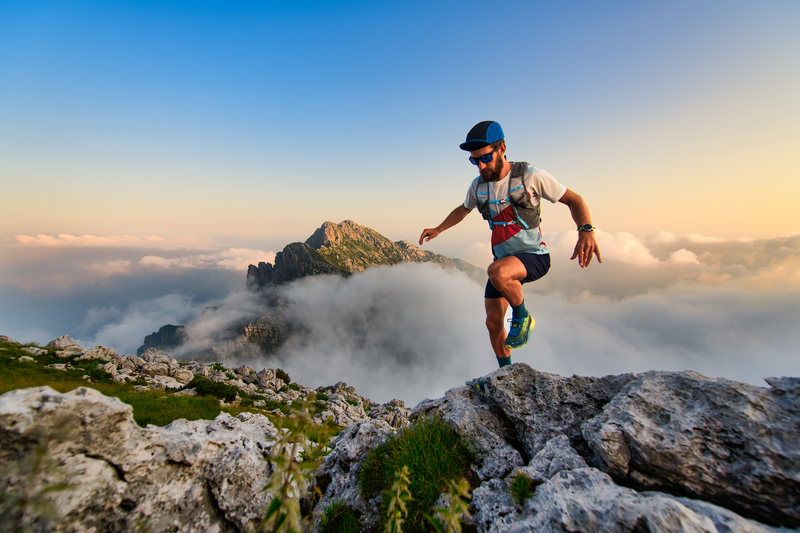
95% of researchers rate our articles as excellent or good
Learn more about the work of our research integrity team to safeguard the quality of each article we publish.
Find out more
ORIGINAL RESEARCH article
Front. Endocrinol. , 17 August 2022
Sec. Cancer Endocrinology
Volume 13 - 2022 | https://doi.org/10.3389/fendo.2022.955250
This article is part of the Research Topic Hormones and Receptors in Breast Cancer View all 9 articles
Background: Pathological complete response (pCR) is considered a surrogate for favorable survival in breast cancer (BC) patients treated with neoadjuvant chemotherapy (NACT), which is the goal of NACT. This study aimed to develop and validate a nomogram for predicting the pCR probability of BC patients after NACT based on the clinicopathological features.
Methods: A retrospective analysis of 527 BC patients treated with NACT between January 2018 and December 2021 from two institutions was conducted. Univariate and multivariate logistic regression analyses were performed to select the most useful predictors from the training cohort (n = 225), and then a nomogram model was developed. The performance of the nomogram was evaluated with respect to its discrimination, calibration, and clinical usefulness. Internal validation and external validation were performed in an independent validation cohort of 96 and 205 consecutive BC patients, respectively.
Results: Among the 18 clinicopathological features, five variables were selected to develop the prediction model, including age, American Joint Committee on Cancer (AJCC) T stage, Ki67 index before NACT, human epidermal growth factor receptor 2 (HER2), and hormone receptor (HR) status. The model showed good discrimination with an area under the receiver operating characteristic curve (AUC) of 0.825 (95% CI, 0.772 to 0.878) in the training cohort, and 0.755 (95% CI, 0.658 to 0.851) and 0.79 (95% CI, 0.724 to 0.856) in the internal and external validation cohorts, respectively. The calibration curve presented good agreement between prediction by nomogram and actual observation, and decision curve analysis (DCA) indicated that the nomogram had good net benefits in clinical scenarios.
Conclusion: This study constructed a validated nomogram based on age, AJCC T stage, Ki67 index before NACT, HER2, and HR status, which could be non-invasively applied to personalize the prediction of pCR in BC patients treated with NACT.
Breast cancer (BC) has become a malignant tumor with the largest number of new cases and deaths in women worldwide, posing a serious threat to women’s health (1). Neoadjuvant chemotherapy (NACT) has been widely used and has become the standard treatment for locally advanced BC (2). It not only can downstage the tumor before surgery and potentially convert an inoperable tumor to be resectable or convert from complete mastectomy to lumpectomy but also can enable the evaluation in vivo for sensitivity to different treatment regimens (3, 4). The pathological response of the primary breast tumor and axillary lymph nodes (LNs) after NACT reflects the sensitivity of the tumor to chemotherapy, which is also significantly associated with the prognosis. Pathological complete response (pCR) includes total pCR with no residual invasive tumor in the breast and LNs, and breast pCR with no residual invasive tumor in the breast only, regardless of the LNs (5, 6). It has been proposed that pCR to the NACT for BC patients is an early surrogate end-point for the prediction of disease-free survival (DFS) and overall survival (OS) (6–8).
However, not all BC patients treated with NACT can achieve pCR. For patients who do not achieve pCR, NACT may cause significant toxicity to the body, which may worsen their prognosis while increasing the cost of treatment (9). Moreover, identification of the pCR prior to surgery can facilitate adjustment of the optimal surgical strategy, especially for the management of the axilla (3). Currently, histopathological examination of the surgically resected specimens remains the gold standard for assessing pathological response after NACT. Although physical examination (PE) and conventional breast imaging such as magnetic resonance imaging (MRI) and ultrasound (US) are the main means to assess the pathological response after NACT, they are not efficient, and their accuracy is not always stable (10). Therefore, it is of great clinical value and significance to develop a safe, efficient, and non-invasive method for personalized prediction of pCR probabilities in BC patients treated with NACT both before NAC and before surgery.
The nomogram was used to assign scores to the level of each factor in the model according to the contribution to the outcome variable (regression coefficient). Then, a function conversion between the total score and the probability of the outcome event was performed, and the predicted probability of a certain clinical outcome was calculated. The nomogram is considered to be a reliable tool to predict the prognosis of cancer patients (11). However, nomograms based on multicenter and with relatively large samples are rarely reported. Thus, this study aims to develop and validate a nomogram for predicting pCR probability of BC patients after NACT based on the clinicopathological features.
This study retrospectively reviewed the data of BC patients treated with NACT at Union Hospital, Tongji Medical College, Huazhong University of Science and Technology, between January 2018 and December 2021. The exclusion criteria in the study were as follows: 1) vital organ was damaged; 2) important clinical parameters were absent or unavailable. A total of 325 BC patients who met the inclusion criteria were included in the primary cohort. Meanwhile, 205 BC patients treated with NACT from January 2018 through December 2021 at the First Affiliated Hospital of Anhui Medical University were screened as an independent external validation cohort. The extracted variables of eligible cases included age, menstrual status, height, weight, chemotherapy regimen, whether chemotherapy is completed (yes or no), and parameters before the start of NACT including peripheral blood albumin level, carbohydrate antigen 125 (CA125), carbohydrate antigen 199 (CA199), carcinoembryonic antigen (CEA), carbohydrate antigen 153 (CA153) levels, platelet count, neutrophil count, lymphocyte count, tumor size and presence of skin or chest wall invasion (yes or no), axillary LN status (positive or negative), Ki67 index, human epidermal growth factor receptor 2 (HER2) status (positive or negative), hormone receptor (HR) status (including estrogen receptor (ER) and progesterone receptor (PR); positive or negative), histological grade (I–III), and pathology-based Miller–Payne (MP) grade (G1–G5) after NACT.
Before NACT, all patients were pathologically confirmed by core needle biopsy (CNB) of the primary breast tumor. All BC biopsy and surgical specimens were processed for histopathology and immunohistochemistry (IHC) and evaluated by two independent pathologists. ER or PR positivity was determined by IHC of at least 1% of the infiltrating tumor cells positive. HER2 positivity was defined as 3+ measured by IHC and/or positive HER2 gene amplification by fluorescence in situ hybridization (FISH) detection; otherwise, they were deemed to be negative. Tumor cell nuclei with a positive background immunostaining score greater than 20% were considered high expressing, and less than 20% were considered low expressing, which was used to discriminate between Luminal A and Luminal B subtypes (12). According to the guideline of the Nottingham Grading System (13), breast tumors were classified into corresponding histological grades. Tumor molecular subtypes were classified as Luminal A, Luminal B/HER2-positive, Luminal B/HER2-negative, HER2-enriched, and triple-negative, based on the IHC and FISH results. The MP grading system (G1–G5) was applied to grade the pathological response of the primary breast tumor after NACT. Grade 5 (G5) indicates no invasive cancer cells in the tumor bed at surgical resection regardless of node status, which was defined as pCR. Correspondingly, pathological reactions classified as grades 1–4 (G1–G4) were considered non-pCR.
The cutoff value for the age group was set at 35 years according to the definition of young BC (14). PLR was defined as the ratio of an absolute number of platelets to lymphocytes in peripheral blood before NACT. Similarly, NLR was defined as the ratio of neutrophils to lymphocytes. Tumor size before NACT was determined by US and/or MRI and was staged according to the eighth edition of the American Joint Committee on Cancer (AJCC). Clinical axillary LN negativity was defined as no abnormal LNs on ultrasound or MRI or confirmed negative by CNB. However, positive axillary LNs must be confirmed by CNB.
The primary cohort consisted of 325 divided into the training and internal validation cohorts with a ratio of 7:3 using the R studio (version 4.0.3, http://www.r-project.org) function ‘caret’ to ensure that outcome events were distributed randomly between the two cohorts. Based on the training cohort, univariate logistic regression was used to preliminarily determine the potential relationship between each parameter and pCR. Subsequently, parameters with a p-value <0.05 in univariate analysis were further analyzed by multivariate logistic regression to select the most useful parameter for predicting pCR. The odds ratio (ORs) and corresponding 95% confidence intervals (CIs) were calculated. According to the regression coefficients of each screened factor in the multivariate analysis, the nomogram was visualized to quantitatively predict the probability of pCR for each BC patient treated with NACT. The internal validation cohort was applied to validate the efficiency and stability of the prediction model. The cohort with 205 BC patients from another institution was used as the external validation cohort to further test the prediction model; hence, it was also called the testing cohort. The flow diagram for developing and validating the prediction model is demonstrated in Figure 1.
Figure 1 The strategy for selecting patients and flow diagram for developing and validating the prediction model.
The performance of the nomogram was evaluated with respect to its discrimination, calibration, and clinical usefulness based on the training cohort, internal validation cohort, and testing cohort. The discrimination and predictive accuracy of the nomogram was quantized to the area under the receiver operating characteristic (ROC) curve (AUC) by the package of ‘pROC’ in R studio. The value of AUC ranged from 0.5 to 1.0, and close to 1.0 meant higher discrimination and accuracy. Typically, a value greater than 0.7 indicates a reasonable estimation (15). Meanwhile, calibration curves based on 1,000 bootstrap resamples were plotted to assess the calibration of the nomogram. It showed the correlation between the predicted and observed probabilities. Therefore, the closer the calibration curve was to the straight line with a slope of 1, the better the predictive ability of the nomogram model. In addition, decision curve analysis (DCA) was applied to quantify the net benefit under different threshold probabilities to evaluate the clinical usefulness of the nomogram.
Continuous variables conforming to normal distribution were presented as the mean and standard deviation (SD) and as the median and interquartile range (IQR) for non-normally distributed. Categorical variables were shown as frequencies and their percentage (%). Student’s t-tests (normally distributed) or Mann–Whitney U test (non-normally distributed) were applied to analyze the statistical difference of continuous variables. Categorical variables were analyzed by Fisher’s exact test or chi-square test. Univariate and multivariate logistic regression analyses were performed to select the most useful predictors from the training cohort. A two-sided p-value <0.05 was considered statistically significant. All statistical analyses and visualization were performed by using R studio statistical software version 4.0.3 (https://www.r-project.org).
This study was exempt from the approval processes of the Institutional Review Boards because no personal information about patients was sought, and their identity would not be revealed in any publication. Written consent for publication was obtained from patients for data about the patients themselves.
The baseline clinicopathological characteristics of BC patients treated with NACT in the primary cohort are concluded in Table 1. Meanwhile, the clinicopathological features of the internal validation and training cohorts are presented in Table 2, which consisted of a random split of the primary cohort in a ratio of 7 to 3. The average age of the population was 48.2 years, of whom 85.2% were aged >35 years and 14.8% were aged ≤35 years, and the mean body mass index (BMI) was 23.2, of whom about 44.9% were postmenopausal and 91.1% completed all chemotherapy cycles. All 325 patients were divided into two groups according to whether pCR was obtained or not. In this cohort, a total of 126 obtained pCR, accounting for 38.8%. Overall, compared with group non-pCR, patients in group pCR had the following significant characteristics: older age, lower CA153 in the peripheral blood, smaller tumor diameter and lower stage, higher Ki67 index, higher proportion of HER2-positive tumors, and lower percentage of HR-positive tumors (all the p-value <0.05). In terms of molecular subtype, HER2-enriched patients have the highest pCR rate, followed by Luminal B/HER2-positive type and Triple-negative type, while Luminal B/HER2-negative type and Luminal A type had the lowest. Additionally, baseline data of 205 patients in the external validation cohort are presented in Table 3. A higher proportion of patients in the pCR group were older than 35 years, although this difference was not statistically significant at a p threshold of less than 0.05. Consistent with the primary cohort, patients receiving pCR in the external validation cohort also showed statistical differences in tumor size, Ki67 index, HER2 status, and HR status as compared with non-pCR patients (all the p-values <0.05). Similarly, patients with subtype HER2-enriched, Luminal B/HER2-positive, and triple-negative obtained higher pCR rates, while patients with subtype Luminal B/HER2-negative and Luminal A had lower rates.
Table 2 Clinicopathological characteristics of BC patients treated with NACT in the training and internal validation cohorts.
Table 3. Clinicopathological characteristics of the test cohort of BC patients treated with NACT in the testing cohort.
As shown in Figure 2, the univariate logistic regression analysis in the training cohort suggested that eight factors including age (p = 0.047), age group (p = 0.026), tumor size (p < 0.001), AJCC_T stage, Ki67 index before NACT (Pre_Ki67, p = 0.011), HER2 status (p < 0.001), HR status (p < 0.001), and molecular subtype were associated with the pCR of NACT. Due to the potential overlap between age and age group, tumor size and AJCC_T stage, molecular subtypes, and markers (Ki67, HER2, and HR), only one of each was further included in the multivariate analysis. Further multivariate logistic regression analysis (Figure 3) showed that five factors including age group (≤35: OR, −1.51; 95% CI, −2.69 to −0.46; p = 0.007), AJCC_T (T2: OR, −1.1; 95% CI, −2.27~0; p = 0.056; T3: OR, −2.89; 95% CI, −4.51 to −1.43; p < 0.001; T4: OR, −2.1; 95% CI, −5.26 to −0.12; p = 0.098), Pre_Ki67 (OR, 1.71; 95% CI, 0.04~3.45; p = 0.048), HER2 status (negative: OR, −1.53; 95% CI, −2.24 to −0.86; p < 0.001), and HR (negative: OR, 1.6; 95% CI, 0.93~2.3; p < 0.001) status were independent predictors for pCR of NACT.
Figure 2 Univariate logistic regression analysis of the clinicopathological parameters for pCR in BC patients treated with NACT using the training cohort. pCR, pathological complete response; BC, breast cancer; NACT, neoadjuvant chemotherapy; BMI, body mass index; PLR, platelet-to-lymphocyte ratio; NLR, neutrophil-to-lymphocyte ratio; AJCC, American Joint Committee on Cancer; LN, lymph node; NACT, neoadjuvant chemotherapy; Pre_Ki67, Ki67 index before NACT; HER2, human epidermal growth factor receptor 2; HR, hormone receptor.
Figure 3 Multivariate logistic regression analysis of the selected clinicopathological parameters for pCR in BC patients treated with NACT using the training cohort. pCR, pathological complete response; BC, breast cancer; NACT, neoadjuvant chemotherapy; AJCC, American Joint Committee on Cancer; Pre_Ki67, Ki67 index before NACT; HER2, human epidermal growth factor receptor 2; HR, hormone receptor.
As shown in Figure 4, using the regression coefficients of multivariate logistic regression models to weight each feature in our models, the nomogram based on the above five independent predictors was developed to quantitatively predict the probability of pCR for each BC patient treated with NACT. Each value of each included variable was assigned a score accordingly on the points scale. The scores for each variable were added to the model to get the total score. The predictions corresponding to the total score contribute to the prediction of the pCR probability of each patient.
Figure 4 Nomogram for predicting probabilities of pCR in BC patients treated with NACT. pCR, pathological complete response; BC, breast cancer; NACT, neoadjuvant chemotherapy; AJCC, American Joint Committee on Cancer; Pre_Ki67, Ki67 index before NACT; HER2, human epidermal growth factor receptor 2; HR, hormone receptor.
The AUC values of the ROC curves were 0.825 (95% CI, 0.772~0.878) in the training cohort and 0.755 (95% CI, 0.658~0.851) in the internal cohort, which were greater than 0.7, reflecting the good accuracy and discrimination of the model (Figures 5A, B). The calibration curve of the nomogram for the probability of pCR showed favorable consistency between prediction and observation in both the training and internal cohorts (Figures 5D, E). The DCA for the nomogram is presented in Figures 5G, H, which indicated that when the threshold probabilities of the training and internal cohorts were in the range of 0%~90% and 0%–55%, respectively, a higher net clinical benefit could be achieved than the hypothetical non-testing or all testing scenarios.
Figure 5 ROC, calibration, and DCA curve of the nomogram prediction models in each cohort. ROC, receiver operating characteristic; AUC, area under the ROC curve; DCA, decision curve analysis. (A, D, G) ROC, calibration, and DCA curve of the nomogram prediction model in the training cohort, respectively. (B, E, H) ROC, calibration, and DCA curve of the nomogram prediction model in the internal validation cohort. (C, F, I) ROC, calibration, and DCA curve of the nomogram prediction model in the testing cohort.
The ROC curve yielded an AUC of 0.79 (95% CI, 0.724 to 0.856) in the external validation cohort (Figure 5C). Consistent with the training and internal validation cohorts, the calibration curve for the testing cohort was also significantly closer to the 45° diagonal, implying a good calibration of the model (Figure 5F). The DCA curve of the nomogram in the testing cohort presented a favorable net benefit when the threshold probability was 0%~50% (Figure 5I).
Predicting the probability of pCR contributes to evaluating the benefit of NACT in newly diagnosed BC patients and assists in selecting the optimal surgical approach before surgery. However, there is still a lack of consensus on imaging-based assessment of pathological response after NACT in BC patients, and pCR cannot be reliably predicted (10, 16). Thus, in this study, 325 patients in one center were randomly divided into the training and validation cohorts in a ratio of 7 to 3, and a nomogram prediction model based on clinicopathological characteristics was constructed and internally validated. Meanwhile, 205 patients from another center were used as the testing cohort to further externally validate the performance of the predictive model.
This study confirmed that HER-2 overexpression, Luminal B HER2+, and triple-negative breast cancer (TNBC) were favorable subtypes to achieve a pCR, while the pCR rates of Luminal B/HER2− and Luminal A type were low, which was consistent with the majority of previously published studies (6, 17–19). One explanation was that luminal BCs were slowly proliferating tumors that were more amenable to local therapy and benefited from longer endocrine therapy, while HER2-amplified BC and TNBC were rapidly proliferating tumors that were sensitive to NACT (20). Therefore, whether in univariate or multivariate logistic analysis, HR status, HER2 status, and Ki67 index before NACT related to molecular subtyping were the independent predictors of pCR. A higher pCR rate could be achieved in patients with negative or low expressed ER, positive or overexpressed HER2, and a higher Ki67 index before NACT. Moreover, a previous study had confirmed that patients with ≤10% ER expression had an extremely high possibility (31.3%) of achieving total pCR; in comparison, the pCR rate of the other patients was only 8.9% by classifying the expression of ER into five levels (21). The index of Ki67 before NACT was considered to reflect the ability of tumor cell proliferation and closely related to the sensitivity to the NACT (22). Thus, the Ki67 index was consistently recognized as an independent predictor of NACT response (23, 24). As for HER2, thanks to the use of trastuzumab and/or pertuzumab, the efficacy of NACT in HER2-positive patients had been greatly improved, which also made HER2 an independent predictor of the efficacy of NACT (25, 26). The pCR rates of the two institutions were 38.8% and 20.5%, respectively, which were consistent with the range reported in the literature (27–29).
The correlations between the tumor size and NACT efficacy have remained controversial. In this study, we concluded that smaller tumor size or lower AJCC T staging was associated with a higher pCR rate, which was consistent with other studies. Goorts et al. made it clear that lower T stages had significantly higher pCR rates than higher T stages, and the clinical tumor stage was the most important predictor of pCR rate after NACT in BC patients (30). Another study confirmed that tumors >5 cm were associated with a lower likelihood of having a pCR following NAC even when accounting for receptor status (31). However, a different view suggested that tumor size on the probability of pCR was not statistically significant in any of the molecular subtypes (32). Another study found a correlation between tumor size and pCR in univariate analysis but disappeared in multivariate analysis (33). The mean age of patients in the pCR group was higher than that of the non-pCR group (49.6 vs. 47.3), and the pCR rate was lower in BC patients younger than 35 years. This result could be explained that younger BC patients in East Asia were characterized by a high prevalence of Luminal A subtype and a low prevalence of basal-like subtype (34, 35). The clinicopathological characteristics and age-specific incidences between East Asian and American women had been proved to be racially different (36). Among American women, the proportion of ER positivity increased gradually with age, while the proportion of HER2+ and TNBC decreased with age (37, 38). The breast tumors in younger women aged <35 were thought to be more aggressive, with larger tumor size, more positive LNs, poorer differentiation, and a lack of HR (34). Additionally, the pathological diagnostic criteria for ER and PR positivity may vary between countries or hospitals (39).
There were several other factors that may affect the efficacy of NACT. Neither PLR nor NLR in this study showed a predictive value for pCR, although the study by Graziano et al. confirmed low levels of both NLR (≤2.42) and PLR (≤104.72), which indicated a status of immune system activation that predict pCR in BC patients treated with NACT (40). It could be interpreted that the levels of inflammatory cells in the body were unstable due to many factors, which made it inaccurate in predicting the efficacy of NACT. There were also reports in the literature that increased tumor-infiltrating lymphocyte (TIL) levels could predict pathological response in all BC subtypes and were associated with a survival benefit in the TNBC and HER2+ but not the luminal-HER2− subtype (41). The value of PLR and NLR in predicting the efficacy of neoadjuvant chemotherapy for other tumors had been widely reported (42, 43). In addition, although the non-PCR group had a higher BMI than the PCR group, which seemed to be consistent with previous reports (44), the difference was not statistically significant. Previous studies have indicated that obesity is an independent prognostic factor of decreased pCR to NACT in BC patients (45, 46). However, Del Prete et al. also reported that obesity does not correlate with pathological responses in their patients’ series (47). Alan et al. concluded that BMI was not a predictive biomarker for pCR, which was similar to the result of our study (48). Furthermore, our study confirmed that tumor makers, axillary LN positive, menstrual status before NACT (49), and albumin levels (50) did not show significant values in predicting PCR, which was consistent with previous studies.
There were several limitations that should be acknowledged. Firstly, due to the variety of regimens and the limited sample size, chemotherapy regimens had not been analyzed, which was an important factor affecting pCR. Secondly, the relatively small sample size and regional variations in the level of medical service may weaken the result. Finally, as a retrospective study, there were inherent selection biases and uncontrollable confounding factors. Therefore, the efficacy of the nomogram needed to be further verified by multicenter large-sample randomized controlled experiments.
This study constructed a validated nomogram based on age, AJCC T stage, Ki67 index before NACT, HER2, and HR status, which can be non-invasively applied to personalize the prediction of pCR in BC patients treated with NACT both before NACT and before surgery. The nomogram has the potential to assist clinicians in screening BC patients for NACT and adjusting the optimal surgical approach for BC patients after NACT.
The raw data supporting the conclusions of this article will be made available by the authors, without undue reservation.
Ethical review and approval was not required for the study on human participants in accordance with the local legislation and institutional requirements. The patients/participants provided their written informed consent to participate in this study.
Conception and design: all authors. Administrative support: XQ, MR, and SZ. Collection and assembly of data: BQ, JY, and JZ. Data analysis and interpretation: BQ, JY, and LH. Manuscript writing: BQ. Critical revision of the manuscript: JZ and SZ. All authors contributed to the article and approved the submitted version.
The authors declare that the research was conducted in the absence of any commercial or financial relationships that could be construed as a potential conflict of interest.
All claims expressed in this article are solely those of the authors and do not necessarily represent those of their affiliated organizations, or those of the publisher, the editors and the reviewers. Any product that may be evaluated in this article, or claim that may be made by its manufacturer, is not guaranteed or endorsed by the publisher.
1. Sung H, Ferlay J, Siegel RL, Laversanne M, Soerjomataram I, Jemal A, et al. Global cancer statistics 2020: GLOBOCAN estimates of incidence and mortality worldwide for 36 cancers in 185 countries. CA: A Cancer J Clin (2021) 71:209–49. doi: 10.3322/caac.21660
2. Korde LA, Somerfield MR, Carey LA, Crews JR, Denduluri N, Hwang ES, et al. Neoadjuvant chemotherapy, endocrine therapy, and targeted therapy for breast cancer: ASCO guideline. J Clin Oncol (2021) 39(13):1485–505. doi: 10.1200/JCO.20.03399
3. Jiang M, Li C-L, Luo X-M, Chuan Z-R, Lv W-Z, Li X, et al. Ultrasound-based deep learning radiomics in the assessment of pathological complete response to neoadjuvant chemotherapy in locally advanced breast cancer. Eur J Cancer (2021) 147:95–105. doi: 10.1016/j.ejca.2021.01.028
4. Derks MGM, van de Velde CJH. Neoadjuvant chemotherapy in breast cancer: more than just downsizing. Lancet Oncol (2018) 19(1):2–3. doi: 10.1016/S1470-2045(17)30914-2
5. Hong J, Rui W, Fei X, Chen X, Shen K. Association of tumor-infiltrating lymphocytes before and after neoadjuvant chemotherapy with pathological complete response and prognosis in patients with breast cancer. Cancer Med (2021) 10(22):7921–33. doi: 10.1002/cam4.4302
6. Cortazar P, Zhang L, Untch M, Mehta K, Costantino JP, Wolmark N, et al. Pathological complete response and long-term clinical benefit in breast cancer: the CTNeoBC pooled analysis. Lancet (2014) 384(9938):164–72. doi: 10.1016/S0140-6736(13)62422-8
7. Broglio KR, Quintana M, Foster M, Olinger M, McGlothlin A, Berry SM, et al. Association of pathologic complete response to neoadjuvant therapy in HER2-positive breast cancer with long-term outcomes: A meta-analysis. JAMA Oncol (2016) 2(6):751–60. doi: 10.1001/jamaoncol.2015.6113
8. Spring L, Greenup R, Niemierko A, Schapira L, Haddad S, Jimenez R, et al. Pathologic complete response after neoadjuvant chemotherapy and long-term outcomes among young women with breast cancer. J Natl Compr Canc Netw (2017) 15(10):1216–23. doi: 10.6004/jnccn.2017.0158
9. Li F, Yang Y, Wei Y, He P, Chen J, Zheng Z, et al. Deep learning-based predictive biomarker of pathological complete response to neoadjuvant chemotherapy from histological images in breast cancer. J Transl Med (2021) 19(1):348. doi: 10.1186/s12967-021-03020-z
10. Fowler AM, Mankoff DA, Joe BN. Imaging neoadjuvant therapy response in breast cancer. Radiology (2017) 285(2):358–75. doi: 10.1148/radiol.2017170180
11. El Sharouni MA, Ahmed T, Varey AHR, Elias SG, Witkamp AJ, Sigurdsson V, et al. Development and validation of nomograms to predict local, regional, and distant recurrence in patients with thin (T1) melanomas. J Clin Oncol (2021) 39(11):1243–52. doi: 10.1200/JCO.20.02446
12. Goldhirsch A, Winer EP, Coates AS, Gelber RD, Piccart-Gebhart M, Thürlimann B, et al. Personalizing the treatment of women with early breast cancer: highlights of the St gallen international expert consensus on the primary therapy of early breast cancer 2013. Ann Oncol (2013) 24(9):2206–23. doi: 10.1093/annonc/mdt303
13. Elston CW, Ellis IO. Pathological prognostic factors in breast cancer. i. the value of histological grade in breast cancer: Experience from a large study with long-term follow-up. Histopathology (1991) 19(5):403–10. doi: 10.1111/j.1365-2559.1991.tb00229.x
14. Cancello G, Maisonneuve P, Rotmensz N, Viale G, Mastropasqua MG, Pruneri G, et al. Prognosis and adjuvant treatment effects in selected breast cancer subtypes of very young women (<35 years) with operable breast cancer. Ann Oncol (2010) 21(10):1974–81. doi: 10.1093/annonc/mdq072
15. Qian B, Qian Y, Hu L, Zhang S, Mei L, Qu X. Prognostic analysis for patients with parathyroid carcinoma: A population-based study. Front Neurosci (2022) 16:784599. doi: 10.3389/fnins.2022.784599
16. Croshaw R, Shapiro-Wright H, Svensson E, Erb K, Julian T. Accuracy of clinical examination, digital mammogram, ultrasound, and MRI in determining postneoadjuvant pathologic tumor response in operable breast cancer patients. Ann Surg Oncol (2011) 18(11):3160–3. doi: 10.1245/s10434-011-1919-5
17. Chou H-H, Kuo W-L, Yu C-C, Tsai H-P, Shen S-C, Chu C-H, et al. Impact of age on pathological complete response and locoregional recurrence in locally advanced breast cancer after neoadjuvant chemotherapy. BioMed J (2019) 42(1):66–74. doi: 10.1016/j.bj.2018.10.007
18. Untch M, Fasching PA, Konecny GE, Hasmüller S, Lebeau A, Kreienberg R, et al. Pathologic complete response after neoadjuvant chemotherapy plus trastuzumab predicts favorable survival in human epidermal growth factor receptor 2-overexpressing breast cancer: Results from the TECHNO trial of the AGO and GBG study groups. J Clin Oncol (2011) 29(25):3351–7. doi: 10.1200/JCO.2010.31.4930
19. Esserman LJ, Berry DA, DeMichele A, Carey L, Davis SE, Buxton M, et al. Pathologic complete response predicts recurrence-free survival more effectively by cancer subset: Results from the I-SPY 1 TRIAL–CALGB 150007/150012, ACRIN 6657. J Clin Oncol (2012) 30(26):3242–9. doi: 10.1200/JCO.2011.39.2779
20. Cui H, Zhao D, Han P, Zhang X, Fan W, Zuo X, et al. Predicting pathological complete response after neoadjuvant chemotherapy in advanced breast cancer by ultrasound and clinicopathological features using a nomogram. Front Oncol (2021) 11:718531. doi: 10.3389/fonc.2021.718531
21. Tang L, Shu X, Tu G. Exploring the influencing factors of the pathologic complete response in estrogen receptor-positive, HER2-negative breast cancer after neoadjuvant chemotherapy: A retrospective study. World J Surg Oncol (2022) 20(1):27. doi: 10.1186/s12957-022-02492-7
22. Ellis MJ, Suman VJ, Hoog J, Goncalves R, Sanati S, Creighton CJ, et al. Ki67 proliferation index as a tool for chemotherapy decisions during and after neoadjuvant aromatase inhibitor treatment of breast cancer: Results from the American college of surgeons oncology group Z1031 trial (Alliance). J Clin Oncol (2017) 35(10):1061–9. doi: 10.1200/JCO.2016.69.4406
23. Zhang J, Gao S, Zheng Q, Kang Y, Li J, Zhang S, et al. A novel model incorporating tumor stiffness, blood flow characteristics, and ki-67 expression to predict responses after neoadjuvant chemotherapy in breast cancer. Front Oncol (2020) 10:603574. doi: 10.3389/fonc.2020.603574
24. Ma Y, Zhang S, Zang L, Li J, Li J, Kang Y, et al. Combination of shear wave elastography and ki-67 index as a novel predictive modality for the pathological response to neoadjuvant chemotherapy in patients with invasive breast cancer. Eur J Cancer (2016) 69:86–101. doi: 10.1016/j.ejca.2016.09.031
25. Hamy AS, Belin L, Bonsang-Kitzis H, Paquet C, Pierga JY, Lerebours F, et al. Pathological complete response and prognosis after neoadjuvant chemotherapy for HER2-positive breast cancers before and after trastuzumab era: Results from a real-life cohort. Br J Cancer (2016) 114(1):44–52. doi: 10.1038/bjc.2015.426
26. Oh DY, Bang YJ. HER2-targeted therapies - a role beyond breast cancer. Nat Rev Clin Oncol (2020) 17(1):33–48. doi: 10.1038/s41571-019-0268-3
27. Sharma P, López-Tarruella S, García-Saenz JA, Ward C, Connor CS, Gómez HL, et al. Efficacy of neoadjuvant carboplatin plus docetaxel in triple-negative breast cancer: Combined analysis of two cohorts. Clin Cancer Res (2017) 23(3):649–57. doi: 10.1158/1078-0432.CCR-16-0162
28. Rastogi P, Anderson SJ, Bear HD, Geyer CE, Kahlenberg MS, Robidoux A, et al. Preoperative chemotherapy: updates of national surgical adjuvant breast and bowel project protocols b-18 and b-27. J Clin Oncol (2008) 26(5):778–85. doi: 10.1200/JCO.2007.15.0235
29. van Nes JG, Putter H, Julien JP, Tubiana-Hulin M, van de Vijver M, Bogaerts J, et al. Preoperative chemotherapy is safe in early breast cancer, even after 10 years of follow-up; Clinical and translational results from the EORTC trial 10902. Breast Cancer Res Treat (2009) 115(1):101–13. doi: 10.1007/s10549-008-0050-1
30. Goorts B, van Nijnatten TJ, de Munck L, Moossdorff M, Heuts EM, de Boer M, et al. Clinical tumor stage is the most important predictor of pathological complete response rate after neoadjuvant chemotherapy in breast cancer patients. Breast Cancer Res Treat (2017) 163(1):83–91. doi: 10.1007/s10549-017-4155-2
31. Livingston-Rosanoff D, Schumacher J, Vande Walle K, Stankowski-Drengler T, Greenberg CC, Neuman H, et al. Does tumor size predict response to neoadjuvant chemotherapy in the modern era of biologically driven treatment? A nationwide study of US breast cancer patients. Clin Breast Cancer (2019) 19(6):e741–e7. doi: 10.1016/j.clbc.2019.05.014
32. Baron P, Beitsch P, Boselli D, Symanowski J, Pellicane JV, Beatty J, et al. Impact of tumor size on probability of pathologic complete response after neoadjuvant chemotherapy. Ann Surg Oncol (2016) 23(5):1522–9. doi: 10.1245/s10434-015-5030-1
33. Kuerer HM, Newman LA, Smith TL, Ames FC, Hunt KK, Dhingra K, et al. Clinical course of breast cancer patients with complete pathologic primary tumor and axillary lymph node response to doxorubicin-based neoadjuvant chemotherapy. J Clin Oncol (1999) 17(2):460–9. doi: 10.1200/JCO.1999.17.2.460
34. Lin CH, Chuang PY, Chiang CJ, Lu YS, Cheng AL, Kuo WH, et al. Distinct clinicopathological features and prognosis of emerging young-female breast cancer in an East Asian country: A nationwide cancer registry-based study. Oncologist (2014) 19(6):583–91. doi: 10.1634/theoncologist.2014-0047
35. Lin CH, Liau JY, Lu YS, Huang CS, Lee WC, Kuo KT, et al. Molecular subtypes of breast cancer emerging in young women in Taiwan: Evidence for more than just westernization as a reason for the disease in Asia. Cancer Epidemiol Biomarkers Prev (2009) 18(6):1807–14. doi: 10.1158/1055-9965.EPI-09-0096
36. Gomez SL, Yao S, Kushi LH, Kurian AW. Is breast cancer in Asian and Asian American women a different disease? J Natl Cancer Inst (2019) 111(12):1243–4. doi: 10.1093/jnci/djz091
37. Lin CH, Yap YS, Lee KH, Im SA, Naito Y, Yeo W, et al. Contrasting epidemiology and clinicopathology of female breast cancer in asians vs the US population. J Natl Cancer Inst (2019) 111(12):1298–306. doi: 10.1093/jnci/djz090
38. Lin CH, Huang RY, Lu TP, Kuo KT, Lo KY, Chen CH, et al. High prevalence of APOA1/C3/A4/A5 alterations in luminal breast cancers among young women in East Asia. NPJ Breast Cancer (2021) 7(1):88. doi: 10.1038/s41523-021-00299-5
39. Howlader N, Altekruse SF, Li CI, Chen VW, Clarke CA, Ries LA, et al. US Incidence of breast cancer subtypes defined by joint hormone receptor and HER2 status. J Natl Cancer Inst (2014) 106(5). doi: 10.1093/jnci/dju055
40. Graziano V, Grassadonia A, Iezzi L, Vici P, Pizzuti L, Barba M, et al. Combination of peripheral neutrophil-to-lymphocyte ratio and platelet-to-lymphocyte ratio is predictive of pathological complete response after neoadjuvant chemotherapy in breast cancer patients. Breast (2019) 44:33–8. doi: 10.1016/j.breast.2018.12.014
41. Denkert C, von Minckwitz G, Darb-Esfahani S, Lederer B, Heppner BI, Weber KE, et al. Tumour-infiltrating lymphocytes and prognosis in different subtypes of breast cancer: A pooled analysis of 3771 patients treated with neoadjuvant therapy. Lancet Oncol (2018) 19(1):40–50. doi: 10.1016/S1470-2045(17)30904-X
42. Zhang X, Gari A, Li M, Chen J, Qu C, Zhang L, et al. Combining serum inflammation indexes at baseline and post treatment could predict pathological efficacy to anti−PD−1 combined with neoadjuvant chemotherapy in esophageal squamous cell carcinoma. J Transl Med (2022) 20(1):61. doi: 10.1186/s12967-022-03252-7
43. Chen L, Chen Y, Zhang L, Xue Y, Zhang S, Li X, et al. In gastric cancer patients receiving neoadjuvant chemotherapy systemic inflammation response index is a useful prognostic indicator. Pathol Oncol Res (2021) 27:1609811. doi: 10.3389/pore.2021.1609811
44. Fontanella C, Lederer B, Gade S, Vanoppen M, Blohmer JU, Costa SD, et al. Impact of body mass index on neoadjuvant treatment outcome: A pooled analysis of eight prospective neoadjuvant breast cancer trials. Breast Cancer Res Treat (2015) 150(1):127–39. doi: 10.1007/s10549-015-3287-5
45. Karatas F, Erdem GU, Sahin S, Aytekin A, Yuce D, Sever AR, et al. Obesity is an independent prognostic factor of decreased pathological complete response to neoadjuvant chemotherapy in breast cancer patients. Breast (2017) 32:237–44. doi: 10.1016/j.breast.2016.05.013
46. Liu YL, Connolly EP, Kalinsky K. Obesity's impact on survival is independent of dose adjustments in neoadjuvant chemotherapy in women with breast cancer. Breast Cancer Res Treat (2018) 168(1):285. doi: 10.1007/s10549-017-4590-0
47. Del Prete S, Caraglia M, Luce A, Montella L, Galizia G, Sperlongano P, et al. Clinical and pathological factors predictive of response to neoadjuvant chemotherapy in breast cancer: A single center experience. Oncol Lett (2019) 18(4):3873–9. doi: 10.3892/ol.2019.10729
48. Alan O, Akin Telli T, Aktas B, Koca S, Ökten IN, Hasanov R, et al. Is insulin resistance a predictor for complete response in breast cancer patients who underwent neoadjuvant treatment? World J Surg Oncol (2020) 18(1):242. doi: 10.1186/s12957-020-02019-y
49. Yan S, Wang W, Zhu B, Pan X, Wu X, Tao W. Construction of nomograms for predicting pathological complete response and tumor shrinkage size in breast cancer. Cancer Manag Res (2020) 12:8313–23. doi: 10.2147/CMAR.S270687
Keywords: breast cancer, BC, neoadjuvant chemotherapy, NACT, pathological complete response, pCR, clinicopathological features, nomogram
Citation: Qian B, Yang J, Zhou J, Hu L, Zhang S, Ren M and Qu X (2022) Individualized model for predicting pathological complete response to neoadjuvant chemotherapy in patients with breast cancer: A multicenter study. Front. Endocrinol. 13:955250. doi: 10.3389/fendo.2022.955250
Received: 28 May 2022; Accepted: 26 July 2022;
Published: 17 August 2022.
Edited by:
Cinthia Rosemblit, CONICET Institute for Biomedical Research (BIOMED), ArgentinaReviewed by:
Xiaolong Shi, Fourth Military Medical University, ChinaCopyright © 2022 Qian, Yang, Zhou, Hu, Zhang, Ren and Qu. This is an open-access article distributed under the terms of the Creative Commons Attribution License (CC BY). The use, distribution or reproduction in other forums is permitted, provided the original author(s) and the copyright owner(s) are credited and that the original publication in this journal is cited, in accordance with accepted academic practice. No use, distribution or reproduction is permitted which does not comply with these terms.
*Correspondence: Xincai Qu, UXV4YzIwMDhAMTI2LmNvbQ==; Min Ren, NDU1NjM5MzAwQHFxLmNvbQ==; Shoupeng Zhang, MjAxM3hoMDkwM0BodXN0LmVkdS5jbg==
†These authors have contributed equally to this work and share first authorship
Disclaimer: All claims expressed in this article are solely those of the authors and do not necessarily represent those of their affiliated organizations, or those of the publisher, the editors and the reviewers. Any product that may be evaluated in this article or claim that may be made by its manufacturer is not guaranteed or endorsed by the publisher.
Research integrity at Frontiers
Learn more about the work of our research integrity team to safeguard the quality of each article we publish.