25, 26).
The criteria used to define and diagnose GDM varied by study. Eight of the studies cited the International Association of Diabetes and Pregnancy Study Groups’ criteria (23, 25, 28, 29, 33, 35–37), six cited the ADA (22, 25–27, 31, 32), two cited the National Diabetes Data Group (24, 30), one cited Society of Obstetricians and Gynaecologists of Canada (34), and one referenced the World Health Organization (37). The exclusion criteria were also unstandardized. Self-reported measures or prior clinic records were used most often to exclude pre-gestational diabetes. Some studies excluded participants with specific conditions, fetal abnormalities, and/or complicated pregnancies from both groups (23, 25–27, 30, 31, 34–37) while another excluded complicated pregnancies only in the control group (32), and still others did not specify if there were exclusions except prior history of diabetes and carbohydrate metabolism disorders (22, 24, 28, 29, 33). Six of the studies included only singleton pregnancies (23, 27, 31, 32, 36, 37); the others did not specify whether the pregnancies reflected by the study results were singletons or multiple gestation.
The point in the pregnancy in which mRNAs and miRNAs were assessed was disparate across the studies, as detailed in Figure 2. In addition, the definition of a particular trimester by gestational age in weeks was unstandardized and inconsistently reported. For example, two studies measured only participants in their first trimester (29, 34), but they used different weeks to define the end of the first trimester (i.e., <12 weeks or <14 weeks). Four studies reported miRNA results discretely within the second trimester, which was defined as weeks 13-26 (25–27, 31), and three studies were discretely within the third trimester, defined as weeks 27 through the end of pregnancy (22, 23, 26). Two studies reported findings from the time period that corresponds with the standard of care for assessing for gestational diabetes (weeks 24-28), although this range spans late second trimester to early third trimester (25, 36). Others’ ranges spanned two or more trimesters (28, 31–33, 37).
3.3 Instrumentation, data acquisition, and data analysis
Instrumentation, data acquisition, and analysis methods were disparate across studies (Tables 1–3). The three transcriptome studies each used different methods for mRNA quantitation (22–24). One study used RNA-Sequencing methods (23), and another used microarray methods (24). Pappa et al. (2013) used real time quantitative polymerase chain reaction (qPCR) for quantitation since they evaluated a panel of ten “clock genes” associated with diurnal rhythms. Both Steyn et al. (2019) and Zhao et al. (2011) used reverse transcription qPCR (RT-qPCR) for internal validation (23, 24). Twelve out of the 13 miRNA studies (92%) used real time qPCR methods for miRNA quantitation (Table 2). The other study (8%) used the Nanostring ncounter platform (29). Internal and external validation methods were included in 54% and 23% of miRNA studies, respectively. Table 3 provides an overview of the bioinformatics tools used in each of the studies where applicable.
3.4 Transcriptome studies
The transcriptome studies collectively identified 6,289 differentially expressed genes (DEGs). Overall, 2,702 DEGs (43.0%) were described as upregulated in participants with GDM compared to controls, while 2,499 DEGs (39.7%) were described as downregulated in GDM compared to controls. The candidate genes and target pathway findings are detailed in Table 1. The transcriptome pathways identified described various aspects of diabetes pathogenesis, including insulin and glucose signaling, regulation, and transport; natural killer cell mediated cytotoxicity; NADP and carbohydrate metabolism; immunity and inflammation; fatty acid biosynthesis and metabolism; and circadian clock rhythms.
The transcriptome studies analyzed correlations between mRNA levels and outcomes like glucose levels in the pregnant person, insulin resistance/insulin sensitivity, hemoglobin A1c (HbA1c), and birth weight. Glucose-6-phosphate dehydrogenase (G6PD), insulin-like growth factor binding protein (IGFBP-1), IGFBP-2, and transketolase (TKT) were inversely correlated with glucose levels in the pregnant person, measured at one or more time points. No significant correlation was found between IGFBP-6 and glucose levels. Period circadian level 3 (PER3) was inversely correlated with HbA1c. IGFBP-1 was positively correlated with the infants’ birthweight, while IGFBP-2 and IGFBP-6 were not significantly correlated with birthweight.
3.5 miRNA studies
In the thirteen miRNA studies, 135 unique miRNAs were associated with GDM. Eight (miR-16-5p, miR-17-5p, miR-20a-5p, miR-29a-3p, miR-195-5p, miR-222-3p, miR-210-3p, and miR-342-3p) were described in two or more studies. See Table 4 for details about the number of studies that described each miRNA, and whether or not it was found to be upregulated, downregulated, or no difference from healthy controls.
Within the studies, miRNA levels varied based on the time in pregnancy when GDM develops, the trimester or time period at which miRNAs were measured, sex of the fetus, obesity in the pregnant person, and treatment type (diet vs. pharmaceutical).
3.5.1 Time in pregnancy
Two studies measured the miRNAs at multiple time points from the same participants (25, 26). In those studies, expression levels of miR-16-5p, miR-17-5p, and miR-20a-5p increased in each trimester as compared with the first trimester in the GDM group but remained constant in the control group (25). In contrast, miR-125b-5p increased over time from the first trimester to the third for the control group (26). Expression of miR-125b-5p was significantly higher in the GDM group compared to healthy controls in the first trimester, but then levels decreased 10-fold below those of the control group in the second trimester GDM samples (26). In control samples, expression levels of miR-183-5p, miR-200b-3p, and miR-125b-5p were the highest in the second trimester, whereas the highest level of miR-137 was observed in the third trimester (26).
When comparing first trimester GDM expression levels to controls, miR-183-5p, miR-200b-3p, miR-125b-5p, miR-1290 (26), miR-223, and miR-23a (29) were higher in the GDM group. During the second trimester, in the GDM group, the expression levels of miR-183-5p (26), miR-16-5p, miR-17-5p, miR-19a-3p, miR-19b-3p, miR-20a-5p (25, 27), were higher and miR-128-5p (26), miR-132, miR-29a, and miR-22 (31) were lower as compared with the control group. Finally, during the third trimester, in the GDM group, studies reported a higher level of miR-137 and lower levels of miR-183-5p and miR-200b-3p relative to the control group (26).
Four studies measured miRNA expression at the time of GDM diagnosis, between 24-28 weeks gestation and collectively identified 35 miRNAs that were significantly different in the GDM group compared to controls (25, 30, 33, 36). MiR-222-3p was significantly lower in South African pregnant people with GDM relative to controls (36). MiR-16-5p, miR-17-5p (25), miR-340 (33), and miR-330-3p (30) were significantly upregulated in the GDM group. Stirm et al. (2018) identified an additional 29 miRNAs that were significantly upregulated in the GDM group (false discovery rate <0.1) compared to controls, although miR-340 was the only one that was validated in their study (33). MiR-20a-5p was reported in two studies with mixed results. In one study conducted in China, miR-20a-5p was significantly higher in the GDM group, whereas in another study of South African pregnant people, miR-20a-5p was significantly lower.
One study measured miRNA levels in the formerly pregnant person an average of 5 years after birth of the baby (35). They found 26 miRNAs that were significantly higher in participants with a history of GDM compared to controls. A composite of 16 of the 26 miRNAs was identified as the best predictor of GDM exposure during pregnancy in their participants.
3.5.2 Sex assigned at birth of the offspring
Two studies considered sex assigned at birth of the offspring in their analyses. Wander et al. (2017) differentiated results by sex of the offspring which was determined using retrospective chart review after delivery (32). They detected six miRNAs (miR-155-5p, miR-21-3p, miR-146b-5p, miR-223-3p, miR-517-5p, and miR-29a-3p) that were significantly different in the blood samples of participants with GDM carrying male fetuses. Conversely, Sorensen et al. (2021) found no sex-dependent differences in expression of miR-29a-3p and miR134-5p (37).
3.5.3 Associations with BMI
In most studies, the GDM group had a significantly higher BMI at the time of the blood draw compared to the control group. Three studies reported findings for lean (i.e., pre-pregnancy BMI <25 kg/m2), overweight/obese (i.e., pre-pregnancy BMI ≥ 25 kg/m2), or obese (i.e., pre-pregnancy BMI ≥ 29 kg/m2) pregnant people (30, 32, 37). Sorensen et al. (2021) identified three miRNAs (miR-16-5p, miR-29a-3p, miR-134-5p) that were higher at baseline in obese women who went on to develop GDM (37). These three miRNAs combined differentiated those women who developed GDM earlier in pregnancy from those who developed GDM later in pregnancy, with the highest levels at baseline in women who developed GDM late in pregnancy. In the other two studies, miR-21-3p, miR-210-3p (32), and miR-330-3p (30) were significantly increased in obese but not lean women.
3.5.4 Differences by treatment type
Two studies analyzed miRNA findings based on GDM treatment type (30, 35). Higher levels of miR-330-3p were associated with patients with GDM treated with diet, but not GDM treated with insulin, compared to controls (30). Hromadnikova et al. (2020) found no difference in miRNA expression profiles between GDM on diet only and GDM on the combination of diet plus pharmacologic therapy, however the pharmacologic group included 17 participants on insulin and 1 participant on metformin (35). Another study by Pheiffer et al. (2018) reported that their participants with GDM were treated with either metformin, insulin, and/or diet, but did not specify any details to characterize the sample or to differentiate results by treatment type (36).
3.5.5 miRNA and health outcome correlations
Three studies described the relationships between miRNAs and related health outcomes (25, 33, 37). MiR-16-5p was positively correlated with insulin resistance (25), 2-hour fasting glucose levels at 24-28 weeks of gestation, and HbA1c (37). There was a positive correlation between miR-17-5p, and miR-20a-5p and insulin resistance, but not with tumor necrosis factor- α (TNF-α) or BMI (25). MiR-29a-3p and miR-134-5p were positively correlated with 2-h fasting glucose levels measured at 24–28 weeks of gestation after adjustment for maternal age and BMI, gestational age, and offspring sex (37). MiR-122-5p was negatively correlated with insulin sensitivity, high density lipoprotein (HDL) cholesterol, and leptin; and positively correlated with birthweight (37). Four miRNAs (miR-4473, miR-199-5a, miR-339-5p, and miR-3653-5p) were positively associated with BMI but were unrelated to GDM (33).
3.6 Risk of bias findings
Risk of bias was assessed in terms of recruitment and sampling methods, confidence in the assessment of exposure, confidence in assessment of the outcomes, matching between cases and controls, potential confounders, and missing data. Most studies described sample recruitment from eligible patients who were seen in a particular clinic. In most studies, the cases and controls were recruited during the same time. However, it is unknown to what extent these cases and controls were drawn from same population, and unknown how representative the samples are of the general population since sample characteristics were described inconsistently.
Confidence in assessment of exposure was assessed by considering the method of diagnosis of GDM and comorbidities. Variations in the GDM definition, diagnostic criteria referenced, and methods of GDM diagnosis were found across studies, although most were assessed between 24-28 weeks gestation. For example, the American Diabetes Association (ADA) recommends that pregnant people who test positive for diabetes during the first trimester be diagnosed with type 2 diabetes (T2D) (1). However, Lamadrid-Romero et al. (2018) classified those participants as having GDM because they had no prior risk factors for T2D (26). It is unknown to what extent undiagnosed T2D prior to pregnancy affected the results, due to the nature of the GDM diagnostic process.
Confidence in the individual studies’ assessment of the molecular markers was mixed. Most studies provided detailed descriptions of their analysis methods, but across the studies, instrumentation and analysis tools were unstandardized. One transcriptome study reported different results in the abstract versus the body of the manuscript. For the review of literature described in this paper, findings were reported from the body of the manuscript because the text in the results section matched the figures and discussion. Finally, validation of the mRNA and miRNA measurements was used only in a subset of studies and was not consistently applied in all studies.
Matching of exposed and unexposed participants for potential confounders affecting the outcome was done in a minority of studies. As expected, in most studies that measured fasting glucose and HbA1c, those levels were higher in the GDM group than the control group at the time of mRNA or miRNA analysis. BMI was also significantly higher in the GDM group in a majority of studies at the time of the blood draw. Other potential confounders such as pre-pregnancy BMI, weight gain during pregnancy, age of the pregnant person, gestational age at time of blood draw, singleton pregnancy, primiparous, behaviors during pregnancy such as smoking, and other sociocultural factors were recorded or adjusted for inconsistently across studies. It is also unknown if behavioral and environmental exposures were consistent among cases and controls because of limited sample characteristics reported. For example, it is unknown to what extent behaviors like physical activity, diet, smoking, exercise or psychosocial factors like stress, environmental factors, access to resources and prenatal care, health literacy, low socioeconomic status (SES), education, or structural racism resulting from being a member of racial ethnic minority groups, may be affecting results.
Missing data were described in some studies. For example, some studies analyzed different sample sizes for different time points (26), some had missing sample characteristics like age, BMI, or gestational age (24, 32), and some had missing blood samples and analyzed a smaller subset for the mRNA or miRNA results (32).
4 Discussion
This systematic review of the literature identified 16 articles that measured the transcriptome or miRNA expression in blood specimens of 684 adults with GDM compared to 691 healthy controls. Most studies applied an observational, cross-sectional design. Collectively, findings span the entire pregnancy from the first to the third trimester. Additional repeated measures studies are needed to compare mRNA or miRNA levels across gestational ages in the same participants.
The studies represent participants residing in 12 countries, with most set in China and South Africa. There is emerging evidence that differences in geographic location and ethnicity may be reflected in circulating miRNA levels (38, 39). Overall, most of the studies included in our review did not cite standardized definitions of race, ethnicity, and nationality to characterize the samples. To ensure high-quality precision healthcare that is equitable and representative, accurate sample descriptions are needed. Samples of diverse individuals should be recruited to ensure that the findings can be generalizable.
Overall, participants with GDM were slightly older with a significantly higher BMI at the time of the blood draw compared to controls. Few studies utilized either matching in their study design or controlled for confounders in their analyses. As age and weight may influence mRNA and miRNA levels, further research is needed to isolate the influence of GDM from other potentially confounding factors.
Three transcriptome studies were found that assessed circulating mRNA levels in pregnant people with GDM compared with healthy controls (22–24). All three studies represent mRNA levels in the third trimester. The transcriptome studies collectively identified 6,289 DEGs, of which 2,702 (43.0%) were described as upregulated in participants with GDM compared to controls, while 2,499 (39.7%) were described as downregulated in GDM compared to controls.
Expression levels of four mRNAs related to circadian clock rhythms were found to be significantly lower in pregnant people with GDM and were significantly correlated with HbA1c levels in one study (22). These four mRNAs were brain and muscle aryl hydrocarbon receptor nuclear translocator-like protein 1 (BMAL1), PER3, peroxisome proliferator activated receptor delta (PPARD), and cryptochrome circadian regulator 2 (CRY2). However, none of the ten circadian clock genes measured by Pappa et al. (2013) were reported in the supplementary results tables of the other two transcriptome studies (22–24). Differentially expressed clock genes are consistent with other studies that showed associations between sleep disruptions/night shift work and GDM (40). Specifically, sleep disruptions in GDM are associated with higher morning blood cortisol and glucose levels, increasing the need for long-acting insulin at night for patients with GDM. Previous studies about the role of circadian genes in T2D have indicated that BMAL1 works in coordination with CLOCK as transcriptional activators of the circadian clock’s self-sustained transcriptional-translational feedback loops (41). These activators function as positive elements driving transcription of PERs, CRYs, and numerous other downstream elements involved in glucose metabolism and postprandial glycemia (41).
We extracted data from the transcriptome articles about the target pathways implicated by their findings and the tools they used to analyze them. Studies used different databases to determine the biological pathways related to their results. For example, Steyn et al. (2019) used the protein analysis through evolutionary relationships (PANTHER) and the database for annotation, visualization and integrated discovery (DAVID) tools (23), while Zhao et al. (2011) used Pathway Express (23, 24).
Collectively, the target pathways identified using these tools described various aspects of diabetes pathogenesis, including insulin and glucose signaling, regulation, and transport; natural killer cell mediated cytotoxicity; NADP and carbohydrate metabolism; immunity and inflammation; fatty acid biosynthesis and metabolism. The transcriptome pathways identified by the findings reflect the multi-system, complex nature of the disease (42). A prior study conducted by Flowers et al. (2022) assessed pathways targeted by differentially expressed miRNAs in people at risk for T2D and identified three themes (i.e., metabolism and inflammation, endocrine, and hormone) (43). Many of the implicated pathways identified by the studies included in this review also fit within these themes. Additional research is needed to validate transcriptome findings related to GDM in larger samples and additional settings and to determine how the pathophysiology of GDM may relate to the pathophysiology that underlies risk for T2D. Standardization is needed for data acquisition and analysis tools.
The miRNA study designs, participant characteristics, gestational age at the time of the miRNA blood analysis, and data analysis tools were disparate. Our analysis revealed that miRNA levels varied based on the time in pregnancy when GDM develops, the trimester or time period at which miRNAs were measured, sex of the fetus, obesity in the pregnant person, and treatment type (diet versus pharmaceutical). We delineated the miRNAs that were found to be significantly different in pregnant people with GDM by first trimester, second trimester, and the gestational age corresponding with GDM diagnosis (24-28 weeks gestation).
In the thirteen miRNA studies, 135 unique miRNAs were associated with GDM. Eight of these circulating miRNAs (miR-16-5p, miR-17-5p, miR-20a-5p, miR-29a-3p, miR-195-5p, miR-222-3p, miR-210-3p, and miR-342-3p) were the most validated for GDM. This list is updated from the previous review by Ibarra et al. (2018) by adding three miRNAs to the list (miR-195-5p, miR-210-3p, and miR-342-3p) and integrating any new findings about the five most-validated miRNAs previously identified (miR-16-5p, miR-17-5p, miR-20a-5p, miR-29a-3p, and miR-222-3p) (44). What is known from previous literature about each of these miRNAs in relation to GDM, other types of diabetes, obesity and weight change, or relevant pregnancy-related outcomes are discussed in more detail below. Animal model study findings are included in those cases where human study findings are unavailable.
4.1 miR-16-5p
MiR-16-5p is a powerful regulator of the insulin signaling pathway, pancreatic β-cell proliferation and apoptosis, and branched chain amino acids involved in insulin dysregulation (45–48). Target genes of miR-16-5p in mouse model and in vitro mechanistic studies include those that encode for insulin receptor substrate (IRS) proteins 1 and 2, the insulin receptor (INSR), and at least 24 other targets in the insulin signaling pathway like ak strain transforming (AKT) protein 1 and 3 (45–48). Other targets include genes encoding for the mammalian target of rapamycin (mTOR) protein and b-cell leukemia/lymphoma 2 protein (BCL-2) expression (45, 48), among others.
Our review found that miR-16-5p was upregulated compared to the control group in 80% (4 out of 5) of the included studies that reported it in their findings (Table 4). It was upregulated prior to onset of GDM in the first trimester (weeks 9-12) (48), during weeks 16-19 (27, 37), and in weeks 24-28 (25, 37, 48). Elevated gestational miR-16-5p may persist beyond pregnancy and may be permanently altered in women with GDM (35). However, in other studies conducted in Turkey, South Africa, and Poland, no significant difference was found in women with GDM compared with controls (36, 48–50). These mixed results may reflect regional environmental or cultural differences in miRNA expression levels, or instrumentation and study design differences, but further research is necessary to validate findings.
MiR-16-5p has been associated with traditional clinical indicators and long- and short-term outcomes. For example, elevated miR-16-5p is correlated with homeostatic model assessment of insulin resistance (HOMA-IR) (47, 48), insulin resistance (25), and cardiovascular disease risk (35), but not with preeclampsia (51). MiR-16-5p may also be associated with pre-pregnancy weight, because it has been reported to be elevated in overweight and obese women before week 20 gestation (37, 48). Further, it is consistently downregulated after surgical weight loss interventions in obese human and animal studies (46). Notably, in our sample of studies, miR-16-5p was not correlated with BMI in women with GDM (25). Future studies may seek to control for pre-pregnancy weight, weight category at the time of the blood draw, or gestational weight changes in their analyses for miR-16-5p to differentiate the effects of obesity from GDM pathology on miR-16-5p levels.
The findings from our review suggest that elevated baseline and first trimester miR-16-5p may be a predictor of late-onset GDM, particularly when combined with other miRNAs associated with GDM (37). These findings suggest that therapies that lower miR-16-5p prior to or early in pregnancy may help to prevent or decreased risk for GDM. However, more studies are needed to validate these findings, to understand regional or cultural differences, and to differentiate the contribution of obesity from pathology unique to GDM.
4.2 miR-17-5p
MiR-17-5p is involved in cell proliferation, inflammation, mitochondrial function, and diabetes-related vascular damage (48, 52). High glucose induction of human trophoblast cells in vitro led to upregulation of miR-17-5p in a simulated diabetic environment (52). There is some evidence that two enzymes associated with mitochondrial function, mitofusins 1 and 2, are targets of miR-17-5p (52).
In our sample, miR-17-5p was upregulated in 75% (3/4) of the studies (25, 27, 35). MiR-17-5p levels were upregulated compared with controls in weeks 16-19 (27) and 3-5 years post-pregnancy (35). During weeks 24-28 at the typical time of GDM diagnosis, expression levels were mixed. One study conducted in a sample of pregnant people in China found miR-17-5p levels to be upregulated (25), while two other studies of samples of South African and Turkish pregnant people, respectively, found no difference (36, 49). These mixed results may reflect cultural and environmental differences between the samples. Like miR-16-5p, miR-17-5p was positively correlated with insulin resistance but not TNF-α or BMI in one study (25). Also, like miR-16-5p, miR-17-5p has been linked to obesity, except that in the obese and coronary artery disease phenotypes the miR-17-5p levels were downregulated compared with healthy controls instead of upregulated as we found in our review (53). Further research is warranted to understand to what extent differences in miRNA expression levels are due to GDM pathology or obesity, and to describe how gestational miR-17-5p levels may change with weight changes.
4.3 miR-195-5p
In mouse and in vitro models, placental miR-195-5p targeted vascular endothelial growth factor A (VEGFA) in placental cells (54), and was an enhancer of zeste homolog 2 (EZH2) in umbilical cells (54), both of which may contribute to endothelial cell dysfunction and GDM progression. In the two studies that were included in our review, miR-195-5p was upregulated in pregnant people with GDM compared with controls in weeks 23-31 (28) and 3-5 years post pregnancy (35). This finding is consistent with previous work by Wang et al. (2020), who also found miR-195-5p to be upregulated at 25 weeks gestation in pregnant people with GDM compared with controls. MiR-195-5p targeted several of the genes important in the fatty acid metabolism pathway, including fatty acid desaturase 2 (FADS2), elongase of very long fatty acid 5, acetyl co-A carboxylase α (ELOVL5), acetyl co-A synthetase 3 and 4 (ACSL3, ACSL4), hydroxyacyl-CoA dehydrogenase trifunctional multienzyme complex subunit α (HADHA), and carnitine palmitoyltransferase 1A genes (CPT1A) (28). This suggests that alterations in lipid metabolism, associated with changes in miR-195-5p expression, may be an important aspect of GDM pathogenesis and an area of focus for future study.
Mechanistic mouse model and in vitro studies about the role of miR-195-5p in GDM suggests that high levels of miR-195-5p inhibits cell proliferation and angiogenesis in human placental microvascular and umbilical endothelial cells treated with a high glucose condition (54, 55). The direction of the effect on cell apoptosis may vary by tissue, as high miR-195-5p inhibited apoptosis in umbilical endothelial cells (55) and increased apoptosis in the placental endothelial cells (54), both of which were undesirable effects contributing to cellular dysfunction. Additional studies are warranted to fully understand the mechanism of miR-195-5p in GDM pathogenesis. Two studies have measured associations between miR-195-5p and body mass and reported no association (35, 56), suggesting that obesity and gestational weight changes may not play a significant role in miR-195-5p levels in GDM. However, due to the connection between miR-195-5p and altered lipid metabolism, further validation of these results is warranted.
4.4 miR-20a-5p
The mechanism of miR-20a-5p’s involvement in GDM is unknown, but miR-20a-5p has been linked to cardiovascular disease, T2D, and pregnancy-related birth outcomes like small for gestational age and fetal growth restriction (57). In a study of participants undergoing coronary angiography, miR-20a-5p was associated with kidney function and estimated glomerular filtration rate after controlling for several confounders including T2D (58). In another study comparing participants with abdominal aortic aneurism with and without T2D, miR-20a-5p was associated with fructosamine concentration (59). MiR-20a-5p levels were significantly upregulated in the group with T2D compared with controls (59).
We found that miR-20a-5p was upregulated in pregnant people with GDM compared with controls in 75% of studies (3/4) in the final sample in this review (25, 27, 35). Levels were downregulated in the other 25% (36). For the studies that showed upregulated expression in pregnant people with GDM compared to controls, two of the studies were done in China (25, 27) and the other from the Czech Republic (35). Expression levels of miR-20a-5p were upregulated compared with controls across gestational periods and beyond—in weeks 16-19 (27), weeks 24-28 (25), and 3-7 years post-pregnancy (35). The average BMI for most study groups (pregnant people with GDM or control) in the three studies that reported upregulation had BMIs in the normal range. In contrast, in a study of South African pregnant people, miR-20a-5p was significantly lower in participants with GDM than participants in the control group in weeks 24-28 (36). The difference in findings may reflect regional or ethnic differences between the samples, but notably, the South African participants were more obese than participants in the other three studies. Previous mouse model studies have linked miR-20a-5p with induction of adipogenesis and lipogenesis via a novel regulatory circuit called CCAAT/enhancer-binding protein α/miR-20a-5p/Transducer Of ERBB2, 2 or TOB2 (60). Yet in the one study in our review that tested correlations between the miRNAs and clinical measures, miR-20a-3p was not correlated with BMI, but it was positively correlated with insulin resistance (25). Further research is warranted to validate previous findings, to understand the relationships between pre-gestational BMI, gestational weight gain or loss, and miR-20a-5p, and to distinguish the influence of obesity versus GDM pathology on miR-20a-5p expression levels.
4.5 miR-210-3p
MiR-210-3p is involved in hypoxia, insulin resistance, and anti-angiogenesis (34). Overexpression of miR-210-3p is predicted to inhibit insulin binding to the insulin receptor protein, the function of AMPK, and ultimately, β-oxidation and glucose transport (34). MiR-210-3p in late pregnancy has been positively associated with gestational age at birth (61). We found that results for miR-210-3p were mixed. Gillet et al. found it to be upregulated in pregnant people with GDM compared with controls (34), while Wander et al. found no difference (32). In one sample, miR-210-3p was associated with GDM in pregnant people with overweight or obesity but not those participants who were lean (32).
4.6 miR-222-3p
The role of miR-222-3p in GDM is unknown, but previous mechanistic studies implicate circulating miR-222-3p levels in both obesity and T2D (62–65). MiR-222-3p targets at least three experimentally validated genes of which low levels have been associated with T2D pathology: O-6-Methylguanine-DNA Methyltransferase (MGMT), Serine/threonine-protein phosphatase 2A 55 kDa regulatory subunit B-α isoform (PPP2R2A), and Reversion Inducing Cysteine Rich Protein With Kazal Motifs (RECK) (62, 63). In a mouse model mechanistic study, miR-222-3p also mediated the therapeutic effects pioglitazone, an oral hypoglycemic drug used for T2D, on skeletal muscle tissue, independent of the PPARγ mechanism-of-action of the drug (66).
In mouse models of obesity, overexpression of miR-222-3p was associated with increased adiposity by targeting DNA damage inducible transcript 4 (Ddit4) in adipocyte specific miR-221/222 knockout, which was associated with the suppression of the tuberous sclerosis complex 2 (TSC2)/mTOR complex 1 (mTORC1)/ribosomal protein S6 kinase (S6K) pathway (65). Circulating levels of miR-222-3p have been downregulated post-bariatric surgery induced weight loss in at least two studies (67). Paradoxically, however, circulating levels of miR-222-3p increased rather than decreased in overweight and obese human participants after a diet-induced weight loss intervention (68).
In our systematic review, results for miR-222-3p in pregnant people with GDM compared with controls were mixed, with one each downregulated during weeks 24-28 gestation (36), upregulated between weeks 23-21 gestation (28), and no difference during weeks 7-22.9 gestation (32). These three studies took place in different countries (South Africa, Estonia, and the United States, respectively). Although the difference was significant between the pregnant people with GDM and controls, miR-222-3p was not significantly, independently associated with GDM in a logistic regression model by Pheiffer et al. (36). While these three studies (28, 32, 36) did not describe correlations between miR-222-3p and any clinical parameters or outcomes, a validation study by Filardi et al. (2022) found that miR-222-3p was positively correlated with fasting blood glucose and birth weight in the third trimester in pregnant people with GDM (62). Overall, the highly disparate findings in studies about T2D, obesity, weight loss, and GDM suggest a complex mechanism that warrants further research.
4.7 miR-29a-3p
In our review, miR-29a-3p was the most validated because it was reported in 46% of the articles in our final sample, but results were mixed. MiR-29a-3p was upregulated compared with controls in 50% (3/5 studies) (34, 35, 37) and downregulated in 17% (1/5 studies) (31). Two studies (33%) found no difference (32, 36). Zhao et al. (2011) inferred miR-29a-3p to be a negative regulator of serum glucose because knockdown of miR-29a led to increased mitochondrial phosphoenolpyruvate carboxykinase 2 (PCK2) expression (31). Increased PCK2 could then lead to an increase in glucose levels. However, their study was the only one in our sample in which the levels of miR-29a-3p were lower in pregnant people with GDM than controls, so the collective evidence does not yet support this inference. Further research is warranted to fully understand the mechanism and how it may vary by region or ethnicity.
4.8 miR-342-3p
The synthesized evidence from our review suggest that not only is miR-342-3p linked to complications of diabetes as previously proposed (18, 69), but it also may be an early indicator of risk. In the three studies in our review that described miR-342-3p levels, the miRNA was upregulated in 100% of them, spanning early to mid-pregnancy and several years post-pregnancy in diverse geographic locations (28, 34, 35). These three study samples were comparable in mean BMI by study group. In type 2 diabetes, miR-342-3p is predicted to inhibit GLUT2 and trigger impaired insulin secretion in pancreatic β islet cells (34). It is one of the miRNAs that has been shown to be dysregulated across types of diabetes, including GDM, T1D, T2D (18, 70), and in people with obesity (71, 72). For people with metabolic syndrome at risk for diabetes, miR-324-3p is one of at least 49 miRNAs associated with insulin resistance in at least three studies (73). MiR-342-3p may also operate as a novel epigenetic integrator linking adipogenic homeostasis and angiogenesis (69). As such, it may be a useful early marker of metabolic risk. In mouse models of obesity, SNAP25 was identified as a major target gene of miR-342-3p and the reduced expression of SNAP25 may link to functional impairment hypothalamic neurons and excess of food intake (74). The inhibition of miR-342-3p may be a potential candidate for miRNA-based therapy for obesity (74). Due to miR-342-3p’s strong associations with obesity in animal studies, GDM studies should consider controlling for baseline obesity and gestational weight changes, or to consider differentiating from metabolically healthy people with obesity any potential mechanisms that are unique to GDM pathology.
4.9 Other subgroup analyses and overlap with T1D and T2D
Other subgroup analyses included differences in miRNAs by sex of the fetus or by GDM treatment type. Findings suggest that the sex of the fetus may affect maternal circulating miRNAs, however the two studies in our final sample that measured sex reported mixed results (32, 37). Further studies are warranted to better understand if or how sex of the offspring may influence GDM.
Only two studies in our final sample differentiated findings by GDM treatment type (diet versus diet with pharmacologic therapy) with mixed results. These results are complicated by mixing treatment types (i.e., insulin, metformin) within the group of participants with GDM, such as in Hromadnikova et al. (2020) included one participant on metformin with the 17 other participants who were treated with insulin (35). MiR-330-3p was the only miRNA found in our systematic review to vary by treatment group, with levels significantly higher in the GDM group treated with diet alone rather than diet plus insulin (30). Additional studies are needed to understand how miRNAs may vary within treatment groups, because metformin has been associated with the expression of miRNA levels in people undergoing treatment for insulin-resistant diseases (75). More research is needed to fully understand how metformin influences the expression of miRNAs in pregnant people with GDM.
The gene targets and miRNAs identified in this review mostly differ from those that have been previously emphasized for T1D and T2D, suggesting a distinct pathophysiology for GDM (76–78). Some exceptions are miR-16-5p (43), miR-29a-3p, miR-146a, miR-182, and miR-342-3p as previously mentioned in section 4.7 (18, 31–34, 36, 37, 43, 70, 76, 77, 79, 80). MiR-29a-3p was reported with mixed results in both GDM and T2D studies, with one or more study showing it to be upregulated and one or more showing it to be downregulated compared with controls. MiR-146a was significantly upregulated in both GDM and T2D studies. MiR-182 was reported to have significantly higher levels in GDM studies but was significantly downregulated in studies of T1D and T2D.
4.10 Risk of bias and limitations
The studies in the final sample are at moderate risk of bias due to several reasons, including the cross-sectional design, differences in sample characteristics between groups at the time of the analysis, inconsistencies in the standards used for GDM diagnosis, varying molecular quantitation methods and data analysis tools, and the handling of missing data. An international consensus is needed for GDM definitions and diagnostic criteria. More rigorous methods are needed to ensure that participants did not have pre-existing diabetes prior to pregnancy. We found that exclusion criteria were unstandardized, which adds additional complexity while synthesizing results across studies.
Replication and validation studies are needed before any of the mRNA or miRNA targets can be useful as clinical biomarkers or therapeutic targets. As this field of science continues to develop, efforts to standardize sample characterization, diagnostic guidelines, validation, and data acquisition and analysis tools and methods would strengthen the synthesis of results. Nonetheless, these studies represent the best available knowledge about transcriptional differences in the blood samples of adults with GDM compared to controls. This review was limited in that the search was conducted in only one database and only articles available in English were included in this analysis. Our ability to integrate the transcriptome studies with the miRNAome studies was limited by the findings available within each of the individual articles.
Many of the studies presented truncated findings or made available partial datasets. This limited our ability to search for overlapping gene targets and pathways between the miRNA and mRNA studies. Journals are increasingly requiring authors to make available full datasets with their publications. If applied consistently, this practice will allow for improved synthesis and validation of findings across multiple omics studies in the future.
4.11 Conclusions
Findings from this systematic review contribute new insights into the state of the science on transcriptomics and miRNA expression in blood from adults with GDM compared with healthy controls. Differences in expression of mRNA and miRNA levels were identified by gestational age at the time of the study, sex of the fetus, BMI of the pregnant person, and GDM treatment type. Metabolic pathways identified in these studies reflect the multi-system, complex pathophysiology of GDM. Eight miRNAs were found to be the most validated in the current literature: miR-16-5p, miR-17-5p, miR-20a-5p, miR-29a-3p, miR-195-5p, miR-222-3p, miR-210-3p, and miR-342-3p. With the exception of miR-222-3p, the most-validated miRs were upregulated in adults with GDM compared with controls in a majority of the studies that reported about them. The reasons for differences in the direction of change in the results (upregulated, downregulated, no difference) across studies are unknown, but may be related to confounding effects like maternal obesity, gestational weight changes, geographic or ethnic differences, instrumentation and data analysis differences, and study designs. Additional research, particularly with repeated measurement designs, is warranted to validate and refine the evidence, with an emphasis on standardization of research methods and recruiting diverse samples with sufficient power for subgroup analyses.
Data availability statement
The original contributions presented in the study are included in the article/supplementary material. Further inquiries can be directed to the corresponding author.
Author contributions
All authors reviewed and approved the final version of the manuscript. EF, KL, JC, BA, LJ-P, MM, BP, LR, and KR contributed to the conceptualization of the work and critically revised the manuscript. KL and JC completed the search, data extraction, and data analysis. KL was lead author. KL and LC wrote and formatted the data tables and figures.
Acknowledgments
The authors would like to acknowledge the contributions of Lena Noya who helped with the search.
Conflict of interest
The authors declare that the research was conducted in the absence of any commercial or financial relationships that could be construed as a potential conflict of interest.
Publisher’s note
All claims expressed in this article are solely those of the authors and do not necessarily represent those of their affiliated organizations, or those of the publisher, the editors and the reviewers. Any product that may be evaluated in this article, or claim that may be made by its manufacturer, is not guaranteed or endorsed by the publisher.
References
1. American Diabetes Association. 2. classification and diagnosis of diabetes: Standards of medical care in diabetes-2020. Diabetes Care (2020) 43(Suppl 1):S14–31. doi: 10.2337/dc20-S002
2. Schneider S, Bock C, Wetzel M, Maul H, Loerbroks A. The prevalence of gestational diabetes in advanced economies. J Perinat Med (2012) 40(5):511–20. doi: 10.1515/jpm-2012-0015
3. Järvelä IY, Juutinen J, Koskela P, Hartikainen AL, Kulmala P, Knip M, et al. Gestational diabetes identifies women at risk for permanent type 1 and type 2 diabetes in fertile age: Predictive role of autoantibodies. Diabetes Care (2006) 29(3):607–12. doi: 10.2337/diacare.29.03.06.dc05-1118
4. Atègbo JM, Grissa O, Yessoufou A, Hichami A, Dramane KL, Moutairou K, et al. Modulation of adipokines and cytokines in gestational diabetes and macrosomia. J Clin Endocrinol Metab (2006) 91(10):4137–43. doi: 10.1210/jc.2006-0980
5. Kong L, Nilsson IAK, Gissler M, Lavebratt C. Associations of maternal diabetes and body mass index with offspring birth weight and prematurity. JAMA Pediatr (2019) 173:371–8. doi: 10.1001/jamapediatrics.2018.5541
6. Ornoy A, Becker M, Weinstein-Fudim L, Ergaz Z. Diabetes during pregnancy: a maternal disease complicating the course of pregnancy with long-term deleterious effects on the offspring. a clinical review. Int J Mol Sci (2021) 22(6):2965. doi: 10.3390/ijms22062965
7. Robitaille J, Grant AM. The genetics of gestational diabetes mellitus: evidence for relationship with type 2 diabetes mellitus. Genet Med (2008) 10(4):240–50. doi: 10.1097/GIM.0b013e31816b8710
8. Evangelista AF, Collares CV, Xavier DJ, Macedo C, Manoel-Caetano FS, Rassi DM, et al. Integrative analysis of the transcriptome profiles observed in type 1, type 2 and gestational diabetes mellitus reveals the role of inflammation. BMC Med Genomics (2014) 7:28. doi: 10.1186/1755-8794-7-28
9. Zhao S, Liu M-F. Mechanisms of microRNA-mediated gene regulation. Sci China Ser C: Life Sci (2009) 52:1111–6. doi: 10.1007/s11427-009-0152-y
10. Moen GH, Sommer C, Prasad RB, Sletner L, Groop L, Qvigstad E, et al. Mechanisms in endocrinology: epigenetic modifications and gestational diabetes: a systematic review of published literature. Eur J Endocrinol (2017) 176(5):R247–67. doi: 10.1530/EJE-16-1017
11. Destefanis E, Avşar G, Groza P, Romitelli A, Torrini S, Pir P, et al. A mark of disease: how mRNA modifications shape genetic and acquired pathologies. RNA (2021) 27(4):367–89. doi: 10.1261/rna.077271.120
12. Vasu S, Kumano K, Darden CM, Rahman I, Lawrence MC, Naziruddin B, et al. MicroRNA Signatures as Future Biomarkers for Diagnosis of Diabetes States. Cells (2019) 8(12):1533. doi: 10.3390/cells8121533
13. O'Brien J, Hayder H, Zayed Y, Peng C. Overview of microRNA biogenesis, mechanisms of actions, and circulation. Front Endocrinol (2018) 9:402. doi: 10.3389/fendo.2018.00402
14. Soifer HS, Rossi JJ, Saetrom P. MicroRNAs in disease and potential therapeutic applications. Mol Ther (2007) 15(12):2070–9. doi: 10.1038/sj.mt.6300311
15. Vaishya S, Sarwade RD, Seshadri V. MicroRNA, proteins, and metabolites as novel biomarkers for prediabetes, diabetes, and related complications. Front Endocrinol (2018) 9:180. doi: 10.3389/fendo.2018.00180
16. Yang X, Wu N. MicroRNAs and exosomal microRNAs may be possible targets to investigate in gestational diabetes mellitus. Diabetes Metab Syndr Obes (2022) 15:321–30. doi: 10.2147/DMSO.S330323
17. Flowers E, Kanaya AM, Fukuoka Y, Allen IE, Cooper B, Aouizerat BE. Preliminary evidence supports circulating microRNAs as prognostic biomarkers for type 2 diabetes. Obes Sci Pract (2017) 3(4):446–52. doi: 10.1002/osp4.134
18. Flowers E, Allen IE, Kanaya AM, Aouizerat BE. Circulating MicroRNAs predict glycemic improvement and response to a behavioral intervention. biomark Res (2021) 9(1):65. doi: 10.1186/s40364-021-00317-5
19. Page MJ, McKenzie JE, Bossuyt PM, Boutron I, Hoffmann TC, Mulrow CD, et al. The PRISMA 2020 statement: an updated guideline for reporting systematic reviews. BMJ (2021) 372:n71. doi: 10.1136/bmj.n71
20. Igelström E, Campbell M, Craig P, Katikireddi SV. Cochrane's risk of bias tool for non-randomized studies (ROBINS-I) is frequently misapplied: A methodological systematic review. J Clin Epidemiol (2021) 140:22–32. doi: 10.1016/j.jclinepi.2021.08.022
21. Sterne JAC, Hernán MA, Reeves BC, Savović J, Berkman ND, Viswanathan M, et al. ROBINS-I: a tool for assessing risk of bias in non-randomized studies of interventions. BMJ (2016) 355:i4919. doi: 10.1136/bmj.i4919
22. Pappa KI, Gazouli M, Anastasiou E, Iliodromiti Z, Antsaklis A, Anagnou NP. Circadian clock gene expression is impaired in gestational diabetes mellitus. Gynecol Endocrinol (2013) 29(4):331–5. doi: 10.3109/09513590.2012.743018
23. Steyn A, Crowther NJ, Norris SA, Rabionet R, Estivill X, Ramsay M. Epigenetic modification of the pentose phosphate pathway and the IGF-axis in women with gestational diabetes mellitus. Epigenomics (2019) 11(12):1371–85. doi: 10.2217/epi-2018-0206
24. Zhao YH, Wang DP, Zhang LL, Zhang F, Wang DM, Zhang WY. Genomic expression profiles of blood and placenta reveal significant immune-related pathways and categories in Chinese women with gestational diabetes mellitus. Diabetes Med (2011) 28(2):237–46. doi: 10.1111/j.1464-5491.2010.03140.x
25. Cao YL, Jia YJ, Xing BH, Shi DD, Dong XJ. Plasma microRNA-16-5p, -17-5p and -20a-5p: Novel diagnostic biomarkers for gestational diabetes mellitus. J Obstet Gynaecol (2017) 6:974–81. doi: 10.1111/jog.13317
26. Lamadrid-Romero M, Solís KH, Cruz-Reséndiz MS, Pérez JE, Díaz NF, Flores-Herrera H, et al. Central nervous system development-related microRNAs levels increase in the serum of gestational diabetic women during the first trimester of pregnancy. J Neurosci Res (2018) 130:8–22. doi: 10.1016/j.neures.2017.08.003
27. Zhu Y, Tian F, Li H, Zhou Y, Lu J, Ge Q. Profiling maternal plasma microRNA expression in early pregnancy to predict gestational diabetes mellitus. Int J Gynaecol Obstet (2015) 130(1):49–53. doi: 10.1016/j.ijgo.2015.01.010
28. Tagoma A, Alnek K, Kirss A, Uibo R, Haller-Kikkatalo K. MicroRNA profiling of second trimester maternal plasma shows upregulation of miR-195-5p in patients with gestational diabetes. Gene (2018) 672:137–42. doi: 10.1016/j.gene.2018.06.004
29. Yoffe L, Polsky A, Gilam A, Raff C, Mecacci F, Ognibene A, et al. Early diagnosis of gestational diabetes mellitus using circulating microRNAs. Eur J Endocrinol (2019) 181(5):565–77. doi: 10.1530/EJE-19-0206
30. Pfeiffer S, Sánchez-Lechuga B, Donovan P, Halang L, Prehn J, Campos-Caro A, et al. Circulating miR-330-3p in late pregnancy is associated with pregnancy outcomes among lean women with GDM. Sci Rep (2020) 10(1):908. doi: 10.1038/s41598-020-57838-6
31. Zhao C, Dong J, Jiang T, Shi Z, Yu B, Zhu Y, et al. Early second-trimester serum miRNA profiling predicts gestational diabetes mellitus. PloS One (2011) 6(8):e23925. doi: 10.1371/journal.pone.0023925
32. Wander PL, Boyko EJ, Hevner K, Parikh VJ, Tadesse MG, Sorensen TK, et al. Circulating early- and mid-pregnancy microRNAs and risk of gestational diabetes. Diabetes Res Clin Pract (2017) 132:1–9. doi: 10.1016/j.diabres.2017.07.024
33. Stirm L, Kovárová M, Perschbacher S, Michlmaier R, Fritsche L, Siegel-Axel D, et al. BMI-independent effects of gestational diabetes on human placenta. J Clin Endocrinol Metab (2018) 103(9):3299–309. doi: 10.1210/jc.2018-00397
34. Gillet V, Ouellet A, Stepanov Y, Rodosthenous RS, Croft EK, Brennan K, et al. miRNA profiles in extracellular vesicles from serum early in pregnancies complicated by gestational diabetes mellitus. J Clin Endocrinol Metab (2019) 104(11):5157–69. doi: 10.1210/jc.2018-02693
35. Hromadnikova I, Kotlabova K, Dvorakova L, Krofta L. Evaluation of vascular endothelial function in young and middle-aged women with respect to a history of pregnancy, pregnancy-related complications, classical cardiovascular risk factors, and epigenetics. Int J Mol Sci (2020) 21(2):430. doi: 10.3390/ijms21020430
36. Pheiffer C, Dias S, Rheeder P, Adam S. Decreased expression of circulating miR-20a-5p in south African women with gestational diabetes mellitus. Mol Diagn (2018) 22(3):345–52. doi: 10.1007/s40291-018-0325-0
37. Sørensen AE, van Poppel MNM, Desoye G, Damm P, Simmons D, Jensen DM, et al. The DALI core investigator group. the predictive value of miR-16, -29a and -134 for early identification of gestational diabetes: A nested analysis of the DALI cohort. Cells (2021) 10(1):170. doi: 10.3390/cells10010170
38. Flowers E, Ramírez-Mares JD, Velazquez-Villafaña M, Rangel-Salazar R, Sucher A, Kanaya AM, et al. Circulating microRNAs associated with prediabetes and geographic location in latinos. Int J Diabetes Dev Ctries (2021) 41(4):570–8. doi: 10.1007/s13410-020-00917-1
39. Flowers E, Kanaya AM, Zhang L, Aouizerat BE. The role of racial and ethnic factors in microRNA expression and risk for type 2 diabetes. Front Genet (2022) 13:853633. doi: 10.3389/fgene.2022.853633
40. Weschenfelder F, Lohse K, Lehmann T, Schleußner E, Groten T. Circadian rhythm and gestational diabetes: working conditions, sleeping habits and lifestyle influence insulin dependency during pregnancy. Acta Diabetol (2021) 58(9):1177–86. doi: 10.1007/s00592-021-01708-8
41. Jakubowicz D, Wainstein J, Tsameret S, Landau Z. Role of high energy breakfast “big breakfast diet” in clock gene regulation of postprandial hyperglycemia and weight loss in type 2 diabetes. Nutrients (2021) 13(5):1558. doi: 10.3390/nu13051558
42. Alejandro EU, Mamerto TP, Chung G, Villavieja A, Gaus NL, Morgan E, et al. Gestational diabetes mellitus: A harbinger of the vicious cycle of diabetes. Int J Mol Sci (2020) 21(14):5003. doi: 10.3390/ijms21145003
43. Flowers E, Asam K, Allen IE, Kanaya AM, Aouizerat BE. Co-expressed microRNAs, target genes and pathways related to metabolism, inflammation and endocrine function in individuals at risk for type 2 diabetes. Mol Med Rep (2022) 25(5):156. doi: 10.3892/mmr.2022.12672
44. Ibarra A, Vega-Guedes B, Brito-Casillas Y, Wägner AM. Diabetes in pregnancy and microRNAs: promises and limitations in their clinical application. Noncoding RNA (2018) 4(4):32. doi: 10.3390/ncrna4040032
45. Calimlioglu B, Karagoz K, Sevimoglu T, Kilic E, Gov E, Arga KY. Tissue-specific molecular biomarker signatures of type 2 diabetes: an integrative analysis of transcriptomics and protein-protein interaction data. OMICS (2015) 19(9):563–73. doi: 10.1089/omi.2015.0088
46. Catanzaro G, Filardi T, Sabato C, Vacca A, Migliaccio S, Morano S, et al. Tissue and circulating microRNAs as biomarkers of response to obesity treatment strategies. J Endocrinol Invest (2021) 44(6):1159–74. doi: 10.1007/s40618-020-01453-9
47. Hubal MJ, Nadler EP, Ferrante SC, Barberio MD, Suh JH, Wang J, et al. Circulating adipocyte-derived exosomal microRNAs associated with decreased insulin resistance after gastric bypass. Obes (2017) 25(1):102–10. doi: 10.1002/oby.21709
48. Juchnicka I, Kuźmicki M, Niemira M, Bielska A, Sidorkiewicz I, Zbucka-Krętowska M, et al. miRNAs as predictive factors in early diagnosis of gestational diabetes mellitus. Front Endocrinol (2022) 13:839344. doi: 10.3389/fendo.2022.839344
49. Balci S, Gorur A, Derici Yıldırım D, Cayan F, Tamer L. Expression level of miRNAS in patients with gestational diabetes. Turkish J Biochem (2020) 45(6):825–31. doi: 10.1515/tjb-2019-0157
50. Hocaoglu M, Demirer S, Loclar Karaalp I, Kaynak E, Attar E, Turgut A, et al. Identification of miR-16-5p and miR-155-5p microRNAs differentially expressed in circulating leukocytes of pregnant women with polycystic ovary syndrome and gestational diabetes. Gynecol Endocrinol (2021) 37(3):216–20. doi: 10.1080/09513590.2020.1843620
51. Hocaoglu M, Demirer S, Senturk H, Turgut A, Komurcu-Bayrak E. Differential expression of candidate circulating microRNAs in maternal blood leukocytes of the patients with preeclampsia and gestational diabetes mellitus. Pregnancy Hypertens (2019), 17:5–11. doi: 10.1016/j.preghy.2019.04.004
52. Li J, Liu Z, Wu T, Li S, Sun Y. MicroRNA-17-5p acts as a biomarker and regulates mitochondrial dynamics in trophoblasts and endothelial cells by targeting the mitofusins Mfn1/Mfn2 in gestational diabetes mellitus. Arch Med Sci (2022) . doi: 10.5114/aoms/145778
53. Deiuliis JA. MicroRNAs as regulators of metabolic disease: pathophysiologic significance and emerging role as biomarkers and therapeutics. Int J Obes (2016) 40(1):88–101. doi: 10.1038/ijo.2015.170
54. Zheng H, Yu Z, Wang H, Liu H, Chen X. MicroRNA-195-5p facilitates endothelial dysfunction by inhibiting vascular endothelial growth factor a in gestational diabetes mellitus. Reprod Biol (2022) 22(1):100605. doi: 10.1016/j.repbio.2022.100605
55. Liao X, Zhou Z, Zhang X. Effects of miR−195−5p on cell proliferation and apoptosis in gestational diabetes mellitus via targeting EZH2. Mol Med Rep (2020), 22(2):803–9. doi: 10.3892/mmr.2020.11142
56. Peña-Cano MI, Saucedo R, Morales-Avila E, Valencia J, Zavala-Moha JA, López A. Deregulated microRNAs and adiponectin in postmenopausal women with breast cancer. Gynecol Obstet Invest (2019) 84(4):369–77. doi: 10.1159/000496340
57. Hromadnikova I, Kotlabova K, Krofta L. First-trimester screening for fetal growth restriction and small-for-Gestational-Age pregnancies without preeclampsia using cardiovascular disease-associated MicroRNA biomarkers. Biomedicines (2022) 10(3):718. doi: 10.3390/biomedicines10030718
58. Muendlein A, Geiger K, Leiherer A, Saely CH, Fraunberger P, Drexel H. Evaluation of the associations between circulating microRNAs and kidney function in coronary angiography patients. Am J Physiol Renal Physiol (2020) 318(2):F315–21. doi: 10.1152/ajprenal.00429.2019
59. Lareyre F, Clément M, Moratal C, Loyer X, Jean-Baptiste E, Hassen-Khodja R, et al. Differential micro-RNA expression in diabetic patients with abdominal aortic aneurysm. Biochimie (2020), 162:1–7. doi: 10.1016/j.biochi.2019.03.012
60. Zhou J, Yang J, Wang X, Li M, Li F, Zhu E, et al. A novel regulatory circuit "C/EBPα/miR-20a-5p/TOB2" regulates adipogenesis and lipogenesis. Front Endocrinol (2020) 10:894. doi: 10.3389/fendo.2019.00894
61. Howe CG, Foley HB, Kennedy EM, Eckel SP, Chavez TA, Faham D, et al. Extracellular vesicle microRNA in early versus late pregnancy with birth outcomes in the MADRES study. Epigenetics (2022) 17(3):269–85. doi: 10.1080/15592294.2021.1899887
62. Filardi T, Catanzaro G, Grieco GE, Splendiani E, Trocchianesi S, Santangelo C, et al. Identification and validation of miR-222-3p and miR-409-3p as plasma biomarkers in gestational diabetes mellitus sharing validated target genes involved in metabolic homeostasis. Int J Mol Sci (2022) 23(8):4276. doi: 10.3390/ijms23084276
63. Goldsworthy M, Bai Y, Li CM, Ge H, Lamas E, Hilton H, et al. Haploinsufficiency of the insulin receptor in the presence of a splice-site mutation in ppp2r2a results in a novel digenic mouse model of type 2 diabetes. Diabetes (2016), 65(5):1434–46. doi: 10.2337/db15-1276
64. Villard A, Marchand L, Thivolet C, Rome S. Diagnostic value of cell-free circulating microRNAs for obesity and type 2 diabetes: A meta-analysis. J Mol biomark Diagn (2015) 6(6):251. doi: 10.4172/2155-9929.1000251
65. Yamaguchi S, Zhang D, Katayama A, Kurooka N, Sugawara R, Albuayjan HHH, et al. Adipocyte-specific inhibition of mir221/222 ameliorates diet-induced obesity through targeting ddit4. Front Endocrinol (2022) 12:750261. doi: 10.3389/fendo.2021.750261
66. de Mendonça M, de Sousa É, da Paixão AO, Araújo Dos Santos B, Roveratti Spagnol A, Murata GM, et al. MicroRNA miR-222 mediates pioglitazone beneficial effects on skeletal muscle of diet-induced obese mice. Mol Cell Endocrinol (2020) 501:110661. doi: 10.1016/j.mce.2019.110661
67. Langi G, Szczerbinski L, Kretowski A. Meta-analysis of differential miRNA expression after bariatric surgery. J Clin Med (2019), 8(8):1220. doi: 10.3390/jcm8081220
68. Hess AL, Larsen LH, Udesen PB, Sanz Y, Larsen TM, Dalgaard LT. Levels of circulating miR-122 are associated with weight loss and metabolic syndrome. Obesity (2020), 28(3):493–501. doi: 10.1002/oby.22704
69. Cheng S, Cui Y, Fan L, Mu X, Hua Y. T2DM inhibition of endothelial miR-342-3p facilitates angiogenic dysfunction via repression of FGF11 signaling. Biochem Biophys Res Commun (2018) 503(1):71–8. doi: 10.1016/j.bbrc.2018.05.179
70. Collares CV, Evangelista AF, Xavier DJ, Rassi DM, Arns T, Foss-Freitas MC, et al. Identifying common and specific microRNAs expressed in peripheral blood mononuclear cell of type 1, type 2, and gestational diabetes mellitus patients. BMC Res Notes (2013) 6:491. doi: 10.1186/1756-0500-6-491
71. Chartoumpekis DV, Zaravinos A, Ziros PG, Iskrenova RP, Psyrogiannis AI, Kyriazopoulou VE, et al. Differential expression of microRNAs in adipose tissue after long-term high-fat diet-induced obesity in mice. PloS One (2012) 7(4):e34872. doi: 10.1371/journal.pone.0034872
72. Wang L, Xu L, Xu M, Liu G, Xing J, Sun C, et al. Obesity-associated miR-342-3p promotes adipogenesis of mesenchymal stem cells by suppressing CtBP2 and releasing C/EBPα from CtBP2 binding. Cell Physiol Biochem (2015) 35(6):2285–98. doi: 10.1159/000374032
73. Solís-Toro D, Mosquera Escudero M, García-Perdomo HA. Association between circulating microRNAs and the metabolic syndrome in adult populations: A systematic review. Diabetes Metab Syndr (2022) 16(1):102376. doi: 10.1016/j.dsx.2021.102376
74. Zhang D, Yamaguchi S, Zhang X, Yang B, Kurooka N, Sugawara R, et al. Upregulation of mir342 in diet-induced obesity mouse and the hypothalamic appetite control. Front Endocrinol (2021) 12:727915. doi: 10.3389/fendo.2021.727915
75. Alimoradi N, Firouzabadi N, Fatehi R. Metformin and insulin-resistant related diseases: Emphasis on the role of microRNAs. BioMed Pharmacother (2021) 139:111662. doi: 10.1016/j.biopha.2021.111662
76. Mao Y, Mohan R, Zhang S, Tang X. MicroRNAs as pharmacological targets in diabetes. Pharmacol Res (2013) 75:37–47. doi: 10.1016/j.phrs.2013.06.005
77. Mononen N, Lyytikäinen LP, Seppälä I, Mishra PP, Juonala M, Waldenberger M, et al. Whole blood microRNA levels associate with glycemic status and correlate with target mRNAs in pathways important to type 2 diabetes. Sci Rep (2019) 9(1):8887. doi: 10.1038/s41598-019-43793-4
78. Pordzik J, Jakubik D, Jarosz-Popek J, Wicik Z, Eyileten C, De Rosa S, et al. Significance of circulating microRNAs in diabetes mellitus type 2 and platelet reactivity: bioinformatic analysis and review. Cardiovasc Diabetol (2019) 18:113. doi: 10.1186/s12933-019-0918-x
79. Karolina DS, Armugam A, Tavintharan S, Wong MT, Lim SC, Sum CF, et al. MicroRNA 144 impairs insulin signaling by inhibiting the expression of insulin receptor substrate 1 in type 2 diabetes mellitus. PloS One (2011) 6(8):e22839. doi: 10.1371/journal.pone.0022839
Keywords: mRNA, miRNA, transcriptome, miRNAome, microRNA (miR), gestational diabetes (GDM), differential gene expression (DGE), metabolic pathways
Citation: Lewis KA, Chang L, Cheung J, Aouizerat BE, Jelliffe-Pawlowski LL, McLemore MR, Piening B, Rand L, Ryckman KK and Flowers E (2023) Systematic review of transcriptome and microRNAome associations with gestational diabetes mellitus. Front. Endocrinol. 13:971354. doi: 10.3389/fendo.2022.971354
Received: 17 June 2022; Accepted: 20 December 2022;
Published: 10 January 2023.
Edited by:
Carlos Guillén, Department of Biochemistry and Molecular Biology, Complutense University, SpainReviewed by:
Jacopo Sabbatinelli, Università Politecnica delle Marche, ItalyAlessandro Rolfo, University of Turin, Italy
Vernon Dolinsky, University of Manitoba, Canada
Copyright © 2023 Lewis, Chang, Cheung, Aouizerat, Jelliffe-Pawlowski, McLemore, Piening, Rand, Ryckman and Flowers. This is an open-access article distributed under the terms of the Creative Commons Attribution License (CC BY). The use, distribution or reproduction in other forums is permitted, provided the original author(s) and the copyright owner(s) are credited and that the original publication in this journal is cited, in accordance with accepted academic practice. No use, distribution or reproduction is permitted which does not comply with these terms.
*Correspondence: Kimberly A. Lewis, kimberly.lewis@ucsf.edu