- 1Department of Breast and Thyroid Surgery, Second Affiliated Hospital of Chongqing Medical University, Chongqing, China
- 2Scientific Research and Education Section, Chongqing Health Center for Women and Children, Chongqing, China
Objectives: To determine the predictors of axillary lymph node metastasis (ALNM), two nomogram models were constructed to accurately predict the status of axillary lymph nodes (ALNs), mainly high nodal tumour burden (HNTB, > 2 positive lymph nodes), low nodal tumour burden (LNTB, 1-2 positive lymph nodes) and negative ALNM (N0). Accordingly, more appropriate treatment strategies for breast cancer patients without clinical ALNM (cN0) could be selected.
Methods: From 2010 to 2015, a total of 6314 patients with invasive breast cancer (cN0) were diagnosed in the Surveillance, Epidemiology, and End Results (SEER) database and randomly assigned to the training and internal validation groups at a ratio of 3:1. As the external validation group, data from 503 breast cancer patients (cN0) who underwent axillary lymph node dissection (ALND) at the Second Affiliated Hospital of Chongqing Medical University between January 2011 and December 2020 were collected. The predictive factors determined by univariate and multivariate logistic regression analyses were used to construct the nomograms. Receiver operating characteristic (ROC) curves and calibration plots were used to assess the prediction models’ discrimination and calibration.
Results: Univariate analysis and multivariate logistic regression analyses showed that tumour size, primary site, molecular subtype and grade were independent predictors of both ALNM and HNTB. Moreover, histologic type and age were independent predictors of ALNM and HNTB, respectively. Integrating these independent predictors, two nomograms were successfully developed to accurately predict the status of ALN. For nomogram 1 (prediction of ALNM), the areas under the receiver operating characteristic (ROC) curve in the training, internal validation and external validation groups were 0.715, 0.688 and 0.876, respectively. For nomogram 2 (prediction of HNTB), the areas under the ROC curve in the training, internal validation and external validation groups were 0.842, 0.823 and 0.862. The above results showed a satisfactory performance.
Conclusion: We established two nomogram models to predict the status of ALNs (N0, 1-2 positive ALNs or >2 positive ALNs) for breast cancer patients (cN0). They were well verified in further internal and external groups. The nomograms can help doctors make more accurate treatment plans, and avoid unnecessary surgical trauma.
Introduction
Breast cancer remains one of the most lethal diseases that threatens women’s health, and its incidence has ranked first among all tumours in 2020 (1, 2). Although breast cancer relies on systemic treatment options, the primary means for most early-stage breast cancer patients remains surgical intervention (3). Minimally invasive breast surgery, such as breast-conserving surgery and sentinel lymph node biopsy (SLNB), has become more common (4, 5). Axillary lymph node metastasis (ALNM) is one of the most crucial factors affecting locoregional recurrence (LRR) and overall survival (6). The 5-year overall survival rate of breast cancer patients without ALNM is as high as 99%, but it decreases to 85.8% for ALNM patients (7). Therefore, accurate assessment of axillary lymph node status is essential for optimal treatment of breast cancer. Although conventional ultrasound and MRI provide great help for the preoperative evaluation of axillary lymph node status, there are still many uncertainties, especially for small lymph nodes. Valente et al. and Hwang et al. found that the false negative rates (FNR) of predicting axillary lymph node positivity by axillary ultrasound were as high as 16.7% and 22.9%, respectively (8, 9).
Given that axillary lymph node dissection (ALND) can lead to a variety of surgical complications, including postoperative upper limb oedema, pain, numbness, and dysfunction (10, 11), the application of SLNB reduces the complications of ALND to a large extent without compromising staging, survival, or local-regional recurrence (12, 13). Although SLNB is less invasive than ALND, approximately 25% of patients still have short-term complications such as lymphedema and pain, which leads to a significant decline in quality of life (14, 15). At present, the method recommended in the guidelines is still the combined method (nuclide tracer and dye) (16). However, dye-related life-threatening anaphylactic reactions occur in approximately 0.25% to 0.5% of patients (17), and the nuclide tracer causes radioactive exposure to both patients and surgeons.
As a consensus, no further axillary surgery is required in cases of negative sentinel lymph nodes (SLNs) (18). However, whether to perform ALND is still controversial in cases of low nodal tumour burden (LNTB, 1-2 positive lymph nodes). The International Breast Cancer Study Group (IBCSG) 23–01 trials concluded that ALND should be avoided for patients with one or more micro-metastatic (≤ 2 mm) SLNs and tumours ≤ 5 cm; as long as patients receive traditional whole-breast radiotherapy and systemic adjuvant therapy, there is no adverse effect on survival (19). The American College of Surgeons Oncology Group (ACOSOG) Z0011 trial demonstrated that patients (T1-2 and cN0) with 1-2 positive SLNs were exempt from ALND by breast-conserving surgery with subsequent radiotherapy and adjuvant therapy (20, 21). While SLNB is the current standard for axillary staging in breast cancer patients (cN0), SLN is negative in approximately 76% of patients (22, 23). Therefore, if accurate preoperative prediction of ALNs can be achieved, SLNB for lymph node-negative patients or SLNB + ALND for LNTB patients would become unnecessary. For high nodal tumour burden patients (HNTB, > 2 positive lymph nodes), it would be beneficial to the choice of further treatment, including neoadjuvant therapy and surgical procedure.
In summary, accurate prediction of ALN status is helpful for reducing unnecessary ALND and SLNB, which is beneficial for individualized and accurate treatment plans. Many studies have focused on the risk factors for ALNM in breast cancer patients (cN0). Zeng et al. found that grade, estrogen receptor (ER) status and human epidermal growth factor receptor 2 (Her-2) status were significantly correlated with ALNM (24). Hu et al. concluded that a significant association between young age, high BMI, high Ki67 and large tumour size was an independent predictor for ALNM (25). However, the accuracy of the above studies remains uncertain due to insufficient population size.
In this study, we used the Surveillance, Epidemiology, and End Results (SEER) database of the National Cancer Institute to identify the factors that affect ALNM and then constructed nomogram models to predict the probability of ALNM (metastases, LNTB and HNTB or not), with the ultimate goal of helping doctors make appropriate treatment plans preoperatively for patients with breast cancer (cN0).
Material and methods
Data source
The data we analyzed were extracted from two databases. First, training and internal validation groups on demographic characteristics and cancer incidence in the United States population were obtained from the SEER Program (https://seer.cancer.gov/). Second, the external validation group was from the Second Affiliated Hospital of Chongqing Medical University.
Patient selection
In the SEER Program, we screened female patients diagnosed with invasive breast cancer (ICD-O-3 codes 8500, 8480/3, 8401/3, 8510/3, 8520/3, 8521/3, 8522/3-8524/3) between 2010 and 2015. Variables included age, race, laterality, primary site, molecular subtype, ER status, progesterone receptor (PR) status, Her-2 status, grade, tumour size, histologic type, total number of postoperative (ALND) lymph nodes and positive lymph nodes. Inclusion criteria included female invasive breast cancer, cN0, unilateral and single tumour. The exclusion criteria included clinical positive axillary lymph node and distant metastasis, multiple breast cancer lesions, neoadjuvant therapy, coexistence with other tumours, and incomplete medical data.
In the Second Affiliated Hospital of Chongqing Medical University, the data of patients with invasive breast cancer were collected between January 2011 and December 2020. The patients were enrolled in this study according to the inclusion and exclusion criteria above.
Grouping
According to the National Comprehensive Cancer Network (NCCN) guidelines and the ACOSOG Z0011 trial (20, 21, 26), ALND is not routinely recommended for patients with 1-2 lymph node metastases for their low lymph node tumor burden. Hence, the patients enrolled in this study were divided into three groups: 1. N0 (without any axillary lymph node metastasis); 2. LNTB (1-2 axillary lymph node metastasis); 3. HNTB (> 2 axillary lymph node metastasis).
Statistical analysis
After screening by inclusion and exclusion criteria, 6314 patients from the SEER database and 503 patients from the Second Affiliated Hospital of Chongqing Medical University were ultimately included in this study. The patients from the SEER database were randomized 3:1 to the training and internal validation groups for the construction and verification of the nomogram, respectively.
SPSS 26.0 software was used for analysis. In univariate analysis, the chi-square test was used for the comparison of categorical variables. Multivariate regression analysis was used to identify the independent predictors in patients. The rms package for R software (Version 4.1.0; https://www.r-project.org) was used to establish nomograms based on the significantly independent predictors. Then, two validation groups were used for internal and external verification. The receiver operating characteristic (ROC) curves of the prediction model and the verification groups were drawn, and the area under the curve (AUC) was calculated to evaluate the discrimination of the prediction models. Two calibration curves were plotted to assess the nomogram prediction ability via the comparison between predictive and actual ALN status. P < 0.05 was considered statistically significant.
Results
Patients’ characteristics
From 2010 to 2015, 6314 patients in the SEER database were included, and 503 patients in the Second Affiliated Hospital of Chongqing Medical University were included from January 2011 to December 2020. The SEER data were divided into two groups: the training group (n = 4751) and the internal validation group (n = 1563). Data from the Second Affiliated Hospital of Chongqing Medical University were used as the external validation group (n = 503). The specific clinicopathological features of the patients in the training and validation groups are summarized in Table 1. In the training group, ALNM was 49.1% (2332/4751), LNTB was 41.3% (1961/4751), and HNTB was 7.8% (371/4751). Approximately 47.0% (734/1563, including 38.9% LNTB and 8.1% HNTB) of patients were identified as having ALNM in the internal validation group, whereas 41.0% (206/503, including 22.3% LNTB and 18.7% HNTB) of patients were identified as having ALNM in the external validation group.
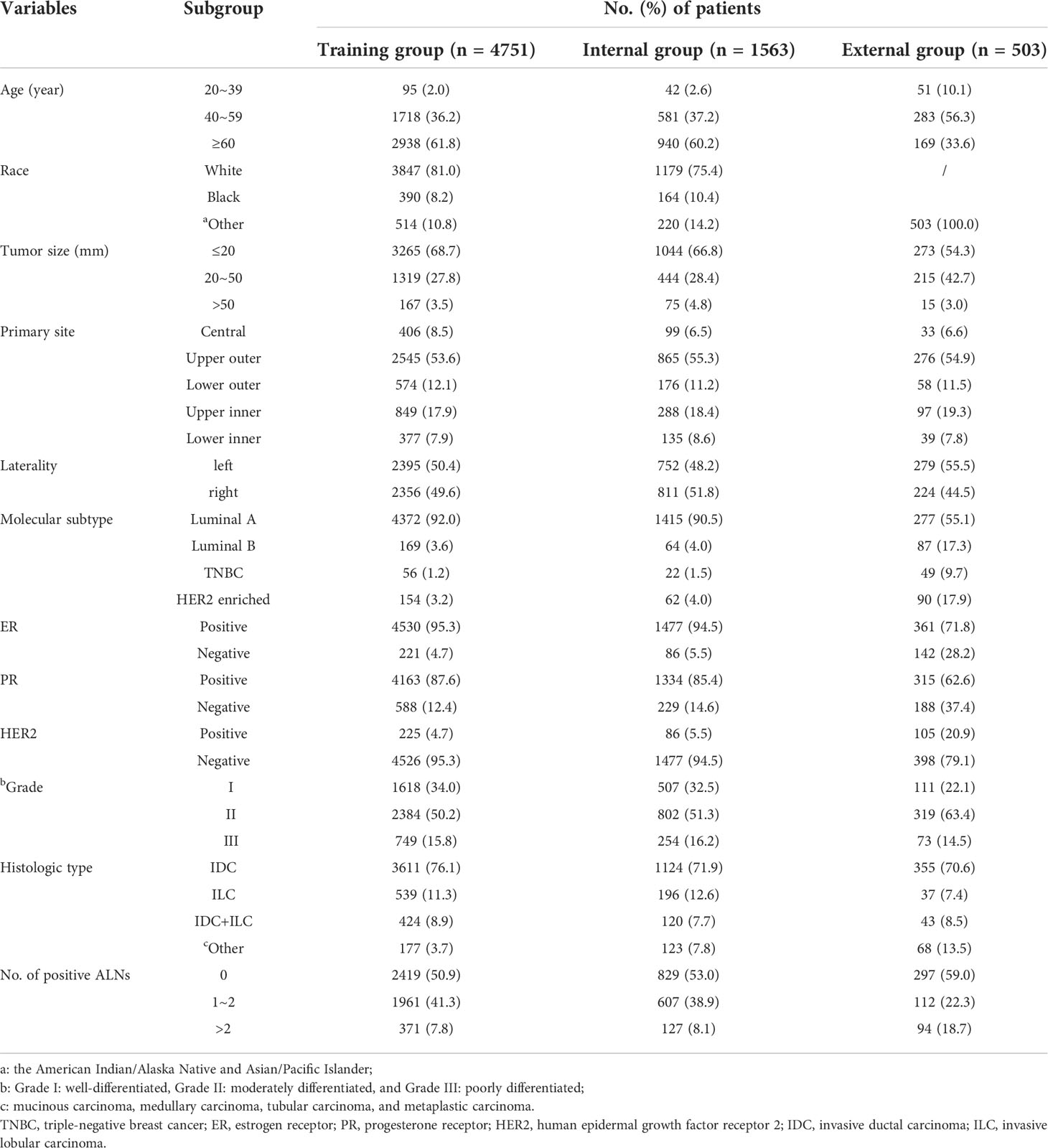
Table 1 Clinicopathological characteristics of patients with breast cancer in SEER Program and our center.
Univariate analysis
Univariate analysis (Table 2) showed that age, race, tumour size, primary site, molecular subtype, histologic type, grade, ER status and PR status (p < 0.05) had statistical significance for ALNM in invasive breast cancer. However, race, Her-2 status and laterality were not statistically significant (P > 0. 05).
Multivariate logistic regression analysis
The above statistically significant factors were included in multivariate logistic regression analysis (Table 3). The results showed that tumour size (20-50 mm, odds ratio (OR) = 3.682, 95% CI: 3.181-4.267; >50 mm, OR = 9.725, 95% CI: 6.240-15.827; p < 0.001), primary site (central, p < 0.001), molecular subtype (Luminal B, OR = 1.127, 95% CI: 0.794-1.609; p < 0.001), grade (II, OR = 1.584, 95% CI: 1.380-1.819; III, OR = 2.311, 95% CI: 1.865-2.868; p<0.001) and histologic type [invasive ductal carcinoma (IDC) + invasive lobular carcinoma (ILC), OR = 1.178, 95% CI: 0.947-1.467; p < 0.001] were independent predictors of ALNM; and age (20-39, p = 0.006), tumour size (20 mm-50 mm, OR = 7.052, 95% CI: 5.628-10.000; > 50 mm, OR = 29.385, 95% CI: 19.613-44.027; p < 0.001), primary site (central, p < 0.001), molecular subtype (Luminal B, OR = 1.328, 95% CI: 0.808-2.122; triple-negative breast cancer (TNBC), OR = 8.097, 95% CI: 1.027-178.441; Her-2 enriched, OR =2.528, 95% CI: 0.337-54.416; p = 0.004) and grade (II, OR = 1.755, 95% CI: 1.243-2.478; III, OR = 3.468, 95% CI: 2.355-5.107; p < 0.001) were independent predictors of HNTB.
Construction and validation of the prediction nomogram
Two nomograms (Figure 1) based on significant and independent predictors determined by multivariate logistic regression analysis were established to predict the ALNM and HNTB of breast cancer patients (cN0). By adding the scores of the corresponding predictors in the respective nomograms, the probability of ALNM and HNTB in a specific patient can be predicted. The effectiveness of the nomograms for predicting ALN status was further evaluated by using ROC curves for the training and validation groups (Figure 2). In the training group, the AUC of ALNM was 0.715 (95% CI: 0.642-0.788), which was similar to the AUCs observed in the internal validation group (0.688, 95% CI: 0.615-0.760) and in the external validation groups (0.876, 95% CI: 0.803-0.948). In another training group, the AUC of HNTB was 0.842 (95% CI: 0.770-0.915), and the AUCs were 0.823 (95% CI: 0.750-0.896) and 0.862 (95% CI: 0.789-0.934) in the corresponding internal validation and external validation groups, respectively. To test the performance of the nomograms, 1,000 bootstrap re-samplings were used for internal verification through calibration charts in each training group (Figure 3). The two calibration curves indicated good calibration effects of the corresponding nomograms.
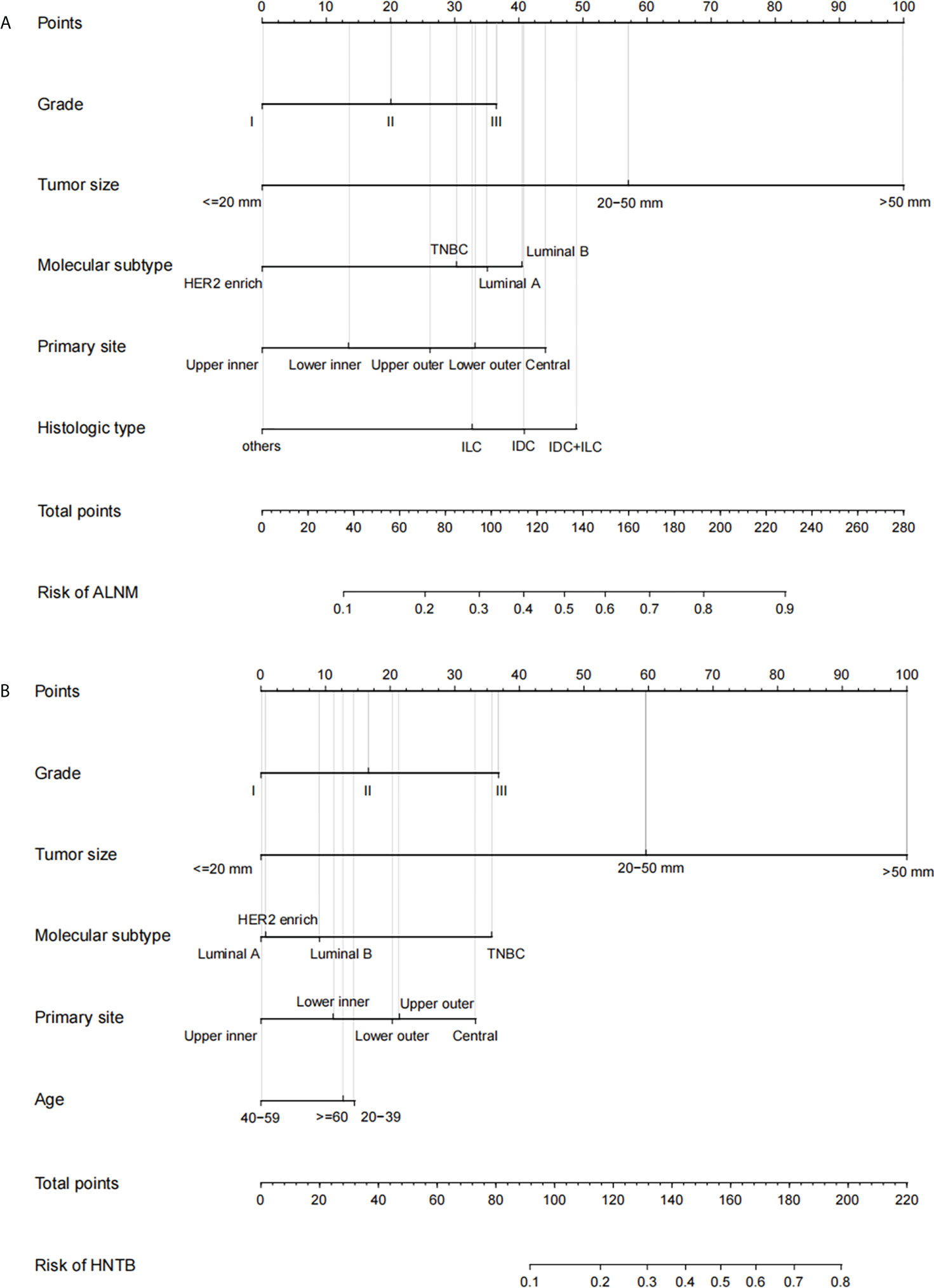
Figure 1 The nomograms of prediction model in breast cancer patients (cN0) Note: (A) the prediction model 1 of axillary lymph node metastasis (ALNM, LN− vs. LN+); (B) the prediction model 2 of high nodal tumor burden [HNTB, LN− and LN+(1–2) vs. LN+(>2)]. LN−, disease-free axillae; LN+: any nodal metastasis; LN+(1–2), 1 or 2 nodal metastasis; LN+(>2), more than 2 nodal metastasis; Grade I, well-differentiated, Grade II, moderately differentiated, and Grade III, poorly differentiated; TNBC, triple-negative breast cancer; IDC, invasive ductal carcinoma; ILC, invasive lobular carcinoma; others: mucinous carcinoma, medullary carcinoma, tubular carcinoma, and metaplastic carcinoma.
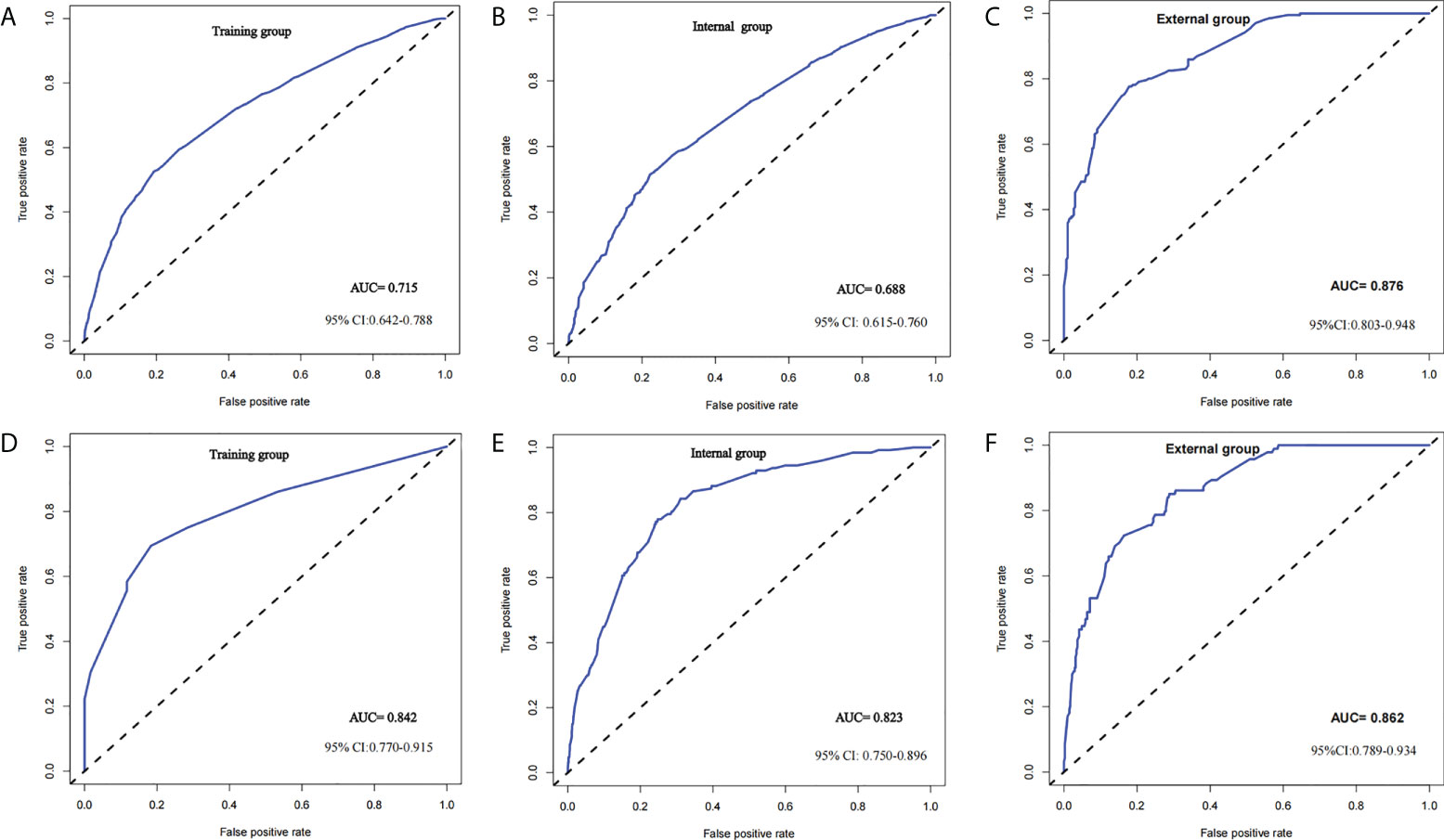
Figure 2 ROC curve of predictive model in breast cancer (cN0) Note: (A–C) ROC curve of predicting ALNM (LN− vs. LN+) in the training group, internal group and external group; (D–F) ROC curve of predicting HNTB [LN− and LN+ (1–2) vs. LN+ (>2)] in the training group, internal group and external group.
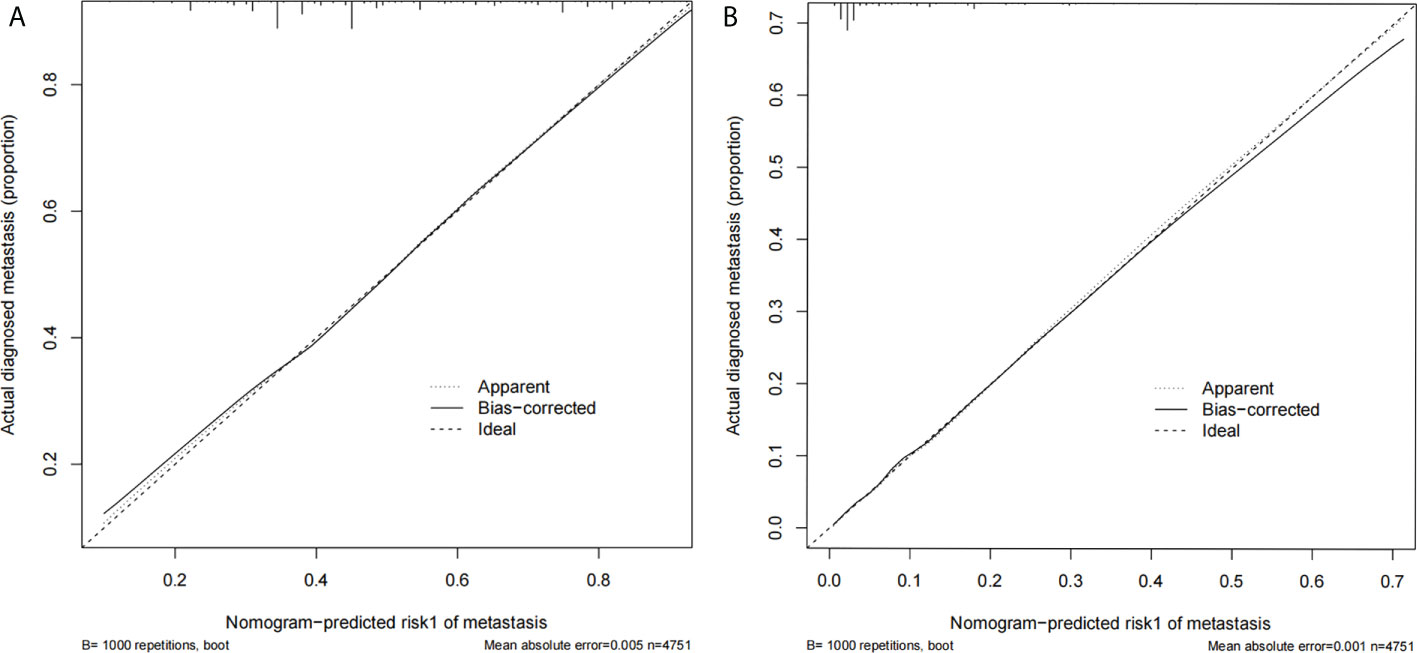
Figure 3 (A) The calibration curve for prediction model 1 of ALNM (LN− vs. LN+); (B) the calibration curve for prediction model 2 of HNTB [LN− and LN+ (1–2) vs. LN+ (>2)].
Discussion
At present, breast cancer is one of the leading diseases that threatens the health of women (2). Although breast cancer relies on comprehensive treatment, including but not limited to surgery, adjuvant chemotherapy, radiotherapy and even immunotherapy, to delay disease progression and improve prognosis, accurate preoperative N staging of breast cancer is of great importance for the selection of an appropriate surgical approach and subsequent individualized comprehensive treatment (26). In particular, the ACOSOG Z0011 trial indicated that a therapeutic strategy for axillary surgery based on the number of positive ALNs became particularly important. Therefore, we established prediction models to accurately predict the status of ALNs.
In this study, the training group was divided into patients without ALNM, 1-2 positive ALNs, and >2 positive ALNs. Our study included 11 clinicopathological features as potential predictors for ALN status in breast cancer. Her-2 status and laterality were associated with ALNM according to the univariate analysis. In contrast, they were not identified as independent factors through multivariate logistic analyses. Finally, by univariate analysis and multivariate logistic regression analysis, we found that tumour size, primary site, molecular subtype and grade were independent predictors of both ALNM and HNTB, which was consistent with the results of previous studies (27–29).
Tumour size was found to be an indisputable independent predictor for ALNM, which was confirmed in many previous studies (30–32). In addition, the consensus was that the larger the tumour is, the greater the likelihood of metastasis to the ALNs. It was concluded that the site of the primary tumour was particularly important for predicting ALN status in this study. In particular, patients with primary tumours located in the central breast are more likely to metastasize to the ALN, which was also consistent with the view of Chen et al. (29, 33). As for histological grade, it is still controversial. Currently, some studies have shown that there is no correlation between grade and ALNM (34, 35). However, in addition to our study, other studies also found that grade II and III cancers were more prone to ALN metastasis than grade I cancers (36, 37).
Moreover, in our study, histologic type and age were independent predictors of ALNM and HNTB, respectively. Tan et al. also showed that non-ductal or lobular histological type (such as mucinous, medullary, tubular or metaplastic carcinoma) compared to invasive ductal or lobular type was associated with a lower risk of ALNM (38, 39). A study showed that compared with older patients, young patients are more likely to have regional lymph node and distant metastasis (40). Other studies have considered age as an independent predictor of ALNM (27–29, 41). We found that HNTB was more likely to occur in young women (age<40).
Finally, based on the above predictors, two different graphical nomograms were developed. The AUCs of the nomograms in ROC curve analysis were 0.715 and 0.842, respectively; internal and external validation showed good discrimination in the prediction of the three ALN statuses. The nomograms can be used to provide a basis for appropriate personalized ALN treatment strategies.
Our study aimed to identify predictors through relevant clinicopathological indicators with a large population size and establish prediction models to reduce unnecessary invasiveness by preoperative prediction. First, our study suggested that SLNB may be avoided in patients with a minimal risk of ALNM if conditions allow (T1-2, cN0, the planned breast-conserving surgery and radiotherapy). Although SLNB is a minimally invasive and well-tolerated surgery, serious allergic reactions and radiation safety caused by tracers of SLNB have been reported (42, 43). Moreover, sentinel lymph node metastasis only exists in 30-35% of patients, and SLNB seems to benefit only a small number of patients (42). More clinical trials are investigating whether SLNB can safely be omitted in breast cancer patients (cN0), such as the SOUND and BOOG 13-08 trials, the results of which are expected in the next few years (44, 45). Second, our study suggested that ALND should be avoided in patients with 1-2 positive SLNs, which is consistent with the prediction result (LNTB). Currently, NCCN guidelines also recommend that for patients with T1 or T2 tumours and 1 to 2 positive SLNs treated with lumpectomy but no preoperative systemic therapy, further axillary surgery could be omitted (26). Finally, we suggested that for predictive HNTB patients (> 2 positive ALNs), who need appropriate neoadjuvant therapy before surgery.
There are several highlights in the study (1): To the best of our knowledge, this is the first internally and externally validated nomogram for accurately stratified prediction of ALN status in female breast cancer patients based on clinicopathological features. Most of the previous studies can only predict ALN status (such as metastasis or no metastasis) or have not been validated (especially external validation), which decreases study credibility (27, 28, 46) (2). Our nomograms can be used extensively with common clinical clinicopathological features, such as tumour size, primary site, grade, age, and molecular subtype, which can be obtained preoperatively (3). This study included a large population size and was validated with external data, which indicated its reliability.
However, this study still has some limitations (1): As a retrospective study, selection or information bias was unavoidable (2). We were unable to obtain additional information from the SEER database, including ultrasound features, oncogenes and more pathological features. If the above information was included, the sensitivity and specificity of the current nomograms would be enhanced (3). The external validation cohort was from a single-center, the number of cases in external validation groups is still significantly less than that in training group (4). Previous study showed that the non-Hispanic black women have a worse prognosis with higher mortality rate (1, 47), it would be a potential predictor for axillary lymph node metastasis. However, the detailed race information wasn’t provided in the SEER database.
Conclusion
In conclusion, we established two nomograms based on common clinical pathology features, including tumour size, primary site, molecular subtype, grade, histologic type and age, to accurately predict the risk of preoperative ALNM and HNTB in breast cancer patients (cN0). The quantitative risk assessment and prediction provided by the nomograms can help doctors avoid additional invasive treatment and provide a new individual strategy for the management of breast cancer patients (cN0).
Data availability statement
The original contributions presented in the study are included in the article/supplementary material. Further inquiries can be directed to the corresponding author.
Ethics statement
The studies involving human participants were reviewed and approved by Ethics Committee of the Second Affiliated Hospital of Chongqing Medical University. Written informed consent for participation was not required for this study in accordance with the national legislation and the institutional requirements.
Author contributions
LY and XG designed the study and wrote the main manuscript text. XG and WL provided the study materials or patients and analyzed all data. LH and LY revised the manuscript. All authors read and approved the final manuscript.
Funding
This study was supported by Chongqing Natural Science Foundation (grant no. cstc2020jcyj-msxmX2011), Science and Technology Bureau of Yuzhong District, Chongqing (grant no. 20200145), and Kuanren Talents Program of the second affiliated hospital of Chongqing Medical University.
Acknowledgments
We would like to thank SEER for providing open access to the database.
Conflict of interest
The authors declare that the research was conducted in the absence of any commercial or financial relationships that could be construed as a potential conflict of interest.
Publisher’s note
All claims expressed in this article are solely those of the authors and do not necessarily represent those of their affiliated organizations, or those of the publisher, the editors and the reviewers. Any product that may be evaluated in this article, or claim that may be made by its manufacturer, is not guaranteed or endorsed by the publisher.
References
1. Siegel RL, Miller KD, Fuchs HE, Jemal A. Cancer statistics, 2021. CA: Cancer J Clin (2021) 71(1):7–33. doi: 10.3322/caac.21654
2. Sung H, Ferlay J, Siegel RL, Laversanne M, Soerjomataram I, Jemal A, et al. Global cancer statistics 2020: GLOBOCAN estimates of incidence and mortality worldwide for 36 cancers in 185 countries. CA: Cancer J Clin (2021) 71(3):209–49. doi: 10.3322/caac.21660
3. Pasta V, Monti M, Cialini M, Vergine M, Urciuoli P, Iacovelli A, et al. Primitive sarcoma of the breast: new insight on the proper surgical management. J Exp Clin Cancer Res CR (2015) 34(1):72. doi: 10.1186/s13046-015-0190-1
4. McDonald ES, Clark AS, Tchou J, Zhang P, Freedman GM. Clinical diagnosis and management of breast cancer. J Nucl Med Off publication Soc Nucl Med (2016) 57 Suppl 1:9s–16s. doi: 10.2967/jnumed.115.157834
5. Harbeck N, Salem M, Nitz U, Gluz O, Liedtke C. Personalized treatment of early-stage breast cancer: present concepts and future directions. Cancer Treat Rev (2010) 36(8):584–94. doi: 10.1016/j.ctrv.2010.04.007
6. Beenken SW, Urist MM, Zhang Y, Desmond R, Krontiras H, Medina H, et al. Axillary lymph node status, but not tumor size, predicts locoregional recurrence and overall survival after mastectomy for breast cancer. Ann Surg (2003) 237(5):732–8. doi: 10.1097/01.Sla.0000065289.06765.71
7. SEER*Explorer: An interactive website for SEER cancer statistics. Surveillance Research Program, National Cancer Institute (2021). Available at: https://seer.cancer.gov/explorer/.
8. Valente SA, Levine GM, Silverstein MJ, Rayhanabad JA, Weng-Grumley JG, Ji L, et al. Accuracy of predicting axillary lymph node positivity by physical examination, mammography, ultrasonography, and magnetic resonance imaging. Ann Surg Oncol (2012) 19(6):1825–30. doi: 10.1245/s10434-011-2200-7
9. Hwang SO, Lee SW, Kim HJ, Kim WW, Park HY, Jung JH. The comparative study of ultrasonography, contrast-enhanced MRI, and (18)F-FDG PET/CT for detecting axillary lymph node metastasis in T1 breast cancer. J Breast Cancer (2013) 16(3):315–21. doi: 10.4048/jbc.2013.16.3.315
10. Hack TF, Cohen L, Katz J, Robson LS, Goss P. Physical and psychological morbidity after axillary lymph node dissection for breast cancer. J Clin Oncol Off J Am Soc Clin Oncol (1999) 17(1):143–9. doi: 10.1200/jco.1999.17.1.143
11. Follacchio GA, Monteleone F, Anibaldi P, De Vincentis G, Iacobelli S, Merola R, et al. A modified sentinel node and occult lesion localization (SNOLL) technique in non-palpable breast cancer: a pilot study. J Exp Clin Cancer Res CR (2015) 34:113. doi: 10.1186/s13046-015-0230-x
12. Wilson JP, Mattson D, Edge SB. Is there a need for axillary dissection in breast cancer? J Natl Compr Cancer Network JNCCN (2011) 9(2):225–30. doi: 10.6004/jnccn.2011.0017
13. Fisher CS, Margenthaler JA, Hunt KK, Schwartz T. The landmark series: Axillary management in breast cancer. Ann Surg Oncol (2020) 27(3):724–9. doi: 10.1245/s10434-019-08154-5
14. Lucci A, McCall LM, Beitsch PD, Whitworth PW, Reintgen DS, Blumencranz PW, et al. Surgical complications associated with sentinel lymph node dissection (SLND) plus axillary lymph node dissection compared with SLND alone in the American college of surgeons oncology group trial Z0011. J Clin Oncol Off J Am Soc Clin Oncol (2007) 25(24):3657–63. doi: 10.1200/jco.2006.07.4062
15. Rao R, Euhus D, Mayo HG, Balch C. Axillary node interventions in breast cancer: a systematic review. Jama (2013) 310(13):1385–94. doi: 10.1001/jama.2013.277804
16. Krag DN, Julian TB, Harlow SP, Weaver DL, Ashikaga T, Bryant J, et al. NSABP-32: Phase III, randomized trial comparing axillary resection with sentinal lymph node dissection: a description of the trial. Ann Surg Oncol (2004) 11(3 Suppl):208s–10s. doi: 10.1007/bf02523630
17. Barthelmes L, Goyal A, Newcombe RG, McNeill F, Mansel RE. Adverse reactions to patent blue V dye - the NEW START and ALMANAC experience. Eur J Surg Oncol J Eur Soc Surg Oncol Br Assoc Surg Oncol (2010) 36(4):399–403. doi: 10.1016/j.ejso.2009.10.007
18. Caudle AS, Cupp JA, Kuerer HM. Management of axillary disease. Surg Oncol Clinics North America (2014) 23(3):473–86. doi: 10.1016/j.soc.2014.03.007
19. Galimberti V, Cole BF, Zurrida S, Viale G, Luini A, Veronesi P, et al. Axillary dissection versus no axillary dissection in patients with sentinel-node micrometastases (IBCSG 23-01): a phase 3 randomised controlled trial. Lancet Oncol (2013) 14(4):297–305. doi: 10.1016/s1470-2045(13)70035-4
20. Giuliano AE, Hunt KK, Ballman KV, Beitsch PD, Whitworth PW, Blumencranz PW, et al. Axillary dissection vs no axillary dissection in women with invasive breast cancer and sentinel node metastasis: a randomized clinical trial. Jama (2011) 305(6):569–75. doi: 10.1001/jama.2011.90
21. Giuliano AE, Ballman K, McCall L, Beitsch P, Whitworth PW, Blumencranz P, et al. Locoregional recurrence after sentinel lymph node dissection with or without axillary dissection in patients with sentinel lymph node metastases: Long-term follow-up from the American college of surgeons oncology group (Alliance) ACOSOG Z0011 randomized trial. Ann Surg (2016) 264(3):413–20. doi: 10.1097/sla.0000000000001863
22. Voogd AC, Coebergh JW, Repelaer van Driel OJ, Roumen RM, van Beek MW, Vreugdenhil A, et al. The risk of nodal metastases in breast cancer patients with clinically negative lymph nodes: a population-based analysis. Breast Cancer Res Treat (2000) 62(1):63–9. doi: 10.1023/a:1006447825160
23. Krag DN, Anderson SJ, Julian TB, Brown AM, Harlow SP, Ashikaga T, et al. Technical outcomes of sentinel-lymph-node resection and conventional axillary-lymph-node dissection in patients with clinically node-negative breast cancer: results from the NSABP b-32 randomised phase III trial. Lancet Oncol (2007) 8(10):881–8. doi: 10.1016/s1470-2045(07)70278-4
24. Zeng D, Lin HY, Zhang YL, Wu JD, Lin K, Xu Y, et al. A negative binomial regression model for risk estimation of 0-2 axillary lymph node metastases in breast cancer patients. Sci Rep (2020) 10(1):21856. doi: 10.1038/s41598-020-79016-4
25. Hu X, Xue J, Peng S, Yang P, Yang Z, Yang L, et al. Preoperative nomogram for predicting sentinel lymph node metastasis risk in breast cancer: A potential application on omitting sentinel lymph node biopsy. Front Oncol (2021) 11:665240. doi: 10.3389/fonc.2021.665240
26. Gradishar WJ, Anderson BO, Balassanian R, Blair SL, Burstein HJ, Cyr A, et al. NCCN guidelines insights: Breast cancer, version 1.2017. J Natl Compr Cancer Netw JNCCN (2017) 15(4):433–51. doi: 10.6004/jnccn.2017.0044
27. Dihge L, Bendahl PO, Rydén L. Nomograms for preoperative prediction of axillary nodal status in breast cancer. Br J Surg (2017) 104(11):1494–505. doi: 10.1002/bjs.10583
28. Zhao YX, Liu YR, Xie S, Jiang YZ, Shao ZM. A nomogram predicting lymph node metastasis in T1 breast cancer based on the surveillance, epidemiology, and end results program. J Cancer (2019) 10(11):2443–9. doi: 10.7150/jca.30386
29. Chen K, Liu J, Li S, Jacobs L. Development of nomograms to predict axillary lymph node status in breast cancer patients. BMC Cancer (2017) 17(1):561. doi: 10.1186/s12885-017-3535-7
30. Takada K, Kashiwagi S, Asano Y, Goto W, Kouhashi R, Yabumoto A, et al. Prediction of lymph node metastasis by tumor-infiltrating lymphocytes in T1 breast cancer. BMC Cancer (2020) 20(1):598. doi: 10.1186/s12885-020-07101-y
31. Illyes I, Tokes AM, Kovacs A, Szasz AM, Molnar BA, Molnar IA, et al. In breast cancer patients sentinel lymph node metastasis characteristics predict further axillary involvement. Virchows Archiv an Int J Pathol (2014) 465(1):15–24. doi: 10.1007/s00428-014-1579-5
32. Yoshihara E, Smeets A, Laenen A, Reynders A, Soens J, Van Ongeval C, et al. Predictors of axillary lymph node metastases in early breast cancer and their applicability in clinical practice. Breast (Edinburgh Scotland) (2013) 22(3):357–61. doi: 10.1016/j.breast.2012.09.003
33. Desai AA, Hoskin TL, Day CN, Habermann EB, Boughey JC. Effect of primary breast tumor location on axillary nodal positivity. Ann Surg Oncol (2018) 25(10):3011–8. doi: 10.1245/s10434-018-6590-7
34. Yenidunya S, Bayrak R, Haltas H. Predictive value of pathological and immunohistochemical parameters for axillary lymph node metastasis in breast carcinoma. Diagn Pathol (2011) 6:18. doi: 10.1186/1746-1596-6-18
35. La Verde N, Biagioli E, Gerardi C, Cordovana A, Casiraghi C, Floriani I, et al. Role of patient and tumor characteristics in sentinel lymph node metastasis in patients with luminal early breast cancer: an observational study. SpringerPlus (2016) 5:114. doi: 10.1186/s40064-016-1720-9
36. Ding J, Jiang L, Wu W. Predictive value of clinicopathological characteristics for sentinel lymph node metastasis in early breast cancer. Med Sci monitor Int Med J Exp Clin Res (2017) 23:4102–8. doi: 10.12659/msm.902795
37. Tseng HS, Chen LS, Kuo SJ, Chen ST, Wang YF, Chen DR. Tumor characteristics of breast cancer in predicting axillary lymph node metastasis. Med Sci monitor Int Med J Exp Clin Res (2014) 20:1155–61. doi: 10.12659/msm.890491
38. Tan LG, Tan YY, Heng D, Chan MY. Predictors of axillary lymph node metastases in women with early breast cancer in Singapore. Singapore Med J (2005) 46(12):693–7.
39. Capdet J, Martel P, Charitansky H, Lim YK, Ferron G, Battle L, et al. Factors predicting the sentinel node metastases in T1 breast cancer tumor: an analysis of 1416 cases. Eur J Surg Oncol J Eur Soc Surg Oncol Br Assoc Surg Oncol (2009) 35(12):1245–9. doi: 10.1016/j.ejso.2009.06.002
40. Erić I, Petek Erić A, Koprivčić I, Babić M, Pačarić S, Trogrlić B. Independent factors FOR poor prognosis in young patients with stage I-III breast cancer. Acta Clinica Croatica (2020) 59(2):242–51. doi: 10.20471/acc.2020.59.02.07
41. Chen JY, Chen JJ, Yang BL, Liu ZB, Huang XY, Liu GY, et al. Predicting sentinel lymph node metastasis in a Chinese breast cancer population: assessment of an existing nomogram and a new predictive nomogram. Breast Cancer Res Treat (2012) 135(3):839–48. doi: 10.1007/s10549-012-2219-x
42. Viale G, Zurrida S, Maiorano E, Mazzarol G, Pruneri G, Paganelli G, et al. Predicting the status of axillary sentinel lymph nodes in 4351 patients with invasive breast carcinoma treated in a single institution. Cancer (2005) 103(3):492–500. doi: 10.1002/cncr.20809
43. Schwartz GF, Giuliano AE, Veronesi U. Proceedings of the consensus conference on the role of sentinel lymph node biopsy in carcinoma of the breast, April 19-22, 2001, Philadelphia, Pennsylvania. Cancer (2002) 94(10):2542–51. doi: 10.1002/cncr.10539
44. Gentilini O, Veronesi U. Abandoning sentinel lymph node biopsy in early breast cancer? a new trial in progress at the European institute of oncology of Milan (SOUND: Sentinel node vs observation after axillary UltraSouND). Breast (Edinburgh Scotland) (2012) 21(5):678–81. doi: 10.1016/j.breast.2012.06.013
45. van Roozendaal LM, Vane MLG, van Dalen T, van der Hage JA, Strobbe LJA, Boersma LJ, et al. Clinically node negative breast cancer patients undergoing breast conserving therapy, sentinel lymph node procedure versus follow-up: a Dutch randomized controlled multicentre trial (BOOG 2013-08). BMC Cancer (2017) 17(1):459. doi: 10.1186/s12885-017-3443-x
46. Cui X, Zhu H, Huang J. Nomogram for predicting lymph node involvement in triple-negative breast cancer. Front Oncol (2020) 10:608334. doi: 10.3389/fonc.2020.608334
Keywords: breast cancer, axillary lymph node metastasis, predictor, nomogram model, stratified prediction
Citation: Gao X, Luo W, He L and Yang L (2022) Nomogram models for stratified prediction of axillary lymph node metastasis in breast cancer patients (cN0). Front. Endocrinol. 13:967062. doi: 10.3389/fendo.2022.967062
Received: 12 June 2022; Accepted: 04 August 2022;
Published: 30 August 2022.
Edited by:
Cristina Pagano, University of Naples Federico II, ItalyReviewed by:
Valerio D’Orazi, Sapienza University of Rome, ItalyLaura Giacomelli, Sapienza University of Rome, Italy
Copyright © 2022 Gao, Luo, He and Yang. This is an open-access article distributed under the terms of the Creative Commons Attribution License (CC BY). The use, distribution or reproduction in other forums is permitted, provided the original author(s) and the copyright owner(s) are credited and that the original publication in this journal is cited, in accordance with accepted academic practice. No use, distribution or reproduction is permitted which does not comply with these terms.
*Correspondence: Lu Yang, MzAyMTE4QGNxbXUuZWR1LmNu
†These authors have contributed equally to this work